ABSTRACT
Virtual reality (VR) has been shown to induce excessive affective processing, which in turn impacts the learning process and learning outcomes. Therefore, a better understanding of emotional dynamics and how emotions are instigated while learning with VR is needed. For this purpose, the students learning process with a VR simulation was captured using continuous measurement of facial expressions. In addition, personality traits and self-regulated emotion (SRE) characteristics were examined. The findings suggest that overall, joy was the most predominant emotion expressed by students, while intraindividual components of personality traits and SRE strategies were associated with negative emotional expressions. Specifically, students with high conscientiousness or agreeableness personality traits tended to experience less anger and/or sadness. In addition, the findings demonstrate that the experience of these negative emotions was mediated by the SRE strategies of reappraisal and suppression, implying a mechanism of emotional engagement while learning with VR. This suggests that while individual personality traits are relatively stable and immutable, SRE preferences can be supported as part of the VR instructional design and scaffolding. Future studies should explore how students’ emotion regulation preferences can be supported to benefit their emotional experiences while learning.
Introduction
Virtual reality (VR) is a computer-generated environment to model and simulate three-dimensional (3D) visual experiences and interactions. While learning with VR students can maneuver and manipulate the 3D representations of a physical location or of an occurrence, which facilitates the illusion that this virtual place truly exists (Di Natale et al., Citation2020; Ghanbarzadeh et al., Citation2014). VR-based simulations create a psychological sensation and illusion of being within the artificially created world as if it was a real place (Radianti et al., Citation2020). This evokes the feeling of “being there” or a “sense of presence” (Witmer & Singer, Citation1998). As such, these unique immersive characteristics of learning with VR can increase learners’ affective arousal and boost their emotional engagement. To this end, the current study examines how and to what extent emotions are being instigated during learning with VR; and what role emotion self-regulation and individual personality components play in emotional dynamics.
Emotional engagement
Engagement is a multi-dimensional construct that integrates three main indicators that relate to behavioral, emotional, and cognitive characteristics of students’ learning (D'Mello et al., Citation2017; Fredricks et al., Citation2004; Sinatra et al., Citation2015; Skinner, Citation2016). This study focuses only on the emotional dimension of engagement, which mainly emphasizes students’ emotional states during learning (Christenson et al., Citation2012). Emotional involvement during learning can be positive, which is characterized by emotions such as enjoyment, interest, and pride (Renninger & Bachrach, Citation2015). Emotional states can also be negative, and students might experience emotions such as boredom, anger, and anxiety (Skinner, Citation2016; Skinner et al., Citation2008). Theories of motivation, including the self-determination theory, and the control-value theory (CVT) of achievement emotions (Deci & Ryan, Citation1985; Pekrun & Linnenbrink-Garcia, Citation2012) underline the role that both positive and negative emotions have on students’ involvement in learning activities and underscore how affective dynamics sustain or disrupt learners’ engagement to impact learning performance (D’Mello & Graesser, Citation2012; Gupta et al., Citation2018; Pekrun & Perry, Citation2014).
Emotional engagement with VR is an important factor to consider when investigating learning processes with VR. This is important because VR fosters a higher level of a sense of presence than standard media (Makowski et al., Citation2017), and as some studies suggest, is also able to elicit different emotional experiences (Awada et al., Citation2021; Felnhofer et al., Citation2015). For example, studies in psychological therapy show that VR can activate different degrees of fear and thus is being used to treat phobias (Freitas et al., Citation2021), such as spider phobia (Miloff et al., Citation2019), claustrophobia (Hudson et al., Citation2021), social anxiety (Horigome et al., Citation2020), and aerophobia (Cardoş et al., Citation2017). Studies have also demonstrated that VR can increase positive affect and decrease negative affect, and as a result has been used in affective computing to improve mental health and emotional well-being (Prabhu et al., Citation2019; Sun et al., Citation2022). While the research in the psychology and therapy domains on emotional experiences in VR is growing, to date only a handful of studies in education have examined emotions instigated during learning with VR. The limited research on emotional engagement while learning with VR suggests that VR induces intense basic emotions, such as joy and sadness (Makransky & Lilleholt, Citation2018; Parong & Mayer, Citation2021), as well as complex emotions such as awe and gratitude (Collange & Guegan, Citation2020). A recent study by Dubovi (Citation2022) demonstrated that learning with VR induced predominantly more joy than other basic emotions, however, only the negative emotion of anger was significantly associated with learning achievements. Nevertheless, while more research is needed on learning with VR and the involved emotional experiences, a recent systematic review on emotions in virtual learning (Henritius et al., Citation2019), concluded that most extant studies rely on post-hoc data and operationalize emotions as traits rather than states, suggesting the need for continuous measurements.
Indeed, emotions are considered reactive by nature, as they occur in response to situations, actions, or events, therefore, during a learning episode, emotions inevitably fluctuate (Ahn & Harley, Citation2020; Pekrun, Citation2006). Facial expression analysis has been suggested as efficient in capturing learners’ fast-changing emotional state during learning with VR (Dubovi, Citation2022). In this way, varying sets of action unit activities can be matched by automated artificial neural networks to different discrete emotions in very short time intervals (Ekman, Citation1992; Ekman & Rosenberg, Citation1997). This approach can provide an objective, continuous and detailed view of students’ transitory emotions throughout a learning session (Dindar et al., Citation2020). Notably, since emotions fluctuate not only as a response to the academic flow of events but may also be triggered by intraindividual personality components and self-regulation tendencies, it is necessary to view emotional engagement through these lenses as well.
Emotion regulation and personality traits
Self-regulated emotion (SRE) refers to the processes of reducing or increasing the intensity or duration of positive and negative emotions during learning to reach optimal levels of functioning (Gross & John, Citation2003). SRE is part of self-regulated learning processes (SRL), which over the past decades are being conceptualized as multi-dimensional constructs which include not only cognitive and metacognitive components but also emotional aspects of learning (Schunk & Greene, Citation2017; Zheng et al., Citation2021; Zimmerman, Citation2013). Moreover, SRE has been demonstrated as an independent SRL construct that should be investigated and explicitly taught in addition to cognitive and behavioral self-regulated strategies (Ben-Eliyahu, Citation2019; Ben-Eliyahu & Linnenbrink-Garcia, Citation2015; Zheng et al., Citation2021). The current study focuses on reappraisal and suppression SRE strategies in learning (Gross, Citation1998).
Reappraisal refers to the reframing of one's perception of a situation or the modification of its emotional significance, by altering the thoughts about the situation or about the capacity to fulfill the demands of the situation (Gross, Citation1998; Gross & John, Citation2003). Reappraisal is antecedent-focused, meaning it is often used prior to experiencing the emotion to decrease negative emotions. For example, reminding oneself that even though preparation for an exam might be arduous, it can lead to better performance on the exam. Reappraisal has been shown to be positively related with higher positive emotions and adaptive outcomes such as higher learning outcomes (Leroy et al., Citation2012; Stiller et al., Citation2019; Strain & D'Mello, Citation2015), and negatively related to negative emotions and maladaptive outcomes (Gross, Citation2015).
Research findings suggest that reappraisal is an adaptive form of emotion regulation which is more effective than suppression at decreasing the experience of emotion (Troy et al., Citation2013). Suppression is response-focused, occurs after the emotional tendencies are triggered, and involves the process of inhibition of ongoing negative or positive emotion-expressive behavior (Gross & John, Citation2003). Suppression has substantial psychological and cognitive costs, such as reliable decrements in both objective memory and memory confidence ratings (Gross, Citation2002). Furthermore, suppression decreases emotion expression but not negative emotional experience and as a result, was found positively related to negative emotions and to impaired memory (Gross, Citation2015; Johns et al., Citation2008).
Learners employ an emotion regulation strategy depending on situational context-specific factors, emotion regulation goals, and self-efficacy (Gross, Citation2015). Ben-Eliyahu and Linnenbrink-Garcia (Citation2013) found that self-regulated emotion strategies are differentially employed based on course preferences (favorite and least favorite courses). Additional studies have also suggested that personality traits of the Five-Factor Model (FFM) are linked with emotion regulation strategies (Barańczuk, Citation2019; Digman, Citation1990). FFM is the universal most used taxonomy of personality traits in psychology (Extraversion, Agreeableness, Conscientiousness, Neuroticism and Openness to Experience) (Barańczuk, Citation2019; McCrae, Citation2020). Berkovich and Eyal (Citation2021) found that a teacher’s personality affects their emotion regulation strategies, pointing toward conscientiousness as a distinct personality trait that differentiates between various used emotion regulation strategies (Berkovich & Eyal, Citation2021). Extraversion and neuroticism are recognized as the three personality traits most relevant to emotion (see Watson et al., Citation1988). Extraverts are characterized as being outgoing, sociable, and enthusiastic, and thus are more prone to positive and happy emotional states (Lucas & Fujita, Citation2000; Reisenzein et al., Citation2020). In contrast, neurotic individuals experience more negative states such as anxiety and anger (De Raad, Citation1996; Watson et al., Citation1988). While a large body of research has examined the relation between personality traits and academic performance, there has been a lack of research on the impact of personality on emotional engagement while learning. Since the mechanism shaping the academic learning outcomes is proposed to be self-regulation (Abe, Citation2020; Komarraju et al., Citation2009; Poropat, Citation2009; Sorić et al., Citation2017; Vedel, Citation2014), there remains much to be learned about the role of SRE, personality traits, and emotional engagement. To the author's best knowledge, this study is the first to examine the association of personality traits, SRE, and emotions within the VR learning context.
Research questions
What emotions are instigated, and to what extent and in which sequence, during a learning process with a VR simulation as depicted by facial expression analysis?
How is emotional engagement while learning with VR impacted by SRE and individual personality traits?
Methods
Participants
Overall, 65 nursing students participated in the study. The final sample size was 61 since four participants were excluded from the data analysis due to facial expression calibration errors. The majority of the participants were female (n = 44) and the mean age of the research sample was 23 (±5.1) years.
The university’s ethics committee approved the study (#0001776-2).
Research design and procedure
This study is part of a more extensive study. Participants’ demographics, personality traits, and SRE strategies were assessed via a questionnaire. Following calibrations required to verify that facial expressions were recorded properly, the learning session with a VR-based simulation was initiated and lasted for a mean time of 41.18 (±13.7) minutes. Facial expressions were recorded and real-time analyzed during the entire learning experience with VR. The data collection was supported by the iMotions 9.0 Biometric Research Platform (https://imotions.com).
The VR-based simulation
The VR simulation was designed using the Unity platform (https://unity.com). VR architecture is based on our previous study (Dubovi et al., Citation2017). The simulation provides a three-dimensional (3D) simulated world where individuals are self-represented as avatars. VR is a responsive platform in which participants can maneuver the 3D representations of a physical location or of a phenomena, which in turn supports the sensation of being immersed in a real world. There is no single type of VR, rather VR environments can be classified along the immersive continuum they offer. The technological attributes of VR differentiate between low-immersive VR which is often called desktop VR, and high-immersive VR. The desktop low-immersive VR takes the form of a window into a virtual world displayed on a computer monitor, with interactions via a mouse, keyboard, or joystick (Choi et al., Citation2016). During the learning process with the low-immersive desktop VR, participants are fully aware of the real physical world and therefore it is considered a safe environment in terms of having a low risk for cybersickness and immersion injury (Martirosov et al., Citation2022). High-immersive VR involves a high degree of interactivity and high-cost peripheral devices. Such devices comprise a head-mounted display in which a high graphical fidelity screen is mounted in front of one’s eyes with separate lenses for each eye and sound delivered through earphones. By blocking out many visual elements of the real-world environment and inducing sensory stimuli that correspond with the virtual environment, high-immersive VR enables the user to more fully immerse in the virtual environment. We chose for the current study the low-immersive VR due to recent studies that show that more immersive technology is related to lower levels of learning, suggesting that high-immersive VR incorporates more presence but less learning (Jensen & Konradsen, Citation2018; Makransky, Terkildsen, et al., Citation2019; Ning Woon et al., Citation2020; Parong & Mayer, Citation2021). The possible reason for this outcome has been explained by Markransky and colleagues (Citation2019), who demonstrated through EEG that high-immersive VR is associated with a triggering of high cognitive load which might prohibit knowledge acquisition, as compared to desktop low-immersive VR.
The simulation was designed to model a hospital ward with two main spaces (). The first space represented the patient room and the second space represented the medicine room with various medical equipment and medications. Clinical scenarios were designed to support procedure training and development of clinical reasoning. Deliberations regarding practice were facilitated by providing students with opportunities to assess the virtual patient for his medical condition, interpret the physician’s instructions, calculate appropriate dosages, administer medication, and monitor the medication’s side-effects. During these steps, the VR technology provided individual feedback on the student’s performance together with video directions.
Data collection instruments
Facial expression recognition. Affectiva Affdex algorithm, which was provided by the iMotions 9.0 (https://imotions.com) platform, allowed this study to collect and extract the 7 basic emotions of joy, anger, surprise, contempt, fear, sadness, and disgust (Ekman & Rosenberg, Citation1997; Kulke et al., Citation2020). The seven emotional dimensions can also be categorized by their valence (positive or negative) or by the absence of valence, i.e. neutral. Facial expressions analysis was at a 30 Hz frequency and was collected for the overall learning experience (). The algorithm uses the Facial Action Coding System, which identifies “action units” and then classifies them to provide the likelihood that each of the 7 emotions will occur. Next, thresholding for time and absolute amplitude probability was carried out, based on the iMotions guidelines (https://imotions.com/blog/facial-expression-analysis/), to extract emotion percentage metrics.
Figure 2. A screenshot of the real-time facial expression data collection while a student is learning with the VR based simulation.
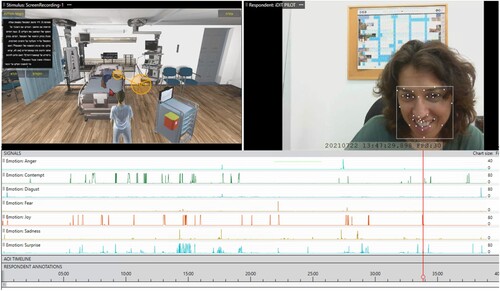
The Big Five Inventory (BFI). The BFI is a widely used measure of the Five-Factor Model (FFM) and yields measures of five dimensions of personality (John et al., Citation1991; John & Srivastava, Citation1999). Five personality traits: Conscientiousness (e.g. “I tend to be reliable and perform my work”); Agreeableness (e.g. “I tend to help others”); Neuroticism (e.g. “I tend to worry”); Extraversion (e.g. “I am an enthusiastic person”); and Openness (e.g. “I tend to have active imagination”). Participants had to indicate how much each phrase item pertains to them on a five-point Likert-type scale. The mean rating of all 44 items was calculated for each personality trait. The Cronbach alphas of the variables in this study were: Conscientiousness, α = .82; Agreeableness, α = .70; Neuroticism, α = .73; Extraversion, α = .79; and Openness, α = .75.
Emotion Regulation Questionnaire (ERQ). An adapted version of the ERQ was used to assess the students’ reappraisal and suppression SRE processes (Ben-Eliyahu & Linnenbrink-Garcia, Citation2013; Gross & John, Citation2003). Five items tapped into the ability to reframe the situation in order to adjust emotions (i.e. reappraisal; e.g. “I control my emotions by changing the way I think about the situation I’m in”); and four items reported on the inhibition of emotional expression strategy (i.e. suppression; e.g. “I control my emotions by not expressing them”). Items were rated on a seven-point Likert scale ranging from 1 (strongly disagree) to 7 (strongly agree). The reliabilities were within an acceptable range, Reappraisal (α = .79) and Suppression (α = .81).
Data analysis
The data analysis is presented with respect to the two research questions.
Analysis regarding research question 1 concerning the emotions elicited during learning with VR:
A Kruskal–Wallis H test was performed to account for the descriptive statistics of the seven emotions as depicted by facial expression analysis. This test was performed due to the data’s nonnormal distribution. This was followed by a one-way repeated measures ANOVA to follow each emotion's fluctuations during the VR time phases.
Analysis regarding research question 2 concerning the impact of SRE and individual personality traits on emotions while learning with VR:
A bivariate parametric correlation analysis was used to evaluate associations between the participants’ personality traits, self-regulation, and emotional engagement in terms of facial expressions. Next, based on the results of the correlation analyses, only variables which were found to be significantly associated were selected for inclusion in the subsequent two models of hierarchical regression analyses. For a similar approach to regression see (Duffy et al., Citation2018; Greene et al., Citation2014). In Model 1 (anger), personality traits (entered first) and SRE strategies (entered second) were included to determine whether these combinations of variables significantly explain anger. In the second model (sadness), personality traits (entered first) and SRE strategies (entered second) were selected to determine whether these combinations of variables significantly explain sadness.
For the mediation analyses, based on the hierarchical multiple regression analysis results, a PROCESS (model 4) was used to assess whether reappraisal strategy mediates the association between conscientiousness, agreeableness, and engagement; and evaluate whether suppression strategy mediates the association between agreeableness and sadness. To evaluate the significance of indirect effects, we used a bootstrap estimation approach (Hayes, Montoya, & Rockwood, Citation2017).
The data analysis was conducted using SPSS version 27.0 software.
Results
Facial expression analysis was used to collect and extract students’ emotional engagement while learning with VR (). The Kruskal–Wallis H test of facial expressions revealed that participants experienced significantly (χ2(6) = 314.710, p < .001) more joy 4.0±3.6% than other emotions such as surprise 1.7±2.6%, fear 0.3±0.9%, contempt 1.5±2.5%, disgust 0.3±0.6%, sadness 0.4±1.2%, and anger 0.8±1.6% (, ). A one-way repeated measures ANOVA analysis was conducted to examine the fluctuation of facial expressions throughout the VR learning process which was divided into three stages during the average time spent with VR (41.18 ± 13.7 min): initial stage (first 0–12 min), mid-stage (13–27 min), and final stage (28–42 min). This division is based on previous research by Tonguç and Ozkara (Citation2020), who suggested splitting facial expression changes across three stages of the learning sequence. The results revealed a significant interaction between the type of emotions derived from the facial expressions analysis and the VR simulation timeline stage (F (2, 580) = 15.214, p < 0.001,). Post-hoc analysis indicated significantly more joyful expressions than other emotions in the initial stage of the VR simulation (p < 0.001; ).
Figure 3. The mean change in students’ emotions as measured by facial expression analysis (time percentage) according to the VR simulation stages (minutes).
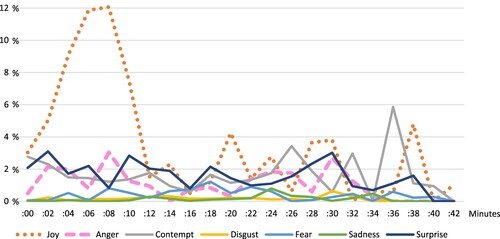
Table 1. Bivariate intercorrelations between personality traits, SRE strategies and emotional engagement as measured by facial expressions.
Personality traits and SRE strategies were assessed by self-reported questionnaires. Results demonstrated that the highest scored personal traits were conscientiousness and agreeableness (; F (4, 240) = 81.356, p < 0.001), and the most used SRE strategy was reappraisal (; t = 10.803, p < 0.001). To account for the impact of personality traits on emotional engagement, a bivariate intercorrelations analysis was performed (). Results revealed that when it comes to emotional engagement, conscientiousness, agreeableness, and openness, these personality traits were negatively associated with anger (r = −0.290 p<0.05, r = −0.276 p<0.05, r = −0.303, p<0.05; respectively); and agreeableness was negatively associated with sadness (r = −0.328 p<0.05). The analysis also showed that SRE impacts emotional engagement. Particularly, there was a significant negative correlation between reappraisal and anger (r = −0.301, p < 0.05), namely, the use of a reappraisal strategy was associated with fewer anger expressions. In contrast, a suppression strategy was correlated with more sadness expressions (r = 0.287, p < 0.05).
In the context of personality traits and emotion regulation processes, as shows, conscientiousness, agreeableness and extraversion were positively correlated with reappraisal (r = 0.368 p<0.01, r = 0.330 p<0.01, r = 0.275, p<0.05; respectively), and negatively with neuroticism (r = −0.459, p<0.001). By contrast, conscientiousness, agreeableness and extraversion personality traits were negatively associated with a suppression strategy of emotion regulation (r = −0.322 p <0.05, r = −0.364 p<0.01, r = −0.452 p<0.001; respectively).
Building upon the bivariate intercorrelation analysis findings (), only the significant intercorrelations were selected for inclusion in the two models of multivariant hierarchical regression analyses. This was to explain emotional engagement in terms of experienced anger and sadness. The hierarchical regression analysis for anger revealed that the inclusion of personality traits of conscientiousness and agreeableness accounted for 10% of the variance, F (2, 58) = 3.397, p < .05 (). Adding the SRE strategy showed that reappraisal explained an additional 15% of the variance (the entire model explained 25%), F(2, 56) = 4.881, p < 0.05 (). Hierarchical regression analysis for sadness revealed that agreeableness accounted for 9% of the variance, F (2, 58) = 3.198, p < .05 (). Adding the SRE strategy of suppression explained an additional 13% of the variance (the entire model explained 22%), F(2, 56) = 2.295, p < 0.5 ().
Table 2. Summary of two multivariate hierarchical regression analyses to explain emotional engagement in terms of anger and sadness (N = 61).
The hierarchical multiple regression analysis proposed two mediation effects: the first is the indirect effect of reappraisal on the association of conscientiousness and agreeableness with anger; and the second is the indirect effect of suppression on the association of agreeableness with sadness. Based on the above results, we used Baron and Kenny’s method (Baron & Kenny, Citation1986) to test the simultaneous direct and indirect effects for each of the two models using the PROCESS (model 4) with the bootstrap estimation approach with 5000 samples (Hayes, Citation2012, Citation2017). The first model is shown in . The results indicated that the indirect coefficients were significant for reappraisal with both independent variables of conscientiousness (b = −0.1007, 95% CI = −0.2205 to −0.0175; ) and agreeableness (b = −.0819, 95% CI = −0.1800 to −0.0030; ). The second model is shown in . Notably, also the association of agreeableness with sadness was fully mediated by the indirect effect of suppression strategy (b = −.0895, 95% CI = −0.1983 to −0.0101; ).
Discussion
The main goal of this study was to examine how emotions are being instigated during a learning process with VR, and are being shaped by intraindividual components of personality tendencies and SRE strategies. The study’s main findings point out that personality does matter when it comes to emotional engagement, and that SRE strategies of reappraisal and suppression play an important mediation role in experiencing less negative emotional engagement. Thus, this study advances our understanding of how personality shapes emotional engagement while learning with VR by the use of emotional SRE strategies.
Overall, the granular, continuous data source of emotional engagement using automatic recognition of students’ facial expressions revealed that joy (i.e. enjoyment or happiness) was the most predominant emotion that students expressed. This research finding is in line with recent studies that showed that joy expressions were triggered with higher intensity by technology-based educational tools, such as games and robot tutors, than by instructional traditional approaches such as lecture (Ninaus et al., Citation2019; Tonguç & Ozkara, Citation2020; Velentza et al., Citation2021). In addition, the current study results showed that the frequency of the joy expression changed across the different VR learning stages. Particularly, the joy expression was more intense during the first 0–12 min of the VR learning session compared to later stages. This rapid and sharp increase in the joy expressions at the beginning of a learning interaction with VR can be explained by the novelty of the VR medium (Jeno et al., Citation2019). Despite the observation that facial expressions of joy were the most frequent emotion expressed by students, there were no significant associations between joy and the FMM taxonomy-based personality tendencies nor the SRE strategies.
Factors that influence emotional engagement with VR
The facial expressions of anger and sadness, which were expressed at a relatively low frequency with no significant changes across the learning session, were significantly associated with personality dispositions and emotion regulation strategies. These findings suggest that students with a high conscientiousness personality trait tended to experience less anger and participants with a high agreeableness personality trait tended to experience less anger and sadness. Importantly, two mediation effects emerged in the analysis which proposes that the mechanism that shapes the experience of negative emotional engagement of conscientiousness and agreeableness learners were the SRE strategies. Findings demonstrate that learners with high scoring characteristics of conscientiousness and agreeableness seemed to employ significantly more of the adaptive SRE strategy of reappraisal, which in turn resulted in experiencing less anger. In addition, learners with high scoring agreeableness characterized by the traits of compliance and tendermindedness also tended to use less of the maladaptive SRE strategy of suppression, which in turn resulted in less sadness.
These results expand the knowledge on what is known about emotional engagement within the learning context. Until now, this understanding was predominately considered as a by-product of achievement motivations across different types of learning contexts and course preferences (Ben-Eliyahu, Citation2019; Ben-Eliyahu & Linnenbrink-Garcia, Citation2013; D’Mello & Graesser, Citation2012; Pekrun, Citation2006; Pintrich, Citation2004). More recently there has been a focus on targeting emotional engagement as an interdependent outcome of personality and interpersonal emotion regulation strategies (Ben-Eliyahu & Zeidner, Citation2020). While individual personality traits are relatively stable and immutable (Bleidorn et al., Citation2021; Stieger et al., Citation2021), previous studies have demonstrated that SRE strategies can be enhanced by counselling and training (Cohen & Mor, Citation2018; Hurtado-Parrado et al., Citation2019; Kim et al., Citation2009; Volkaert et al., Citation2020). Thus, the current study’s findings suggest that the magnitude of negative emotional experiences can be altered by impacting emotion regulation preferences. Since the CVT theory categorizes negative emotions as experiences which are expected to hinder learning (Camacho-Morles et al., Citation2021; Pekrun & Perry, Citation2014), the current study suggests that SRE strategies should be explicitly taught in schools to combat such negative experiences. Future research should investigate how students’ propensity to use the adaptive SRE strategy of reappraisal can be supported and how this can impact students’ tendency to experience negative emotions while learning.
The intraindividual components of personality and the SRE link
The students reported using more reappraisal strategies than suppression strategies, and this was associated with their personality traits. Notably, students who had higher conscientiousness, agreeableness, and extraversion tended to use more reappraisal strategies and less of the maladaptive suppression strategies. These findings are consistent with the Barańczuk (Citation2019) metanalysis which showed that the characteristics of conscientiousness such as being disciplined, diligent, and striving for goals are positively linked to the adaptive reappraisal strategy and negatively to the suppression strategy (Connor-Smith & Flachsbart, Citation2007). Furthermore, the association of higher agreeableness with a higher use of the reappraisal strategy and a lower maladaptive use of the suppression strategy was demonstrated and theoretically explained previously (John & Srivastava, Citation1999; Reisenzein et al., Citation2020). Barańczuk (Citation2019) proposed that the link to greater compliance, empathy, and altruism that agreeable individuals are characterized by, forms a more supportive emotion socialization process that might explain the relationship between agreeableness and emotion regulation strategies.
The findings showed that being more extravert was related to a greater use of adaptive SRE strategies. Previous studies have shown that extroverted individuals are more enthusiastic, sociable and problem solving, which in turn increases the possibility of greater typically adaptive and lower typically maladaptive emotion regulation strategies (Barańczuk, Citation2019; Connor-Smith & Flachsbart, Citation2007). Moreover, in contrast to conscientiousness, agreeableness and extraversion, neuroticism was found to be negatively related to reappraisal use. Considering the avoidance, low emotional stability and behavioral inhibition characteristics of neuroticism, it might be supposed that students who declare greater neuroticism may use more strategies that cut them off from the negative experiences through using avoidance rather than adaptive strategies (Barańczuk, Citation2019; De Raad, Citation1996).
This study’s finding of an association between personality traits and the use of SRE strategies therefore reveals that interpersonal characteristics have a pivotal role in students’ emotional engagement while learning.
Limitations and future research
There are some limitations of the current study. Previous research has suggested that negative facial expressions are quicker to appear and thus facial expressions are more sensitive to detecting negative emotional valence (van Bommel et al., Citation2020; Zeinstra et al., Citation2009). Thus, further studies should also incorporate other emotional engagement measurements to illuminate the role of positive emotions. Moreover, the current analysis focused on the aggregate facial expressions view, further studies should also incorporate the individual perspective of facial expressions coding (Liaw et al., Citation2021; Sawyer et al., Citation2017). For example, it is important to understand what exactly, from the individual perspective, triggered a facial expression of anger when interacting with VR, using the bottom-up approach. It is also important to unfold how emotions are manifested during specific events in the simulation, for example when negative feedback is received due to drug dosage miscalculation. Finally, it is important to note that in the context of CVT theory, there is an ongoing debate regarding the effect of activating the negative emotion of anger on learning outcomes (Ahn & Harley, Citation2020; Pekrun et al., Citation2009). Therefore, the effect of anger as a precursor of deeper cognitive processing should be further studied.
Conclusion
Using facial expression analysis to detect students’ emotional dynamics moment-by-moment while learning with VR demonstrated that overall, joy was the most predominant emotion expressed by students. However, in the case of the tendency to negative emotions, this study shows that intraindividual components of personality traits and SRE strategies might color the entire gamut of student emotional engagement. While personality traits are relatively stable and immutable, SRE strategies can be altered and supported.
A body of recent psychology research shows that VR can be used for the development of adaptive SRE strategies to modify the emotional response (Colombo et al., Citation2021; Montana et al., Citation2020). We therefore suggest that further research should evaluate the utilization of explicit SRE strategy prompts to benefit students’ emotional experiences while learning with VR (Price et al., Citation2018). Moreover, multimodal software such as for unintrusive facial expression analysis can be used to analyze in real-time emotional experiences to provide personalized automated SRE strategy prompts and to evaluate their impact (Wiedbusch et al., Citation2021). Since the research in this area is in its infancy, it is important to further develop our understanding of how to design explicit prompts to help learners be more capable of regulating their affective states and increasing learning outcomes.
Acknowledgements
The author would like to thank engineer Sergey Bondarchuck with whom the VR learning environment was designed and programmed, Liat Liberman for assisting with data collection.
Disclosure statement
No potential conflict of interest was reported by the author(s).
Additional information
Funding
Notes on contributors
Ilana Dubovi
Ilana Dubovi is a learning science researcher at the Nursing Department at Tel Aviv University. Her work spans the fields of educational technology, lifelong learning, cognition and emotion in learning processes, and learning analytics.
References
- Abe, J. A. A. (2020). Big five, linguistic styles, and successful online learning. The Internet and Higher Education, 45, 100724. https://doi.org/10.1016/j.iheduc.2019.100724
- Ahn, B. T., & Harley, J. M. (2020). Facial expressions when learning with a queer history app: Application of the control value theory of achievement emotions. British Journal of Educational Technology, 51(5), 1563–1576. https://doi.org/10.1111/bjet.12989
- Awada, M., Zhu, R., Becerik-Gerber, B., Lucas, G., & Southers, E. (2021). An integrated emotional and physiological assessment for VR-based active shooter incident experiments. Advanced Engineering Informatics, 47, 101227. https://doi.org/10.1016/j.aei.2020.101227
- Barańczuk, U. (2019). The five factor model of personality and emotion regulation: A meta-analysis. Personality and Individual Differences, 139, 217–227. https://doi.org/10.1016/j.paid.2018.11.025
- Baron, R. M., & Kenny, D. A. (1986). The moderator–mediator variable distinction in social psychological research: Conceptual, strategic, and statistical considerations.. Journal of Personality and Social Psychology, 51(6), 1173–1182. https://doi.org/10.1037/0022-3514.51.6.1173
- Ben-Eliyahu, A. (2019). Academic emotional learning: A critical component of self-regulated learning in the emotional learning cycle. Educational Psychologist, 54(2), 84–105. https://doi.org/10.1080/00461520.2019.1582345
- Ben-Eliyahu, A., & Linnenbrink-Garcia, L. (2013). Extending self-regulated learning to include self-regulated emotion strategies. Motivation and Emotion, 37(3), 558–573. https://doi.org/10.1007/s11031-012-9332-3
- Ben-Eliyahu, A., & Linnenbrink-Garcia, L. (2015). Integrating the regulation of affect, behavior, and cognition into self-regulated learning paradigms among secondary and post-secondary students. Metacognition and Learning, 10(1), 15–42. https://doi.org/10.1007/s11409-014-9129-8
- Ben-Eliyahu, A., & Zeidner, M. (2020). Personality in educational psychology. In P. J. Corr, & G. Matthews (Eds.), The Cambridge handbook of personality psychology (pp. 439–450). Cambridge University Press.
- Berkovich, I., & Eyal, O. (2021). Teachers’ big five personality traits, emotion regulation patterns, and moods: Mediation and prototype analyses. Research Papers in Education, 36(3), 332–354. https://doi.org/10.1080/02671522.2019.1677758
- Bleidorn, W., Hopwood, C. J., Back, M. D., Denissen, J. J., Hennecke, M., Hill, P. L., Jokela, M., Kandler, C., Lucas, R. E., & Luhmann, M. (2021). Personality trait stability and change. Personality Science, 2, 1–20. https://doi.org/10.5964/ps.6009
- Camacho-Morles, J., Slemp, G. R., Pekrun, R., Loderer, K., Hou, H., & Oades, L. G. (2021). Activity achievement emotions and academic performance: A meta-analysis. Springer.
- Cardoş, R. A., David, O. A., & David, D. O. (2017). Virtual reality exposure therapy in flight anxiety: A quantitative meta-analysis. Computers in Human Behavior, 72, 371–380. https://doi.org/10.1016/j.chb.2017.03.007
- Choi, D., Dailey-Hebert, A., Estes, S. (2016). Emerging tools and applications of virtual reality in education. IGI Global.
- Christenson, S. L., Reschly, A. L., & Wylie, C. (2012). Handbook of research on student engagement. Springer.
- Cohen, N., & Mor, N. (2018). Enhancing reappraisal by linking cognitive control and emotion. Clinical Psychological Science, 6(1), 155–163. https://doi.org/10.1177/2167702617731379
- Collange, J., & Guegan, J. (2020). Using virtual reality to induce gratitude through virtual social interaction. Computers in Human Behavior, 113, 106473. https://doi.org/10.1016/j.chb.2020.106473
- Colombo, D., Díaz-García, A., Fernandez-Álvarez, J., & Botella, C. (2021). Virtual reality for the enhancement of emotion regulation. Clinical Psychology & Psychotherapy, 28(3), 519–537. https://doi.org/10.1002/cpp.2618
- Connor-Smith, J. K., & Flachsbart, C. (2007). Relations between personality and coping: A meta-analysis. Journal of Personality and Social Psychology, 93(6), 1080. https://doi.org/10.1037/0022-3514.93.6.1080
- Deci, E. L., & Ryan, R. M. (1985). Intrinsic motivation and self-determination in human behavior. Plenum.
- De Raad, B. (1996). Personality traits in learning and education. European Journal of Personality, 10(3), 185–200. https://doi.org/10.1002/(SICI)1099-0984(199609)10:3<185::AID-PER256>3.0.CO;2-M
- Digman, J. M. (1990). Personality structure: Emergence of the five-factor model. Annual Review of Psychology, 41(1), 417–440. https://doi.org/10.1146/annurev.ps.41.020190.002221
- Di Natale, A. F., Repetto, C., Riva, G., & Villani, D. (2020). Immersive virtual reality in K-12 and higher education: A 10-year systematic review of empirical research. British Journal of Educational Technology, 51(6), 2006–2033. https://doi.org/10.1111/bjet.13030
- Dindar, M., Jarvela, S., Ahola, S., Huang, X., & Zhao, G. (2020). Leaders and followers identified by emotional mimicry during collaborative learning: A facial expression recognition study on emotional valence. IEEE Transactions on Affective Computing, 13(3), 1390–1400.
- D'Mello, S., Dieterle, E., & Duckworth, A. (2017). Advanced, analytic, automated (AAA) measurement of engagement during learning. Educational Psychologist, 52(2), 104–123. https://doi.org/10.1080/00461520.2017.1281747
- D’Mello, S., & Graesser, A. (2012). Dynamics of affective states during complex learning. Learning and Instruction, 22(2), 145–157. https://doi.org/10.1016/j.learninstruc.2011.10.001
- Dubovi, I. (2022). Cognitive and emotional engagement while learning with VR: The perspective of multimodal methodology. Computers & Education, 183, 104495. https://doi.org/10.1016/j.compedu.2022.104495
- Dubovi, I., Levy, S. T., & Dagan, E. (2017). Now i know how! The learning process of medication administration among nursing students with non-immersive desktop virtual reality simulation. Computers and Education, 113, 16–27.
- Duffy, M. C., Lajoie, S. P., Pekrun, R., & Lachapelle, K. (2018). Emotions in medical education: Examining the validity of the medical emotion scale (MES) across authentic medical learning environments. Learning and Instruction, 70, 101150. https://doi.org/10.1016/j.learninstruc.2018.07.001
- Ekman, P. (1992). Are there basic emotions?
- Ekman, P., & Rosenberg, E. L. (1997). What the face reveals: Basic and applied studies of spontaneous expression using the facial action coding system (FACS). Oxford University Press.
- Felnhofer, A., Kothgassner, O. D., Schmidt, M., Heinzle, A.-K., Beutl, L., Hlavacs, H., & Kryspin-Exner, I. (2015). Is virtual reality emotionally arousing? Investigating five emotion inducing virtual park scenarios. International Journal of Human-Computer Studies, 82, 48–56. https://doi.org/10.1016/j.ijhcs.2015.05.004
- Fredricks, J. A., Blumenfeld, P. C., & Paris, A. H. (2004). School engagement: Potential of the concept, state of the evidence. Review of Educational Research, 74(1), 59–109. https://doi.org/10.3102/00346543074001059
- Freitas, J. R. S., Velosa, V. H. S., Abreu, L. T. N., Jardim, R. L., Santos, J. A. V., Peres, B., & Campos, P. F. (2021). Virtual reality exposure treatment in phobias: A systematic review. Psychiatric Quarterly, 92(4), 1685–1710. https://doi.org/10.1007/s11126-021-09935-6
- Ghanbarzadeh, R., Ghapanchi, A. H., Blumenstein, M., & Talaei-Khoei, A. (2014). A decade of research on the use of three-dimensional virtual worlds in health care: A systematic literature review. Journal of Medical Internet Research, 16(2), e47. https://doi.org/10.2196/jmir.3097
- Greene, J. A., Seung, B. Y., & Copeland, D. Z. (2014). Measuring critical components of digital literacy and their relationships with learning. Computers & Education, 76, 55–69. https://doi.org/10.1016/j.compedu.2014.03.008
- Gross, J. J. (1998). Antecedent- and response-focused emotion regulation: Divergent consequences for experience, expression, and physiology.. Journal of Personality and Social Psychology, 74(1), 224. https://doi.org/10.1037/0022-3514.74.1.224
- Gross, J. J. (2002). Emotion regulation: Affective, cognitive, and social consequences. Psychophysiology, 39(3), 281–291.
- Gross, J. J. (2015). Emotion regulation: Current status and future prospects. Psychological Inquiry, 26(1), 1–26. https://doi.org/10.1080/1047840X.2014.940781
- Gross, J. J., & John, O. P. (2003). Individual differences in two emotion regulation processes: Implications for affect, relationships, and well-being. Journal of Personality and Social Psychology, 85(2), 348. https://doi.org/10.1037/0022-3514.85.2.348
- Gupta, A., Elby, A., & Danielak, B. A. (2018). Exploring the entanglement of personal epistemologies and emotions in students’ thinking. Physical Review Physics Education Research, 14(1), 010129. https://doi.org/10.1103/PhysRevPhysEducRes.14.010129
- Hayes, A. F. (2012). PROCESS: A versatile computational tool for observed variable mediation, moderation, and conditional process modeling. University of Kansas.
- Hayes, A. F. (2017). Introduction to mediation, moderation, and conditional process analysis: A regression-based approach. Guilford Press.
- Hayes, M., Hayes, A. F., Montoya, A. K., & Rockwood, N. J. (2017). The analysis of mechanisms and their contingencies: PROCESS versus structural equation modeling. Australasian Marketing Journal, 25(1), 76–81.
- Henritius, E., Löfström, E., & Hannula, M. S. (2019). University students’ emotions in virtual learning: A review of empirical research in the 21st century. British Journal of Educational Technology, 50(1), 80–100. https://doi.org/10.1111/bjet.12699
- Horigome, T., Kurokawa, S., Sawada, K., Kudo, S., Shiga, K., Mimura, M., & Kishimoto, T. (2020). Virtual reality exposure therapy for social anxiety disorder: A systematic review and meta-analysis. Psychological Medicine, 50(15), 2487–2497. https://doi.org/10.1017/S0033291720003785
- Hudson, D., Heales, C., & Vine, S. (2021). Scoping review: How is virtual reality being used as a tool to support the experience of undergoing magnetic resonance imaging? Radiography, 28(1), 199–208. https://doi.org/10.1016/j.radi.2021.09.008
- Hurtado-Parrado, C., Sierra-Puentes, M., El Hazzouri, M., Morales, A., Gutiérrez-Villamarín, D., Velásquez, L., Correa-Chica, A., Rincón, J. C., Henao, K., Castañeda, J. G., & López-López, W. (2019). Emotion regulation and attitudes toward conflict in Colombia: Effects of reappraisal training on negative emotions and support for conciliatory and aggressive statements. Frontiers in Psychology, 10(908), https://doi.org/10.3389/fpsyg.2019.00908
- Jeno, L. M., Vandvik, V., Eliassen, S., & Grytnes, J.-A. (2019). Testing the novelty effect of an m-learning tool on internalization and achievement: A self-determination theory approach. Computers & Education, 128, 398–413. https://doi.org/10.1016/j.compedu.2018.10.008
- Jensen, L., & Konradsen, F. (2018). A review of the use of virtual reality head-mounted displays in education and training. Education and Information Technologies, 23, 1515–1529.
- John, O. P., Donahue, E. M., & Kentle, R. L. (1991). Big five inventory. Journal of Personality and Social Psychology. https://doi.org/10.1037/t07550-000
- John, O. P., & Srivastava, S. (1999). The big-five trait taxonomy: History, measurement, and theoretical perspectives. In L. A. Pervin, & O. P. John (Eds.), Handbook of personality: Theory and research (Vol. 2 ((2nd ed, pp. 102–138). Guilford Press.
- Johns, M., Inzlicht, M., & Schmader, T. (2008). Stereotype threat and executive resource depletion: Examining the influence of emotion regulation. Journal of Experimental Psychology: General, 137(4), 691. https://doi.org/10.1037/a0013834
- Kim, M. Y., Lee, J. Y., & Kim, J. (2009). Relationships among burnout, social support, and negative mood regulation expectancies of elementary school teachers in Korea. Asia Pacific Education Review, 10(4), 475–482. https://doi.org/10.1007/s12564-009-9045-9
- Komarraju, M., Karau, S. J., & Schmeck, R. R. (2009). Role of the big five personality traits in predicting college students’ academic motivation and achievement. Learning and Individual Differences, 19(1), 47–52. https://doi.org/10.1016/j.lindif.2008.07.001
- Kulke, L., Feyerabend, D., & Schacht, A. (2020). A comparison of the affectiva imotions facial expression analysis software with EMG for identifying facial expressions of emotion. Frontiers in Psychology, 11, 329. https://doi.org/10.3389/fpsyg.2020.00329
- Leroy, V., Grégoire, J., Magen, E., Gross, J. J., & Mikolajczak, M. (2012). Resisting the sirens of temptation while studying: Using reappraisal to increase focus, enthusiasm, and performance. Learning and Individual Differences, 22(2), 263–268. https://doi.org/10.1016/j.lindif.2011.10.003
- Liaw, H., Yu, Y.-R., Chou, C.-C., & Chiu, M.-H. (2021). Relationships between facial expressions, prior knowledge, and multiple representations: A case of conceptual change for kinematics instruction. Journal of Science Education and Technology, 30(2), 227–238. https://doi.org/10.1007/s10956-020-09863-3
- Lucas, R. E., & Fujita, F. (2000). Factors influencing the relation between extraversion and pleasant affect. Journal of Personality and Social Psychology, 79(6), 1039. https://doi.org/10.1037/0022-3514.79.6.1039
- Makowski, D., Sperduti, M., Nicolas, S., & Piolino, P. (2017). “Being there” and remembering it: Presence improves memory encoding. Consciousness and Cognition, 53, 194–202. https://doi.org/10.1016/j.concog.2017.06.015
- Makransky, G., & Lilleholt, L. (2018). A structural equation modeling investigation of the emotional value of immersive virtual reality in education. Educational Technology Research and Development, 66(5), 1141–1164. https://doi.org/10.1007/s11423-018-9581-2
- Makransky, G., Terkildsen, T. S., & Mayer, R. E. (2019). Adding immersive virtual reality to a science lab simulation causes more presence but less learning. Learning and Instruction, 60, 225–236.
- Martirosov, S, Bureš, M, & Zítka, T. (2022). Cyber sickness in low-immersive, semi-immersive, and fully immersive virtual reality. Virtual Reality, 26(1), 15–32.
- McCrae, R. R. (2020). The five-factor model of personality: Consensus and controversy. In G. Matthews, & P. J. Corr (Eds.), The Cambridge handbook of personality psychology ((2nd ed, pp. 129–141). Cambridge University Press.
- Miloff, A., Lindner, P., Dafgård, P., Deak, S., Garke, M., Hamilton, W., Heinsoo, J., Kristoffersson, G., Rafi, J., & Sindemark, K. (2019). Automated virtual reality exposure therapy for spider phobia vs. In-vivo one-session treatment: A randomized non-inferiority trial. Behaviour Research and Therapy, 118, 130–140. https://doi.org/10.1016/j.brat.2019.04.004
- Montana, J. I., Matamala-Gomez, M., Maisto, M., Mavrodiev, P. A., Cavalera, C. M., Diana, B., Mantovani, F., & Realdon, O. (2020). The benefits of emotion regulation interventions in virtual reality for the improvement of wellbeing in adults and older adults: A systematic review. Journal of Clinical Medicine, 9(2), 500. https://doi.org/10.3390/jcm9020500
- Ninaus, M., Greipl, S., Kiili, K., Lindstedt, A., Huber, S., Klein, E., Karnath, H.-O., & Moeller, K. (2019). Increased emotional engagement in game-based learning – A machine learning approach on facial emotion detection data. Computers & Education, 142, 103641. https://doi.org/10.1016/j.compedu.2019.103641
- Ning Woon, A. P., Mok, W. Q., Chieng, Y. J. S., Zhang, H. M., Ramos, P., & Mustadi, H. B. (2020). Effectiveness of virtual reality training in improving knowledge among nursing students: A systematic review, meta-analysis and meta-regression. Nurse Education Today.
- Parong, J., & Mayer, R. E. (2021). Cognitive and affective processes for learning science in immersive virtual reality. Journal of Computer Assisted Learning, 37(1), 226–241. https://doi.org/10.1111/jcal.12482
- Pekrun, R. (2006). The control-value theory of achievement emotions: Assumptions, corollaries, and implications for educational research and practice. Educational Psychology Review, 18(4), 315–341. https://doi.org/10.1007/s10648-006-9029-9
- Pekrun, R., Elliot, A. J., & Maier, M. A. (2009). Achievement goals and achievement emotions: Testing a model of their joint relations with academic performance. Journal of Educational Psychology, 101(1), 115. https://doi.org/10.1037/a0013383
- Pekrun, R., & Linnenbrink-Garcia, L. (2012). Academic emotions and student engagement. In A. R. S. Christenson, & C. Wylie (Springer ed., pp. 259-282). Springer US
- Pekrun, R., & Perry, R. P. (2014). Control-value theory of achievement emotions. In R. Pekrun & R. P. Perry (Eds.), International handbook of emotions in education (pp. 120–141). Routledge.
- Pintrich, P. R. (2004). A conceptual framework for assessing motivation and self-regulated learning in college students. Educational Psychology Review, 16(4), 385–407. https://doi.org/10.1007/s10648-004-0006-x
- Poropat, A. E. (2009). A meta-analysis of the five-factor model of personality and academic performance. Psychological Bulletin, 135(2), 322. https://doi.org/10.1037/a0014996
- Prabhu, V. G., Linder, C., Stanley, L. M., & Morgan, R. (2019). An affective computing in virtual reality environments for managing surgical pain and anxiety. In 2019 IEEE International Conference on Artificial Intelligence and Virtual Reality (AIVR).
- Price, M. J., Mudrick, N. V., Taub, M., & Azevedo, R. (2018). The role of negative emotions and emotion regulation on self-regulated learning with MetaTutor. In International Conference on Intelligent Tutoring Systems.
- Radianti, J., Majchrzak, T. A., Fromm, J., & Wohlgenannt, I. (2020). A systematic review of immersive virtual reality applications for higher education: Design elements, lessons learned, and research agenda. Computers & Education, 147, 103778. https://doi.org/10.1016/j.compedu.2019.103778
- Reisenzein, R., Hildebrandt, A., & Weber, H. (2020). Personality and emotion. In G. Matthews, & P. J. Corr (Eds.), The Cambridge handbook of personality psychology ((2nd ed, pp. 81–100). Cambridge University Press.
- Renninger, K. A., & Bachrach, J. E. (2015). Studying triggers for interest and engagement using observational methods. Educational Psychologist, 50(1), 58–69. https://doi.org/10.1080/00461520.2014.999920
- Sawyer, R., Smith, A., Rowe, J., Azevedo, R., & Lester, J. (2017). Enhancing student models in game-based learning with facial expression recognition. In Proceedings of the 25th Conference on User Modeling, Adaptation and Personalization.
- Schunk, D. H., & Greene, J. A. (2017). Historical, contemporary, and future perspectives on self-regulated learning and performance. In D. H. Schunk & J. A. Greene (Eds.), Handbook of self-regulation of learning and performance (pp. 1–15). Routledge.
- Sinatra, G. M., Heddy, B. C., & Lombardi, D. (2015). The challenges of defining and measuring student engagement in science (Vol. 50). Routledge.
- Skinner, E. (2016). Handbook of motivation at school. Routledge.
- Skinner, E., Furrer, C., Marchand, G., & Kindermann, T. (2008). Engagement and disaffection in the classroom: Part of a larger motivational dynamic? Journal of Educational Psychology, 100(4), 765–781. https://doi.org/10.1037/a0012840
- Sorić, I., Penezić, Z., & Burić, I. (2017). The big five personality traits, goal orientations, and academic achievement. Learning and Individual Differences, 54, 126–134. https://doi.org/10.1016/j.lindif.2017.01.024
- Stieger, M., Flückiger, C., Rüegger, D., Kowatsch, T., Roberts, B. W., & Allemand, M. (2021). Changing personality traits with the help of a digital personality change intervention. Proceedings of the National Academy of Sciences, 118(8). https://doi.org/10.1073/pnas.2017548118
- Stiller, A.-K., Kattner, M. F., Gunzenhauser, C., & Schmitz, B. (2019). The effect of positive reappraisal on the availability of self-control resources and self-regulated learning*. Educational Psychology, 39(1), 86–111. https://doi.org/10.1080/01443410.2018.1524851
- Strain, A. C., & D'Mello, S. K. (2015). Affect regulation during learning: The enhancing effect of cognitive reappraisal. Applied Cognitive Psychology, 29(1), 1–19. https://doi.org/10.1002/acp.3049
- Sun, Y., Li, F., He, T., Meng, Y., Yin, J., Yim, I. S., Xu, L., & Wu, J. (2022). Physiological and affective responses to green space virtual reality among pregnant women. Environmental Research, 216, 114499. https://doi.org/10.1016/j.envres.2022.114499
- Tonguç, G., & Ozkara, B. O. (2020). Automatic recognition of student emotions from facial expressions during a lecture. Computers & Education, 148, 103797. https://doi.org/10.1016/j.compedu.2019.103797
- Troy, A. S., Shallcross, A. J., & Mauss, I. B. (2013). A person-by-situation approach to emotion regulation. Psychological Science, 24(12), 2505–2514. https://doi.org/10.1177/0956797613496434
- van Bommel, R., Stieger, M., Visalli, M., de Wijk, R., & Jager, G. (2020). Does the face show what the mind tells? A comparison between dynamic emotions obtained from facial expressions and temporal dominance of emotions (TDE). Food Quality and Preference, 85, 103976. https://doi.org/10.1016/j.foodqual.2020.103976
- Vedel, A. (2014). The big five and tertiary academic performance: A systematic review and meta-analysis. Personality and Individual Differences, 71, 66–76. https://doi.org/10.1016/j.paid.2014.07.011
- Velentza, A.-M., Fachantidis, N., & Lefkos, I. (2021). Learn with surprize from a robot professor. Computers & Education, 173, 104272. https://doi.org/10.1016/j.compedu.2021.104272
- Volkaert, B., Wante, L., Van Beveren, M.-L., Vervoort, L., & Braet, C. (2020). Training adaptive emotion regulation skills in early adolescents: The effects of distraction, acceptance, cognitive reappraisal, and problem solving. Cognitive Therapy and Research, 44(3), 678–696. https://doi.org/10.1007/s10608-019-10073-4
- Watson, D., Clark, L. A., & Tellegen, A. (1988). Development and validation of brief measures of positive and negative affect: The PANAS scales. Journal of Personality and Social Psychology, 54(6), 1063–1070. https://doi.org/10.1037/0022-3514.54.6.1063
- Wiedbusch, M. D., Kite, V., Yang, X., Park, S., Chi, M., Taub, M., & Azevedo, R. (2021). A theoretical and evidence-based conceptual design of metadash: An intelligent teacher dashboard to support teachers’ decision making and students’ self-regulated learning. Frontiers in Education, 6. https://doi.org/10.3389/feduc.2021.570229
- Witmer, B. G., & Singer, M. J. (1998). Measuring presence in virtual environments: A presence questionnaire. Presence: Teleoperators and Virtual Environments, 7(3), 225–240. https://doi.org/10.1162/105474698565686
- Zeinstra, G. G., Koelen, M., Colindres, D., Kok, F., & De Graaf, C. (2009). Facial expressions in school-aged children are a good indicator of ‘dislikes’, but not of ‘likes’. Food Quality and Preference, 20(8), 620–624. https://doi.org/10.1016/j.foodqual.2009.07.002
- Zheng, J., Huang, L., Li, S., Lajoie, S. P., Chen, Y., & Hmelo-Silver, C. E. (2021). Self-regulation and emotion matter: A case study of instructor interactions with a learning analytics dashboard. Computers & Education, 161, 104061. https://doi.org/10.1016/j.compedu.2020.104061
- Zimmerman, B. J. (2013). From cognitive modeling to self-regulation: A social cognitive career path. Educational Psychologist, 48(3), 135–147. doi:10.1080/00461520.2013.794676