ABSTRACT
The number of the massive open online courses (MOOCs) around the globe is on the rise. Despite the popularity of MOOCs, they have received less attention from faculty members around the globe compared to other less-traditional and digital education models. MOOCs can be challenging for teachers to use. As such, understanding how to facilitate teachers’ adoption of MOOCs is very important to better promote their use. The aim of this research paper is to investigate the drivers of teachers’ acceptance and use of MOOCs from the perspective of the extended unified theory of acceptance and use of technology (UTAUT2). An online survey was used to collect responses from university faculty in Taiwan. Partial least squares structural equation modeling (PLS-SEM) was utilized for data analysis. The findings reveal that performance expectancy, social influence, facilitating conditions, and price value facilitated teachers’ behavioral intention to adopt MOOCs. Furthermore, facilitating conditions and behavioral intention determined teachers’ adoption of MOOCs. However, effort expectancy and hedonic motivation failed to drive teachers’ adoption of MOOCs. Based on the findings, several important theoretical and practical implications are discussed.
1. Introduction
Advances in information and communication technology have impacted people around the globe; this extends to the educational context. Educators and researchers are interested in how technology can help improve the effectiveness and efficiency of education, and thereby facilitate learning (Hew & Brush, Citation2007). One type of technology that has increased in popularity is massive open online courses (MOOCs). MOOCs are classes conducted in an online environment, but differ from previous approaches to online education (Alraimi, Zo, & Ciganek, Citation2015). MOOCs are open to anyone and free of charge, which often results in much larger audiences than traditional online education (Voss, Citation2013). The number of MOOCs offered around the globe has been increasing; according to the statistics released by CLASS CENTRAL, there were 2,450 in 2015, 4,250 in 2016, 6,850 in 2017, and 9,400 in 2018 (CLASS CENTRAL, Citation2018). Despite the popularity of MOOCs, a survey by Statista in 2015 revealed that compared to other online education platforms, faculty worldwide rank MOOCs low (Statista, Citation2015). Additionally, MOOCs have raised three types of challenges for teachers (Hew & Cheung, Citation2014). First, teachers have to devote significant amounts of time and money. Second, it is difficult to evaluate students’ work or achievement. Third, teachers receive little feedback from students. These challenges can discourage teachers’ interest in the acceptance and use of MOOCs. As such, it is imperative to understand the drivers of teachers’ acceptance and use of MOOCs.
Most prior relevant studies have examined MOOC use from the learner’s perspective using technology acceptance model (TAM) (Hsu, Chen, & Ting, Citation2018; Shao, Citation2018; Wu & Chen, Citation2017; Yang & Su, Citation2017; Zhou, Citation2016), task-technology fit (Huang, Zhang, & Liu, Citation2017; Jo, Citation2018; Wu & Chen, Citation2017), the theory of planned behavior (Yang & Su, Citation2017; Zhou, Citation2016), the social support theory (Hsu et al., Citation2018), the social cognitive theory (Shao, Citation2018), the self-determination theory (Fang, Tang, Yang, & Peng, Citation2019; Zhou, Citation2016), the technology-user-environment perspective (Ma & Lee, Citation2019a), network externalities (Li, Wang, & Tan, Citation2018), the regulatory focus theory (Zhang, Citation2016), the expectation-confirmation paradigm (Alraimi et al., Citation2015; Zhou, Citation2017), and the innovation resistance theory (Ma & Lee, Citation2019b). Relatively few studies have examined this issue from the perspective of teachers (Liyanagunawardena, Adams, & Williams, Citation2013), even though teachers play a key facilitating role in MOOCs and are instrumental in terms of engaging students in the learning process through course design (e.g. course content, mode of interaction with students), which can influence students’ MOOC experiences and in turn their continuance intention. Thus, it is imperative to investigate MOOC usage and acceptance from the perspective of teachers.
Among all theories or models used to predict an individual’s IT acceptance and use, the unified theory of acceptance and use of technology (UTAUT) and its extended version (UTAUT2) are the most comprehensive, since they were developed by integrating different theories/models (Venkatesh, Morris, Davis, & Davis, Citation2003; Venkatesh, Thong, & Xu, Citation2012). However, they have yet to be utilized within a MOOC context. Compared to UTAUT, UTAUT2 is more appropriate to this context from the teacher perspective for several reasons. First, UTAUT2 was developed from the perspective of voluntary users (i.e. consumers), rather than mandatory users (i.e. organizational users/employees) (Venkatesh et al., Citation2012), which matches well with a key feature of MOOCs – participation is completely voluntary for teachers (Liyanagunawardena et al., Citation2013). Second, the inclusion of hedonic motivation and price value in UTAUT2 is congruent to current MOOC practices whereby teachers include cartoon animations to increase both entertainment in learning (Laaser & Toloza, Citation2017) and the perceived value derived from teachers sharing knowledge around the globe to exert a personal impact (Hew & Cheung, Citation2014; Li & Li, Citation2016). Given the above, this study adopts UTAUT2 as its key theoretical foundation to investigate the determinants of teachers’ acceptance and use of MOOCs. Specifically, we examine the relationships among performance expectancy, effort expectancy, social influence, facilitating conditions, hedonic motivation, price value, behavioral intention, and technology use. This study contributes to the literature by assessing the acceptance and use of MOOCs as an educational technology tool from a teacher perspective that is currently lacking. Furthermore, based on the findings, we also provide practical guidelines to facilitate university teachers’ acceptance and use of MOOCs.
2. Theoretical foundations
2.1. Massive open online courses (MOOCs)
MOOCs can be categorized under the larger framework of open educational resources; they consist of digitized materials offered freely and openly to educators, students, and self-learners to use and reuse for teaching, learning, and research (OECD, Citation2007, p. 2). George Siemen and Stephen Downers created the term MOOCs in 2008, and MOOCs have been and are being run by numerous public and elite universities (Liyanagunawardena et al., Citation2013). MOOCs are classified into connectivist MOOCs and AI-Stanford-like courses. The former focuses on a more individualist learning approach, while the latter uses a more social approach for learning (Rodriguez, Citation2012). MOOCs have pros and cons. Supporters note that they: contribute to the democratization of education by making it more accessible, increase the prestige of institutions that offer MOOCs, provide opportunities for professors to experiment with pedagogy when teaching online courses (Hew & Cheung, Citation2014; Jacobs, Citation2013). Opponents are concerned about the quality of education being watered down, and potential harm for less prestigious education institutions (Hew & Cheung, Citation2014; Jacobs, Citation2013). Furthermore, the quality and effectiveness of MOOCs have been questioned due to the high dropout and incompletion rates (Alraimi et al., Citation2015).
According to a systematic review by Liyanagunawardena et al. (Citation2013), research on MOOCs began in 2008 and increased in 2012, covering a variety of topics and themes, such as agency, connectivism, actor network theory, dangers, learning experience, pedagogies, technology, and trends. Another review by Hew and Cheung (Citation2014) categorized MOOC studies into two themes: student perspective and instructor perspective. The former examined students’ sign-up motives, attitudes toward MOOCs, and challenges and learning effectiveness of using MOOCs, while the latter examined teachers’ reasons for utilizing MOOCs, ways to engage students when using MOOCs, and challenges associated with teaching using MOOCs. However, many more researchers have focused on the former (e.g. Alraimi et al., Citation2015; Fang et al., Citation2019; Hsu et al., Citation2018; Ma & Lee, Citation2019a; Ma & Lee, Citation2019b; Shao, Citation2018; Wu & Chen, Citation2017; Zhou, Citation2016) as compared to the latter; the current study intends to fill this gap.
2.2. Teachers’ acceptance toward online learning technology
Past research on teachers’ acceptance toward online learning technology is summarized in . Among these studies, TAM is the mostly adopted theoretical model. Furthermore, facilitating conditions has frequently been integrated into TAM, as it is a key driver of technology use (Mei, Brown, & Teo, Citation2018; Nikou & Economides, Citation2019; Teo, Sang, Mei, & Hoi, Citation2019; Wong, Citation2016). Relatively few studies have examined teachers’ acceptance from the perspective of UTAUT, while UTAUT2 has not been a focus at all in the extant literature. In the current research context, due to the entertaining (Laaser & Toloza, Citation2017) and knowledge-sharing (Hew & Cheung, Citation2014; Li & Li, Citation2016) nature of MOOCs, it is imperative to examine teachers’ adoption and use of MOOCs based on the perspective of UTAUT2, as these topics have yet to receive any attention.
Table 1. Past research on teachers’ acceptance toward online learning technology.
2.3. Unified theory of acceptance and use of technology
Individual acceptance and use of information technology (IT) represent an active information systems (IS) research stream (Venkatesh et al., Citation2016). Many past studies have utilized TAM and the theory of planned behavior (TPB) to predict IT adoption or use (Venkatesh et al., Citation2016). Venkatesh et al. (Citation2003) synthesized eight theories and models concerning technology use and proposed the UTAUT, which examines the causal relationships among several key constructs: performance expectancy, effort expectancy, social influence, facilitating conditions, behavioral intention, and technology use. Performance expectancy refers to the degree to which a person believes that using the system can help attain goals in terms of job performance (Venkatesh et al., Citation2003). Effort expectancy is defined as the degree of ease associated with using the system (Venkatesh et al., Citation2003, p. 450). Social influence refers to the degree to which a person perceives that important others want him/her to use the system (Venkatesh et al., Citation2003, p. 451). Facilitating conditions refer to the degree to which a person believes that an organizational and technical infrastructure exists to support the system use (Venkatesh et al., Citation2003, p. 453). The UTAUT has been validated in different educational technology contexts (e.g. Chen & Lin, Citation2016; Lai, Citation2018; Olasina, Citation2019; Teo & Noyes, Citation2014; Wang, Wu, & Wang, Citation2009). It postulates that performance expectancy, effort expectancy, and social influence facilitate behavioral intention to use a technology, while facilitating conditions and behavioral intention determine technology use (Venkatesh et al., Citation2003; Venkatesh et al., Citation2016). Furthermore, gender, age, experience, and voluntariness are four moderators for the aforementioned causal relationships (Venkatesh et al., Citation2003; Venkatesh et al., Citation2016).
Past studies have applied the theory in the contexts of organization e-learning (Yoo, Han, & Huang, Citation2012), E-government services and technology (Al-Shafi, Weerakkody, & Janssen, Citation2009; Venkatesh, Thong, Chan, Hu, & Brown, Citation2011), agile IS (Hong, Thong, Chasalow, & Dhillon, Citation2011), online shopping (Lian & Yen, Citation2014), and mobile services (Oliveira, Faria, Thomas, & Popovič, Citation2014; Zhou, Lu, & Wang, Citation2010). The UTAUT has been widely applied in educational settings such as the adoption of web-based learning environments for learning by students (Liao, Shim, & Luo, Citation2004; Olasina, Citation2019), the adoption of mobile learning systems by students (Sharma, Sarrab, & Al-Shihi, Citation2017), the adoption of tablet PCs for learning by students (El-Gayar & Moran, Citation2007), the intention to use technology by pre-service teachers (Teo & Noyes, Citation2014), teacher administered programs focused on the initial use and final use of digital learning environments for teaching communication (Pynoo et al., Citation2011), and acceptance and use of social media for research by academic researchers (Gruzd et al., Citation2012). These studies have indicated that performance expectancy is the key driver of system use. However, the effects of other UTAUT drivers on system use were inconclusive. For instance, in some studies, effort expectancy had a greater influence (Gruzd et al., Citation2012; Teo & Noyes, Citation2014), while other researchers indicated a minor (Pynoo et al., Citation2011) or even negative influence (Liao et al., Citation2004). The effect of social influence on system use was positive in studies by Olasina (Citation2019), Pynoo et al. (Citation2011) and Teo and Noyes (Citation2014), but uncertain in Gruzd et al. (Citation2012). Facilitating conditions had greater importance in two studies (El-Gayar & Moran, Citation2007; Liao et al., Citation2004) but was of minor importance in three others (Gruzd et al., Citation2012; Pynoo et al., Citation2011; Teo & Noyes, Citation2014). Furthermore, although behavioral intention had a positive influence on system use in most of these studies, El-Gayar and Moran (Citation2007) noted a non-significant effect. These inconsistent findings suggest that additional studies using UTAUT are needed in the educational research context.
2.4. Extended unified theory of acceptance and use of technology (UTAUT2)
Consumer IT represents a huge market: many technology devices, applications, and services are well received by consumers (Stofega & Llamas, Citation2009). The original UTAUT can be insufficient to predict the use and adoption of consumer IT since it was developed in the organizational context. Accordingly, Venkatesh et al. (Citation2012) proposed the UTAUT2 by adding additional constructs (i.e. hedonic motivation, price value, and habit) and more causal relationships. Hedonic motivation refers to the fun or pleasure derived from using a technology; price value is defined as consumers’ cognitive tradeoff between the perceived benefits of the applications and the monetary cost associated with using them; habit refers to a perceptual construct reflecting the results of prior experiences (Venkatesh et al., Citation2012, p. 161). In addition to the construct relationships proposed in the original UTAUT, the following relationships were added: first, hedonic motivation and price value can determine behavioral intention; second, habit can influence both behavioral intention and usage behavior; and third, facilitating conditions can influence behavioral intention. The proposed moderators in the UTAUT2 included age, gender, and experience (Venkatesh et al., Citation2012, p. 161).
Past studies have extended or revised the UTAUT2 in various contexts including the adoption of social networking sites, mobile payment, and mobile shopping apps. Oliveira, Thomas, Baptista, and Campos (Citation2016) extended the UTAUT2 with innovative technology characteristics and found that compatibility, perceived technology security, performance expectations, innovativeness, and social influence all effect the adoption and intention to recommend mobile payments. Herrero and San Martín (Citation2017) revised the UTAUT2 by replacing price value with privacy concerns in the context of tourism products/services and found that the key drivers of users’ intention to use social networking sites to share content about tourism experiences include performance expectancy, hedonic motivation, and habit. Chopdar, Korfiatis, Sivakumar, and Lytras (Citation2018) integrated two manifestations of perceived risk into the UTAUT2 and uncovered several key findings: all drivers of the UTAUT2 can predict behavioral intention to use mobile shopping apps, which in turn influences use behavior, but perceived risk has no influence on behavioral intention.
However, few studies have applied the UTAUT2 in educational settings. Mtebe, Mbwilo, and Kissaka (Citation2016) examined factors affecting teachers’ use of multimedia-enhanced content and found that all the factors influence teachers’ acceptance and use of the developed content, except for performance expectancy. El-Masri and Tarhini (Citation2017) investigated factors influencing the adoption of e-learning systems with trust as an additional driver and found that the proposed relationships differ across different countries (developed vs. developing). Aria and Archer (Citation2018) examined the effectiveness of online-video vs. in-person user training for an online self-management support system for chronic illness and found the use of a short video to educate users on the features of a highly complex system is as effective as more time-consuming in-person education programs. Since relevant studies have yielded inconsistent findings regarding the effects of performance expectancy, effort expectancy, social influence, facilitating conditions, and price value, more studies are required to affirm the UTAUT2 as a theoretical basis for examining drivers of technology acceptance and use in the educational settings. Given that the UTAUT2 has not been examined in the context of MOOCs, the current study investigates factors influencing teacher acceptance and use of MOOCs based on the UTAUT2.
2.5. Hypotheses development
This study developed hypotheses based on the UTAUT2 notion that performance expectancy, effort expectancy, social influence, facilitating conditions, hedonic motivation, and price value determine users’ behavioral intention to adopt or use IT (Venkatesh et al., Citation2012). Furthermore, facilitating conditions and behavioral intention can facilitate IT use behavior. Since MOOCs are a form of educational technology, it is expected that the aforementioned relationships are applicable to MOOCs. Thus, we posit the following hypotheses.
H1: Performance expectancy positively influences teachers’ behavioral intention to adopt MOOCs.
H2: Effort expectancy positively influences teachers’ behavioral intention to adopt MOOCs.
H3: Social influence positively influences teachers’ behavioral intention to adopt MOOCs.
H4a: Facilitating conditions positively influence teachers’ behavioral intention to adopt MOOCs.
H4b: Facilitating conditions influence teachers’ adoption of MOOCs.
H5: Hedonic motivation positively influences teachers’ behavioral intention to adopt MOOCs.
H6: Price value positively influences teachers’ behavioral intention to adopt MOOCs.
H7: Teachers’ behavioral intention to adopt MOOCs influences their adoption of MOOCs.
The research model is shown in .
3. Methodology
3.1. Sample and data collection
An online survey was conducted to collect the sample data, which was analyzed to validate the research model shown in . Ethical approval clearance and informed consent clearance were granted by Ministry of Science and Technology (MOST) in Taiwan due to the use of anonymous questionnaires. The online questionnaire was composed of two portions. The first portion collected demographic information, while the second focused on the key constructs. The research target was university teachers (i.e. professors, associate professors, assistant professors, lecturers, etc.). The sample recruiting process was as follows. First, a university teacher list for Taiwan was accessed. Second, an invitation letter with the online questionnaire link was emailed to the teachers on the list. Simultaneously, the invitation letter and the questionnaire link were put on MOOC communities in Taiwan. Data was collected from November 2017 to May 2018. A total of 166 responses were collected. Respondents with incomplete answers were excluded, resulting in 161 usable surveys.
The sample characteristics are presented in . Of the respondents, 63.5% were male, most were 41–50 years old (47.3%), and 90.7% were professors of different levels (i.e. assistant professor, associate professor, professor).
Table 2. Sample characteristics.
3.2. Measurement
The items measuring performance expectancy, effort expectancy, social influence, facilitating conditions, hedonic motivation, price value, behavioral intention, and usage behavior were adapted from Venkatesh et al. (Citation2012) to suit the MOOC research context. These items were measured using 5-point Likert scales anchored by 1 (strongly disagree) and 5 (strongly agree). Two IS professors were invited to evaluate the survey tool first as a pretest. Ambiguous items (those having confusing or inappropriate wording) were identified and modified according to the consensus of the members in the pretest. displays the finalized items.
Table 3. Examining convergent validity and construct reliability.
4. Results
4.1. Data analysis
Since the aim of this study was to examine the drivers of teachers’ MOOCs usage behavior, partial least squares structural equation modeling (PLS-SEM) was deemed to be appropriate, as it helps to explain causal relationships among constructs (Hair, Ringle, & Sarstedt, Citation2011). SmartPLS 3 was used for the analysis (Ringle, Wende, & Becker, Citation2015), and results were reported following Hair, Hollingsworth, Randolph, and Chong’s (Citation2017) guidelines. PLS-SEM evaluations are comprised of an outer model (measurement model) and an inner model (structural model). Internal consistency reliability, convergent validity, and discriminant validity are used to assess the measurement model. Composite reliability (CR) and Cronbach’s α are used to evaluate internal consistency reliability. Both CR and Cronbach’s α values are recommended to be greater than 0.7(Hair et al., Citation2017). Convergent validity is supported if all the standardized item loadings are greater than 0.70, and if the values of the average variance extracted (AVE) for every construct exceed 0.5, the threshold value recommended by Fornell and Larcker (Citation1981). Discriminant validity is achieved if both AVE estimates of a given pair of constructs are greater than the square of the construct correlation. Alternatively, Heterotrait-Monotrait (HTMT) ratios can be calculated to evaluate discriminant validity. If all HTMT ratios are lower than 0.85, discriminant validity is achieved (Henseler, Ringle, & Sarstedt, Citation2015). The second step is to validate the structural model using a nonparametric bootstrap procedure to test the statistical significance of path coefficients. Regarding parameter settings, 5,000 bootstrap samples were adopted (Hair et al., Citation2017). One-tailed tests were used to test for the directional research hypotheses.
4.2. Measurement model
From , internal consistency reliabilities for all constructs were greater than the threshold value (i.e. α ≥ 0.7; CR ≥ 0.7) (Hair et al., Citation2017). All the item loadings were statistically significant (p < 0.001) and were greater than 0.70 (Hair et al., Citation2017). From , AVE values for all constructs were greater than 0.5 (Hair et al., Citation2017). Thus, convergent validity was supported. Furthermore, as shown in , the estimates of the correlation coefficients were smaller than the corresponding square roots of the AVE estimates for all pairs of constructs (Chin, Citation1998) and all HTMT ratios were lower than 0.85 (Hair et al., Citation2017; Henseler et al., Citation2015). Therefore, discriminant validity was achieved.
Table 4. Correlation matrix and descriptive statistics of the constructs.
4.3. Structural models
The values of R2, the path coefficients, and the corresponding levels of significance are shown in . Performance expectancy (β = 0.33, p < 0.001), social influence (β = 0.33, p < 0.001), facilitating conditions (β = 0.18, p < 0.01), and price value (β = 0.15, p < 0.05) positively influenced behavioral intention. Thus, H1, H3, H4a and H6 were supported. Furthermore, facilitating conditions (β = 0.16, p < 0.05) and behavioral intention (β = 0.27, p < 0.01) positively influenced usage behavior. Thus, H4b and H7 were supported. However, effort expectancy (β = −0.03, p > 0.10) and hedonic motivation (β = 0.01, p > 0.10) had no effect on behavioral intention. Thus, H2 and H5 were not supported.
Figure 2. Hypotheses test results. Note: Standardized path coefficients are shown. *p < 0.05; **p < 0.01; ***p < 0.001.
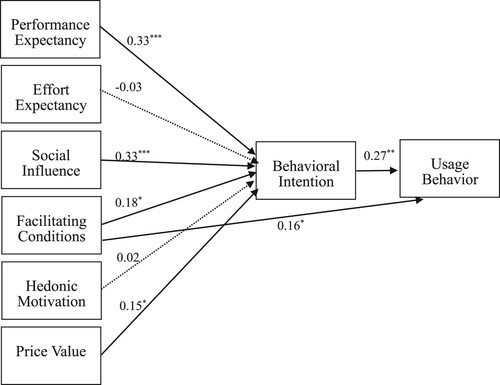
Since the objective of this study was to predict usage behavior in the context of MOOCs, the R2 value of usage behavior was reported to assess the predictive capability of the research model. Chin (Citation1998) posited that R2 values of 0.67, 0.33, or 0.19 for endogenous constructs are substantial, moderate, and weak, respectively. Based on this rule of thumb, the R2 value of usage behavior (0.633) near eda rating of substantial. Furthermore, the Q2 value reflecting the model’s out-of-sample predictive relevance with regard to usage behavior was calculated, and was greater than zero, which supports the model’s predictive relevance (Hair, Hult, Ringle, & Sarstedt, Citation2016, p. 202).
5. Discussion
The use of MOOCs can make a course easily accessible by people around the globe. It can create opportunities for students from many different countries to attend courses developed by a well-known professor in a prestigious university through the power of digital technology. Despite the increasing popularity of MOOCs worldwide, they have encountered some resistance from faculty. According to a global survey by Statista in 2015, compared to other digital educational models, the percentage of faculty members who support MOOCs is the lowest (Statista, Citation2015). Literature on MOOCs also summarize some challenges faced by instructors when they use MOOCs for teaching (Hew & Cheung, Citation2014), which discourage teacher acceptance and use of MOOCs. This context motivated the current study to uncover the key determinants of teacher acceptance and use of MOOCs.
While most previous studies approached this research question using TAM (e.g. Mei et al., Citation2018; Nikou & Economides, Citation2019; Teo et al., Citation2019; Wong, Citation2016), UTAUT2 (Venkatesh et al., Citation2012) has a more comprehensive theoretical lens, but has not been used in the context of MOOCs. Hedonic motivation and price value are two newly included antecedents of UTAUT2 that match the entertaining and knowledge sharing features of MOOCs (Hew & Cheung, Citation2014; Laaser & Toloza, Citation2017; Li & Li, Citation2016). Thus, examining teacher acceptance and use of MOOCs using UTAUT2 is of practical relevance. Our findings suggest that performance expectancy, social influence, facilitating conditions, and price value perceived by teachers regarding MOOCs can drive their behavioral intention to adopt MOOCs. Moreover, facilitating conditions and behavioral intention determine usage behavior. However, effort expectancy and hedonic motivation as perceived by teachers pertaining to the use of MOOCs have no effect on behavioral intention. Based on these findings, relevant theoretical and practical implications are discussed below.
5.1. Theoretical implications
The results of this study reveal that most of the main effects proposed by the UTAUT2 are applicable to MOOCs, which indicates the validity of using UTAUT2. Among all drivers of behavioral intention, we found that performance expectancy was a key driver, which is consistent with past studies in other consumer IT contexts (e.g. Chopdar et al., Citation2018; Herrero & San Martín, Citation2017; Oliveira et al., Citation2016; Venkatesh et al., Citation2012), and which also conforms to earlier technology acceptance model (TAM) studies in educational settings (Hu, Clark, & Ma, Citation2003; Ma, Andersson, & Streith, Citation2005; Teo, Citation2009; Teo, Lee, & Chai, Citation2008). Furthermore, although social influence was a key determinant for behavioral intention in the MOOC context, this was not true in consumer IT contexts, where there were no effects (Chopdar et al., Citation2018; Herrero & San Martín, Citation2017) or only small effects (Oliveira et al., Citation2016) on behavioral intention. This could be attributed to the different research contexts. Based on the social influence theory, social groups can exert normative influence to make group members adopt a certain behavior through compliance mechanisms (Deutsch & Gerard, Citation1955; Hsu & Lu, Citation2004). The teacher group may be more concerned about obtaining rewards (e.g. support and resources) and avoiding punishments (e.g. budget cut) from top management of their universities, while firms have less power to directly affect consumer groups. Thus, it is plausible that the normative influence is more pronounced for the teacher group than the consumer group.
Price value is an indispensable factor influencing teachers’ behavioral intention to adopt and use MOOCs. Teachers tend to use MOOCs if they believe the perceived benefits exceed the perceived costs. Although many teachers feel tired when preparing MOOC course materials and courses, MOOCs allow teachers to share knowledge to larger audience (Voss, Citation2013) and exert personal influence around the globe (Hew & Cheung, Citation2014; Li & Li, Citation2016). Compared to traditional online education, MOOCs help these teachers gain a greater sense of achievement that outweighs the required time and mental effort, which further facilitates the adoption and use of MOOCs. This result also validates the use ofUTAUT2 rather than UTAUT in the current research context.
Surprisingly, we found that effort expectancy and hedonic motivation had no influence on teachers’ behavioral intention to adopt MOOCs. Effort expectancy was conceptualized as the ease of use perceived by teachers in using MOOCs. It is possible that effort expectancy was viewed by teachers as a baseline regarding the intent to adopt MOOCs, while performance expectancy was a key driver. Past studies on consumer IT support this view. In these studies, effort expectancy had mixed or no effect on behavioral intention, while performance expectancy had the largest effect (e.g. Chopdar et al., Citation2018; Herrero & San Martín, Citation2017; Oliveira et al., Citation2016). On the other hand, the TAM indicates that compared to perceived usefulness, perceived ease of use has a weak effect on intention to use an IS (Davis, Bagozzi, & Warshaw, Citation1992). Since ease of use corresponds to effort expectancy and perceived usefulness corresponds to performance expectancy, Davis et al. (Citation1992)’s result helps to explain why effort expectancy has a weaker effect than performance expectancy on behavioral intention to use MOOCs.
Hedonic motivation had no effect on teachers’ behavioral intention to adopt and use MOOCs. This could be explained by the task nature. Using MOOCs to teach is similar to a utilitarian task, and less like a hedonic task. As indicated in the literature review, teachers use MOOCs to increase personal reputation, show they are the first to use MOOCs, help them achieve tenure, and brandish their influential power by connecting to large, diverse audiences (Hew & Cheung, Citation2014). It is less likely for teachers to use MOOCs for fun or pleasure. Although it is possible to use cartoon animations to increase the entertainment and fun aspects of MOOCs (Laaser & Toloza, Citation2017), the aim of this technology is to facilitate learning effectiveness and has a utilitarian purpose. Thus, our findings suggest that task nature can be considered as a new moderator for the UTAUT2. When the task is utilitarian by nature, hedonic motivation may have limited effect on behavioral intention. Conversely, hedonic motivation can have a larger effect on behavioral intention when the task associated with the technology is hedonic in nature (e.g. playing video games).
Another reason for the nonsignificant effects of effort expectancy and hedonic motivation is the sample composition. Most of the sample consisted of senior faculty (60.3%). Since teachers with shorter and longer years of teaching service have different attitudes toward technology (Teo, Citation2014), years of teaching can moderate the proposed relationships. The original UTAUT postulates that the effect of effort expectancy on behavioral intention decreases with experience (Venkatesh et al., Citation2003). Similarly, the UTAUT2 posits the effect of hedonic motivation on behavioral intention decreases with experience (Venkatesh et al., Citation2012). Thus, effort expectancy and hedonic motivation are likely to have minimal effects on behavioral intention to use MOOCs in this study. If a sample of more junior faculty was to be used, hedonic motivation and effort expectancy would likely have stronger effects on behavioral intentions.
This study neglected to investigate the effects of habit on both behavioral intention and usage behavior as proposed by the UTAUT2 (Venkatesh et al., Citation2012). This is inevitable since our sample included both new users and experienced users. It is unlikely for new users to have habits associated with using the system. The UTAUT2 postulates that habit has a lesser role when focusing on less experienced users, while the reverse is true when focusing on more experienced users (Venkatesh et al., Citation2012). However, the theory neglects to consider those with no experience using an IT. When non-users are considered in the model, habit has to be removed from the UTAUT2.
5.2. Practical implications
Our findings have significant practical implications, as they can help provide guidelines for university administrators to promote teachers’ acceptance and use of MOOCs. Among all drivers of teachers’ intention to adopt MOOCs, performance expectancy and social influence are two key drivers. As such, practitioners should improve teachers’ perception of performance expectancy and social influence regarding the acceptance and use of MOOCs. For performance expectancy, the key reason that teachers adopt MOOCs for teaching is undeniably their usefulness; however, the narrative of MOOCs as a useful technology that can promote one’s teaching goals is vague and not persuasive. It is likely that many teachers have not adopted MOOCs because they do not understand the specific merits of doing so. Thus, university leaders should communicate clearly the benefits of using MOOCs to teachers. They can survey all faculty using MOOCs and summarize a list of benefits of doing so. The top five benefits can be conveyed to persuade teachers to adopt MOOCs. For social influence, the leaders of universities can demonstrate positive attitudes toward MOOCs by including the usage rate of MOOCs in faculty performance assessment criteria. This can promote the acceptance and use of MOOCs by teachers, as it offers a positive incentive. University administrators can also invite experienced faculty to share their successful experiences to motivate other teachers.
Since facilitating conditions can drive both teachers’ behavioral intention and usage behavior of MOOCs, practitioners should give teachers resources and knowledge pertaining to the use of MOOCs. A short introductory video can be recorded to educate teachers about the basics of using the system. Universities should assign a group of people to respond to teachers’ questions and help teachers use MOOCs. Seminars can be arranged for teachers to discuss their usage experiences and share teaching resources. MOOC users who have a positive experience can be invited to share their experiences and respond to potential questions.
Price value has an influence on teachers’ behavioral intention to adopt MOOCs. University administrators have to instill a positive feeling among teachers that the benefits of using MOOCs outweigh the costs. Even though this is unattainable in the short-term, university administrators need to constantly communicate the specific benefits of using MOOCs for teaching, and provide support to reduce the time and effort required for teachers to prepare and use them. Additionally, effort can be quantified by using benefit/cost ratios (B/C ratio) as a new metric to measure the success of MOOCs compared to other teaching methods. This metric is better to evaluate the success of using MOOCs than traditional metrics (i.e. the adoption or usage rate) since the former considers both the benefits and costs of (Hew & Cheung, Citation2014). Although adoption/usage rate is an important outcome metric, it does not suggest ways to improve the metric. The use of the B/C ratio has diagnostic value since it can suggest whether the benefits are greater than, smaller than, or equal to the costs, and can be further decomposed into different types of benefits and costs. University administrators can invite experts to determine these benefit and cost types for MOOCs and other teaching methods, and then calculate the B/C ratios each method on a periodical basis (e.g. semesterly). To reduce resistance from those opposed to MOOCs, the B/C ratio of MOOCs must show improvement compared to the other teaching methods.
5.3. Limitations and future research
This research study has the following limitations. First, MOOCs only represent one type of e-learning system, so our findings may not be generalizable to other systems; future studies can apply our research framework to other systems. Second, as revealed in the discussion section, we suspect teachers’ acceptance and use of MOOCs is largely driven by extrinsic motivation rather than intrinsic motivation. As such, an interesting avenue for future studies is to decompose different facets of extrinsic motivation and examine their relative impact on the use and adoption of MOOCs. Third, although this research study investigated teachers’ acceptance and use of MOOCs from the perspective of the UTAUT2, only the main effects proposed by UTAUT2 were utilized, while the moderators were excluded. An interesting avenue for future studies is to examine the moderating effects on the proposed relationships. For instance, teacher’s prior MOOC exposure as a learner or developer, generic competence toward education technology, and number of years of teaching are possible moderators. Based on the UTAUT (Venkatesh, 2003) and UTAUT2 (Venkatesh et al., Citation2012), users’ experience negatively moderates the relationships between effort expectancy and behavioral intention, between social influence and behavioral intention, and between hedonic motivation and behavioral intention, but positively moderates the relationship between facilitating conditions and usage behavior. Future studies should further verify these findings. Finally, future studies may adopt a longitudinal design to examine the proposed model and further investigate different adoption stages of MOOCs.
Disclosure statement
No potential conflict of interest was reported by the authors.
Additional information
Funding
Notes on contributors
Timmy H. Tseng
Timmy H. Tseng is an Assistant Professor in the Department of Business Administration at Fu Jen Catholic University, Taiwan. He received his PhD in Marketing from National Chengchi University, Taiwan. His current research interests include experiential marketing, branding strategy, e-marketing, and service marketing. His work has been published in Internet Research, International Journal of Hospitality Management, European Journal of Marketing, International Journal of Mobile Communications, Computers in Human Behavior, Online Information Review, Information Technology and Management, and Journal of Business Economics and Management.
Shinjeng Lin
Shinjeng Lin is a Professor in the Department of Management, Leadership and Information Systems at Le Moyne College, USA. He received his Ph.D. in Information Science from Rutgers University, USA. His current research interests include information seeking process, design and evaluation of interactive user interfaces, acceptance and adoption of innovative technologies. He has published in journals such as Academy of Management Learning and Education, Computers & Education, Internet Research, Journal of American Society for Information Science and Technology, Journal of Educational Computing Research, Thinking Skills and Creativity, Information Technology & Management, Information Processing and Management, and Journal of Computer Information Systems.
Yi-Shun Wang
Yi-Shun Wang is a Distinguished Professor in the Department of Information Management at the National Changhua University of Education, Taiwan. He received his Ph.D. in MIS from National Chengchi University, Taiwan. His current research interests include information and educational technology adoption strategies, IS success models, online user behavior, knowledge management, Internet entrepreneurship education, and e-learning. He has published papers in journals such as Interactive Learning Environments, Academy of Management Learning and Education, Computers & Education, British Journal of Educational Technology, Information Systems Journal, Information & Management, International Journal of Information Management, Government Information Quarterly, Internet Research, Computers in Human Behavior, International Journal of Human–Computer Interaction, Information Technology and People, Information Technology and Management, Journal of Educational Computing Research, among others. He is currently serving as the Chairman for the Research Discipline of Applied Science Education in the Ministry of Science and Technology of Taiwan.
Hui-Xuan Liu
Hui-Xuan Liu received her master’s degree in Information Management from National Changhua University of Education, Taiwan. Her research interests include information and educational technology adoption and online user behavior.
References
- Al-Shafi, S., Weerakkody, V., & Janssen, M. (2009). Investigating the adoption of eGovernment services in Qatar using the UTAUT model. In Proceedings of the 19th Americas conference on information systems (AMCIS) (p. 260).
- Alraimi, K. M., Zo, H., & Ciganek, A. P. (2015). Understanding the MOOCs continuance: The role of openness and reputation. Computers & Education, 80, 28–38. doi: https://doi.org/10.1016/j.compedu.2014.08.006
- Aria, R., & Archer, N. (2018). Using an educational video vs. In-person education to measure patient perceptions of an online self-management support system for chronic illness. Computers in Human Behavior, 84, 162–170. doi: https://doi.org/10.1016/j.chb.2018.01.041
- Chen, C.-C., & Lin, P.-H. (2016). Development and evaluation of a context-aware ubiquitous learning environment for astronomy education. Interactive Learning Environments, 24(3), 644–661. doi: https://doi.org/10.1080/10494820.2014.915417
- Cheung, E. Y. M., & Sachs, J. (2006). Student teachers’ acceptance of a web-based information system. Psychologia, 49(2), 132–141. doi: https://doi.org/10.2117/psysoc.2006.132
- Chin, W. W. (1998). The partial least squares approach to structural equation modeling. Modern Methods for Business Research, 295(2), 295–336.
- Chopdar, P. K., Korfiatis, N., Sivakumar, V. J., & Lytras, M. D. (2018). Mobile shopping apps adoption and perceived risks: A cross-country perspective utilizing the unified theory of acceptance and use of technology. Computers in Human Behavior, 86, 109–128. doi: https://doi.org/10.1016/j.chb.2018.04.017
- CLASS CENTRAL. (2018). By the numbers: MOOCS in 2017. Retrieved from https://www.class-central.com/report/mooc-stats-2017/
- Cuhadar, C. (2014). Information technologies pre-service teachers’ acceptance of tablet PCs as an innovative learning tool. Educational Sciences: Theory and Practice, 14(2), 741–753.
- Davis, F. D., Bagozzi, R. P., & Warshaw, P. R. (1992). Extrinsic and intrinsic motivation to use computers in the workplace 1. Journal of Applied Social Psychology, 22(14), 1111–1132. doi: https://doi.org/10.1111/j.1559-1816.1992.tb00945.x
- Deutsch, M., & Gerard, H. B. (1955). A study of normative and informational social influences upon individual judgment. Journal of Abnormal and Social Psychology, 51(3), 629–636. doi: https://doi.org/10.1037/h0046408
- El-Gayar, O., & Moran, M. (2007). Evaluating students’ acceptance and use of tablet PCs in collegiate classrooms. In Proceedings of the 13th Americas conference on information systems (AMCIS) (p. 91).
- El-Masri, M., & Tarhini, A. (2017). Factors affecting the adoption of e-learning systems in Qatar and USA: Extending the unified theory of acceptance and use of technology 2 (UTAUT2). Educational Technology Research and Development, 65(3), 743–763. doi: https://doi.org/10.1007/s11423-016-9508-8
- Fang, J., Tang, L., Yang, J., & Peng, M. (2019). Social interaction in MOOCs: The mediating effects of immersive experience and psychological needs satisfaction. Telematics and Informatics, 39, 75–91. doi: https://doi.org/10.1016/j.tele.2019.01.006
- Fornell, C., & Larcker, D. F. (1981). Evaluating structural equation models with unobservable variables and measurement error. Journal of Marketing Research, 18(1), 39–50. doi: https://doi.org/10.1177/002224378101800104
- Gruzd, A, Staves, K, & Wilk, A. (2012). Connected scholars: Examining the role of social media in research practices of faculty using the UTAUT model. Computers in Human Behavior, 28(6), 2340–2350. doi: https://doi.org/10.1016/j.chb.2012.07.004
- Hair, J., Hollingsworth, C. L., Randolph, A. B., & Chong, A. Y. L. (2017). An updated and expanded assessment of PLS-SEM in information systems research. Industrial Management & Data Systems, 117(3), 442–458. doi: https://doi.org/10.1108/IMDS-04-2016-0130
- Hair, J. F. Jr., Hult, G. T. M., Ringle, C., & Sarstedt, M. (2016). A primer on partial least squares structural equation modeling (PLS-SEM) (2nd ed.). Los Angeles: Sage Publications.
- Hair, J. F., Ringle, C. M., & Sarstedt, M. (2011). PLS-SEM: Indeed a silver bullet. Journal of Marketing Theory and Practice, 19(2), 139–152. doi: https://doi.org/10.2753/MTP1069-6679190202
- Henseler, J., Ringle, C. M., & Sarstedt, M. (2015). A new criterion for assessing discriminant validity in variance-based structural equation modeling. Journal of the Academy of Marketing Science, 43(1), 115–135. doi: https://doi.org/10.1007/s11747-014-0403-8
- Herrero, Á, & San Martín, H. (2017). Explaining the adoption of social networks sites for sharing user-generated content: A revision of the UTAUT2. Computers in Human Behavior, 71, 209–217. doi: https://doi.org/10.1016/j.chb.2017.02.007
- Hew, KF, & Brush , T. (2007). Integrating technology into K-12 teaching and learning: Current knowledge gaps and recommendations for future research. Educational Technology Research and Development, 55(3), 223–252. doi: https://doi.org/10.1007/s11423-006-9022-5
- Hew, K. F., & Cheung, W. S. (2014). Students’ and instructors’ use of massive open online courses (MOOCs): Motivations and challenges. Educational Research Review, 12, 45–58. doi: https://doi.org/10.1016/j.edurev.2014.05.001
- Hong, W., Thong, J. Y., Chasalow, L. C., & Dhillon, G. (2011). User acceptance of agile information systems: A model and empirical test. Journal of Management Information Systems, 28(1), 235–272. doi: https://doi.org/10.2753/MIS0742-1222280108
- Hsu, J. Y., Chen, C. C., & Ting, P. F. (2018). Understanding MOOC continuance: An empirical examination of social support theory. Interactive Learning Environments, 26(8), 1100–1118. doi: https://doi.org/10.1080/10494820.2018.1446990
- Hsu, C. L., & Lu, H. P. (2004). Why do people play on-line games? An extended TAM with social influences and flow experience. Information & Management, 41(7), 853–868. doi: https://doi.org/10.1016/j.im.2003.08.014
- Hu, P. J. H., Clark, T. H., & Ma, W. W. (2003). Examining technology acceptance by school teachers: A longitudinal study. Information & Management, 41(2), 227–241. doi: https://doi.org/10.1016/S0378-7206(03)00050-8
- Huang, L., Zhang, J., & Liu, Y. (2017). Antecedents of student MOOC revisit intention: Moderation effect of course difficulty. International Journal of Information Management, 37(2), 84–91. doi: https://doi.org/10.1016/j.ijinfomgt.2016.12.002
- Jacobs, A. J. (2013). Two cheers for Web U!. New York Times, 162(56113), 1–7.
- Jo, D. (2018). Exploring the determinants of MOOCs continuance intention. KSII Transactions on Internet & Information Systems, 12(8), 3992–4005.
- Laaser, W., & Toloza, E. (2017). The changing role of the educational video in higher distance education. The International Review of Research in Open and Distributed Learning, 18(2), 264–276. doi: https://doi.org/10.19173/irrodl.v18i2.3067
- Lai, H.-J. (2018). Investigating older adults’ decisions to use mobile devices for learning, based on the unified theory of acceptance and use of technology. Interactive Learning Environments. doi:https://doi.org/10.1080/10494820.2018.1546748.
- Li, X., & Li, Z. (2016, December). The application of information technology in neurobiology education. In 2016 8th International conference on information technology in medicine and education (ITME) (pp. 310–313). IEEE.
- Li, B., Wang, X., & Tan, S. C. (2018). What makes MOOC users persist in completing MOOCs? A perspective from network externalities and human factors. Computers in Human Behavior, 85, 385–395. doi: https://doi.org/10.1016/j.chb.2018.04.028
- Lian, J. W., & Yen, D. C. (2014). Online shopping drivers and barriers for older adults: Age and gender differences. Computers in Human Behavior, 37, 133–143. doi: https://doi.org/10.1016/j.chb.2014.04.028
- Liao, Q., Shim, J. P., & Luo, X. (2004). Student acceptance of web-based learning environment: An empirical investigation of an undergraduate IS course. In Proceedings of the 10th Americas conference on information systems (AMCIS) (p. 377).
- Liyanagunawardena, T. R., Adams, A. A., & Williams, S. A. (2013). MOOCs: A systematic study of the published literature 2008–2012. The International Review of Research in Open and Distributed Learning, 14(3), 202–227. doi: https://doi.org/10.19173/irrodl.v14i3.1455
- Ma, W. W. K., Andersson, R., & Streith, K. O. (2005). Examining user acceptance of computer technology: An empirical study of student teachers. Journal of Computer Assisted Learning, 21(6), 387–395. doi: https://doi.org/10.1111/j.1365-2729.2005.00145.x
- Ma, L., & Lee, C. S. (2019a). Investigating the adoption of MOOC s: A technology–user–environment perspective. Journal of Computer Assisted Learning, 35(1), 89–98. doi: https://doi.org/10.1111/jcal.12314
- Ma, L., & Lee, C. S. (2019b). Understanding the barriers to the use of MOOCs in a developing country: An innovation resistance perspective. Journal of Educational Computing Research, 57(3), 571–590. doi: https://doi.org/10.1177/0735633118757732
- Mei, B., Brown, G. T., & Teo, T. (2018). Toward an understanding of preservice English as a Foreign language teachers’ acceptance of computer-assisted language learning 2.0 in the people’s Republic of China. Journal of Educational Computing Research, 56(1), 74–104. doi: https://doi.org/10.1177/0735633117700144
- Mtebe, J. S., Mbwilo, B., & Kissaka, M. M. (2016). Factors influencing teachers’ use of multimedia enhanced content in secondary schools in Tanzania. The International Review of Research in Open and Distributed Learning, 17(2), 65–84. doi: https://doi.org/10.19173/irrodl.v17i2.2280
- Nikou, S. A., & Economides, A. A. (2019). Factors that influence behavioral intention to use mobile-based assessment: A STEM teachers’ perspective. British Journal of Educational Technology, 50(2), 587–600. doi: https://doi.org/10.1111/bjet.12609
- OECD. (2007). Giving knowledge for free: The emergence of open educational resources. Retrieved from http://www.oecd.org/education/ceri/38851849.pdf
- Olasina, G. (2019). Human and social factors affecting the decision of students to accept e-learning. Interactive Learning Environments, 27(3), 363–376. doi: https://doi.org/10.1080/10494820.2018.1474233
- Oliveira, T., Faria, M., Thomas, M. A., & Popovič, A. (2014). Extending the understanding of mobile banking adoption: When UTAUT meets TTF and ITM. International Journal of Information Management, 34(5), 689–703. doi: https://doi.org/10.1016/j.ijinfomgt.2014.06.004
- Oliveira, T., Thomas, M., Baptista, G., & Campos, F. (2016). Mobile payment: Understanding the determinants of customer adoption and intention to recommend the technology. Computers in Human Behavior, 61, 404–414. doi: https://doi.org/10.1016/j.chb.2016.03.030
- Pynoo, B., Devolder, P., Tondeur, J., Van Braak, J., Duyck, W., & Duyck, P. (2011). Predicting secondary school teachers’ acceptance and use of a digital learning environment: A cross-sectional study. Computers in Human Behavior, 27(1), 568–575. doi: https://doi.org/10.1016/j.chb.2010.10.005
- Pynoo, B., Tondeur, J., Van Braak, J., Duyck, W., Sijnave, B., & Duyck, P. (2012). Teachers’ acceptance and use of an educational portal. Computers & Education, 58(4), 1308–1317. doi: https://doi.org/10.1016/j.compedu.2011.12.026
- Ringle, C. M., Wende, S., & Becker, J.-M. (2015). SmartPLS 3. Bönningstedt: SmartPLS. Retrieved from http://www.smartpls.com
- Rodriguez, C. O. (2012). MOOCs and the AI-Stanford like courses: Two successful and distinct course formats for massive open online courses. European Journal of Open, Distance and E-Learning. Retrieved from https://files.eric.ed.gov/fulltext/EJ982976.pdf
- Scherer, R., Siddiq, F., & Teo, T. (2015). Becoming more specific: Measuring and modeling teachers’ perceived usefulness of ICT in the context of teaching and learning. Computers & Education, 88, 202–214. doi: https://doi.org/10.1016/j.compedu.2015.05.005
- Shao, Z. (2018). Examining the impact mechanism of social psychological motivations on individuals’ continuance intention of MOOCs: The moderating effect of gender. Internet Research, 28(1), 232–250. doi: https://doi.org/10.1108/IntR-11-2016-0335
- Sharma, S. K., Sarrab, M., & Al-Shihi, H. (2017). Development and validation of mobile learning acceptance measure. Interactive Learning Environments, 25(7), 847–858. doi: https://doi.org/10.1080/10494820.2016.1224250
- Statista. (2015). Percentage of faculty worldwide who support less traditional and digital education models as of March 2015. Retrieved from https://proxy.statista.com/statistics/548257/digital-education-faculty-support-worldwide/
- Stofega, W., & Llamas, R. T. (2009). Worldwide mobile phone 2009–2013 forecast update. IDC Document Number 217209, IDC, Framingham, MA.
- Teo, T. (2009). Modelling technology acceptance in education: A study of pre-service teachers. Computers & Education, 52(2), 302–312. doi: https://doi.org/10.1016/j.compedu.2008.08.006
- Teo, T. (2014). Unpacking teachers’ acceptance of technology: Tests of measurement invariance and latent mean differences. Computers & Education, 75, 127–135. doi: https://doi.org/10.1016/j.compedu.2014.01.014
- Teo, T., Lee, C. B., & Chai, C. S. (2008). Understanding pre-service teachers’ computer attitudes: Applying and extending the technology acceptance model. Journal of Computer Assisted Learning, 24(2), 128–143. doi: https://doi.org/10.1111/j.1365-2729.2007.00247.x
- Teo, T., & Noyes, J. (2014). Explaining the intention to use technology among pre-service teachers: A multi-group analysis of the unified theory of acceptance and use of technology. Interactive Learning Environments, 22(1), 51–66. doi: https://doi.org/10.1080/10494820.2011.641674
- Teo, T., Sang, G., Mei, B., & Hoi, C. K. W. (2019). Investigating pre-service teachers’ acceptance of Web 2.0 technologies in their future teaching: A Chinese perspective. Interactive Learning Environments, 27(4), 530–546. doi: https://doi.org/10.1080/10494820.2018.1489290
- Venkatesh, V., Morris, M. G., Davis, G. B., & Davis, F. D. (2003). User acceptance of information technology: Toward a unified view. MIS Quarterly, 27(3), 425–478. doi: https://doi.org/10.2307/30036540
- Venkatesh, V., Thong, J. Y., Chan, F. K., Hu, P. J. H., & Brown, S. A. (2011). Extending the two-stage information systems continuance model: Incorporating UTAUT predictors and the role of context. Information Systems Journal, 21(6), 527–555. doi: https://doi.org/10.1111/j.1365-2575.2011.00373.x
- Venkatesh, V., Thong, J. L., & Xu, X. (2016). Unified theory of acceptance and use of technology: A synthesis and the road ahead. Journal of the Association for Information Systems, 17(5), 328–376. doi: https://doi.org/10.17705/1jais.00428
- Venkatesh, V., Thong, J. Y., & Xu, X. (2012). Consumer acceptance and use of information technology: Extending the unified theory of acceptance and use of technology. MIS Quarterly, 36(1), 157–178. doi: https://doi.org/10.2307/41410412
- Voss, B. D. (2013). Massive open online courses (MOOCs): A primer for university and college board members. Washington, DC: AGB Association of Governing Boards of Universities and Colleges.
- Wang, Y.-S., Wu, M.-C., & Wang, H.-Y. (2009). Investigating the determinants and age and gender differences in the acceptance of mobile learning. British Journal of Educational Technology, 40(1), 92–118. doi: https://doi.org/10.1111/j.1467-8535.2007.00809.x
- Wong, G. K. (2016). The behavioral intentions of Hong Kong primary teachers in adopting educational technology. Educational Technology Research and Development, 64(2), 313–338. doi: https://doi.org/10.1007/s11423-016-9426-9
- Wu, B., & Chen, X. (2017). Continuance intention to use MOOCs: Integrating the technology acceptance model (TAM) and task technology fit (TTF) model. Computers in Human Behavior, 67, 221–232. doi: https://doi.org/10.1016/j.chb.2016.10.028
- Yang, H. H., & Su, C. H. (2017). Learner behaviour in a MOOC practice-oriented course: In empirical study integrating TAM and TPB. The International Review of Research in Open and Distributed Learning, 18(5), 35–63. doi: https://doi.org/10.19173/irrodl.v18i5.2991
- Yoo, S. J., Han, S. H., & Huang, W. (2012). The roles of intrinsic motivators and extrinsic motivators in promoting e-learning in the workplace: A case from South Korea. Computers in Human Behavior, 28(3), 942–950. doi: https://doi.org/10.1016/j.chb.2011.12.015
- Zhang, J. (2016). Can MOOCs be interesting to students? An experimental investigation from regulatory focus perspective. Computers & Education, 95, 340–351. doi: https://doi.org/10.1016/j.compedu.2016.02.003
- Zhou, J. (2017). Exploring the factors affecting learners’ continuance intention of MOOCs for online collaborative learning: An extended ECM perspective. Australasian Journal of Educational Technology, 33(5), 127–135.
- Zhou, M. (2016). Chinese university students’ acceptance of MOOCs: A self-determination perspective. Computers & Education, 92–93, 194–203. doi: https://doi.org/10.1016/j.compedu.2015.10.012
- Zhou, T., Lu, Y., & Wang, B. (2010). Integrating TTF and UTAUT to explain mobile banking user adoption. Computers in Human Behavior, 26(4), 760–767. doi: https://doi.org/10.1016/j.chb.2010.01.013