Abstract
Objective: While the CORE-10 inventory for Clinical Outcome Routine Evaluation has shown good psychometric properties in cross-sectional assessment, the feasibility of generic, short, and easy-to-use longitudinal assessment of a broadband construct such as the targeted “general psychological distress” remains to be psychometrically demonstrated. Therefore, we investigated longitudinal measurement invariance (LMI) of CORE-10. For comprehensiveness, we also analyzed its parent inventory, CORE-OM. Method: We investigated the LMI of pre- and post-therapy CORE-10 and -OM assessments in a naturalistic treatment register of 1715 patients’ short psychotherapies, testing whether the construct of “psychological distress” remained the same across the treatments. Results: We observed good psychometric properties and no violations of LMI for CORE-10 in chi-squared equivalence tests, nor in effect-size-based evaluations. Only the highly sensitive chi-squared difference tests detected LMI violations but these had little practical influence. The CORE-OM data did not fit well with factorial models but this was cross-sectional rather than a genuinely longitudinal (LMI-related) issue. Conclusions: CORE-10 appeared a structurally valid measure of general psychological distress and suitable for longitudinal assessment, whereas the CORE-OM had a less clear factorial structure. Regarding psychometrics, these findings support the use of CORE-10 in longitudinal assessment during psychotherapy and do not support CORE-OM.
Clinical or methodological significance of this article: Longitudinal assessment plays a key role in psychotherapy. We integrated repeated measurement into the psychometric evaluation of treatment success, showing that a simple 10-item inventory for general psychological distress (CORE-10) was a structurally valid psychometric tool despite possible logical objections based on etiologic data. The longer CORE-OM it is a part of did not enjoy a similarly simple psychometric structure and caution in its interpretation seems warranted.
Recent psychometric investigations suggest that changes in depression and anxiety over the course of psychotherapeutic treatment can be efficiently interpreted from self-report instruments (Rosenström et al., Citation2021; Stochl et al., Citation2020). That is, the scores of these instruments appear to reflect changes in the intended construct of total depression or anxiety, rather than shifts in patients’ response style and interpretation of specific questions. Researchers have also claimed to have produced self-report instruments for the common presentation of psychological distress that are “generic, short, and easy-to-use” in psychological therapies (Barkham et al., Citation2013). Here, we take a further look at the feasibility of such an aim by investigating the CORE-10 inventory aiming at it. For comprehensiveness, we also study the properties of Clinical Outcomes in Routine Evaluation-Outcome Measure (CORE-OM) inventory of which CORE-10 is a sub-part (Barkham et al., Citation1998, Citation2013; Evans et al., Citation2002; Zeldovich & Alexandrowicz, Citation2019).
Individual psychiatric disorders, such as depression, have been placed on a hierarchy of etiologic factors in the recent empirical work on psychiatric nosology (for reviews Kotov et al., Citation2021; Lahey et al., Citation2021; Ringwald et al., Citation2021). This means that the individual disorders reflect both their own etiologic factors and cross-disorder etiologic factors. Changes in diagnostic status, as well as normative individual differences, appear to reflect a similar hierarchy (Gluschkoff et al., Citation2019; Oltmanns et al., Citation2018; Rosenström et al., Citation2019). This hierarchical model suggests that, for example, depressive symptoms reflect both depression-specific etiologic influences and general etiologic influences that affect multiple disorders. Hence, we can use a sum of depressive symptoms to track changes in the depressive disorder, despite our inability to unambiguously attribute the changes to depression-specific versus general etiologic sources. In contrast, it is not clear whether such a hierarchical model allows one to track changes in a higher-order construct of general “psychological distress” with a simple sum of questionnaire items, as we next explain.
Hierarchical models of psychopathology tend to contain a general psychopathology factor—a “p factor” (Caspi et al., Citation2014; Caspi & Moffitt, Citation2018). This p factor is a latent construct for capturing comorbidity. Any psychiatric symptom one might inquire about in a questionnaire is typically considered to reflect both the p factor and etiologic influences specific to a disorder. That is, a change in any sum of symptoms will inevitably confound changes in the p factor with disorder-specific changes. Thus, the idea of a simple measure of generic psychological distress appears incompatible with the hierarchical model of psychopathology. It also appears incompatible with the phase model of behavior change that suggests the existence of three distinct constructs that change asynchronously during psychotherapy (Howard et al., Citation1993). In a longitudinal assessment, the phase model predicts that items pertaining well-being (remoralization), symptomatic distress (remediation), and life functioning (rehabilitation) diverge from a single construct even if they would not seem distinct based on cross-sectional individual differences.
Nevertheless, CORE-OM is a widely used self-report questionnaire for evaluating the generic efficacy of psychotherapy and other mental-health interventions (Barkham et al., Citation1998; Evans et al., Citation2002; Zeldovich & Alexandrowicz, Citation2019). It aims to assess psychological distress by tapping into core components of presenting problems across the widest range of psychotherapy clients. However, the full multidimensional factorial structure of the CORE-OM instrument generally has not aligned with its original four measurement domains (well-being, problems, functioning, and risks) and has remained elusive in psychometric investigations (Lyne et al., Citation2006; Murray et al., Citation2020; Skre et al., Citation2013; Zeldovich & Alexandrowicz, Citation2019). The internal structure of the measure also appears to change during psychotherapy (Murray et al., Citation2020). Because good cross-sectional psychometric properties are a prerequisite to good longitudinal psychometric properties, and because there already is evidence on longitudinal issues for CORE-OM, the longitudinal psychometric investigation of CORE-10 was our primary objective and that of CORE-OM a secondary interest for the sake of comprehensiveness. An abbreviated version, CORE-10, is strongly correlated with the CORE-OM total score (at 0.94) and has a high internal consistency (alpha of 0.90), and by definition, a lower dimensionality (less factors) compared to CORE-OM (Barkham et al., Citation2013). As the stated “purpose of the CORE-10 is to be used as a single score” (Barkham et al., Citation2013), we considered it well-suited to the psychometric investigation of feasibility of the “generic, short, and easy-to-use” assessment of psychological distress.
Barkham and colleagues (Barkham et al., Citation1998, Citation2013; Evans et al., Citation2002) may not have claimed existence of a latent construct of distress, but neither did they explicitly express building a formative construct (Diamantopoulos et al., Citation2008). Research on the CORE-10 and -OM measures have typically reported high internal-consistence (alpha) coefficients to claim good psychometric properties, which suggests they identify distress with a latent (reflective) construct. This is because high alpha values indicate highly correlated items which, while favored in reflective measure construction, are dropped out in formative measure construction due to near-multicollinearity issues (Diamantopoulos et al., Citation2008). Thus, we ask here does the CORE-10 sum score assess change in latent distress during psychotherapy or is it confounded with other systematic changes not attributable to its purpose of providing a “single score.” Specifically, we ask does CORE-10 have the property of being longitudinally measurement invariant (LMI) over the course of psychotherapy (Liu et al., Citation2017; Murray et al., Citation2020)? In other words, does it measure the same construct of distress before and after the treatment? And, if not, how might the construct change and what might explain the change? For comprehensiveness and to provide a point of comparison for the reader, we also ask analogous questions for the CORE-OM inventory.
Based on the past research and the above discussion on the hierarchical models of etiology, we expected CORE-10 to show good psychometric properties in a cross-sectional evaluation, but not longitudinally, because a simple sum score cannot tease apart general distress from disorder-specific changes. We expected to find violations of LMI and planned to study their associations with more specific problem presentations, such as depression or anxiety. Based on our representative data, we hoped to reveal and interpret LMI violations that inform and encourage new research. Finally, we discuss the measurement of general distress, specific symptoms, and functioning in psychotherapy context.
Methods
Participants
HUS Helsinki University Hospital in Finland recently introduced a psychotherapy quality registry, which records pre- and post-therapy CORE-OM. All patients referred to psychotherapy are entered into the register. Questionnaires are recorded at the beginning and at the end of the treatment. Use of the register is an obligatory part of the treatment process for the private therapists producing outsourced psychotherapies for the hospital district. At the beginning of the therapy, patients get a personal link and a unique pin-code to the questionnaires, which can be filled in using a smartphone, tablet or computer. Alternatively, the patient questionnaires can be filled in with help from the therapist. Therapists are mainly independent registered and licensed practitioners who get a coupon-based reimbursement upon therapy completion. The registry contains several hundred therapists, representing all outsourced psychotherapy assignments from HUS, a hospital district serving a population of 1.7 million. The therapists can represent most of the nationally accepted psychotherapeutic frameworks, but commonly represented solution-focused therapy (25% in our present sample), cognitive therapy (24%), cognitive behavioral therapy (13%), integrative therapy (9%), and psychodynamic therapy (11%). A great majority of patients (92%) had a depressive or anxiety disorder as a primary diagnosis, with a minority (8%) having physiology-related, psychotic, bipolar, or other disorders. A separate paper by Saarni et al. has been submitted on the Finnish psychotherapy quality register, its rationale, development, and baseline results.Footnote1
In this study, we included adult patients who had been in short psychotherapy (typically up to 20 reimbursed sessions) and had both pre- and post-therapy questionnaire data. The patients were referred from both specialized and primary healthcare services. Our sample from these data contained overall 1715 patients, registered during three first years of the registry operation (starting 12 June 2018 and ending 29 August 2021). Our research access to the pseudonymized data was based on a research permission, a permission from the ethical review board of HUS, and national regulations on the secondary use of healthcare registers.
Measurements and Procedures
CORE-OM contains 34 items, of which a subset of 10 has been selected for the shorter CORE-10 inventory, which we used (Barkham et al., Citation1998, Citation2013; Evans et al., Citation2002; Zeldovich & Alexandrowicz, Citation2019). We used the Finnish versions of the inventories (Honkalampi et al., Citation2017; Juntunen et al., Citation2015). The clinical score for CORE-10 is simply the sum of the 10 items, each getting a value of 0, 1, 2, 3, or 4, whereas the clinical score for CORE-OM is the average of all 34 items multiplied by 10. Thus, both the clinical scales range from 0 to 40 points and are just re-scaled sum scores.
Statistics
We verified that one factor sufficed to capture item correlations of CORE-10 using a parallel analysis test for factor number, applied to polychoric correlation matrices (Garrido et al., Citation2013, Citation2016). The parallel analysis compares relative variance captured by real-data principal components (i.e., eigenvalues) to that of similarly sized simulated null-correlation data. As chance variation creates some correlations (eigenvalues > 1) in the 1000 simulated datasets, the true number of factors can be estimated by the average number of real-data eigenvalues exceeding their simulated-data equivalents. We used parallel analysis, because approaches based on fit indices and tests may be inappropriate for factor-number estimation (Garrido et al., Citation2016; Hayashi et al., Citation2007), despite their widespread use (e.g., Zeldovich & Alexandrowicz, Citation2019). In the case of more than one factor (for CORE-OM), we used (orthogonal) exploratory bifactor rotation to identify the loadings structure in the baseline data prior to applying the LMI models to the full data (Jennrich & Bentler, Citation2011). We used R package GPArotation version 2014.11-1, freeing exploratory loadings > 0.3 in confirmatory analyses (i.e., items with at least ∼10% of variance linked to a factor).
LMI was tested using a series of confirmatory factor analysis models (Liu et al., Citation2017). We used the lavaan R package, version 0.6-7, with the “WLSMVS” estimator, which is a diagonally weighted least squares estimator with mean and variance adjusted test statistics. We report robust versions of the popular root mean squared error of approximation (RMSEA) and comparative fit index (CFI) too. RMSEA values ≤ 0.06 and CFI values ≥ 0.95 indicate a relatively good fit, although caution is needed in their interpretation under ordinal-valued data. To facilitate the interpretation of effect sizes, we diverted from Liu et al. (Citation2017) model specification by fixing time 1 latent factor variances to 1 instead of using anchor factor loadings (Murray et al., Citation2020; Rosenström et al., Citation2021). The pre-therapy item liabilities were also fixed to unit variance and zero mean for model identification. We used all pairwise complete observations when computing polychoric correlation matrices for structural equation modeling, as missing data imputation remains understudied in this context (Arndt et al., Citation2020; Rosenström et al., Citation2021).
illustrates the series of nested models typical to LMI testing. First, configural (or “weak”) invariance is evaluated by assessing whether the same factor loading structure applies in time 1 and time 2 (pre- and post-therapy), explaining the cross-lagged item-item covariances in addition to cross-sectional ones. In practice, configural invariance is established if the model fits data. Configural invariance ensures that changes in the CORE-OM responses align with the cross-sectionally established factorial structure (e.g., loadings structure or dimension does not change over time). However, it does not ensure that the factor loadings, the relation between item liabilities and the latent factor, stay invariant across time. Second, loading invariance is established if a model that fixes time 1 and time 2 factor loadings equal does not significantly differ in fit from the configural invariance model. However, the relation of the ordinal item categories to the underlying factor and item-endorsement liabilities could still change in time despite the loading invariance. Third, threshold invariance is established if the ordinal item thresholds can be fixed equal across time without deteriorating fit with respect to the loading-invariance model. However, this only fixes thresholds relative to each other but not their overall scale. Finally, if fixing all unique variances to unit in a unique factor invariance model does not significantly reduce the model fit, the scales of the thresholds are considered time-invariant (for discussion on meanings of thresholds, see Liu et al., Citation2017; Rosenström et al., Citation2021; Verhulst & Neale, Citation2021). Only then, the changes in average ordinal responses are attributable to changes in the latent constructs or changes unique to pertinent items, and we can conclude that there is no evidence for an LMI violation at any level of our conceptual measurement model. The intended measurement construct stays conceptually immutable over time, with people changing their levels of the construct.
Figure 1. A concept-illustrating joint sketch of path-diagrams for longitudinal measurement invariance assessment in a unidimensional factor analysis model (see Liu et al., Citation2017 and Rosenström et al., Citation2021 for accurate details). Transparent “⌀” symbols over red and dashed elements highlight possible features indicating lack of invariance. Configural invariance is assessed from model fit and requires that the same factor model fits in times 1 and 2 and that cross-time cross-item correlations reflect only the intended factor structure (e.g., time 2 extra factors or cross-time correlations between unique variance of different items are forbidden). Loading invariance is assessed by testing an additional constraint that time 1 and time 2 factor loadings (λ values) are equal to each other (i.e., items measure the latent factor equally well in all times). Threshold invariance is tested by further constraining ordinal item-response category thresholds to equal across time (i.e., the items behave similarly in relation to some liability continuum behind the ordered self-report, or Likert, categories). Unique factor invariance is tested by further constraining time 2 unique item variances to 1 (as in time 1), meaning that means, variances, and within-time covariances of latent continuous responses are entirely attributable to changes in the latent common factor over time.
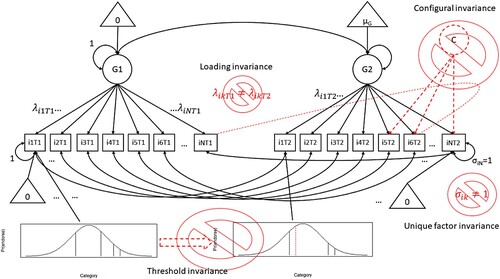
The above LMI model series can be tested in several ways and the state-of-art is under rapid evolution. Therefore, we conservatively interpreted three different forms of evidence for LMI. First, a CFI decrease greater than 0.01 may indicate a lack of measurement invariance. We examined this frequently used index (Cheung & Rensvold, Citation2002; de Jonge et al., Citation2018; Gluschkoff et al., Citation2019). Second, we also interpreted chi-squared difference tests between successive models, as recommended (Liu et al., Citation2017). This approach may be oversensitive in big data, however, because it assesses deviations from perfect fit to data. Therefore, and thirdly, we also conducted chi-squared “equivalence tests” to see if we could reject a hypothesis that a fit is poor. This provides more direct support for the alternative hypothesis of acceptable fit than is available from the traditional null hypothesis testing (Counsell et al., Citation2020; Yuan et al., Citation2016; Yuan & Chan, Citation2016). We used a population RMSEA of 0.08 together with the previously published equiv_chi R function (Counsell et al., Citation2020). For sample size, we investigated both full and listwise-deleted sample sizes to get p-values associated with upper and lower bounds of effective sample size, respectively. We considered evidence against LMI solid if all the three assessment strategies detected a violation of LMI. As an additional probe into the practical significance of potential LMI violations, we compared model-predicted item category endorsement frequencies between the violated LMI model and the last non-violated model in the sequence (Liu et al., Citation2017).
Results
Overall, the data included 1715 psychotherapy patients of which 379 were men (22.1%), and 943 were patients coming to treatment with a referral from primary care setting (54.99%; compared to special care). The median time the patients took to complete the therapy was 195 days, or 6.5 months (212 days on average; s.d. 68 days). As most patients had a coupon for a certain number of sessions, the therapists had not always reported the actual number of sessions, but it was 14 on average amongst the patients with a positive entry (median 18, s.d. 7, range 1–40). All the patients had full baseline data on CORE-10 and -OM, whereas 1521 had also full post-therapy follow-up data (88.69%). Those who lacked the post-therapy data did not differ from others in terms of gender (26% men vs. 22%; χ2 = 1.989, d.f. = 1, p = 0.158), average age (39.4 vs. 40.2 years; t = −0.739, p = 0.460), and most common primary diagnosis (11.1% with unspecified anxiety disorder, F41.9, vs. 11.1%; χ2 = 0.000, d.f. = 1, p ≈ 1), but they did have 1.36 points higher baseline clinical score in CORE-10 (t = 2.566, p = 0.011) and 1.29 points higher CORE-OM score (t = 2.771, p = 0.006). Only 41 patients had more than one treatment course, and we used only the data from the first short treatment course in such cases. All the pairwise complete observations (i.e., all 1715 patients) contributed to the below LMI analyses.
The average pre- and post-therapy clinical scores (s.d.) were 15.9 (6.6) and 11.7 (7.0) for CORE-10, and 14.7 (5.7) and 11.0 (6.1) for CORE-OM. Hedge’s g effect sizes were −0.62 for CORE-10 and −0.63 for CORE-OM, whereas the paired Cohen’s d effects were −0.62 for CORE-10 and −0.65 for CORE-OM (Goulet-Pelletier & Cousineau, Citation2018 for definitions). The supplementary online material provides basic data on the item level.
Factor Number (Dimensionality) for CORE-10 and -OM
In the baseline, CORE-10 was clearly unidimensional according to parallel analysis (Supplementary Figure S1) and, consistent with other studies, it had a good internal consistency (α = 0.84, with 95% bootstrap CI of 0.82–0.85). We detected 5 factors, or dimensions of covariation, for the full 34 items in CORE-OM but neither 5- nor 1-factor LMI models converged in this full set of items. Items #6 (“I have been physically violent to others”) and #22 (“I have threatened or intimidated another person”) had very few endorsements in categories like “often” or “all or most the time” referring to the last week. Therefore, we combined them as in Murray et al. (Citation2020) to better estimate correlations in categorical data. Consequently, the factor number was reduced to four and convergence was achieved. We discuss the four-factor solution in these item-combined data below, whereas the supplementary online material covers the parallel analysis tests and a five-factor exploratory bifactor analysis of the original data.
Longitudinal Measurement Invariance (LMI) of CORE-10
The unidimensional measurement model fitted well with the CORE-10 data overall (). When evaluating effect-size (CFI criterion) or chi-squared equivalence (acceptable-fit) tests, we observed no violations of LMI (). We detected threshold and unique-factor LMI violations using chi-squared difference (perfect-fit) tests, but this is a rather sensitive test in large samples (see Methods). In practice, the maximum absolute difference between item-category endorsement probabilities predicted by loading vs. threshold LMI models was 0.036, which is less than 0.05 that Liu et al. (Citation2017) used as a threshold for practical significance. The supplementary online materials provide full parameter estimates on the final (unique-factor) LMI model.
Table I. Longitudinal measurement invariance model fit and tests for CORE-10 and CORE-OM inventories.
LMI of CORE-OM
For comprehensiveness, we also assessed LMI for the 34-item CORE-OM inventory. As per our analytic strategy (see Methods), we first investigated an exploratory bifactor-rotated factor solution in the baseline (pre-therapy) data to define the meaningful factors (). Then, we tested whether we could confirm LMI for the indicated factor structure (, lower 4 rows). Effect-size and equivalence tests detected no LMI violation, but the overall fit of the model was rather bad (RMSEA > 0.5 and CFI < 0.95 throughout; chi-squared difference tests detected less than perfect LMI in all cases). Since, in principle, the configural LMI model should have a good fit to data for one to continue assessing the full LMI, the finding on low overall fit warranted further attention. Is it a genuinely longitudinal issue to begin with?
Table II. Factor loadings from an exploratory bifactor analysis on pre-therapy data.
Observations on Cross-Sectional Factor Structure of CORE-OM
Similar to the configural LMI model above (RMSEA = 0.066 and CFI = 0.872), a confirmatory factor model for CORE-OM items at baseline only also had a low fit to data (RMSEA = 0.092 and CFI = 0.815). We verified that this is not an issue related to confirmatory constraints by computing fit-index values directly from the polychoric correlation matrix and a four-factor exploratory factor analysis solution on it (RMSEA = 0.084, CFI = 0.868). Using a five-factor solution made only a little difference (RMSEA = 0.080, CFI = 0.888), as could be anticipated from the parallel analysis plots (supplementary online file). The full CORE-OM item set simply did not fit very well to a factor model of any kind. But, could the CORE-OM bifactor solution nevertheless suggest why the subset of items belonging to the CORE-10 yielded such a fitting factor model? We thought so (see Discussion).
Discussion
Unlike hypothesized, our study revealed good cross-sectional and longitudinal psychometric properties for CORE-10. As for CORE-OM, the cross-sectional psychometric properties and longitudinal model fit were not good, although we did not detect robust violations of longitudinal measurement invariance (LMI) on top of the overall low fit. Only the sensitive chi-squared test always suggested a lack of perfect LMI but this is not necessarily a good index in large samples (Counsell et al., Citation2020; Rosenström et al., Citation2021). Thus, rather than being a genuinely longitudinal issue of response shifts, the low fit indexes for CORE-OM were observed cross-sectionally as well.
Although past studies using a highly sensitive statistical chi-squared difference tests have frequently found LMI violations in psychiatric symptom scores for depression or anxiety, more recent studies testing for acceptable fit or using effect-size-based measures have indicated that the scores fulfill the LMI requirement (Rosenström et al., Citation2021; Stochl et al., Citation2020). Here, we showed that also CORE-10 did fulfill the approximate LMI assessed in this manner while failing the perfect LMI assessed via the chi-squared difference test. In terms of psychometric structure, these findings support the use of CORE-10 sum scores in the longitudinal assessment of psychotherapy effectiveness.
While not failing tests of LMI per se, CORE-OM failed the tests of adequate model fit. This is perhaps not surprising. A comparison of CORE-OM translations revealed that none replicated the original CORE-OM structure and the fit indexes were generally below the typically recommended values (Zeldovich & Alexandrowicz, Citation2019). Lyne et al. (Citation2006) studied 2140 patients receiving psychotherapy in the UK and introduced a seven-factor bifactor model to achieve fit-index values close to usual recommendations. However, they relied on sequential comparisons of confirmatory SEMs which tends to lead to too many (artefactual) factors (Hayashi et al., Citation2007), and also used confirmatory bifactor models that are prone to overfit (Eid et al., Citation2017; Markon, Citation2019). In contrast, the exploratory bifactor rotation of has the exact same fit as the classic exploratory factor analysis model and thus does not overfit relative to it (Jennrich & Bentler, Citation2011; Rosenström et al., Citation2019).
The CORE-OM item set may not adhere to a simple factorial structure, but why CORE-10 showed LMI despite expectations to the contrary and why did it fit data so much better than CORE-OM? Looking at our bifactor solution for the full CORE-OM item set, we observed that no more than two of the items selected to CORE-10 (dagger-superscripted in ) load on a same specific factor. Most of the items of CORE-10 load only on the general factor of CORE-OM and not its specific factors. Since at least three items are required to form a factor, the CORE-10 items lie on an item-subspace where item-item covariation is attributable to the general factor, whereas the (CORE-OM) specific factors get isolated in the residuals of (CORE-10) single items. Hence, the CORE-10 items appear a convenient set to measure the general factor, as long as one keeps in mind that the unique item variance is not all error (i.e., it contains parts of specific factors among other things). Interestingly, CORE-10 items are not particularly characteristic of internalizing (e.g., depressive and anxiety disorders) or externalizing (e.g., antisocial personality and substance-use disorders) spectra of psychopathology but rather somewhere in between (unwanted image/memories, panic/terror, social-interaction difficulties, plans to end life, etc.). Amongst psychopathologies, also borderline personality traits fall between internalizing and externalizing spectra and show particularly strong association with general psychopathology factor (Eaton et al., Citation2011; Gluschkoff et al., Citation2021). Because specific internalizing and externalizing traits are residual to the general factor in a bifactor structure, pure indexes of the general factor should not be particularly characteristic of neither. As the CORE-10 items appeared to fulfill this criterion, future research could address correspondence between general psychological distress in CORE-10 and the general psychopathology factor.
A simple and efficient measure for general psychopathology could be useful in clinical practice. For example, Constantinou et al. (Citation2019) modeled general and specific psychopathology factors over a psychosocial intervention, finding that within-person levels of a general psychopathology factor and specific antisocial factor declined but those specific to anxiety increased. A typical anxiety sum score would likely have decreased in treatment because it largely reflects general pathology, being confounded with it (Caspi & Moffitt, Citation2018). Item-sum measures like CORE-10 inevitably confound anxiety and general psychopathology. However, it remains for future research to show whether they nevertheless are sufficiently saturated with general psychopathology to tease apart such findings as Constantinou et al. (Citation2019) did use structural equation models. CORE-OM, the parent inventory of CORE-10, contains multiple factors but their psychometric validity and LMI remains unclear (Lyne et al., Citation2006; Murray et al., Citation2020; Skre et al., Citation2013; Zeldovich & Alexandrowicz, Citation2019), making it less salient choice for such endeavors than CORE-10.
It seems plausible that both general psychopathology and at least some specific disorders could be assessed with a hierarchy of factor models that fulfill LMI (Gluschkoff et al., Citation2019; Rosenström et al., Citation2021; Stochl et al., Citation2020). Perhaps future measures of psychotherapeutic core outcomes will be built around hierarchical models of etiology (Hopwood et al., Citation2020). From a purely statistical perspective, our study suggests that the CORE-10 sum score fulfills LMI and might play a role in such measures. Non-confounded measurements on various general and specific effects eventually might take us a long way towards understanding specific effects of psychotherapy, which currently remain poorly understood (Cuijpers, Cristea, et al., Citation2019; Cuijpers, Reijnders, et al., Citation2019). Teasing apart general and specific mechanisms that influence psychotherapy outcomes has been notoriously difficult (Cuijpers, Reijnders, et al., Citation2019; Wampold & Imel, Citation2015).
A strength of the present study compared to some previous ones (e.g., Murray et al., Citation2020) was our ability to investigate practical consequences and correlates for violations of longitudinal measurement invariance, or “response shifts.” Other significant strengths of this study were our comprehensive registry of naturally occurring psychotherapies and comprehensive set of (three different) criteria to detect LMI violations. Limitations include potentially non-benign latent normality assumptions in the LMI models and their inability to exhaust all forms of unintended response shifts (see Rosenström et al., Citation2021). Furthermore, generalizability of the results is limited by possible differences between different language versions of the CORE-OM that might remain despite the extensive work to accurately translate the English version to Finnish to mitigate such sources of bias (Juntunen et al., Citation2015). One practical implication of the study was that the CORE-10 item set offers a short core outcomes self-report inventory that is psychometrically satisfactory when measuring the change in distress under psychotherapy. Nevertheless, it may be debatable whether CORE-10 offers adequate content coverage for a wide-band construct like “general psychological distress.” The CORE-OM that has a wider content coverage did not enjoy similarly satisfactory psychometric properties. In what pertains to the psychometric structure only, however, these findings support the use of CORE-10 sum scores in the longitudinal assessment of psychotherapy effectiveness and do not support CORE-OM. Besides psychometrics, other arguments for and against their use exist but are outside the scope here.
Supplemental Material
Download ()Supplemental data
Supplemental data for this article can be accessed at https://doi.org/10.1080/10503307.2022.2074807.
Additional information
Funding
Notes
1 Local online pages also provide more information: https://www.mielenterveystalo.fi/aikuiset/itsehoito-ja-oppaat/oppaat/hus-ostopalvelupsykoterapia/Pages/psykoterapian-laaturekisteri.aspx.
References
- Arndt, A., Lutz, W., Rubel, J., Berger, T., Meyer, B., Schröder, J., Späth, C., Hautzinger, M., Fuhr, K., Rose, M., Hohagen, F., Klein, J. P., & Moritz, S. (2020). Identifying change-dropout patterns during an internet-based intervention for depression by applying the Muthen-Roy model. Cognitive Behaviour Therapy, 49(1), 22–40. https://doi.org/10.1080/16506073.2018.1556331
- Barkham, M., Bewick, B., Mullin, T., Gilbody, S., Connell, J., Cahill, J., Mellor-Clark, J., Richards, D., Unsworth, G., & Evans, C. (2013). The CORE-10: A short measure of psychological distress for routine use in the psychological therapies. Counselling and Psychotherapy Research, 13(1), 3–13. https://doi.org/10.1080/14733145.2012.729069
- Barkham, M. C., Evans, C., Margison, F., McGrath, G., Mellor-Clark, J., Milne, D., & Connell, J. (1998). The rationale for developing and outcome batteries for routine use in service settings and psychotherapy outcome research implementing core. Journal of Mental Health, 7(1), 35–47. https://doi.org/10.1080/09638239818328
- Caspi, A., Houts, R. M., Belsky, D. W., Goldman-Mellor, S. J., Harrington, H., Israel, S., Meier, M. H., Ramrakha, S., Shalev, I., Poulton, R., & Moffitt, T. E. (2014). The p factor: One general psychopathology factor in the structure of psychiatric disorders? Clinical Psychological Science, 2(2), 119–137. https://doi.org/10.1177/2167702613497473
- Caspi, A., & Moffitt, T. E. (2018). All for one and one for all: Mental disorders in one dimension. The American Journal of Psychiatry, 175(9), 831–844. https://doi.org/10.1176/appi.ajp.2018.17121383
- Cheung, G. W., & Rensvold, R. B. (2002). Evaluating goodness-of-fit indexes for testing measurement invariance. Structural Equation Modeling: A Multidisciplinary Journal, 9(2), 233–255. https://doi.org/10.1207/S15328007SEM0902_5
- Constantinou, M. P., Goodyer, I. M., Eisler, I., Butler, S., Kraam, A., Scott, S., Pilling, S., Simes, E., Ellison, R., Allison, E., & Fonagy, P. (2019). Changes in general and specific psychopathology factors over a psychosocial intervention. Journal of the American Academy of Child and Adolescent Psychiatry, 58(8), 776–786. https://doi.org/10.1016/j.jaac.2018.11.011
- Counsell, A., Cribbie, R. A., & Flora, D. B. (2020). Evaluating equivalence testing methods for measurement invariance. Multivariate Behavioral Research, 55(2), 312–328. https://doi.org/10.1080/00273171.2019.1633617
- Cuijpers, P., Cristea, I. A., Karyotaki, E., Reijnders, M., & Hollon, S. D. (2019). Component studies of psychological treatments of adult depression: A systematic review and meta-analysis. Psychotherapy Research, 29(1), 15–29. https://doi.org/10.1080/10503307.2017.1395922
- Cuijpers, P., Reijnders, M., & Huibers, M. J. H. (2019). The role of common factors in psychotherapy outcomes. Annual Review of Clinical Psychology, 15(1), 207–231. https://doi.org/10.1146/annurev-clinpsy-050718-095424
- de Jonge, P., Wardenaar, K. J., Lim, C. C. W., Aguilar-Gaxiola, S., Alonso, J., Andrade, L. H., Bunting, B., Chatterji, S., Ciutan, M., Gureje, O., Karam, E. G., Lee, S., Medina-Mora, M. E., Moskalewicz, J., Navarro-Mateu, F., Pennell, B.-E., Piazza, M., Posada-Villa, J., Torres, Y., … Scott, K. (2018). The cross-national structure of mental disorders: Results from the world mental health surveys. Psychological Medicine, 48(12), 2073–2084. https://doi.org/10.1017/S0033291717003610
- Diamantopoulos, A., Riefler, P., & Roth, K. P. (2008). Advancing formative measurement models. Journal of Business Research, 61(12), 1203–1218. https://doi.org/10.1016/j.jbusres.2008.01.009
- Eaton, N. R., Krueger, R. F., Keyes, K. M., Skodol, A. E., Markon, K. E., Grant, B. F., & Hasin, D. S. (2011). Borderline personality disorder co-morbidity: Relationship to the internalizing–externalizing structure of common mental disorders. Psychological Medicine, 41(5), 1041–1050. https://doi.org/10.1017/S0033291710001662
- Eid, M., Geiser, C., Koch, T., & Heene, M. (2017). Anomalous results in G-factor models: Explanations and alternatives. Psychological Methods, 22(3), 541–562. https://doi.org/10.1037/met0000083
- Evans, C., Connell, J., Barkham, M., Margison, F., McGrath, G., Mellor-Clark, J., & Audin, K. (2002). Towards a standardised brief outcome measure: Psychometric properties and utility of the CORE-OM. The British Journal of Psychiatry, 180(1), 51–60. https://doi.org/10.1192/bjp.180.1.51
- Garrido, L. E., Abad, F. J., & Ponsoda, V. (2013). A new look at Horn’s parallel analysis with ordinal variables. Psychological Methods, 18(4), 454–474. https://doi.org/10.1037/a0030005
- Garrido, L. E., Abad, F. J., & Ponsoda, V. (2016). Are fit indices really fit to estimate the number of factors with categorical variables? Some cautionary findings via Monte Carlo simulation. Psychological Methods, 21(1), 93–111. https://doi.org/10.1037/met0000064
- Gluschkoff, K., Jokela, M., & Rosenström, T. (2019). The general psychopathology factor: Structural stability and generalizability to within-individual changes. Frontiers in Psychiatry, 10, 594. https://doi.org/10.3389/fpsyt.2019.00594
- Gluschkoff, K., Jokela, M., & Rosenström, T. (2021). The general psychopathology factor and borderline personality disorder: Evidence for significant overlap from two nationally representative surveys of US adults. Personality Disorders: Theory, Research, and Treatment, 12(1), 86–92. https://doi.org/10.1037/per0000405
- Goulet-Pelletier, J.-C., & Cousineau, D. (2018). A review of effect sizes and their confidence intervals, part I: The Cohen’s d family. The Quantitative Methods for Psychology, 14(4), 242–265. https://doi.org/10.20982/tqmp.14.4.p242
- Hayashi, K., Bentler, P. M., & Yuan, K.-H. (2007). On the likelihood ratio test for the number of factors in exploratory factor analysis. Structural Equation Modeling: A Multidisciplinary Journal, 14(3), 505–526. https://doi.org/10.1080/10705510701301891
- Honkalampi, K., Laitila, A., Juntunen, H., Lehmus, K., Piiparinen, A., Törmänen, I., Inkinen, M., & Evans, C. (2017). The Finnish clinical outcome in routine evaluation outcome measure: Psychometric exploration in clinical and non-clinical samples. Nordic Journal of Psychiatry, 71(8), 589–597. https://doi.org/10.1080/08039488.2017.1365378
- Hopwood, C. J., Bagby, R. M., Gralnick, T., Ro, E., Ruggero, C., Mullins-Sweatt, S., Kotov, R., Bach, B., Cicero, D. C., Krueger, R. F., Patrick, C. J., Chmielewski, M., DeYoung, C. G., Docherty, A. R., Eaton, N. R., Forbush, K. T., Ivanova, M. Y., Latzman, R. D., Pincus, A. L., … Zimmermann, J. (2020). Integrating psychotherapy with the hierarchical taxonomy of psychopathology (HiTOP). Journal of Psychotherapy Integration, 30(4), 477–497. https://doi.org/10.1037/int0000156
- Howard, K. I., Lueger, R. J., Maling, M. S., & Martinovich, Z. (1993). A phase model of psychotherapy outcome: Causal mediation of change. Journal of Consulting and Clinical Psychology, 61(4), 678–685. https://doi.org/10.1037/0022-006X.61.4.678
- Jennrich, R. I., & Bentler, P. M. (2011). Exploratory bi-factor analysis. Psychometrika, 76(4), 537–549. https://doi.org/10.1007/s11336-011-9218-4
- Juntunen, H., Piiparinen, A., Honkalampi, K., Inkinen, M., & Laitila, A. (2015). CORE-OM-mittarin suomalainen validointitutkimus yleisväestössä [The Finnish validation study of the CORE-OM measure: Non-clinical sample]. Psykologia, 50(4), 257–276. https://urn.fi/URN:NBN:fi:ELE-1768688
- Kotov, R., Krueger, R. F., Watson, D., Cicero, D. C., Conway, C. C., DeYoung, C. G., Eaton, N. R., Forbes, M. K., Hallquist, M. N., Latzman, R. D., Mullins-Sweatt, S. N., Ruggero, C. J., Simms, L. J., Waldman, I. D., Waszczuk, M. A., & Wright, A. G. C. (2021). The hierarchical taxonomy of psychopathology (HiTOP): A quantitative nosology based on consensus of evidence. Annual Review of Clinical Psychology, 17(1), 83–108. https://doi.org/10.1146/annurev-clinpsy-081219-093304
- Lahey, B. B., Moore, T. M., Kaczkurkin, A. N., & Zald, D. H. (2021). Hierarchical models of psychopathology: Empirical support, implications, and remaining issues. World Psychiatry, 20(1), 57–63. https://doi.org/10.1002/wps.20824
- Liu, Y., Millsap, R. E., West, S. G., Tein, J.-Y., Tanaka, R., & Grimm, K. J. (2017). Testing measurement invariance in longitudinal data with ordered-categorical measures. Psychological Methods, 22(3), 486–506. https://doi.org/10.1037/met0000075
- Lyne, K. J., Barrett, P., Evans, C., & Barkham, M. (2006). Dimensions of variation on the CORE-OM. The British Journal of Clinical Psychology, 45(Pt 2), 185–203. https://doi.org/10.1348/014466505X39106
- Markon, K. E. (2019). Bifactor and hierarchical models: Specification, inference, and interpretation. Annual Review of Clinical Psychology, 15(1), 51–69. https://doi.org/10.1146/annurev-clinpsy-050718-095522
- Murray, A. L., McKenzie, K., Murray, K., & Richelieu, M. (2020). Examining response shifts in the clinical outcomes in routine evaluation-outcome measure (CORE-OM). British Journal of Guidance & Counselling, 48(2), 276–288. https://doi.org/10.1080/03069885.2018.1483007
- Oltmanns, J. R., Smith, G. T., Oltmanns, T. F., & Widiger, T. A. (2018). General factors of psychopathology, personality, and personality disorder: Across domain comparisons. Clinical Psychological Science, 6(4), 581–589. https://doi.org/10.1177/2167702617750150
- Ringwald, W. R., Forbes, M. K., & Wright, A. G. C. (2021). Meta-analysis of structural evidence for the hierarchical taxonomy of psychopathology (HiTOP) model. Psychological Medicine, 1–14. https://doi.org/10.1017/S0033291721001902
- Rosenström, T., Gjerde, L. C., Krueger, R. F., Aggen, S. H., Czajkowski, N. O., Gillespie, N. A., Kendler, K. S., Reichborn-Kjennerud, T., Torvik, F. A., & Ystrom, E. (2019). Joint factorial structure of psychopathology and personality. Psychological Medicine, 49(13), 2158–2167. https://doi.org/10.1017/S0033291718002982
- Rosenström, T., Ritola, V., Saarni, S., Joffe, G., & Stenberg, J.-H. (2021). Measurement invariant but non-normal treatment responses in guided internet psychotherapies for depressive and generalized anxiety disorders. Assessment, https://doi.org/10.1177/10731911211062500
- Skre, I., Friborg, O., Elgarøy, S., Evans, C., Myklebust, L. H., Lillevoll, K., Sørgaard, K., & Hansen, V. (2013). The factor structure and psychometric properties of the clinical outcomes in routine evaluation-outcome measure (CORE-OM) in Norwegian clinical and non-clinical samples. BMC Psychiatry, 13(1), 99. https://doi.org/10.1186/1471-244X-13-99
- Stochl, J., Fried, E. I., Fritz, J., Croudace, T. J., Russo, D. A., Knight, C., Jones, P. B., & Perez, J. (2020). On dimensionality, measurement invariance, and suitability of sum scores for the PHQ-9 and the GAD-7. Assessment, 29(3), 355–366. https://doi.org/10.1177/1073191120976863
- Verhulst, B., & Neale, M. C. (2021). Best practices for binary and ordinal data analyses. Behavior Genetics, 51(3), 204–214. https://doi.org/10.1007/s10519-020-10031-x
- Wampold, B. E., & Imel, Z. E. (2015). The great psychotherapy debate: The evidence for what makes psychotherapy work (2nd ed.). Routledge.
- Yuan, K.-H., & Chan, W. (2016). Measurement invariance via multigroup SEM: Issues and solutions with chi-square-difference tests. Psychological Methods, 21(3), 405–426. https://doi.org/10.1037/met0000080
- Yuan, K.-H., Chan, W., Marcoulides, G. A., & Bentler, P. M. (2016). Assessing structural equation models by equivalence testing with adjusted fit indexes. Structural Equation Modeling: A Multidisciplinary Journal, 23(3), 319–330. https://doi.org/10.1080/10705511.2015.1065414
- Zeldovich, M., & Alexandrowicz, R. W. (2019). Comparing outcomes: The clinical outcome in routine evaluation from an international point of view. International Journal of Methods in Psychiatric Research, 28(3), e1774. https://doi.org/10.1002/mpr.1774