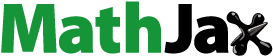
ABSTRACT
Computer-based virtual learning environments (CBVLEs) have attracted attention as a learning innovation that can foster students’ self-efficacy and intrinsic motivation. Research on the instructional design regarding these aspects of learning in a virtual learning environment is rather piecemeal. This study investigates the instructional design of a CBVLE for mathematical medication learning by nursing students in vocational education. The instructional design was based on a task-centered approach, and students’ future learning tasks formed the backbone. We examine the extent to which the CBVLE fostered the nursing students’ mathematical learning, self-efficacy, and intrinsic motivation, and the ways in which the design components of the CBVLE met nursing students’ satisfaction. In total, 118 nursing students were assigned to four groups, with or without extra support from worked examples, and were trained via the CBVLE on mathematical medication learning tasks over four consecutive weeks. Students were pre- and post-tested on their mathematical medication learning, self-efficacy, and intrinsic motivation. Students also rated their satisfaction with the instructional design. Our results showed that the CBVLE fostered nursing students’ mathematical medication learning, self-efficacy, and intrinsic motivation, but no significant differences were found between the four conditions. Overall, student satisfaction was above average. The design components were able to predict nursing students’ mathematical medication learning, self-efficacy, and intrinsic motivation.
1. Introduction
This study focuses on the instructional design of a computer-based virtual learning environment (CBVLE) for mathematical medication learning by nursing students in vocational education. The mathematical medication process is complex, and requires conceptual understanding that includes both a theoretical knowledge of medicines and a domain-specific mathematical knowledge of medication (Prins et al., Citation2019). Domain-specific mathematical knowledge also involves a knowledge of the underlying unifying mathematical principles (Canobi, Citation2009). In current traditional educational programmes, mathematical medication learning is often taught in the form of fragmented mathematics lessons that are too different from the future professional competence of nursing students, and the main emphasis is placed on passing the mathematics test rather than learning. It has been noted that “when instructional programmes are assessed by traditional assessments they tend to get reduced to a ‘teach for efficiency’ profile” (Bransford et al., Citation2005, p. 52).
An alternative that enables teachers to prepare nursing students for future employment and shift assessments of students’ abilities in a knowledge-rich environment is to use a CBVLE (Bransford et al., Citation2005). A CBVLE can be used to impart an in-depth knowledge of mathematics in relation to the medication skills of nursing students. CBVLEs offer educational opportunities, such as place- and time-independent learning, that traditional on-site learning environments cannot offer (Van Merriënboer & Kirschner, Citation2018; Zwart et al., Citation2020). CBVLEs are particularly interesting in terms of the teaching of more concrete academic concepts (Buchanan, Citation2003), as they enable students to instantly experience situations that are similar to real-life scenarios, such as nursing tasks involving the medication process. CBVLEs can increase the diversity of learning experiences and provide flexible and meaningful instruction by combining teaching of the mathematical medication process and domain-specific mathematical knowledge without constraints on time and place (Zwart et al., Citation2020).
CBVLEs can enable self-directed learning by enhancing students’ self-efficacy and boosting their intrinsic motivation (e.g. Huang et al., Citation2013; Xinhao & Fengfeng, Citation2016). Progress has been made in terms of design principles that allow for more or less learning via CBVLEs (e.g. Makransky et al., Citation2019), but research in this area is rather piecemeal (Boyle et al., Citation2016), and the ways in which these design principles facilitate students’ learning, self-efficacy, and intrinsic motivation remain unclear.
In this study, we are interested in the extent to which these instructional design principles are related to student satisfaction in terms of mathematical medication learning via the CBVLE. Furthermore, the aim is to investigate the extent to which such instructional design principles facilitate students’ mathematical medication learning, self-efficacy, and intrinsic motivation for mathematical medication learning via the CBVLE.
2. Theoretical background
2.1. Computer-based virtual learning environments
CBVLEs are digital environments based on desktop virtual reality (VR) that allow the users to dynamically interact with learning materials via 3D images on a computer screen (Lee et al., Citation2010; Merchant et al., Citation2012). CBVLEs can be defined as simulated learning environments containing various problem-solving scenarios (Wang et al., Citation2018). Students use a keyboard or mouse to navigate through the CBVLE. A CBVLE enables the user to specify both the relevant elements in a domain and the relationships between these elements, and are designed with VR features. According to Merchant et al. (Citation2012), the two main VR features are “representational fidelity” and “learners’ interaction” (see Dalgarno & Lee, Citation2010). Since Merchant et al. (Citation2012) used the same innovative 3D technology in Second Life® as in this study, we apply the same definitions here. In their work, they stated: “Representational fidelity refers to the realistic display of the virtual environment that can be attained by physical characteristics of the environment. In the context of this study, these are rich graphics such as hospital tents, doctors, and patients. Learners’ interaction is the ability of users to influence the occurrences of events in the virtual environment by their actions” (Merchant et al., Citation2012, p. 552). In the context of this study, the learners are students who log in into the Second Life® platform as nursing avatars to help doctors and their patients with their medication issues. These VR features offer opportunities to display interactive stories representing the students’ future professional tasks. The graphical design of the CBVLE allows for a realistic representation, since a high degree of realism can cognitively engage students and motivate them to learn (Csikszentmihalyi, Citation1990; Shute et al., Citation2009). Given that the students’ learning tasks correspond to those that will be performed in future in the workplace, these tasks can serve as encouragement for learning (Merchant et al., Citation2014), and are therefore essential for instructional design via technology. Designing instruction for VR involves developing and adapting instructional strategies to new technology. In order to represent the tasks that students will undertake in their future employment, the instructional design benefits from a task-focused approach.
2.2. Instructional design of the computer-based virtual learning environment
Van Merriënboer and Kirschner (Citation2018) developed a task-centred design approach in which the design of the instruction is based on real-life tasks. This task-centred design approach, described as four-component instructional design for complex learning (4C/ID), takes advantage of a holistic approach to design learning environments. The four basic components are (i) learning tasks, (ii) supportive information, (iii) procedural information, and (iv) part-task practice. This overcomes the problems of compartmentalisation and fragmentation of traditional instructional design models, and hence is a particularly interesting design model for teaching academic concepts via CBVLE. Each of these four basic components are described in more detail below.
2.2.1. The learning tasks design component of a CBVLE
Learning tasks form the backbone of the CBVLE learning programme. “The learning task confronts the learner with all or almost all of the constituent skills important for real-life task performance, together with their associated knowledge and attitudes. The learning tasks are meaningful, authentic, and representative for the tasks that a professional might encounter in the real world” (Van Merriënboer & Kirschner, Citation2007, p. 7). Learning tasks encourage students to learn; when the motive for learning arises from the activity itself, the activity of learning helps students to successfully complete learning tasks (Merchant et al., Citation2014; Pajares & Schunk, Citation2001). Practising many different activities, such as domain-specific types of problems, permits students to grasp learning procedures and make connections (Dunlosky et al., Citation2013; Rohrer et al., Citation2015). Although learning via CBVLEs should be challenging, it is easy to place excessive demands on students with activities that cause cognitive overload (Sweller, Citation2010), and this may result in negative emotions (Pekrun, Citation2006). One way to support students is to add supportive information into the CBVLE.
2.2.2. The supportive information design component of a CBVLE
According to Van Merriënboer and Kirschner (Citation2018), supportive information explains to students how a learning domain is organised and how to approach problems in that domain. Supportive information is necessary for students to develop cognitive models and strategies for completing learning tasks (Frerejean et al., Citation2019). For example, the future medication tasks of nursing students involve a range of nursing activities such as verifying, preparing, checking, administering, registering, monitoring, and evaluating medication. Calculations are necessary for almost all of these tasks. Seen in terms of the instructional design model of Van Merriënboer and Kirschner (Citation2018), the first two components of 4C/ID, learning tasks and supportive information, can help students to build cognitive schemas through the acquisition of new knowledge that contributes to their existing knowledge. The other two components, procedural information and part-task practice, stimulate the automation of schemas and the development of automatic, task-specific procedures that can be applied without undue demand on cognitive processing resources (Frerejean et al., Citation2019, p. 516).
2.2.3. The procedural information design component of a CBVLE
Procedural information specifies how to perform recurrent aspects of the learning tasks (Van Merriënboer & Kirschner, Citation2018), and involves corrective feedback and demonstrations of rules, procedures, and prerequisite knowledge. It should be displayed “just in time”. Instructional activities that can show students how to apply procedures to specific problems in a CBVLE include worked examples (Chen et al., Citation2016; Kirschner et al., Citation2018; Van Gog et al., Citation2011). While solving a problem in the CBVLE, students can click and open a worked example that provides them with step-by-step instruction. A CBVLE could also be pre-programmed with feedback on domain-specific knowledge. When students’ responses to the problems are incorrect, they receive corrective verification feedback in the CBVLE (Maier et al., Citation2016). Moreover, detailed feedback on domain-specific knowledge can draw students’ attention to the domain-specific knowledge needed to correctly execute task-specific procedures. This feedback can support reflection and improvement, and is crucial for learning and achievement (Yuang et al., Citation2020). When students cannot master certain aspects of a learning task, part-task practice can be virtually embedded.
2.2.4. The part-task practice design component of a CBVLE
The use of part-task practice to train one or more selected recurrent aspects of learning tasks is an instructional strategy for recurrent constituent skills that are critical to safety (Van Merriënboer & Kirschner, Citation2018). Mathematical mistakes in the medication process can have serious consequences, meaning that the inclusion of exercises in the CBVLE that develop an in-depth knowledge of the underlying domain-specific mathematical knowledge is necessary if a high level of automation is desired. Part-task practice can be treated as a feedback facilitator for students’ understanding and performance (Yuang et al., Citation2020). In this way, students can make connections between the underlying knowledge and the problems to be solved (Tynjälä, Citation2013).
The monitoring of students’ learning processes also involves their reflections on learning. Van Merriënboer and Kirschner (Citation2018) place this under the category of cognitive feedback; however, reflection on learning is not simply feedback on the quality of students’ learning. In the context of this study, reflection on learning is implemented as a self-assessment exercise regarding students’ mathematical medication learning results, in terms of their experience of the whole learning task. We therefore describe this step separately as a design component of the CBVLE.
2.3. Cognitive feedback as a reflection on learning
Feedback is a process in which students interpret comments about the quality of their work as input for the development of their future performance or learning strategies (Carless, Citation2019; Latifi et al., Citation2021). Ideally, feedback contains both cognitive and affective features (Nelson & Schunn, Citation2009): cognitive features influence performance and understanding, allowing students to know what to improve, whereas affective features relate to the students’ agreement and disagreement with feedback. Feedback must enable students in the CBVLE to move from an initial state of mind to a desired state of mind (Mor et al., Citation2015). Reflection on learning via the CBVLE stimulates students to identify their strengths and weaknesses in terms of the actions taken for learning. This computer-based feedback on learning provides information drawn from the analysis of learning logs, and is based on each student’s performance. gives an overview of the implementation of the design components of the instructional design of the CBVLE in this study.
Table 1. Overview of the design components of the task-centered instructional design of the CBVLE.
Since the CBVLE is a structured system in which activities, exercises, and support form an integral part of the virtual educational learning environment, it can increase nursing students’ intrinsic motivation and enhance their self-efficacy (Merchant et al., Citation2014; Pekrun, Citation2006).
2.4. Self-efficacy and intrinsic motivation in a cvble
Self-efficacy refers to the student’s judgements of their own level of competence in dealing with prospective situations (Bandura, Citation1982). Pajares and Schunk (Citation2001) state that the successful completion of learning tasks contributes to students’ self-efficacy. Self-efficacy is also enhanced when students value the task that they are undertaking (Schunk & DiBenedetto, Citation2016) and is positively associated with motivation, which in turn enhances the learning outcomes of students (Schunk & DiBenedetto, Citation2016). When students are intrinsically motivated to learn, their satisfaction is associated with the performance of learning tasks (Ryan & Deci, Citation2000). In fact, it has been shown that the relevant outcomes are of less importance than the activities themselves (Pekrun & Stephens, Citation2010). Interactions in CBVLEs can enable students’ self-directed learning and can stimulate their intrinsic motivation and contribute to their self-efficacy (e.g. Huang et al., Citation2013; Lee et al., Citation2010; Xinhao & Fengfeng, Citation2016), but this depends on whether students are satisfied with the instructional design of the CBVLE. Hence, with respect to the issues of mathematical medication learning, self-efficacy, and intrinsic motivation, the research questions addressed in this study are as follows:
What are the effects of the instructional design in a CBVLE in terms of facilitating nursing students’ mathematical medication learning, self-efficacy, and intrinsic motivation for learning via the CBVLE?
To what extent are nursing students satisfied with the instructional design, and how do the design components account for nursing students’ mathematical medication learning, self-efficacy, and intrinsic motivation for learning via the CBVLE?
3. Method
3.1. Context and participants
The study took place at five post-secondary vocational nursing schools (N = 44) and at seven universities of applied sciences (N = 74) in the Netherlands. The participants, who were compensated with a €15 voucher for their contribution, were 118 students enrolled in these schools. The educational levels of the participants were different, but the mathematical medication training and its content was the same in both educational programmes. The mean age of the participants was 19.6 (SD = 2.4), and 92% were female. Students worked on a bring your own device (BYOD) basis in this study, except for two schools in which students used PCs, but they all worked with the same platform (Second Life®) in the same virtual learning environment. Although students had basic computer skills (Mpre = 3.7, SD = 0.7; Min = 1.0, Max = 5.0), working with a CBVLE was new to them.
All students were provided with equivalent learning content in the CBVLE. Moreover, the CBVLE was the same for all students, and provided a structured learning environment based on the task-centred design approach with the five instructional design components (). All students received their assignments via doctors, and all students received verification feedback and elaboration feedback on domain-specific knowledge that was pre-programmed in the CBVLE. The students performed the tasks on their own. However, the support arrangements in the CBVLE differed. Students were randomly assigned to one of four groups with different support conditions: mathematical medication learning via the CBVLE without worked examples (condition 1); mathematical medication learning via the CBVLE with worked examples involving domain-specific knowledge (condition 2); mathematical medication learning via the CBVLE with worked examples involving regular thinking strategies (condition 3); and mathematical medication learning via the CBVLE with a combination of both types of worked example (condition 4).
3.2. Learning materials
The subject matter to be learned involved the concept of mathematical medication, which included three mathematical domains: liquid medication, infusion of fluids, and solid medication. These different mathematical domains involve different mathematical rules, and students need to master these. The details of the learning tasks and the principles of the underlying design and mathematical content are summarised in .
Table 2. Learning tasks involving domain-specific mathematical medication knowledge related to liquid medication, infusion of fluids and solid medication in a CBVLE.
About 30 scenarios were developed for each domain, at three levels (easy, difficult, and very difficult), so that students could practice each type of problem at different levels and multiple times in the CBVLE. Almost all the constituent skills that are important for the future performance of nursing students in relation to mathematical medication tasks were included. In this way, the skills important for real-life task performance could be trained, together with their associated knowledge and attitudes (Van Merriënboer & Kirschner, Citation2018). illustrates the procedure of mathematical medication learning via the CBVLE. shows four screenshots of the CBVLE environment.
3.3. Procedure
A pilot test was conducted with 11 students to determine the feasibility of the study with respect to the introduction, learning tasks, materials, instruments, feedback, and platform. This pilot study resulted in a slight modification to the introduction section to include an extra short meeting, and a paper-based manual that was provided before and during the exercises. We also introduced an instruction in the CBVLE, using photos with a description of the simulation script to guide students in their initial activities in the hospital field.
The experimental session consisted of four main phases (see ). During the introduction and personal data phase (1), which took 70 min, students received introductory explanations about the CBVLE training. Students were then assigned to avatars and logged into the CBVLE. These avatars (students) were randomly allocated to one of the four groups.
Table 3. Procedure used for the CBVLE study.
Students carried out one practice round in the CBVLE, and it was made clear that they were expected to work individually during the game, without help from the researcher or their peers, while paying attention to the information on the screen (30 min). Students were then asked to complete a questionnaire on their personal data, including their name, gender, age, and computer skills (10 min).
During the individual phase (2), students first received an introductory explanation of how to fill in the questionnaire and a description of the domain-specific mathematical knowledge test (5 min). Next, they were asked to fill in a questionnaire (20 min) on intrinsic motivation and self-efficacy, and then to complete the domain-specific mathematical knowledge test (60 min).
From the second week onwards, the learning phase (3) took 90 min per week, over four consecutive weeks for each student. In those weeks the students work individually in the CBVLE and performed their own mathematical medication learning tasks during 90 min per week. Students completed the domain-specific mathematical knowledge test (60 min) during the post-test phase (4) in the sixth week and were then given two questionnaires: the first measured self-efficacy and intrinsic motivation, and the second measured their satisfaction with the components of the instructional design of the CBVLE (30 min). Finally, the participants received a short debriefing for about 10 min.
3.4. Measurements and instruments
3.4.1. Measurement of nursing students’ mathematical medication knowledge
Domain-specific mathematical medication knowledge was assessed via a test provided by Cito for this research (the Dutch national organisation for test development; see Lampe et al., Citation2011). This test was based on the domain-specific mathematical medication content and measured the domain-specific mathematical medication knowledge of the nursing students. Cito offered two parallel versions, which were applied before and after intervention with the CBVLE. The original domain-specific mathematical medication knowledge test consisted of 50 multiple choice questions, with 20 questions on domain-specific mathematical medication knowledge on the infusion of fluids, 19 on liquid medication, and 11 on solid medication (tablets). An item response analysis showed that four items in both versions had poor discriminatory power. These items were eliminated so that students could obtain a maximum score of 46 for both tests. The reliability for the pre-test was good (Cronbach’s α = .80). The post-test reliability was also good (α = .83).
3.4.2. Measurement of nursing students’ self-efficacy and intrinsic motivation
Self-efficacy and intrinsic motivation were assessed using a questionnaire with 12 items on a five-point Likert scale ranging from “fully disagree” to “fully agree”. The questionnaire was designed by Oprins et al. (Citation2015) and adjusted for this experiment. Seven items of this questionnaire asked students to ascertain the extent to which they believed in successfully completing learning tasks (self-efficacy); for example, they were asked to rate their own expertise in mathematical medication and to assess themselves against the other students. The reliability coefficients were high in both the pre-assessment (α = .81) and in the post-assessment (α = .86). Five items of this questionnaire aimed to ascertain students’ intrinsic motivation towards mathematical medication; for example, they were asked to rate whether mathematical medication lessons held any excitement for them and their level of pleasure during these lessons. The reliability coefficient was satisfactory at the pre-assessment stage (α = .78) and high at the post-assessment stage (α = . 84).
3.4.3. Measurement of nursing students’ satisfaction with the instructional design
Using a modified questionnaire from Oprins et al. (Citation2015), students were asked to score their satisfaction with the components of the instructional design of the CBVLE after training. On a five-point Likert scale ranging from “fully disagree” to “fully agree”, students rated their satisfaction with the instructional design. These items could be related to the design components of the 4C/ID model (Van Merriënboer & Kirschner, Citation2018); for example, students were asked whether they could relate to a particular character, and whether what they learned could be transferred to different situations. The reliability coefficient of the questionnaire was high (Cronbach’s α = .79).
3.4.3.1. Ethics
Students freely participated in this study. After giving active informed consent and receiving an introductory verbal explanation about the aims of the study, students could still decline to take part. Students were assured that identifying information would not be accessible by anyone except the researchers. Results were de-identified by numbering both the students and their tests, and files were held in different places under different names. The Faculty Ethics Review Committee (FETC) of the Faculty of Social Sciences of Utrecht University in the Netherlands reviewed and approved this research study, under case number 19-230.
3.4.3.2. Data analysis
A total of 118 students participated in the study. Due to external circumstances (scheduling, internships, illness) and missing data, around 10% of the students dropped out of the study. Our results were based on analyses of data on the 101 students who completed all the sessions and tests. Repeated measure ANOVAs were applied in order to answer the first research question regarding the individual acquisition of mathematical medication learning, the self-efficacy and the intrinsic motivation of the nursing students in each of the four training groups. Post-hoc tests were conducted to determine the significant differences between the four training groups. For the second research question, the questionnaire items were tested with an ANOVA to check whether there were differences between the satisfaction levels of nursing students learning under the four different conditions. Multiple regression analyses were also applied to determine whether each combination of design components accounted for the mathematical medication learning, self-efficacy, and intrinsic motivation of the nursing students.
4. Results
For the first research question, we found significant differences between the pre-test and post-test stages in the mathematical medication learning of the nursing students, F(1,97) = 119.80, p < .001, η2 = .55. We found no significant differences in mathematical medication learning between the four different training conditions, F(3,97) = 1.36, p = .26.
The students’ outcomes in terms of self-efficacy showed significant differences between the pre-test and post-test stages, F(1,88) = 17.64, p < .001, η2 = .17. The different training conditions in the CBVLE did not give rise to any significant differences in the self-efficacy of the nursing students, F(3,88) = 2.31, p = .08.
With respect to the students’ intrinsic motivation, significant differences were found between the pre-test and post-test stages, F(1,85) = 33.69, p < .001, η2 = .28. No differences were observed between the four training conditions in the CBVLE in terms of the intrinsic motivation outcomes, F(3,85) = .25, p = .86. shows the values for the mean and standard deviation for the mathematical medication learning, self-efficacy, and intrinsic motivation of nursing students under the four training conditions.
Table 4. Summary of outcomes.
For the second research question, we found that the satisfaction of the students with the components of the design were above average (see for mean scores per design component), but no differences were found between the four training conditions, F(3,93) = 0.37, p = .08.
Table 5. Outcomes for nursing students’ satisfaction with the instructional design components.
In combination, the students’ satisfaction with the components of the instructional design did significantly account for their mathematical medication learning, R² = .21, F(5,87) = 4.48, p < .001. Significant results were also obtained for satisfaction with the components of the instructional design and self-efficacy, R² = .38, F(5,88) = 10.72, p < .001, and intrinsic motivation, R² = .47, F(5,84) = 15.11, p < .001.
5. Conclusion and discussion
This study investigated the instructional design of a CBVLE for mathematical medication learning by nursing students in (higher) vocational education. The outcomes of the study showed that training via the CBVLE did enhance the mathematical medication learning, self-efficacy, and intrinsic motivation of these students; however, no differences were found between the results for the students in the four groups. We also accounted for the students’ satisfaction with the instructional design: they were satisfied with the separate design components to an above average level, but no differences were found between the four groups. The nursing students were satisfied with the instructional design of the CBVLE. We found that a small variance in the mathematical learning results could be explained by the combination of the instructional components. The combination of the instructional components explained a medium level of variance in self-efficacy and intrinsic motivation for learning with the CBVLE. The structure of the learning environment in the CBVLE outlined a mathematical medication task and students had access to a great deal of information via verification feedback and elaborated feedback on domain-specific knowledge that was pre-programmed for them in the CBVLE. The additional worked examples used in some groups may have introduced redundancy; however, this did not negatively influence students’ satisfaction with the instructional design components.
With regard to the design component of learning tasks, positive emotions were associated with the nursing students’ future professional medication tasks, as they believed these to be important and this influenced their learning behaviour (Csikszentmihalyi, Citation1990; Wigfield & Eccles, Citation2000). Most of the nursing students (80%) confirmed that they were able to relate to the character of the nurse avatar, and that the virtual learning environment was sufficiently realistic for their learning goals. These results reflect Csikszentmihalyi’s (Citation1990) “presence”, which was defined as a realistic and attractive learning environment that engages students and motivates them to learn. It should be noted that we chose not to make the environment realistic, and it simply represented a virtual encampment. However, we analysed a real professional learning task, and used strategies to structure this learning task in the CBVLE. The efforts made by the students in terms of mathematical medication learning might therefore have been driven by the relevance of these learning tasks. This corresponds to the findings of Grigg et al. (Citation2018), who state that students with higher interest in learning tasks enjoy learning, which leads to better task performance.
In terms of the design component of supportive information, the learning domain was organised in such a way that it enabled the students to understand how the mathematical medication domain was organised and how they should complete the learning tasks (Frerejean et al., Citation2019; Van Merriënboer & Kirschner, Citation2018). Self-directed learning was therefore programmed by navigating students towards activities involving the learning goals, and students were unable to control the virtual environment. In contrast to the findings of Bandura (Citation1993), who stated that students’ self-efficacy was influenced by their ability to control the environment, our study showed that controlling the environment seemed less important to nursing students, as they preferred to focus on the mathematical medication task. This was also illustrated by the students’ satisfaction with the design component of supportive information, since 77% of the nursing students were satisfied to an above average level with the choices made for this design component.
For the design component of procedural information, we included worked examples that enabled nursing students to apply procedures to specific mathematical medication problems (Chen et al., Citation2016; Kirschner et al., Citation2018; Van Gog et al., Citation2011). Although these worked examples were embedded to support students, this did not lead to different outcomes from the four different learning conditions. The demonstrations of rules and procedures in the worked examples, which were displayed “just in time”, showed only one standard example per domain-specific mathematical medication problem. This may have posed a challenge to students in terms of finding out how the rules and procedures fitted their own mathematical medication problems that they needed to solve. These worked examples could therefore have caused cognitive overload (Kalyuga et al., Citation2001; Sweller, Citation2010), due to the presence of other feedback elements in the CBVLE that already supported nursing students in solving the mathematical medication problems. For example, students received corrective feedback after providing wrong answers, and also feedback on the rules of mathematical medication. An analysis by Merchant et al. (Citation2014) found that different learning tasks require different types of feedback: when learning tasks are declarative in nature, elaborate explanations seem to be more effective as feedback, while for procedural learning tasks, providing the correct response is sufficient for learning. This corroborates the results of a study by Maier et al. (Citation2016) on elaborated feedback, including explanatory information and verification feedback, in response to students’ right or wrong answers to a question. Their findings revealed that verification feedback was more effective, and the authors concluded that the text used for feedback was too long and detailed. The feedback in this CBVLE study was provided in manageable units (e.g. Shute et al., Citation2009), and the outcomes showed that this did enhance the nursing students’ feeling of competency in relation to mathematical medication learning via the CBVLE. Providing students with more cognitive approaches via the use of worked examples was therefore unnecessary.
The design component of part-task practice included short exercises on the underlying domain-specific mathematical knowledge that were embedded in the CBVLE to stimulate higher automaticity in terms of the schemas and procedures necessary to solve mathematical medication problems. However, Dunlosky et al. (Citation2013) and Makransky et al. (Citation2019) state that these practices can also be designated as a function of feedback. In addition, the exercises in this CBVLE study enabled the nursing students to make connections between their prior mathematical knowledge and new knowledge related to mathematical medication learning tasks (Tynjälä, Citation2013).
The cognitive feedback component for reflection on learning, which was introduced after six practice problems on mathematical medication and 10 short exercises, was considered to be crucial for learning and achievement (Yuang et al., Citation2020). It was intended to take the students from an initial state of mind to a desired state of mind after training on mathematical medication learning via the CBVLE (Mor et al., Citation2015). However, the nursing students were not satisfied with this component, and only 47% of them were satisfied with this design principle. This was as expected, since the cognitive feedback for reflection on learning in this study was based on a continuum of points for four levels. Each level was between two extreme values, but the ratings did not always meet nursing students’ expectations. To enable the nursing students to reach the desired level of skill after training on mathematical medication learning via the CBVLE, this cognitive feedback should be customised more specifically to the students’ own mathematical medication knowledge.
We structured the CBVLE environment using a task-centred design model, and feedback elements were incorporated that could strengthen the nursing students’ learning (Nelson & Schunn, Citation2009; Sadler, Citation2010). The CBVLE allowed nursing students to navigate their own self-directed learning, and produced cognitive engagement (Csikszentmihalyi, Citation1990). The outcomes of this study not only demonstrate that the mathematical medication learning of these students was enhanced; they also confirm the results of other studies showing that virtual learning environments support students’ self-efficacy and boost their intrinsic motivation (e.g. Huang et al., Citation2013; Lee et al., Citation2010; Xinhao & Fengfeng, Citation2016).
This study was conducted in order to show that mathematical medication learning in secondary and higher vocational nursing education can be carried out via a CBVLE with a task-centred design, rather than with a focus on one particular domain of mathematics learning (Van Merriënboer & Kirschner, Citation2018). In current vocational education programmes for nursing students, mathematics is distinguished from future professional competences in the administration of medication. Furthermore, mathematical medication learning is broken into isolated parts, and little attention is paid to structure of the domain (Zwart et al., Citation2017). It is therefore worth noting that in the Netherlands, mathematics is assessed only once in the educational programme of nursing students, and that they are then presumed to be familiar with the mathematical medication content. We designed a CBVLE specifically so that nursing students could practice mathematics in a meaningful context in relation to medication and could also maintain their knowledge and skills in this domain by periodic training via the CBVLE. When nursing students practice their mathematical medication knowledge and skills in this way, the emphasis may shift from the outcomes produced to training on the activities necessary for future mathematical medication tasks (Pekrun & Stephens, Citation2010).
Limitations
This study was a first attempt to concretise (i) the conceptual model of mathematical medication learning by classifying the objects, events and activities of the mathematical medication domain; (ii) the structural model, in order to outline how the objects, events, and activities are related to each other in order to reach specific goals; and (iii) the causal model, in order to address the effects that objects, events and activities have on each other (Van Merriënboer & Kirschner, Citation2007). Nevertheless, the results in this study should be interpreted with some caution. The study was conducted in a context that was not part of the standard curriculum, and the results may also have been affected by the reward received by students. The sample of participants was also small, which might limit the generalisability of the findings and the making of strong claims that are not based on coincidence. It is therefore recommended that this study should be replicated with more nursing students to confirm the results.
Suggestions for future research
Future research should be characterised by further development of the design of the CBVLE, and especially by an investigation of how standardised pre-programmed feedback steers nursing students’ mathematical medication learning via the CBVLE. The domain-specific knowledge of nursing students significantly improved over time, regardless of which of the four learning conditions was used in the CBVLE. This may imply that extra support from worked examples with step-by-step digital instruction involving domain-specific knowledge and regular thinking strategies in the CBVLE did not have any added value, but it could have also been caused by the passive approach of students towards dealing with worked examples (Kyun et al., Citation2013). Hence, to investigate the effectiveness of support from worked examples in the CBVLE, future research should incorporate quantitative scoring rubrics to measure nursing students’ active use of the worked examples. The use of qualitative methods to analyse nursing students’ satisfaction and ideas will also reveal more information that can be used to tailor the feedback in the CBVLE to their needs. In this way, the design could shift from an instructional design for learning to a learning design tailored to the feedback that nursing students need in order to be prepared for future mathematical medication tasks.
This study represents a first step towards influencing the science of assessment of mathematical medication learning in (higher) vocational education through providing feedback in a CBVLE. It aims to contribute to a more dynamic understanding of the integration of assessment with technology that captures activities as evidence of nursing students’ levels of mathematical medication learning (Kim et al., Citation2016).
Ethics approval
In the method presented in Section 3.5, the authors describe the ethical guidelines under which the research was carried out. The Faculty Ethics Review Committee (FETC) of the Faculty of Social Sciences of Utrecht University reviewed and approved the research study under case 19-230.
Open data
The data can be requested by e-mailing the corresponding author.
Disclosure statement
No potential conflict of interest was reported by the author(s).
References
- Bandura, A. (1982). Self-efficacy mechanism in human agency. American Psychologist, 37(2), 122–147. https://doi.org/10.1037/0003-066X.37.2.122
- Bandura, A. (1993). Perceived self-efficacy in cognitive development and functioning. Educational Psychologist, 28(2), 117–148. https://doi.org/10.1207/s15326985ep2802_3
- Boyle, E. A., Hainey, T., Connolly, T. M., Gray, G., Earp, J., Ott, M., Lim, T., Ninaus, M., Ribeiro, C., & Pereira, J. (2016). An update to the systematic literature review of empirical evidence of the impacts and outcomes of computer games and serious games. Computers & Education, 94, 178–192. https://doi.org/10.1016/j.compedu.2015.11.003
- Bransford, J., Vye, N., Stevens, R., Kuhl, P., Schwartz, D., Bell, P., Meltzoff, A., Barron, B., Pea, R., Reeves, B., Roschelle, J., & Sabelli, N. (2005). Learning theories and education: Toward a decade of synergy. In P. Alexander, & P. Winne (Eds.), Handbook of educational psychology (2nd ed.) (pp. 1–95). Erlbaum.
- Buchanan, K. (2003). Opportunity knocking: Co-opting and games. ALT-N, 43, 10–11. https://www.alt.ac.uk/sites/default/files/assets_editor_uploads/documents/ALT43weg.pdf
- Canobi, K. H. (2009). Concept-procedure interactions in children’s addition and subtraction. Journal of Experimental Child Psychology, 102(2), 131–149. https://doi.org/10.1016/j.jecp.2008.07.008
- Carless, D. (2019). Feedback loops and the longer-term: Towards feedback spirals. Assessment & Evaluation in Higher Education, 44(5), 705–714. https://doi.org/10.1080/02602938.2018.1531108
- Chen, O., Kalyuga, S., & Sweller, J. (2016). Relations between the worked example and generation effects on immediate and delayed tests. Learning and Instruction, 45, 20–30. https://doi.org/10.1016/j.learninstruc.2016.06.007
- Csikszentmihalyi, M. (1990). Flow: The psychology of optimal experience. Harper-Collins.
- Dalgarno, B., & Lee, J. W. M. (2010). What are the learning affordances of 3-D virtual environments? British Journal of Educational Technology, 41(1), 10–32. https://doi.org/10.1111/j.1467-8535.2009.01038.x
- Dunlosky, J., Rawson, K. A., Marsh, E. J., Nathan, M. J., & Willingham, D. T. (2013). Improving students’ learning With effective learning techniques. Psychological Science in the Public Interest, 14(1), 4–58. https://doi.org/10.1177/1529100612453266
- Frerejean, J., Van Merriënboer, J. J. G., Kirschner, P. A., Roex, A., Aertgeerts, B., & Marcelis, M. (2019). Designing instruction for complex learning: 4C/ID in higher education. European Journal of Education, 54(4), 513–524. https://doi.org/10.1111/ejed.12363
- Grigg, S., Perera, H. N., McIlveen, P., & Svetleff, Z. (2018). Relations among math self- efficacy, interest, intentions, and achievement: A social cognitive perspective. Contemporary Educational Psychology, 53, 73–86. https://doi.org/10.1016/j.cedpsych.2018.01.007
- Huang, W. D., Johnson, T. E., & Han, S.-H. C. (2013). Impact of online instructional game features on college students perceived motivational support and cognitive investment: A structural equation modeling study. The Internet and Higher Education, 17, 58–68. https://doi.org/10.1016/j.iheduc.2012.11.004
- Kalyuga, S., Chandler, P., Tuovinen, J., & Sweller, J. (2001). When problem solving is superior to studying worked examples. Journal of Educational Psychology, 93(3), 579–588. https://doi.org/10.1037/0022-0663.93.3.579
- Kim, Y. J., Almond, R. G., & Shute, V. J. (2016). Applying evidence-centered design for the development of game-based assessments in physics playground. International Journal of Testing, 16(2), 142–163. https://doi.org/10.1080/15305058.2015.1108322
- Kirschner, P. A., Sweller, J., Kirschner, F., & Zambrano, R. J. (2018). From cognitive load theory to collaborative load theory. International Journal of Computer-Supported Collaborative Learning, 13, 213–233. https://doi.org/10.1007/s11412-018-9277-y
- Kyun, S., Kalyuga, S., & Sweller, J. (2013). The effect of worked examples when learning to write essays in English literature. The Journal of Experimental Education, 81(3), 385–408. https://doi.org/10.1080/00220973.2012.727884
- Lampe, T., Straetmans, G., & Eggen, T. (2011). De rekenvaardigheid van de nederlandse verpleegkundige. Onderwijs en Gezondheidszorg, 35(3), 3–9. https://doi.org/10.1007/s12477-011-0030-y
- Latifi, S., Noroozi, O., Hatami, J., & Biemans, H. J. (2021). How does online peer feedback improve argumentative essay writing and learning? Innovations in Education and Teaching International, 58(2), 195–206. https://doi.org/10.1080/14703297.2019.1687005
- Lee, E. A.-L., Wong, K. W., & Fung, C. C. (2010). How does desktop virtual reality enhance learning outcomes? A structural equation modeling approach. Computers & Education, 55(4), 1424–1442. https://doi.org/10.1016/j.compedu.2010.06.006
- Maier, U., Wolf, N., & Randler, C. (2016). Effects of a computer-assisted formative assessment intervention based on multiple-tier diagnostic items and different feedback types. Computers & Education, 95, 85–98. https://doi.org/10.1016/j.compedu.2015.12.002
- Makransky, G., Terkildsen, T. S., & Mayer, R. E. (2019). Adding immersive virtual reality to a science lab simulation causes more presence but less learning. Learning and Instruction, 60, 225–236. https://doi.org/10.1016/j.learninstruc.2017.12.007
- Merchant, Z., Goetz, E. T., Cifuentes, L., Keeney-Kennicutt, W., & Davis, T. J. (2014). Effectiveness of virtual reality-based instruction on students’ learning outcomes in K-12 and higher education: A meta-analysis. Computers & Education, 70, 29–40. https://doi.org/10.1016/j.compedu.2013.07.033
- Merchant, Z., Goetz, E. T., Keeney-Kennicutt, W., Oi-man, K., Cifuentes, L., & Davis, T. J. (2012). The learner characteristics features of desktop 3D virtual reality environments, and college chemistry instruction: A structural equation modeling analysis. Computers & Education, 59(2), 551–568. https://doi.org/10.1016/j.compedu.2012.02.004
- Mor, Y., Ferguson, R., & Wasson, B. (2015). Editorial: Learning design, teacher inquiry into student learning and learning analytics: A call for action. British Journal of Educational Technology, 46(2), 221–229. https://doi.org/10.1111/bjet.12273
- Nelson, M. M., & Schunn, C. D. (2009). The nature of feedback: How different types of peer feedback affect writing performance. Instructional Science, 37(4), 375–401. https://doi.org/10.1007/s11251-008-9053-x
- Oprins, E., Visschedijk, G., Bakhuys-Roozeboom, M., Dankbaar, M., & Trooster, W. (2015). The game-based learning evaluation model (GEM): measuring the effectiveness of serious games using a standardised method. International Journal of Technology Enhanced Learning, 7(4), 326–345. https://doi.org/10.1504/IJTEL.2015.074189
- Pajares, F., & Schunk, D. H. (2001). Self-beliefs and school success: Self-efficacy, self-concept, and school achievement. In R. Riding, & S. Rayner (Eds.), Perception (pp. 239–266). Ablex.
- Pekrun, R. (2006). The control-value theory of achievement emotions: Assumptions, corollaries, and implications for educational research and practice. Educational Psychology Review, 18(4), 315–341. https://doi.org/10.1007/s10648-006-9029-9
- Pekrun, R., & Stephens, S. J. (2010). Achievement emotions in higher education. In J. C. Smart (Ed.), Higher education: Handbook of theory and research 25 (pp. 257–306). Springer. https://doi.org/10.1007/978-90-481-8598-6_7
- Prins, H., Zwart, D. P., Voogt, J., & Hettinga, M. (2019). Which professional contexts can enrich the training of medication skills of nurses through virtual reality? http://healthbytech.com/wp-content/uploads/Abstract-book-SupportingHealth-5.pdf
- Rohrer, D., Dedrick, R. F., & Stershic, S. (2015). Interleaved practice improves mathematics learning. Journal of Educational Psychology, 107(3), 900–908. https://doi.org/10.1037/edu0000001
- Ryan, R. M., & Deci, E. L. (2000). Self-determination theory and the facilitation of intrinsic motivation, social development, and well-being. American Psychologist, 55(1), 68–78. https://selfdeterminationtheory.org/SDT/documents/2000_RyanDeci_SDT.pdf https://doi.org/10.1037/0003-066X.55.1.68
- Sadler, D. R. (2010). Beyond feedback: Developing student capability in complex appraisal. Assessment & Evaluation in Higher Education, 35(5), 535–550. https://doi.org/10.1080/02602930903541015
- Schunk, D. H., & DiBenedetto, M. K. (2016). Self-efficacy theory in education. In K. R. Wentzel, & D. B. Miele (Eds.), Handbook of motivation at school 2 (pp. 34–54). Routledge. https://doi.org/10.4324/9781315773384.ch3
- Shute, V., Ventura, M., Bauer, M., & Zapata-Rivera, D. (2009). Melding the power of serious games and embedded assessment to monitor and foster learning. In U. Ritterfeld, M. Cody, & P. Vorderer (Eds.), Serious games: Mechanisms and effects (pp. 295–321). Routledge.
- Sweller, J. (2010). Element interactivity and intrinsic, extraneous, and germane cognitive load. Educational Psychology Review, 22(2), 123–138. https://doi.org/10.1007/s10648-010-9128-5
- Tynjälä, P. (2013). Toward a 3-P model of workplace learning: A literature review. Vocations and Learning, 6(1), 11–36. https://doi.org/10.1007/s12186-012-9091-z
- Van Gog, T., Kester, L., & Paas, F. (2011). Effects of worked examples, example-problem, and problem-example pairs on novices' learning. Contemporary Educational Psychology, 36, 212–218. https://doi.org/10.1016/j.cedpsych.2010.10.004
- Van Merriënboer, J. J. G., & Kirschner, P. A. (2007). Ten steps to complex learning. A systematic approach to four-component instructional design. Lawrence Erlbaum.
- Van Merriënboer, J. J. G., & Kirschner, P. A. (2018). Ten steps to complex learning (3rd ed.). Taylor & Francis.
- Wang, M., Kirschner, P. A., Spector, J. M., & Ge, X. (2018). Computer-based learning environments for deeper learning in problem-solving contexts. Computers in Human Behavior, 87, 403–405. https://doi.org/10.1016/j.chb.2018.06.026
- Wigfield, A., & Eccles, J. S. (2000). Expectancy-value theory of achievement motivation. Contemporary Educational Psychology, 25(1), 68–81. https://doi.org/10.1006/ceps.1999.1015
- Xinhao, X., & Fengfeng, K. (2016). Designing a virtual-reality-based, gamelike math learning environment. American Journal of Distance Education, 30(1), 27–38. https://doi.org/10.1080/08923647.2016.1119621
- Yuang, B., Wang, M., Van Merriënboer, J., Tao, X., Kushniruk, A., & Peng, J. (2020). Investigating the role of cognitive feedback in practice-oriented learning for clinical diagnostics. Vocations and Learning, 13(1), 159–177. https://doi.org/10.1007/s12186-019-09234-z
- Zwart, D. P., Noroozi, O., Van Luit, J. E. H., Goei, S. L., & Nieuwenhuis, A. (2020). Effects of digital learning materials on nursing students’ mathematics learning, self-efficacy, and task value in vocational education. Nurse Education in Practice, 44, 102755. https://doi.org/10.1016/j.nepr.2020.102755
- Zwart, D. P., Van Luit, J. E. H., Noroozi, O., & Goei, S. L. (2017). The effects of digital learning material on students’ mathematics learning in vocational education. Cogent Education, 4(1), 1313581. doi:10.1080/2331186X.2017.1313581