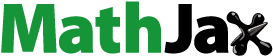
ABSTRACT
Objective: Existing evidence highlights the importance of modeling differential therapist effectiveness when studying psychotherapy outcome. However, no study to date examined whether this assertion applies to the study of within-patient effects in mechanisms of change. The study investigated whether therapist effects should be modeled when studying mechanisms of change on a within-patient level.
Methods: We conducted a Monte Carlo simulation study, varying patient- and therapist level sample sizes, degree of therapist-level nesting (intra-class correlation), balanced vs. unbalanced assignment of patients to therapists, and fixed vs random within-patient coefficients. We estimated all models using longitudinal multilevel and structural equation models that ignored (2-level model) or modeled therapist effects (3-level model).
Results: Across all conditions, 2-level models performed equally or were superior to 3-level models. Within-patient coefficients were unbiased in both 2- and 3-level models. In 3-level models, standard errors were biased when number of therapists was small, and this bias increased in unbalanced designs. Ignoring random slopes led to biased standard errors when slope variance was large; but 2-level models still outperformed 3-level models.
Conclusions: In contrast to treatment outcome research, when studying mechanisms of change on a within-patient level, modeling therapist effects may even reduce model performance and increase bias.
目的:在研究心理治療療效時,目前的研究證據強調將治療師效果的差異放入模式的重要性。然而迄今尚無研究檢驗這個主張是否適用於研究改變機制時的患者內部效應。本研究探討在研究改變機制的患者內層級時是否應該將治療師效果放入模式。 方法:我們進行了蒙地卡羅模擬分析研究,包括改變患者與治療師層次的樣本數、治療師層次的鑲嵌程度(組內相關)、患者對治療師的平衡與不平衡分配以及固定與隨機的患者內係數。我們以不放入(二階模式)及放入(三階模式)治療師效果的縱貫多層次和結構方程模式來估算所有模式。 結果:在所有條件下,二階模式的表現等同或優於三階模式。在二階和三階模式中, 患者內係數均無偏誤。在三階模式中,當治療師人數較少時,標準誤差會出現偏誤, 而這種偏誤會因不平衡分配的設計而增加。當斜率變異數較大時,忽略隨機斜率會導致標準誤差有偏誤;但二階模式仍勝過三階模式。 結論:與治療療效研究相反,當在患者內層次研究改變機制時,將治療師效果放入模式可能會降低模式的表現並增加偏差。
Objetivo: A evidência existente destaca a importância de modelar a eficácia diferencial do terapeuta ao estudar o resultado da psicoterapia. No entanto, nenhum estudo até o momento examinou se esta afirmação se aplica ao estudo dos efeitos intra-paciente nos mecanismos de mudança. O estudo procurou investigar se os efeitos do terapeuta devem ser modelados ao estudar mecanismos de mudança ao nível intra-paciente. Métodos: Conduziu-se um estudo de simulação de Monte Carlo, variando os tamanhos de amostra ao nível de paciente e terapeuta, grau de aninhamento ao nível do terapeuta (correlação intraclasse), atribuição balanceada vs. desequilibrada de pacientes aos terapeutas e coeficientes entre-pacientes fixos vs. aleatórios. Analisaram-se todos os modelos usando modelos de equações estruturais e longitudinais multinível que ignoraram (modelo de 2 níveis) ou modelaram os efeitos do terapeuta (modelo de 3 níveis). Resultados: Em todas as condições, os modelos de 2 níveis tiveram um desempenho igual ou superior aos modelos de 3 níveis. Os coeficientes intra-paciente foram imparciais nos modelos de 2 e 3 níveis. Em modelos de 3 níveis, os erros-padrão foram enviesados quando o número de terapeutas era pequeno, e esse viés aumentou em desenhos não-balanceados. Ignorar inclinações aleatórias resultou em erros padrão enviesados quando a variância da inclinação era grande; mas os modelos de 2 níveis conseguiram superarar os modelos de 3 níveis. Conclusões: Em contraste com a investigação de resultados do tratamento, ao estudar os mecanismos de mudança ao nível intra-paciente, modelar os efeitos do terapeuta pode até reduzir a performance do modelo e aumentar o seu enviesamento.
Obiettivo: Le evidenze esistenti sottolineano l'importanza di modellizzare l'efficacia del terapeuta quando si studia l'esito in psicoterapia. Tuttavia, nessuno studio ha esaminato se questa affermazione si applichi allo studio degli effetti al livello entro il paziente nei meccanismi di cambiamento. Lo studio ha indagato se gli effetti del terapeuta dovrebbero essere modellizzati quando si studiano i meccanismi di cambiamento entro il paziente. Metodo: Abbiamo condotto uno studio di simulazione Monte Carlo, variando le dimensioni del campione a livello dei pazienti e dei terapeuti, grado di annidamento a livello del terapeuta (correlazioni intra-classe), accoppiamenti paziente-terapeuta bilanciati vs. non bilanciati, e coefficienti entro il paziente fissi vs random. Abbiamo stimato tutti i modelli usando modelli longitudinali multilivello emodelli di equazioni strutturali che ignoravano (modello a due livelli) o modellizzassero gli effetti del terapeuta (modello a tre livelli). Risultati: In tutte le condizioni, i modelli a due livelli si sono comportati allo stesso modo o erano superiori ai modelli a 3 livelli.Entro il pazientei coefficienti erano imparziali in entrambi i modelli a 2 e 3 livelli. Nei modelli a 3 livelli, gli errori standard erano distorti quando il numero di terapeuta erano piccoli e questo pregiudizio aumentava nei progetti sbilanciati. Ignorare le pendenze casuali ha portato a errori standard distortiquando la varianza della pendenza era grande; ma i modelli a 2 livelli hanno ancora sovraperformato i modelli a 3 livelli. Conclusioni: Contrariamente alla ricerca sull'esito del trattamento, quando si studiano i meccanismi di cambiamento a livello interno al paziente,gli effetti del terapeuta di modellazione possono persino ridurre le prestazioni del modello e aumentare il bias.
Clinical or Methodological Significance of this Article: Our findings suggest that therapist effects can be ignored in longitudinal studies focusing on within-patient effects. Including therapist effects in statistical models focusing on within-patient effects when the number of therapists is small and/or therapists treat unequal numbers of patients may even increase the risk of biased inference.
In recent years, there has been an upsurge of interest in studying mechanisms of change in psychotherapy research. A mechanism of change is a theoretically postulated underlying target for therapeutic interventions that, if changed, theoretically leads to change in outcome (Kazdin, Citation2007). Therapeutic approaches differ in hypothesized mechanisms presumed to lead to clinical improvement. For example, cognitive therapy for depression targets patients’ distorted cognitions about the self, the world, and the future (Beck et al., Citation1979). Psychodynamic therapy facilitates patients’ insight into problematic relationship patterns, awareness of avoided emotions, and/or a corrective emotional experiences with the therapist (Summers & Barber, Citation2010). Some mechanisms are trans-theoretical and assumed to facilitate therapeutic change across treatment models (e.g., the working alliance).
This increased focus on change mechanisms has been accompanied by an emphasis on collecting longitudinal data with repeated measurements of both candidate mechanisms and outcome throughout treatment, allowing researchers to study nuanced and complex associations over time (Falkenström et al., Citation2017). Recent decades have also been marked with developments in the statistical modeling of longitudinal data and increased awareness of their complexity (e.g., Allison et al., Citation2017; Curran et al., Citation2014; Curran & Bauer, Citation2011; Hamaker et al., Citation2015; Wang & Maxwell, Citation2015; Zyphur et al., Citation2019). One such complexity is the issue of separating within- from between patient effects. Traditionally, mechanisms of change research focused on between-patient effects—examining whether average differences in a mechanism across patients predicts change in outcome across patients. Within-patient effects are relationships between fluctuations over time in two or more variables for each given patient (Hamaker, Citation2012; Lundh & Falkenström, Citation2019; Molenaar & Campbell, Citation2009). That is, within-patient effects focus on associations between variables over time (across time-points) for each given patient. In contrast to between-patient effects, within-patient effects are not influenced by any factor that does not change during the time of the study. This characteristic of within-patient effects allows the exclusion of a number of alternative explanations connected to potentially confounding stable variables that are relegated to the between-patient level, and thus provide stronger evidence for causality. Conceptually, within-patient effects are also better aligned with theories of therapeutic change that focus on change processes on an individual-patient level, as well as recent emphasis on personalization of therapeutic processes (Barber & Solomonov, Citation2019; Rubel et al., Citation2019). Our focus on mechanisms of change should not be confused with mediation analysis, which is a method commonly used for studying mechanisms of change by estimating indirect effects of treatment on outcome via a candidate mechanism. Our focus is instead on within-patient fluctuations in a candidate mechanism predicting within-patient changes in outcome, which we would argue is a method well-suited for studying mechanisms of change (Falkenström et al., Citation2020).
Therapist Effects in Mechanisms of Change Research
Therapists vary in their ability to help their patients get better (e.g., Baldwin & Imel, Citation2013). While therapists’ efficacy in reducing symptomatic distress has been widely studied, little is known about the effects of therapists’ variability in facilitating improvement in candidate mechanisms of change. Inclusion of therapist effects when modeling change over time requires attention to “nesting”—most therapists treat more than one patient, and thus observations collected from their patients are dependent. This nested structure violates the assumption of independence of observations required for most statistical tests (Adelson & Owen, Citation2012).
Early on, Crits-Christoph and Mintz (Citation1991) noted the risk for increased Type-I error rate, and overestimation of population treatment effects, if nesting of patients within therapists is ignored. Since then, a number of simulation studies have confirmed this premise, suggesting that therapist effects should be modeled when examining outcome over time to avoid bias in the estimation of treatment effects and increased risk for Type-I error (de Jong et al., Citation2010; Magnusson et al., Citation2018; Wampold & Serlin, Citation2000). The most common method to adjust for therapist effects is allowing for variation in outcome among therapists by inclusion of random effects in multilevel models (e.g., Snijders & Bosker, Citation2012). While therapist effects in outcome studies have been relatively widely studied, much less is known regarding the impact of modeling therapist effects in the study of mechanisms of change, especially when studying within-patient effects.
Therapist Effects in Within-patient Studies
Consider first a simple within-patient regression model, also called fixed effects or mean centered model, in which patient means in both independent and dependent variables are eliminated by person-mean centering, i.e.:
(Fixed effects/mean centered model)
In this model, is outcome for individual i at time t,
is the intercept term,
is the effect of X for individual i at time t-1, and
is the model error term.Footnote1 All between-patient variance is eliminated by person-mean centering, i.e., subtracting the patient means from each observation of Y and X. What is left after centering is time-specific deviations from each patient’s mean, which constitute the within-patient scores. Regression of within-patient scores in Y on within-patient scores in X yields the within-patient effect. In this model, therapist mean differences in Y and X are both eliminated through mean centering, because when there are no mean differences among patients, there are no therapist differences either.
Multilevel modeling (MLM). In MLM, person-level means in Y are not eliminated by mean centering, but rather modeled using a random intercept term, which captures the variance in means across individuals. Similar to mean centering, what remains is within-patient deviation scores in Y. However, in standard MLM it is not possible to estimate a random intercept for X. In order to acquire a true within-patient regression model, X still needs to be person-mean centered manually. The person-mean centered model (Firebaugh et al., Citation2013; Hoffman & Stawski, Citation2009), combines these two methods by using mean centering/fixed effect for X but random effect for Y. It is one of the most commonly used within-patient effects model in psychotherapy research:
Level 1:
Level 2:
(2-level Person-mean centered model)
In this model, Level-1 refers to the within-patient level, i.e., repeated measurements across time, and Level-2 refers to the between-patient level. Between-person variation in Y is captured by the patient-level random intercept , while X is still person-mean centered. The estimate for coefficient
should, however, be the same as in the fixed effects model. The advantage of the hybrid random effects model, compared to the fixed effects model, is that between-patient variance in Y is not eliminated but can be modeled and potentially explained using between-patient predictors. For instance, the Level-2 intercept
, can, in principle, be modeled further by adding a random intercept on the therapist level, thus creating a three-level model with repeated measures nested within patients, which in turn are nested within therapists:
Level 1:
Level 2:
Level 3:
(3-level Person-mean centered model)
In this model, we have added Level-3 which is the between-therapist level. represents outcome for therapist T with patient i at time t. X is centered at the patient level, as in the previous model. The only difference between this and the previous model is that the additional random effect
models the between-therapist variance among patient-level means, i.e., it is
in the previous model which is further divided into one between-therapist component
and one within-therapist deviation component
.
The consensus in psychotherapy research suggests that ignoring a level of nesting in the model (in our case—therapists) can lead to a bias in standard errors resulting in too liberal testing (e.g., Crits-Christoph & Mintz, Citation1991; Wampold & Serlin, Citation2000). Although usually not explicitly stated, this refers to when the ignored level is the one immediately “above” the one on which the effect of interest is located, e.g., ignoring therapist effects (Level-3) when focusing on differential outcome among patients (a Level-2 effect). However, in the case of Level-1 effects, Moerbeek (Citation2004) argued that in balanced designs these are unaffected by the omission of Level-3 nesting, because variance components at Level-3 will only be redistributed at Level-2, leaving Level-1 unaffected. Thus, leaving out Level-3 will affect standard errors at Level-2, but not at Level-1. Given that psychotherapy studies are almost invariably unbalanced, i.e., in our case when therapists treat equal numbers of patients, this finding may not apply. Thus, there is a need to evaluate the impact of ignoring therapist effects in within-person mechanism of change studies.
So far, we have only considered therapist effects in the form of average differences in predictor and/or outcome variable among therapists. However, there is also the possibility that the within-patient coefficient itself (i.e., ) may vary among therapists. For instance, in alliance-outcome research, it is possible that the lagged effect of alliance on symptomatic distress in the next session will be stronger for some therapists than for others, perhaps because some therapists are more able to utilize the alliance in an effective way. Alternatively, the effect of challenging cognitions on subsequent depressive symptoms may be more pronounced in therapists working within a cognitive model than in therapists working within a psychodynamic model of depression. The following equations show the hybrid random effects model with a therapist-level random slope:
Level 1:
Level 2:
Level 3:
(3-level Person-mean centered model with random slopes)
In this model we have allowed the slope of the within-patient coefficient to vary across therapists and patients. The variation in slopes across patients, within therapists, is captured by the term
, and the variation in slopes across therapists is captured by the term
. If the above is the true population model, and estimation is carried out using a fixed coefficient model, results may be biased (Baird & Maxwell, Citation2016). To our knowledge, there are no studies testing whether ignoring therapist-level random slopes affects estimates of within-person effects.
Limitations of MLM estimation of lagged within-person effects. So far, we have considered only a fairly simple lagged effect model within an MLM framework. This model ignores several complexities that may be present in time-series or panel data frameworks, some which can be addressed within an SEM framework. First, there is the issue of autoregression, i.e., the influence of the outcome variable at time t on itself at time t+1. This effect needs to be adjusted for, otherwise we need to assume that there are no pre-existing differences in Y at time t. Adjusting for the prior value of Y means that lagged X predicts residualized change in Y, thus ensuring correct temporality—i.e., that the prediction of Y at time t+1 from X at time t are not purely a consequence pre-existing differences in Y at time t.
Within the standard MLM framework, it is complicated to estimate autoregression while simultaneously separating within- from between-patient variance. If a lagged dependent variable is added as a covariate, the estimated model coefficients will be biased. This is variously called “dynamic panel bias” or “Nickel’s bias” (Nickell, Citation1981). It is possible to allow an autoregressive structure of the residuals, but this model still does not fully take into account the dynamic implications of the time-series data structure (Asparouhov & Muthén, Citation2019).
Another limitation of the standard MLM framework is that it assumes unidirectionality, and thus, does not include reverse effects, i.e., “feedback effects,” from the dependent variable back to the predictor (i.e., from Y to X). In studies of working alliance and psychological distress, for instance, it is now fairly well-established that these variables mutually influence each other over time, so that higher alliance scores in one session predicts lower distress in the next session, which in turn predicts higher alliance quality and so on (e.g., Falkenström et al., Citation2013; Xu & Tracey, Citation2015; Zilcha-Mano & Errázuriz, Citation2015). In SEM framework, this issue can be addressed through modeling of bidirectional relationships in cross-lagged panel models (e.g., Hamaker et al., Citation2015).
Structural Equation Modeling. In SEM several equations are estimated simultaneously, with data in wide format (i.e., one row per patient in a data frame) where each variable and each wave of data has its own equation, e.g.:
(Unidirectional Cross-lagged panel model)
In these equations, is the intercept, while
is the coefficient for the cross-lagged effect of X on Y.Footnote2 In addition, we have here incorporated the autoregressive effect, which is captured in
The above equations only incorporate three waves, but additional waves can easily be added.
To model the reverse effects, i.e., the feedback from Y to X, we add the following equations:
(Bidirectional Cross-lagged panel model)
Here, is the intercept,
is the coefficient for the cross-lagged effect of Y on X, and
is the autoregression coefficient for X. This model, with all the above equations estimated simultaneously, is called cross-lagged panel model. Usually, the error terms at the same time-point are allowed to covary (i.e.,
and
and
, and so on) to capture contemporaneous relationships between variables.
In the classical cross-lagged panel model, within- and between-patient effects are conflated. The separation of within- and between-patient variances in the dependent variable is accomplished in both MLM and SEM through the inclusion of random intercept terms. This turns SEM into Multilevel SEM (ML-SEM; e.g., Mehta & Neale, Citation2005), which will be represented by the addition of the person-specific random intercept This is shown in the following equation (now using general rather than time-specific notation as above):
The difference from standard MLM is that in ML-SEM it is possible to add a random intercept term for X:
Similar to person-mean centering in MLM, the inclusion of a random intercept term for X separates the person-specific means, that are captured in the random intercept, from the time-specific deviations from the person’s mean that are used for the cross-lagged model. The above model is called Random Intercept Cross-Lagged Panel Model (RI-CLPM; Hamaker et al., Citation2015). The random intercepts and
are allowed to covary, since it is likely that if there is a relationship between X and Y at the within-person level, there will also be a relationship between X and Y at the between-person level. Due to the fact that between- and within levels are separated by means of random effects in both X and Y, the problem of modeling autoregression that is present in MLM does not occur in SEM (Allison et al., Citation2017). Again, however, adding a third level random intercept will simply model the between-therapist variances in patient-level means; i.e.,
and
are further separated into between- and within-therapist components:
(RI-CLPM with random intercepts for therapists)
Theoretically, the within-patient estimates for and
should be unaffected, since
and
only models the variances in
and
, respectively.
Limitations of SEM estimation of lagged within-person effects. As in MLM, there is the possibility that (or any other within-level coefficient) varies among patients and/or therapists. Estimating random slope models in ML-SEM is possible, just as in standard MLM, although this requires numerical integration which is computationally burdensome and time-consuming, and may become intractable when the number of integration dimensions becomes large (Asparouhov & Muthén, Citation2007).
The Simulation Study
The aim of this Monte Carlo simulation study was to test the impact of ignoring the nesting of patients within therapists in within-patient analyses, under various conditions reasonably representative for psychotherapy research. The focus of this analysis was in the previous equations, i.e., the within-patient cross-lagged effect of X on Y. A recent study of therapist effects in outcome research suggested bias when ignoring therapist effects in samples with few therapists, many patients per therapist, large proportion of variance at the therapist level (relative to the within-patient residual variance), and unbalanced allocation of patients to therapists (Magnusson et al., Citation2018). Thus, we decided to experimentally manipulate the following: (a) degree of therapist-level nesting; (b) number of therapists; (c) number of patients per therapist; (d) MLM and SEM models; and (e) balanced and unbalanced allocation of patients to therapists. In addition, we tested the impact of ignoring therapist-level random slopes for the within-patient coefficient under (1) different total slope variances; and (2) different proportions of slope variance at the therapist level, as well as conditions (a)–(d) above.
Models and Software
We created the datasets and estimated MLM simulations using the Person-mean centered model, and SEM models using the RI-CLPM (for equations see above) using Mplus v.8.1.7 (Muthén & Muthén, Citation1998-2017) and the MplusAutomation package in R (Hallquist & Wiley, Citation2018). All models were estimated using Maximum Likelihood with robust standard errors.
Fixed Conditions
We set the effect size (standardized regression coefficient) for the cross-lagged effect to β = .30. In addition, we also tested β = 0 to see whether the models correctly indicate non-significance when there is no effect in the population, and to calculate the empirical alpha level. For the RI-CLPM, we set the autoregressive effects and
to .50 and the contemporaneous error correlations to .20.
Experimental Conditions for Data Generation
Degree of nesting. We included three conditions for the Intraclass Correlation Coefficient (ICC) at the therapist level: (a) ICC = 0 i.e., no nesting at therapist level, (b) ICC = .05; the average ICC reported in meta-analyses of therapist effects in psychotherapy research (e.g., Baldwin & Imel, Citation2013); (c) ICC = .20; and (d) ICC = .40. Although an ICC of .40 may seem large, this ICC size may be found when therapists differ in their self-report style on change in mechanisms (Hatcher et al., Citation2019). In all simulations, we assigned 50% of variance to Level-1, the within-patient (repeated measures) level, while splitting the remaining 50% variance between Level-2 and Level-3 depending on the therapist-level ICC. For example, when the therapist-level ICC was set to .05, Level-3 was assigned 5%, and Level-2 45% of total variance, while when the ICC was set to .40, Level-3 was assigned 40% variance and Level-2 10%.
Sample size. We created datasets with five repeated measurements, nested within 50, 100 or 200 patients, to reflect fairly realistic scenarios in psychotherapy studies (e.g., Lambert, Citation2013).
Number of therapists and number of patients per therapist. Each sample size was created with a different number of therapists, and a different number of patients per therapist. Thus, N = 50 was either 5 therapists with 10 patients each, or 10 therapists with five patients each, N = 100 was either five therapists with 20 patients each or 20 therapists with five patients each, and N = 200 was five therapists with 40 patients each, 20 therapists with 10 patients each, or 40 therapists with five patients each.
Unbalanced allocation. We created six conditions with unbalanced allocation of patients to therapists, common in psychotherapy studies. Within each of these sample sizes we created two unbalanced conditions—one with relatively many therapists and one with relatively few therapists. We varied the numbers of patients treated by therapists as much as possible, within reasonable limits. The conditions were the following:
N = 50. (a) Two therapists treating 15 patients each and ten therapists treating two patients each (total of 12 therapists); (b) One therapist treating 30 patients, two therapists treating eight patients each, and two therapists treating two patients each (total of five therapists).
N = 100. (a) Two therapists treating 20 patients, four therapists treating ten patients each, and ten therapists treating two patients each (total of 16 therapists); (b) Two therapists treating 25 patients, two therapists treating 15 patients each, and two therapists treating 10 patients each (total of six therapists).
N = 200 (a) Three therapists treating 25 patients each, five therapists treating 15 patients each, and ten therapists treating five patients each (total of 18 therapists); (b) Two therapists treating 50 patients each, three therapists treating 30 patients each, and two therapists treating five patients each (total of seven therapists).
Random slopes for the cross-lagged effect. We chose large values for the slope variances to create conditions where potential problems can emerge while remaining within reasonable realistic conditions. Since the fixed effect was set to a standardized regression coefficient of .30, a therapist-level standard deviation of 0.15 would mean that approximately 95% (corresponding to about 2 standard deviation units) of the therapists had effects that were larger than zero. With larger variation than that, a substantial proportion of patients would have zero or even negative effects of the mechanism on outcome, which seems unlikely. Thus, we chose SD = 0.15 as our upper boundary for the total between-therapist variation in slopes. We also examined a condition of SD = 0.10, to test a somewhat smaller variation in slopes. In the next step, we included the Slope Intraclass Correlation, or Slope ICC (Magnusson et al., Citation2018), which represents therapists’ contribution to the total slope variance. Slope ICC is calculated as the therapist-level variance in slopes divided by the total variance in slopes (i.e., therapist + patient level variances). Since meta-analytic evidence has shown therapist effects on outcome to be between 5-10% (Baldwin & Imel, Citation2013), we set the Slope ICC to be either .05, .10 or .20.
Estimation and Assessment of Estimator Performance
In each condition, we created 2000 samples and subsequently analyzed the data using the Person-mean centered MLM or RI-CLPM, in one of two formats: (1) a 2-level model which ignored therapist-level nesting and (2) a 3-level model which took therapist-level nesting into account. The difference between these two models is thus whether the v variables were included or not, i.e., /
in the Person-mean centered MLM, and the
and
in the RI-CLPM. We tested each model estimates for:
Proportional coefficient bias. Calculated as the difference between the average estimate of β and the true population value for β, divided by the true population value for β, i.e.,
. This represents the percentage average bias from the true coefficient value. A rule of thumb for unbiased models is that this should not exceed 5% (Muthén & Muthén, Citation2002). For the models with true population value β = 0, coefficient bias cannot be calculated as a proportion since that would involve division by zero. Instead, we report the average deviation score (which will be the average estimate of β).
Coverage of the 95% confidence intervals. This is calculated as the proportion of times the estimated 95% confidence interval of β contains the true effect. Coverage is considered a robust indicator of standard error estimation (T. Asparouhov, personal communication, January 2nd, 2020). Coverage should be close to 95%, and a rule of thumb is that it should be between 91 and 98% (Muthén & Muthén, Citation2002).
Statistical power. This is calculated as the proportion of times the model yielded a statistically significant effect when the population effect was non-zero. As is common in the behavioral sciences, we used 80% power as the criterion for large-enough statistical power (Cohen, Citation1992).
Empirical alpha level. In models in which the population effect of β was set to zero, the proportion of times the model yielded a false-positive statistically significant effect can be interpreted as the empirical alpha level. This should be close to 5%.
Results
Overall, results of the MLM and SEM models were similar across conditions (instances of differences are described below). Model convergence rates were good, with 100% of MLM models and between 97.8-100% of SEM models converging, with the exception for the 3-level SEM models with random slopes for which none of the estimations converged. The complete results are summarized in –.3
Balanced Allocation of Patients to Therapists ( and Tables S2-S3)
Degree of therapist-level nesting (ICC). With balanced assignment of patients to therapists, ignoring therapist-level nesting did not impact estimated within-patient coefficients. In MLM, with manually patient-mean centered predictor variables, the estimates were identical for conditions of ICC = .00, .05, .20, or .40 (). In SEM, where centering is accomplished by latent variable modeling, estimates were highly similar but not identical (Tables S2 and S3).
Table I. Simulation results for Person-mean centered model with balanced assignment of patients to therapists at ICC = 0, .05, .20, and .40a.
Number of therapists. The 3-level models showed poor performance in the estimation of standard errors when the number of therapists was small. For the 3-level model, at least 10 therapists were needed to achieve accurate estimation of standard errors, as shown by coverage rates falling below 91% and alpha levels increasing to ∼ 15% in the conditions with only five therapists (, Table S2 and Table S3). Thus, estimating a 3-level model with too few therapists increases the risk of Type-I error. In contrast, the 2-level model, which ignores therapist-level nesting, showed no problems regardless of the number of therapists.
Number of patients per therapist. Overall, the number of patients per therapist did not impact the performance of neither 2- nor 3-level models for MLM and SEM (see , S2 and S3).
Unbalanced Allocation of Patients to Therapists (, and S4)
For both MLM () and SEM ( and S4), the two-level model was unaffected by unbalanced allocation, while the 3-level model showed poorer performance in terms of bias in standard errors compared to balanced conditions. Coverage rates for the three-level models were below the 91% threshold in almost all conditions, and alpha levels ranged between 9 and 24%.
Table II. Simulation results for Person-mean centered model with unbalanced assignment of patients to therapists at ICC = 0, .05, .20, and .40.
Degree of therapist-level nesting (ICC). Results were comparable to the balanced conditions, such that estimates did not depend on the degree of nesting (for MLM exactly identical results across all ICC levels, for SEM similar but not identical results).
Number of therapists. In the 3-level models, results were similar to the balanced conditions such that the largest bias was seen in conditions with few therapists. In contrast, the 2-level models were unaffected by unbalanced assignment, regardless of the number of therapists.
Number of patients per therapist. Since the number of patients per therapist varied within each condition (by definition to create unbalanced data), it is not possible to make inferences with certainty regarding results under this condition.
Varying Coefficients among Therapists (Random Slope Models; Tables , and S1)
We created data for which the slopes for the Level-1 coefficient varied at both Level-2 (patients) and Level-3 (therapists). The 2-level estimation models were the same as previously, i.e., assuming a fixed Level-1 coefficient—thus ignoring random slopes altogether,Footnote4 while the 3-level model estimated random slopes on both Level-2 and -3. Overall, results were mostly unbiased in the fixed coefficient (2-level) model, in both MLM ( and S1) and SEM (). Of note, none of the 3-level SEM models converged. In MLM, 3-level models converged but there were problems in the estimation of standard errors in some of the conditions.
Table III. Simulation results for Person-mean centered model with therapist-level random slopes (slope SD = 0.15).
Table IV. Simulation results for Random Intercept Cross-Lagged Panel Model with unbalanced assignment of patients to therapists at ICC = 0, .05, .20, and .40 and β = .30.
Table V. Two-level simulation results for Random Intercept Cross-Lagged Panel Model, ignoring therapist-level random slopes.
Total slope variance. In conditions with larger total slope variance (SD = 0.15, compared to SD = 0.10) the 2-level model showed slightly worse coverage rates (, S1 and ). For the 3-level model, the amount of total slope variance did not impact estimation performance. However, in both conditions the 2-level model performed better than the 3-level model.
Proportion of slope variance at the therapist level (ICCslope). For the 2-level model, a larger proportion of slope variance at the therapist level reduced coverage rates slightly. The 3-level model was almost unaffected by the increased slope ICC.
Number of therapists. In this condition, increasing the number of therapists improved estimates in both the 3-level and 2-level models. However, this effect was smaller for the 2-level model than the 3-level model.
Number of patients per therapist. In the 2-level model, larger number of patients per therapist was related to worse estimation of standard error. However, this effect was relatively small when evaluated by coverage rate of the 95% confidence intervals. For the 3-level model, the number of patients per therapist did not affect estimation performance.
In sum, the 2-level model was superior to the 3-level model in all random slope conditions. The 2-level model performance was reduced in the following conditions: (1) the variance in slopes at the therapist level was very large; (2) the number of therapists was small (< 10); (3) the number of patients per therapist was very large (40 or more). Nevertheless, even in its worst condition (slope SD = 0.15, slope ICC = .20, N = 200 and five therapists), the 2-level model still outperformed the 3-level model.
Discussion
Our results suggest that therapist effects do not have a significant impact on within-patient estimates in mechanisms of change studies. Consistent with previous work (Moerbeek, Citation2004), therapist effects did not affect estimates in balanced design conditions (when all therapists treat the same number of patients), and with within-patient coefficients fixed to be the same across all therapists. This is the first study to expand this finding to various study conditions, including unbalanced designs (unequal assignment of patients to therapists), and variation in within-patient coefficients across therapists. We found that if anything, inclusion of therapist effects in 3-level models in these designs reduced model performance compared to when therapist effects were ignored (i.e., 2-level models).
This is the first study to show that therapist effects can be ignored when within-patient effects (i.e., Level-1 effects) are the primary focus in either MLM or SEM frameworks across various conditions. Taking therapist effects into account did not improve model performance in any of the simulation conditions. When the number of therapists was small, 3-level models resulted in increased risk of Type-I error. Although it is possible to improve estimation of MLM with small samples on the highest level, e.g., by using Restricted Maximum Likelihood with adjusted degrees of freedom (McNeish, Citation2017), this is seldom done in psychotherapy studies.
Previous simulation studies that focused on the study of treatment efficacy have shown that therapist effects should be modeled when investigating differential treatment effects, estimated at between-patient levels (Magnusson et al., Citation2018). Our results suggest that this conclusion does not apply to the study of within-patient effects (i.e., Level-1 effects in MLM terminology). This result challenges a common tendency to include therapist effects as a better safe than sorry strategy in all psychotherapy studies.
Our findings also suggest that ignoring therapist-level random slopes does not, generally, affect coefficient estimates (which represent a weighted average between therapists). An exception was for SEM models with very small samples (N = 50), the coefficients were slightly downward biased (just above the 5% criterion). Standard errors were biased only if the slope variance at the therapist level was large, the number of therapists small, and/or the number of patients per therapist was large. Still, 2-level models outperformed 3-level models even in the most stringent condition, likely uncommon in psychotherapy studies, with a slope SD = 0.15 (in the context of standardized regression coefficients), slope ICC = .20, and only five therapists who treat 40 patients each. Notably, the simulation study by Magnusson et al. (Citation2018) found substantial bias when ignoring random slopes of similar magnitudesFootnote5 when studying the effects of ignoring therapist effects in outcome research (i.e., the effect of ignoring Level-3 on Level-2 coefficients).
Our simulations showed that statistical power needed to find significant effects was above 90% in all MLM conditions tested, and above 80% for all SEM conditions, even when sample size was as small as N = 50. Although the effect size used in simulations was fairly large (β = .30), this finding is still notable. The high statistical power of these models is most likely attributed to additional information provided by repeated observations beyond sample size, i.e., with N = 50 and T = 5, there are 50 × 4 = 200 observationsFootnote6 used to calculate the within-patient effect.
MLM and SEM showed similar findings, although with slightly higher bias and lower power for the SEM models. Considering the greater complexity of the SEM models used, the reduction in power was smaller than expected, suggesting good performance of these models. Notably, an important disadvantage of MLM compared to SEM models is that they do not allow adjusting the cross-lagged coefficient estimate for the dependent variable at the prior session. Ignoring the autoregressive effect in panel data results in mis-specified models and in turn in an increased risk for biased estimates of the cross-lagged effects of interest.
Our findings should be considered in the context of several limitations. First, we were unable to estimate therapist-level random slope models in any of the SEM conditions tested. If a researcher is interested in the average effect of a putative mechanism (i.e., the within-patient effect), results from our 2-level models show that random slopes can be ignored as long as they are not very large, the number of therapists is not very small, and the number of patients per therapist is not very large. In practice, the size of random slopes is of course unknown, but if the other conditions (i.e., small number of therapists and large number of patients per therapist) are fulfilled, a researcher should consider whether large slope variance at the therapist level is expected. For our simulation study, we chose the maximum slope variance so that 95% of the slopes would not cross zero, because it is unlikely that any theoretically proposed mechanism in psychotherapy research would have opposite effects among therapists. However, if in a particular research context this may be a possibility, our simulation results may not hold.
If therapist-level random slopes are the focus of research interest, SEM might not be the best modeling framework to use. As mentioned, estimating random slopes in SEM is complex and requires numerical integration which is highly computationally demanding and time-consuming (Asparouhov & Muthén, Citation2007). Other estimators, such as Bayesian estimation, might be preferable but are beyond the scope of the current study. Additionally, it is likely that the performance of the 3-level MLM models with small numbers of therapists would have been improved with Restricted Maximum Likelihood, perhaps with some adjustment to degrees of freedom (McNeish, Citation2017). However, since the focus of our study was to investigate whether therapist effects impact within-patient estimates in 2-level models rather than testing the performance of 3-level models in small samples, we did not pursue this further.
Second, for feasibility purposes and given the number of conditions tested, we chose to focus on an effect size of β = .30, which represents a medium-sized effect, common in mechanisms of change research. Of note, the β = 0 models ( and ) showed very similar findings as β = .30, and we also tested some of our models using smaller (0.10 and 0.20) and larger (.40 and .50) effects and results were comparable. Future studies can expand our work by testing these models using a range of effect sizes.
Conclusions, Recommendations, and Suggestions for Future Research
Due to the increased interest in mechanisms of change research in general, and within-patient effects in particular, it is vital that researchers use the most robust and accurate methods available. Since adjusting for therapist effects has proven to be important in other areas of psychotherapy research, it is understandable that many researchers believe that this is the case for within-patient mechanism studies. However, our simulation study shows that when interest is in within-patient associations, and models are used that separate these from between-patient differences, the safest strategy is to ignore therapist effects. Thus, we recommend using 2-level models (i.e., repeated measures nested within patients) when estimating these effects. If researchers are specifically interested in therapist effects, these should be models with an effort to recruit a sufficient number of therapists. Assuming an unbalanced assignment of patients to therapists this should likely be at least 15–20 therapists. Alternatively, with fewer therapists, differences among therapists can be modeled using a fixed effects approach (Snijders & Bosker, Citation2012).
Further research is needed on optimal estimation of random slopes in SEM models. The newly developed Dynamic Structural Equation Model, which can be used for similar research questions (i.e., estimating within-patient cross-lagged coefficients) may be optimal, given its combination of the advantages of both MLM and SEM approaches (DSEM; Asparouhov et al., Citation2018). However, at present, it requires at least around 10 repeated measurements for stable effects and good model performance (Schultzberg & Muthén, Citation2018).
The growing interest in personalization of psychotherapy processes and the study of individual differences across treatment requires use of complex modeling techniques. Our study demonstrates that such modeling should be used with caution and awareness of potential pitfalls and challenges that may affect inferences from estimation results. When studying within-person effects, we encourage researchers to expand our line of inquiry by cautiously considering the impact of their study conditions on modeling, selecting the most optimal and appropriate statistical approach, and investigating whether results are replicated under a range of conditions. As with treatment packages, therapeutic processes, and mechanisms of change, when it comes to modeling—one size does not fit all.
Data Transparency Statement
The data for this study has never been used before.
Supplemental Material
Download MS Word (44.9 KB)Supplemental Data
Supplemental data for this article can be accessed at https://doi.org/10.1080/10503307.2020.1769875 description of location.
Additional information
Funding
Notes
1 Errors are assumed to be independent in all of the models, unless otherwise specified.
2 It is also possible in SEM to assign different coefficients to each time-point, thus allowing for different effects between, say, time 1 to time 2 than from time 2 to time 3, and so on. However, we focus on comparable effects in SEM and MLM and thus only discuss models in which (cross-) associations are constrained to be equal over time, i.e. there is only one coefficient for the within-person effect of X on Y.
3 More tables are included in the online supplement, see Tables S1-S4.
4 A model that estimated random slopes on Level-2, i.e. still ignoring the therapist-level, turned out to result in very similar estimates as the model with completely fixed slopes.
5 Our largest slope variance represents a variance ratio (slope variance/within-patient variance) of 0.022, which is in-between the two largest slope variance conditions (0.02 and 0.03) used in the study by Magnusson et al. (Citation2018). In that study, however, the largest slope ICC tested was .10 while we also included slope ICC = .20.
6 Due to lagging, there are only T-1 repeated observations used in the estimate of β, which is why we multiply by 4 instead of 5.
References
- Adelson, J. L., & Owen, J. (2012). Bringing the psychotherapist back: Basic concepts for reading articles examining therapist effects using multilevel modeling. Psychotherapy, 49(2), 152–162. https://doi.org/10.1037/a0023990
- Allison, P. D., Williams, R., & Moral-Benito, E. (2017, August). Maximum likelihood for cross-lagged panel models with fixed effects. Socius: Sociological Research for a Dynamic World, 3, 2378023117710578. https://doi.org/10.1177/2378023117710578
- Asparouhov, T., Hamaker, E. L., & Muthén, B. (2018). Dynamic structural equation models. Structural Equation Modeling: A Multidisciplinary Journal, 25(3), 359–388. https://doi.org/10.1080/10705511.2017.1406803
- Asparouhov, T., & Muthén, B. (2007). Computationally efficient estimation of multilevel high-dimensional latent variable models. Paper presented at the proceedings of the 2007 JSM meeting in Salt Lake City, Utah, Section on Statistics in Epidemiology.
- Asparouhov, T., & Muthén, B. (2019). Comparison of models for the analysis of intensive longitudinal data. Structural Equation Modeling: A Multidisciplinary Journal, 1–23. https://doi.org/10.1080/10705511.2019.1626733
- Baird, R., & Maxwell, S. E. (2016). Performance of time-varying predictors in multilevel models under an assumption of fixed or random effects. Psychological Methods, 21(2), 175–188. https://doi.org/10.1037/met0000070
- Baldwin, S. A., & Imel, Z. E. (2013). Therapist effects: Findings and methods. In M. J. Lambert (Ed.), Bergin and Garfield's handbook of psychotherapy and behavior change (6th ed., pp. 258–297). Wiley.
- Barber, J. P., & Solomonov, N. (2019). Toward a personalized approach to psychotherapy outcome and the study of therapeutic change. World Psychiatry, 18(3), 291–292. https://doi.org/10.1002/wps.20666
- Beck, A. T., Rush, A., Shaw, B., & Emery, G. (1979). Cognitive therapy of depression. Guilford Press.
- Cohen, J. (1992). A power primer. Psychological Bulletin, 112(1), 155–159. https://doi.org/10.1037/0033-2909.112.1.155
- Crits-Christoph, P., & Mintz, J. (1991). Implications of therapist effects for the design and analysis of comparative studies of psychotherapies. Journal of Consulting and Clinical Psychology, 59(1), 20–26. https://doi.org/10.1037/0022-006X.59.1.20
- Curran, P. J., & Bauer, D. J. (2011). The disaggregation of within-person and between-person effects in longitudinal models of change. Annual Review of Psychology, 62(1), 583–619. https://doi.org/10.1146/annurev.psych.093008.100356
- Curran, P. J., Howard, A. L., Bainter, S. a., Lane, S. T., & McGinley, J. S. (2014). The separation of between-person and within-person components of individual change over time: A latent curve model with structured residuals. Journal of Consulting and Clinical Psychology, 82(5), 879–894. https://doi.org/10.1037/a0035297
- de Jong, K., Moerbeek, M., & van der Leeden, R. (2010). A priori power analysis in longitudinal three-level multilevel models: An example with therapist effects. Psychotherapy Research, 20(3), 273–284. https://doi.org/10.1080/10503300903376320
- Falkenström, F., Finkel, S., Sandell, R., Rubel, J. A., & Holmqvist, R. (2017). Dynamic models of individual change in psychotherapy process research. Journal of Consulting and Clinical Psychology, 85(6), 537–549. https://doi.org/10.1037/ccp0000203
- Falkenström, F., Granström, F., & Holmqvist, R. (2013). Therapeutic alliance predicts symptomatic improvement session by session. Journal of Counseling Psychology, 60(3), 317–328. https://doi.org/10.1037/a0032258
- Falkenström, F., Solomonov, N., & Rubel, J. (2020). Using time-lagged panel data analysis to study mechanisms of change in psychotherapy research: Methodological recommendations. Counselling and Psychotherapy Research. https://doi.org/10.1002/capr.12293
- Firebaugh, G., Warner, C., & Massoglia, M. (2013). Fixed effects, random effects, and hybrid models for causal analysis. In L. S. Morgan (Ed.), Handbook of causal analysis for social research (pp. 113–132). Springer Netherlands.
- Hallquist, M. N., & Wiley, J. F. (2018). Mplusautomation: An R package for facilitating large-scale latent variable analyses in Mplus. Structural Equation Modeling: A Multidisciplinary Journal, 25(4), 621–638. https://doi.org/10.1080/10705511.2017.1402334
- Hamaker, E. L. (2012). Why researchers should think ‘within-person': A paradigmatic rationale. Handbook of Research Methods for Studying Daily Life, 43–61.
- Hamaker, E. L., Kuiper, R. M., & Grasman, R. P. P. P. (2015). A critique of the cross-lagged panel model. Psychological Methods, 20(1), 102–116. https://doi.org/10.1037/a0038889
- Hatcher, R. L., Lindqvist, K., & Falkenström, F. (2019). Psychometric evaluation of the working alliance Inventory—therapist version: Current and new short forms. Psychotherapy Research, 1–12. https://doi.org/10.1080/10503307.2019.1677964
- Hoffman, L., & Stawski, R. S. (2009). Persons as contexts: Evaluating between-person and within-person effects in longitudinal analysis. Research in Human Development, 6(2-3), 97–120. https://doi.org/10.1080/15427600902911189
- Kazdin, A. E. (2007). Mediators and mechanisms of change in psychotherapy research. Annual Review of Clinical Psychology, 3(1), 1–27. https://doi.org/10.1146/annurev.clinpsy.3.022806.091432
- Lambert, M. J. (2013). Bergin and Garfield's handbook of psychotherapy and behavior change (6th ed.). Wiley.
- Lundh, L. G., & Falkenström, F. (2019). Towards a person-oriented approach to psychotherapy research. Journal for Person-Oriented Research, 5(2), 65–79. https://doi.org/10.17505/jpor.2019.07
- Magnusson, K., Andersson, G., & Carlbring, P. (2018). The consequences of ignoring therapist effects in trials with longitudinal data: A simulation study. Journal of Consulting and Clinical Psychology, 86(9), 711–725. https://doi.org/10.1037/ccp0000333
- McNeish, D. (2017). Small sample methods for multilevel modeling: A colloquial elucidation of REML and the Kenward-Roger correction. Multivariate Behavioral Research, 52(5), 661–670. https://doi.org/10.1080/00273171.2017.1344538
- Mehta, P. D., & Neale, M. C. (2005). People are variables too: Multilevel structural equations modeling. Psychological Methods, 10(3), 259–284. https://doi.org/10.1037/1082-989X.10.3.259
- Moerbeek, M. (2004). The consequence of ignoring a level of nesting in multilevel analysis. Multivariate Behavioral Research, 39(1), 129–149. https://doi.org/10.1207/s15327906mbr3901_5
- Molenaar, P. C. M., & Campbell, C. G. (2009). The new person-specific paradigm in psychology. Current Directions in Psychological Science, 18(2), 112–117. https://doi.org/10.1111/j.1467-8721.2009.01619.x
- Muthén, L. K., & Muthén, B. O. (1998-2017). Mplus user's guide.
- Muthén, L. K., & Muthén, B. O. (2002). How to use a Monte Carlo study to decide on sample size and determine power. Structural Equation Modeling: A Multidisciplinary Journal, 9(4), 599–620. https://doi.org/10.1207/S15328007SEM0904_8
- Nickell, S. (1981). Biases in dynamic models with fixed effects. Econometrica, 49(6), 1417–1426. https://doi.org/10.2307/1911408
- Rubel, J. A., Zilcha-Mano, S., Giesemann, J., Prinz, J., & Lutz, W. (2019). Predicting personalized process-outcome associations in psychotherapy using machine learning approaches—A demonstration. Psychotherapy Research, 1–10. https://doi.org/10.1080/10503307.2019.1597994
- Schultzberg, M., & Muthén, B. (2018). Number of subjects and time points needed for multilevel time-series analysis: A simulation study of dynamic Structural equation modeling. Structural Equation Modeling: A Multidisciplinary Journal, 25(4), 495–515. https://doi.org/10.1080/10705511.2017.1392862
- Snijders, T. A. B., & Bosker, R. J. (2012). Multilevel analysis: An introduction to basic and advanced multilevel modeling. Sage Publishers.
- Summers, R. F., & Barber, J. P. (2010). Psychodynamic therapy: A guide to evidence-based practice. Guilford Press.
- Wampold, B. E., & Serlin, R. C. (2000). The consequence of ignoring a nested factor on measures of effect size in analysis of variance. Psychological Methods, 5(4), 425–433. https://doi.org/10.1037/1082-989X.5.4.425
- Wang, L. P., & Maxwell, S. E. (2015). On disaggregating between-person and within-person effects with longitudinal data using multilevel models. Psychological Methods, 20(1), 63–83. https://doi.org/10.1037/met0000030
- Xu, H., & Tracey, T. J. G. (2015). Reciprocal influence model of working alliance and therapeutic outcome over individual therapy course. Journal of Counseling Psychology, 62(3), 351–359. https://doi.org/10.1037/cou0000089
- Zilcha-Mano, S., & Errázuriz, P. (2015). One size does not fit all: Examining heterogeneity and identifying moderators of the alliance – outcome association. Journal of Counseling Psychology, 62(4), 579–591. doi:http://doi.org/10.1037/cou0000103
- Zyphur, M. J., Allison, P. D., Tay, L., Voelkle, M., Preacher, K. J., Zhang, Z., Hamaker, E. L., Shamsollahi, A., Pierides, D. C., Koval, P., & Diener, E. (2019). From data to causes I: Building a general cross-lagged panel model (GCLM). Organizational Research Methods. doi: 10.1177/1094428119847278