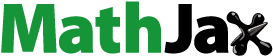
Abstract
Objective: Aspects of our emotional state are constantly being broadcast via our facial expressions. Psychotherapeutic theories highlight the importance of emotional dynamics between patients and therapists for an effective therapeutic relationship. Two emotional dynamics suggested by the literature are emotional reactivity (i.e., when one person is reacting to the other) and emotional stability (i.e., when a person has a tendency to remain in a given emotional state). Yet, little is known empirically about the association between these dynamics and the therapeutic alliance. This study investigates the association between the therapeutic alliance and the emotional dynamics of reactivity and stability, as manifested in the facial expressions of patients and therapists within the session. Methods: Ninety-four patients with major depressive disorder underwent short-term treatment for depression (N = 1256 sessions). Results: Both therapist reactivity and stability were associated with the alliance, across all time spans. Patient reactivity was associated with the alliance only in a short time span (1 s). Conclusions: These findings may potentially guide therapists in the field to attenuate not only their emotional reaction to their patients, but also their own unique presence in the therapy room.
Clinical or methodological significance of this article: Our facial behavior is constantly broadcasting our internal emotional state to the outside world. For the therapeutic relationship, facial behavior is a central channel of communication, yet research on this subject is lacking. This study is a proof-of-concept of not only the automatic assessment of facial behavior within the therapeutic session, but also of a systematic exploration of the interdependence in facial behavior between patients and therapists, and what it may indicate for the therapeutic relationship.
Introduction
Aspects of our emotional state are constantly being broadcast via our facial expressions (Ekman & Oster, Citation1979; Matsumoto et al., Citation2008). This broadcast occurs in every relationship, including the therapeutic relationship, most commonly conceptualized as the therapeutic alliance. In psychotherapy, therapist’s reactivity to their patient’s facial expressions can follow many possible dynamics (e.g., Rasting & Beutel, Citation2005): A mirroring dynamic may emerge when the therapist expresses sadness in reaction to their patient’s sadness over a recent breakup; A complementing dynamic may emerge when the therapist expresses compassion in reaction to their patient’s anger over a conversation with their parents. Therapists can also demonstrate emotional stability in response to patient distress (Ford, Citation2021). Emotional reactivity (i.e., when one person is reacting to the other) and emotional stability (i.e., when a person has a tendency to remain in a given emotional state) have already been empirically documented in the literature of interpersonal emotional dynamics (Butler, Citation2011). However, it is still an open theoretical question whether they are associated with a stronger therapeutic alliance. The therapeutic alliance refers to the collaborative relationship between patients and therapists, and encompasses the emotional bond between them, as well as the agreement between them on the goals and tasks of therapy (Bordin, Citation1979). A strong therapeutic alliance is one of the most consistent predictors of therapy success (Flückiger et al., Citation2012). Given the importance of the therapeutic alliance, exploration of this question has potential clinical significance, as therapists may be able to observe the emergence of emotional dynamics within sessions and interpret their meaning for the therapy process based on empirical data. Despite the potential benefits of this avenue of research, little is known about whether and which types of emotional dynamics are associated with the strength of the therapeutic alliance.
Much is known about emotions and emotional dynamics from the literature of emotion outside the field of psychotherapy. While disagreements abound between emotion researchers, the synthesis of the literature suggests that there are five distinct components that are involved in emotions: feeling, cognitive, motivational, somatic, and motor (Moors, Citation2009; Scherer, Citation2005). These components may manifest in the context of the therapeutic session, for example, when the patient decides to share a shameful experience (cognitive). Sharing this experience may cause them to feel anxious (feeling), and even though they want to share this information (motivational), their body may become tense (somatic), and their facial expression may show that they are distressed (motor). While the construct of facial expressions does not completely overlap with emotions (Ekman, Citation1993; Ekman & Friesen, Citation1969), facial expressions are conceptualized as a part of a single component in the process of emotion (Moors, Citation2009). In the context of psychotherapy, facial expressions can be specifically important as they encompass a central channel of communication between patients and therapists (Bänninger-Huber, Citation1992), and were shown to correlate with conscious aspects of emotional experience (Gentsch et al., Citation2015) as well as unconscious aspects (Dimberg et al., Citation2000). Psychotherapy sessions entail two (or more) people, each with their own individual emotional systems. When these emotional systems interact with one another, interpersonal emotional dynamics emerge.
Interpersonal emotional dynamics can be defined as a state in which the level of activity in one component of emotion of a person is dependent upon the level of activity in another component of emotion of another person (Butler, Citation2011; Sels et al., Citation2016). This interdependence in activity of emotion components between two people can bring about different types of interpersonal emotional dynamics. While there is an open question regarding which types of emotional dynamics exist, there is a consensus among researchers on the existence of at least two types of dynamics: emotional reactivity and emotional stability. Emotional reactivity is defined as one person’s emotional reaction to another person’s behavior (Butler, Citation2011), as can be manifested by their facial expressions. Emotional stability is defined as a tendency to remain in a given emotional state (also termed inertia; Butler, Citation2011), as can be manifested by the person’s facial expressions. There is no consensus, however, regarding the time it would take for one partner’s emotional state to influence the other partner’s emotional state (i.e., the time span of the emotional dynamic); the literature exploring emotional dynamics does not converge on a single time span. Published studies have explored emotional dynamics in time spans of anywhere between a handful of seconds (Bar-Kalifa et al., Citation2019; Bänninger-Huber, Citation1992; Frank et al., Citation1993; Schmidt et al., Citation2006) to a few minutes (Main et al., Citation2016; Rasting & Beutel, Citation2005; Tal et al., Citation2023; Tschacher & Meier, Citation2020). Moreover, studies that explored acoustic markers of emotional state throughout the conversation could not have controlled for the time span in which emotional dynamics were analyzed, as the timing of samples was dependent upon when participants chose to speak (Bryan et al., Citation2018; Reich et al., Citation2014).
In recent years, the field of psychotherapy research has witnessed a rising trend in exploring the emotional dynamics that emerge within therapeutic sessions. These studies assessed nonverbal synchrony, defined as any concurrent association (Atzil-Slonim et al., Citation2023) or shared temporal organization (Kleinbub et al., Citation2020) between patients’ and therapists’ nonverbal behavior. Conceptualized as a possible manifestation of the therapeutic relationship (Koole & Tschacher, Citation2016; Ramseyer & Tschacher, Citation2014), researchers are looking for predictors of nonverbal synchrony (e.g., McParlin et al., Citation2022) as well as its meaning to the therapeutic process and outcome (e.g., Tschacher & Meier, Citation2020). As its definition implies, synchrony describes a broader phenomenon than reactivity or stability, one which probably includes many possible types of emotional dynamics (Butler, Citation2011; for a review of this literature see Atzil-Slonim et al., Citation2023; and Kleinbub, Citation2017 for physiological synchrony specifically). Despite this currently flourishing research avenue of nonverbal behavior in psychotherapy, however, only a limited number of empirical studies have examined the association between types of emotional dynamics and the therapeutic alliance, and results have been mixed. These studies have evaluated the emotional states of patients and therapists by measuring nonverbal behavior in different modalities: physiology (Bar-Kalifa et al., Citation2019; Tschacher & Meier, Citation2020; see Tourunen et al., Citation2020 for an example of couples therapy), acoustic properties (Bryan et al., Citation2018; Reich et al., Citation2014), and facial expressions (Yokotani et al., Citation2020). While some studies found a positive association between the emergence of emotional dynamics and alliance strength (Bar-Kalifa et al., Citation2019; Bryan et al., Citation2018; Tschacher & Meier, Citation2020), another study found a negative association (Reich et al., Citation2014). One methodological factor that may have contributed to these mixed results is the disentangling of the different types of emotional dynamics that are at play during the therapeutic session. Despite the consensus regarding the existence of reactivity and stability as types of emotional dynamics in interpersonal relationships (Butler, Citation2011), to our knowledge, only a single study in psychotherapy disentangled these two emotional dynamics when testing the association with the alliance. Bryan et al. (Citation2018) analyzed acoustic interdependence within the session and explored reactivity and stability of both patients and therapists. They reported that reactivity of either side (i.e., patient or therapist), was correlated with a stronger therapeutic bond. Stability of either patient or therapist was not found to correlate with the alliance in that study.
Another methodological factor that could contribute to the mixed results regarding the association between emotional dynamics and the alliance, additionally to disentangling of emotional dynamic types, is the chosen time span in which to explore these dynamics. Researchers focusing on physiological behavior have explored emotional dynamics in different time spans, such as 10 (Bar-Kalifa et al., Citation2019), 60 (Tschacher & Meier, Citation2020), and 90 (Tal et al., Citation2023) seconds, but, as mentioned before, researchers focused on acoustic behavior were not able to keep the time span of analyzed emotional dynamics constant, as time spans were dependent upon when patients and therapists decided to talk (Bryan et al., Citation2018; Reich et al., Citation2014). Taking these methodological factors into consideration, it is preferable to design a methodology that can: (1) distinguish between the emotional dynamics of reactivity and stability, (2) and can systematically control for the time span in which these dynamics are explored. Exploring facial expressions in the therapeutic session can potentially meet these methodological requirements.
Facial expressions are a universal, reliable marker of emotional state, that covaries with subjective experience, and serves many interpersonal and social regulatory functions (Ekman & Oster, Citation1979; Matsumoto et al., Citation2008). In recent years, the number of published studies focusing on facial expressions has increased, largely due to technological advances that allow automatic assessment of facial behavior (e.g., Baltrusaitis et al., Citation2018). Despite this increase, still only a handful of studies have explored facial expressions in psychotherapy. These studies suggest that the therapeutic context is characterized by lower levels of emotional expressiveness, as compared with everyday life (Merten, Citation2003). Furthermore, it was suggested that therapists are even less emotionally expressive with their facial expressions than their patients (Merten, Citation2003, Citation2005; Merten & Brunnhuber, Citation2004; Rasting & Beutel, Citation2005). This difference may be explained by patients being generally more active in the session (Rasting & Beutel, Citation2005). The emotional dynamics between patients and therapists, as they emerge via facial expressions, are perceived as part of a dynamic and dyadic emotional pattern that can vary in its complexity, as well as in the specific emotions that are expressed (Merten, Citation2003). Empirical evidence suggests that these dynamics can emerge as similar to, as well as complementing, emotions between patients and therapists (Bänninger-Huber, Citation1992; Rasting & Beutel, Citation2005).
Published research establishes the potential of exploring facial expressions in the therapeutic session by demonstrating what emotional dynamics may emerge between patients and therapists. All published research into facial expressions in psychotherapy, however, has investigated facial expressions as they are reduced to discrete groups of basic emotions (Ekman, Citation1992; Ekman & Friesen, Citation1977). This was usually done by focusing on the most prominent expressed emotion in any given segment of the therapeutic session (also termed “leitaffect”; Merten, Citation2003), while putting aside other emotions that are relatively less intensely expressed. While there are advantages in focusing on discrete emotions, there is also ample empirical evidence and theory that supports representing emotions as placed on a continuous spectrum (Calder et al., Citation2001; Russell, Citation1980). Furthermore, this approach requires researchers to define the specific discrete emotions to test for, a priori. A possible everyday example from within a therapeutic session that emphasizes this point could be as follows: a patient expresses anger due to some disagreement with their therapist, who first reacts with surprise and then continues to empathically respond to the patient’s anger, which causes the patient to experience relief and express joy, or even tell a joke to lighten the mood, that makes the therapist laugh. Exploring discrete emotions would require testing for this exact emotional pattern (i.e., patient-joy, in reaction to therapist-surprise, in reaction to patient-anger). Another option, however, is exploring facial expressiveness, the mere intensity of facial movement exerted by patients and therapists, without assigning discrete emotional states to them. Investigating facial expressiveness would allow identification of the emotional ping-pong described in the above example, while not requiring any a priori assumptions regarding what discrete emotions will be expressed. As far as we know, only one study has explored facial expressiveness as an indicator of the therapeutic alliance. Yokotani et al. (Citation2020) investigated interviews of psychology students and tested correlation between interviewer and interviewee in the movement of specific facial muscles (e.g., left eye, right eyebrow). They found that correlation in facial movements is correlated with a stronger therapeutic alliance.
The overarching goal of the present study is to explore reactivity and stability between patients’ and therapists’ facial expressions, as indicators of therapeutic alliance strength. Consistently with literature in the field, we used the Actor-Partner Interdependence Model (APIM; Kenny et al., Citation2006) which is designed to disentangle reactivity and stability by modeling partner effects alongside actor effects in the same statistical model. We had two aims: (1) to test whether the levels of emotional reactivity and emotional stability are associated with a stronger therapeutic alliance, and (2) to systematically explore how this association varies in different time spans. Based on the literature reviewed above, our hypotheses were: (1) therapist reactivity will indicate a stronger therapeutic alliance; (2) patient reactivity will indicate a stronger alliance; (3) the more the therapist exhibits stability the stronger the therapeutic alliance will be; (4) given the mixed results in the literature, we may expect that some effects may emerge only in specific time spans. This aspect of the analysis (i.e., analysis of different time spans) was exploratory without hypothesizing which time spans will prove more relevant than others. To test these hypotheses, we analyzed facial behavior and self-report data from 1256 sessions of 94 therapeutic dyads enrolled in a randomized control trial of short-term psychodynamic psychotherapy for major depressive disorder (MDD). The focus on MDD is important, as there is substantial literature demonstrating both the phenomenon of nonverbal interdependence between therapists and patients diagnosed with MDD (e.g., Deres-Cohen et al., Citation2021) and the reduced levels of facial activity in the psychopathology of depression (e.g., Gehricke & Shapiro, Citation2000).
Method
Design
Ninety-four patients diagnosed with major depressive disorder (MDD) were recruited through advertisements offering free-of-charge psychodynamic treatment for depression. This study is part of a larger randomized control trial (RCT), which began recruitment in March 2016. The trial compared supportive and supportive expressive therapy for MDD (Zilcha-Mano et al., Citation2018, Citation2021). Patients were randomly assigned to either supportive or supportive-expressive psychodynamic therapy (Luborsky, Citation1984; Luborsky et al., Citation1995). Patients from each treatment group received 16 sessions of therapy. All participants signed an informed consent form that included an agreement for the therapy sessions to be recorded. All procedures were approved by the institutional review board of the University of Haifa, Israel (375/19).
Patients
Inclusion criteria of the RCT were (a) meeting MDD criteria, which included an MDD diagnosis according to the MINI (International Neuropsychiatric Interview; Sheehan et al., Citation1998) and scores above 14 on the 17-item Hamilton Rating Scale for Depression (HRSD; Hamilton, Citation1967) at two evaluations 1 week apart; (b) Patients who were on medication were required to remain on a stable dosage for at least 3 months prior to beginning the therapy, as well as maintain this dosage throughout therapy; (c) patients were between 18 and 60 years old; (d) Hebrew language fluency; and (e) provision of an informed consent. Exclusion criteria were (a) current risk of suicide or self-harm (HRSD suicide item higher than 2); (b) current substance abuse disorder; (c) current or past schizophrenia or psychosis, bipolar disorder, or severe eating disorder requiring medical monitoring; (d) history of organic mental disease; and (e) currently receiving psychotherapy elsewhere.
Patients in the included sample had a mean age of 31.25 years (SD = 8.69). 60.2% of patients were women. The mean of education years was 14.5 (SD = 2.1). 80.6% of patients identified as Jewish, 8.6% as Muslim, one as Christian, one as Druze, and 7.5% as other. Regarding income status, 50.5% of patients reported their monthly family income as below the average monthly income per family, 21.5% reported their income as average, 24.7% reported it was above average, and 3.3% did not report their income. Regarding employment, 65.6% of the patients were employed, 32.3% unemployed, 2.1% reported “other”. Regarding marital status, 78.5% of patients were single, 15.1% were married, 5.4% divorced or separated, 1% widowed. In addition to MDD, 74.2% of the patients were diagnosed with one or more personality disorders using the Structured Interview for DSM-IV Personality (SIDP; Pfohl et al., Citation1997). The most frequent personality disorders were avoidant (41%), obsessive-compulsive (39%), and dependent (13%). The percentage of comorbidity with anxiety disorders was 67.7%. The most frequent anxiety disorders were generalized anxiety (61%), social anxiety (29%), agoraphobia (15%). Additionally, 3% were diagnosed with obsessive compulsive disorder, and 5% were diagnosed with posttraumatic stress disorder.
Therapists and Treatment
Seven therapists participated in this study, each with at least 5 years of expertise in psychodynamic treatment. All therapists had formal experience in psychodynamic treatment. The therapists attended a 20-hour training workshop in supportive and expressive techniques prior to seeing patients. Therapists received weekly private and group supervision, provided by two experienced and licensed clinical psychologists. Supervisors were themselves under supervision provided by an international supportive-expressive therapy expert. Each therapist provided both treatment conditions to act as their own controls. The mean clinical experience of the therapists was 13.36 years (SD = 5.7), mean age was 40.3 years (SD = 6.75), and only one of the therapists was male. The mean number of patients per therapist was 13.29 (SD = 8, range = 2–25). Patients received 16 weekly 50-minute sessions of either supportive expressive treatment, or supportive treatment, both of which are time-limited psychodynamic therapies adapted for depression. Supportive expressive treatment is based on the use of expressive techniques, such as interpretation, confrontation, and clarification (Luborsky et al., Citation1995). Supportive treatment includes the use of supportive techniques aimed at alliance strengthening, such as affirmation and empathic validation (Leibovich et al., Citation2018). For more details, see the study protocol (Zilcha-Mano et al., Citation2018);
Assignment to treatment condition was conducted via an outside institution not involved in the study. Comprehensive treatment protocols for supportive expressive treatment were used (Luborsky, Citation1984; Luborsky et al., Citation1995). The supportive treatment condition included all supportive techniques detailed in the manual, but excluded any expressive techniques (for more details, see Leibovich et al., Citation2020).
Measures
Alliance
The therapeutic alliance was measured using the Working Alliance Inventory—Short Form: Patient version (WAI; Horvath & Greenberg, Citation1989). This is a 12-item self-reported inventory based on Bordin’s (Citation1979) conceptualization of the working alliance. The score is calculated as an average of all 12 items, and addresses the three components of the alliance: the emotional bond between patient and therapist, their agreement on the tasks, and their agreement on goals of treatment. The WAI was administered to the patients after each session. In the current study, mean WAI score was 5.62 (SD = 0.98) and the internal reliability range across measurement points of the WAI was 0.9–0.95.
Facial expressiveness
Facial expressions were automatically assessed from video-recorded sessions. All sessions were video-recorded with two cameras, one pointing at the patient, the other pointing at the therapist. Videos were recorded at 25 frames per second. Out of 118 patients who participated in the randomized control trial: one patient has requested for their data to be deleted. 11 patients received the therapy in other facilities at the beginning of the trial, with nonstationary, mostly lower-quality recording equipment; 13 patients received their entire therapy via remote video conversation after the outbreak of COVID-19 toward the end of the randomized control trial. In these remote therapy sessions, patients and therapists were not recorded simultaneously and thus analysis of simultaneous facial expressions was impossible. These 25 patients were excluded from the analysis. Still, not all sessions of the remaining 94 patients were analyzable, for the same two reasons: 10 patients have moved in the middle of their therapy into the main facility of the research trial, with the appropriate recording equipment; COVID-19 broke out when 14 patients were in the middle of their therapy, necessitating a sudden move to video therapy which disallowed further assessment of facial behavior. Eventually, 94 patients were included in the analyzed data, though some sessions of some patients could not be analyzed as mentioned above. The final sample had 7 patients who dropped out (7.4%). Overall, 1256 sessions were included in the analyzed data overall.
Facial expressions were measured using the OpenFace 2.0 software (Baltrusaitis et al., Citation2018) which is an open-source software applying a machine learning algorithm (convolutional neural network) aimed at identifying facial expressions. Facial expression analysis of OpenFace is based on the Facial Action Coding System (FACS; Ekman & Friesen, Citation1977). The automatic coding of OpenFace results in estimations of activation in single muscle innervations, termed “action units”. Essentially, OpenFace analyzes each individual frame separately. After an initial phase of identifying facial landmarks in the frame, OpenFace is capable of estimating the intensity of 17 action units separately, all of which were included in the analysis (for a list of all AUs, see ). Validation studies of OpenFace reported that its accuracy surpasses other methods (e.g., generative latent tree, convolutional neural networks; Baltrusaitis et al., Citation2018). This was also true when externally validated using unseen manually coded facial data (Namba et al., Citation2021). Additionally, OpenFace-based evaluations of arousal and valence were significantly correlated with therapists’ evaluations of their patients’ arousal and valence, albeit not with patients’ own reports (Terhürne et al., Citation2022).
Table I. List of action units assessed by OpenFace 2.0.
To calculate facial expressiveness from the extracted intensity of action units, we used a simple distance calculation between Cartesian coordinates. Each accumulated sample (representing a frame of the video recording) was placed as a point in a Cartesian system with 17 dimensions, for the 17 action units measured. We then measured the distance between each two samples representing consecutive frames. Distance was measured via the numpy.linalg.norm function in the NumPy library (Harris et al., Citation2020), using Python (Python 3.6.8; Van Rossum & Drake, Citation2009). The code has been made available on a public repository (https://osf.io/6th5q/?view_only = bea87b55c1be4c16ac9f18a3548d58ab). The result was a difference score between each two consecutive facial expression samples, representing the change in facial expressions. Higher scores represent more facial behavior. A value of zero (the lowest difference score achievable) represents a face that did not change between two consecutive frames. This distance score was named “facial expressiveness.”
To allow the exploration of different time spans, facial expressiveness was averaged with moving, non-overlapping windows. As there were no hypotheses as to why a certain time span is more relevant than another, we explored all time spans reported by studies investigating the association between emotional dynamics and the therapeutic alliance. Overall, we created 90 versions of the data (a maximum of 90 s was chosen due to this being analyzed by Tal et al., Citation2023). The first version included facial expressiveness with a moving window of 1 s length, the second version included facial expressiveness with a moving window of 2 s length, etc. Eventually, time spans of 1–90 s were analyzed for. However, only a subgroup of these windows will be reported (length of 1, 5, 10, 30, 60, and 90 s) in the results section. A full description of the entire 90-windows analysis can be found in the supplementary material. Additionally, in segmenting the data into windows, this analysis explores emotional dynamics in facial behavior with a constant internal temporal structure. While a case can be made for why emotional dynamics should be analyzed with dynamic temporal structure, keeping the temporal structure constant does have some advantages. One advantage is that it allows contrasting short-term reactivity with long-term reactivity. For example, keeping the temporal structure constant allows the contrasting of reactivity in shorter time spans (e.g., 1 s) with longer time spans (e.g., 10 s or 30 s). In other words, keeping the internal temporal structure constant, while it has its caveats, may allow taping into different mechanisms that are at play. See for an illustration and for descriptive statistics.
Figure 1. Preprocessing pipeline of facial reactivity and stability.
Notes: This figure demonstrates the pipeline implemented to achieve an estimate of facial reactivity and stability for both patients and therapists. First, we obtained the raw scores of facial expressiveness for patients and therapists, as they are calculated for each frame of video recording. The left-most panel shows an example of 8 s of expressiveness, with a hypothetical dyad of patient and therapist. Second, we create 90 datasets, for 90 different possible time spans (i.e., 1, 2 s, etc.). The second panel from the left shows the result of this step. Third, an APIM model is fitted to each of these datasets. The model is made to predict expressiveness at time t with expressiveness at time t–1. The APIM model was defined with random effects of dyad and session. Finally, this results in an estimation of reactivity and stability, of both patients and therapists, in each session separately. These estimations were then used to predict WAI scores from the same session. This is demonstrated by the right-most panel, that shows the resulting datasets. Pr = patient reactivity, Ps = patient stability, Tr = therapist reactivity, Ts = therapist stability, WAI = Working Alliance Inventory.

Table II. Descriptives statistics.
Data Analysis
All analyses were conducted in the PROC MIXED program (Littell et al., Citation2006) in SAS 9.4 (SAS Institute, Citation2015) due to the nested structure of the data. To measure reactivity and stability in facial expressiveness, we fitted an Actor-Partner Interdependence Model (APIM; Kenny et al., Citation2006) unto the data. The APIM estimates the level of activity (i.e., facial expressiveness) in each participant as predicted by that participant’s previous level, and the previous level of their partner. This results in estimates of actor and partner effects. If the participant’s levels are consistent across time, the estimate of the actor effect will be greater. If a change in the participant’s levels is commonly followed by a similar change in their partner’s levels, the estimate of the partner effect will be greater. Patient stability was defined as the effect of patient expressiveness when predicting subsequent patient expressiveness (patient actor effect). Therapist stability was defined as the effect of therapist expressiveness when predicting subsequent therapist expressiveness (therapist actor effect). Patient reactivity was defined as the effect of therapist expressiveness when predicting subsequent patient expressiveness (therapist partner effect). Therapist reactivity was defined as the effect of patient expressiveness when predicting subsequent therapist expressiveness (patient partner effect). To achieve an estimation of stability and reactivity for each session separately, dyad and session were random effects in the model. These estimations of reactivity and stability were later used to predict the therapeutic alliance strength of the same sessions in which they were measured (see for descriptive statistics of reactivity and stability).
An analysis of APIM is based on the premise that one partner’s attributes and behaviors can affect the other partner’s behavior (Bolger & Laurenceau, Citation2013; Kenny et al., Citation2006). This is a valid assumption in the context of facial expressions of two individuals in an interaction. Another requirement of APIM is that the two variables are placed upon the same evaluation rating scale (Bolger & Laurenceau, Citation2013; Kenny et al., Citation2006). This is also a valid assumption in the case of the current study, as the same phenomenon (i.e., facial expressiveness) was assessed in both patients and therapists, in the exact same manner.
The data were hierarchically nested on two levels: assessments nested within patients. Following Bosker and Snijders (Citation2011; see also Falkenström et al., Citation2020), we adhered to the rule of thumb noting that cases in which there are less than ten groups (our sample had seven therapists) should not be estimated using random effects, thus no therapist effect was included in the model (adding a therapist effect did not change the results, this sensitivity analysis is reported in the supplementary material). To account for the resulting non-independence of assessments, and to prevent inflation of the effects, we added the patient as random effects. To measure the amount of unexplained variance in outcome due to the random effects of the patient, we used intra-class correlations (ICCs). The patient’s random variance component was estimated based on a model with only random intercept of the patient with no other covariates.
To examine alliance changes over time, we evaluated the following trend models for each: stability over time, and log of time as fixed effect. We started with a model with only a fixed intercept and random effect of log of time, and added a fixed effect of log of time. We used the Bayesian information criterion (BIC) to determine whether the inclusion of each term improved the model fit, and chose the model that reached the lowest BIC. As the aim of this model selection was to examine alliance changes over time, facial data was not included. The choice of modeling time logarithmically is due to the literature demonstrating that the psychotherapy process can follow a logarithmic pattern (Gallop & Tasca, Citation2009; Nissen-Lie et al., Citation2015; Tasca & Gallop, Citation2009), and therapeutic alliance literature emphasizing the importance of the early stages of treatment in building and establishing the working alliance (Horvath & Luborsky, Citation1993). Results of a similar analysis with time modeled linearly are presented in the supplementary material.
With the chosen model, we estimated the effects of stability and reactivity of both patients and therapists on rated alliance. The final model was as follows:
with
as WAI rated by patient from dyad i at session t;
as patient stability of patient from dyad i at session t;
as patient reactivity of patient from dyad i at session t;
as therapist stability of therapist from dyad i at session t;
as therapist stability of therapist from dyad i at session t; and
as error of dyad i at session t.
Results
Estimated variance of the patient’s random effect for alliance was significant ( = 0.7, p < 0.001, ICC = 0.71). This means that there were significant differences in reported WAI scores between patients, regardless of the estimated effects. The model that was found to have the best fit based on the BIC for alliance was with a fixed and random effect of log of time. This model was used in all analyses. In all models analyzed, the effect of log of time was stable and significantly positive (estimates range was 0.27–0.28, mean of estimates was 0.27, SE was 0.04, p < 0.0001). This means that alliance levels tended to rise quickly and remain strong across treatment. Therapist’s reactivity in facial expressiveness, in each time window (i.e., 1, 5, 10, 30, 60, 90 s) was significantly associated with the therapeutic alliance (estimates range was 0.52–0.71 (mean = 0.6), SE was 0.17–0.27, p-value was 0.001–0.017). Therapist’s stability in facial expressiveness, in each time window was also significantly associated with the therapeutic alliance (estimates range was 1.6–2.84 (mean = 2), SE was 0.47–0.83, p-value was 0.001–0.009). Patient’s reactivity in facial expressiveness was not significantly associated with the therapeutic alliance in almost all time windows. Only in time windows of 1 s was patient reactivity in facial expressiveness significantly associated with the therapeutic alliance (B = 2.23, SE = 0.78, p = 0.004). Patient stability in facial expressiveness was significantly associated with the therapeutic alliance only in windows of 5 s (B = 0.56, SE = 0.28, p = 0.042). All reported effects regarding therapist reactivity and stability, and patient reactivity, remained significant when excluding outliers. In contrast, the significant effect of patient stability did not remain once outliers were excluded (higher than 3.3 standardized score in absolute value), and thus this effect was not further interpreted. Analyses of windows under one second (0.4, 0.6, and 0.8 s) revealed that therapist reactivity and stability, as well as patient reactivity remained significant in predicting the alliance. See for an illustration of the results and for a complete description of linear models. Analyses of WAI subscales, and therapist-rated alliance were added in the supplementary material.
Figure 2. Types of emotional dynamics that are associated with the therapeutic alliance.
Notes: This figure presents the results of the main statistical analysis. Each line represents a dynamic that was significantly associated with the therapeutic alliance. All estimated effects were positive. The line with the square-shaped head represents therapist reactivity (significant across all analyzed time spans). The line with the arrow-shaped head represents therapist stability (significant across all analyzed time spans). The line with the circle-shaped head represents patient reactivity (significant only in time span of one second).
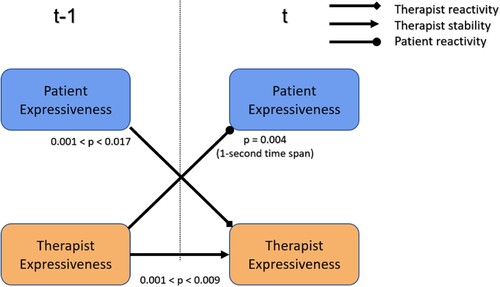
Table III. Results of all regression models.
Discussion
The present study explored emotional reactivity and emotional stability between patients’ and therapists’ facial expressions as indicators of therapeutic alliance strength. Findings suggest that both therapist reactivity and therapist stability are uniquely associated with a stronger alliance. Findings also suggest that patient reactivity is associated with a stronger alliance, but only in a time span of one second. Patients’ stability, in contrast, was not consistently associated with alliance strength, no matter the specific time span analyzed. These findings contribute to the currently developing knowledge regarding the association between types of emotional dynamics between therapists and patients and the strength of the therapeutic alliance.
Therapist emotional reactivity has emerged as a significant indicator of the therapeutic alliance. As hypothesized, our findings suggest that the more the therapist reacts to their patient, via their facial expressions, the stronger is the therapeutic alliance. The effect remained significant across all analyzed time spans (i.e., 1–90 s). This finding is consistent with the literature. The notion that therapists’ emotional reactivity may be beneficial for the therapeutic relationship has already been suggested (e.g., Kimerling et al., Citation2000). However, most studies have focused on self-report measures, administered before or after the session (Sloan & Kring, Citation2007). There is only one other study, to our knowledge, that has investigated the association between the alliance and emotional reactivity within the therapeutic session. In this study, Bryan et al. (Citation2018) explored acoustic behavior and reported that the more a therapist’s emotional arousal follows that of the patient’s, the stronger is the therapeutic bond. The current study adds to the literature by reporting a similar effect to that reported by Bryan et al. (Citation2018), albeit with facial expressions. Specifically, this may suggest that the association between therapist reactivity and the alliance is robust enough that it transcends the specific modality it is measured in (e.g., facial behavior, acoustic properties).
Constructs with some possible overlap with therapist reactivity have also been referenced in other areas of psychotherapy research, further supporting the reported findings of the current study. Elkin et al. (Citation2014) used the term “therapist responsiveness” to describe situations when the therapist is attentive to the patient, makes eye contact, makes an effort to see the patient’s perspective, and more. The authors suggested that therapist responsiveness, manually coded within sessions by outside observers, is associated with a higher quality of the therapeutic relationship. It is important to note that therapist reactivity and therapist responsiveness are not interchangeable terms. Therapist reactivity is being suggested by this study as a possible indicator of therapeutic alliance strength. Therapist responsiveness, in contrast, has already been heavily explored and is more broadly defined as any behavior of the therapist that is being influenced by any emerging context in the therapy (Kramer & Stiles, Citation2015). When therapist reactivity is low, however, this may be a sign that the therapist is bored, tired, or simply emotionally distant. Dahl et al. (Citation2017) used the term “therapist disengagement” to describe similar situations. The authors found that therapist disengagement, as indicated by a post-session self-report measure, indicates poorer therapy outcomes. Therapist disengagement was suggested in the literature both as a possible result of emotional burnout (Delgadillo et al., Citation2018), and as a necessary self-care measure for therapists to prevent a state of emotional burnout (Figley, Citation2002). The current study adds to this literature by demonstrating the importance of therapist reactivity to the therapy process while using an objective methodology, capable of automatically analyzing large amounts of data. This type of methodology potentially allows a more fine-grained analysis of within-session processes, while providing a labor-free alternative to the labor-intensive option of manual coding.
Therapist emotional stability has also emerged as a significant indicator of the therapeutic alliance. As hypothesized, our findings suggest that the more the therapist stays emotionally stable within the session, as expressed via their facial expressions, the stronger is the therapeutic alliance. The effect remained significant across all analyzed time spans (i.e., 1–90 s). This finding seemingly contradicts Bryan et al. (Citation2018), who did not find a correlation between therapist emotional stability and the therapeutic bond. One possible post-hoc explanation of this contradiction may be rooted in the differences in stability measurement between the two studies. While our methodology entailed exploration of emotionally relevant behavior continuously, Bryan et al. (Citation2018), by analyzing acoustic behavior, were inherently limited in their exploration, measuring emotional arousal only at times when talking took place. If replicated in other studies, it may be suggested that therapists experience different emotional arousal (e.g., higher or lower levels) when they talk, in comparison to when they listen. This hypothesis should be explored in other studies in a more direct manner. Still, this contradiction may mean that the two studies have tapped into different aspects of emotional stability; hence the contradictory findings. If replicated, this discrepancy suggests that emotional stability may be too broad of a term, and that different types of therapist emotional stability should be further considered and explored.
Therapist emotional stability is less commonly explored empirically in the literature, in comparison to therapist emotional reactivity. One area of psychotherapy research that supports these findings is countertransference management. Countertransference was originally introduced by Freud (Citation1910) and can be defined as the thoughts, perceptions, feelings, and behavior of the therapist, as they are being influenced by the patient (Hayes et al., Citation2011). Oftentimes this influence, taken at face value, can be detrimental to the therapeutic process. At the same time, psychotherapeutic theories see emerging countertransference as an impasse in therapy, which, if managed by the therapist, can facilitate the therapy process instead of hindering it (Hayes et al., Citation2011; Levenson, Citation1995). An example of such an impasse can be as follows: a person is seeking therapy due to recurring painful breakups. They find themselves eventually losing patience with their partner’s behavior and acting rudely toward them, which inevitably leads to clashes and yelling. When this patient comes to treatment, they may find themselves losing patience toward their therapist as well, which may cause the patient to behave rudely in sessions. In response to this attitude, the therapist may feel hurt, belittled and even the need to “strike back”. In other words, the therapist may find themselves influenced and subtly coerced into this maladaptive dance, leading to the same fights with the patient that the therapy is meant to mitigate. Alternatively, at this impasse, the therapist may be able to remain emotionally stable, managing this countertransference (Hayes et al., Citation2011), and exploring the patient’s current attitude. Eventually, this stability may allow the patient a better understanding of themselves and of the therapy process (Hayes et al., Citation2011), which may manifest as a stronger therapeutic alliance. This rationale may hold some resemblance to the psychoanalytic rationale of therapist abstinence (Freud, Citation1915), according to which the therapist is advised to keep from providing the patient with the immediate emotional response they are expecting or searching for, in order to facilitate the therapy process. These post-hoc interpretations should be considered carefully, however, as this is the first study to measure patients’ and therapists’ facial expressiveness within the session. Further exploration into the stability of facial expressiveness is required to better understand the underlying subjective experience it indicates.
Turning to the patient’s facial expressions, patient emotional reactivity has emerged as a significant indicator of the therapeutic alliance. As hypothesized, our findings suggest that the more the patient reacts to the therapist, via their facial expressions, the stronger is the therapeutic alliance. The effect reached significance, however, only in a time span of 1 s. Analyses of longer time spans did not yield a significant effect for patient reactivity. This finding is consistent with the literature. Specifically, by exploring acoustic behavior, Bryan et al. (Citation2018) reported that when a patient's emotional arousal follows that of the therapist’s, the therapeutic bond is stronger. Our study adds to this literature by suggesting that the effect of patient reactivity may only emerge in very short time spans (e.g., 1 s).
It is intriguing to speculate why patients’ reactivity was associated with the therapeutic alliance only in a short time span. This consideration should be taken carefully, as this is an exploratory analysis without prior hypothesis and the first study that systematically explores different time spans of emotional dynamics between patients and therapists. Still, while these findings require further replication in future studies, a possible post-hoc explanation can be suggested when considering other areas of research that have also focused on human emotional reaction in short time spans (i.e., around 1 s). One such area of research is that of the orienting response. The orienting response can be defined as a person’s immediate response to a novel or significant change in their environment (Bradley, Citation2009), as long as this change is not sudden enough to elicit a startle response (Graham, Citation2021). Some behavioral measures of the orienting response (e.g., skin conductance, pupil dilation) were demonstrated to also peak around the same time span as our reported effect of patient reactivity (around 1 s after the stimulus was introduced; Nieuwenhuis et al., Citation2011). Importantly, focusing more on the significance of a stimulus rather than its novelty, it was suggested that motivational systems are mediating the orienting response (Bradley, Citation2009). In other words, the orienting response may be at least partially dependent on the meaning of the stimulus for the person (Johnson, Citation1986) and the person’s attitude toward it (Sutton et al., Citation1965). For the case of the current study, if considered in terms of the orienting response, the effect of patient reactivity may potentially mean that the therapeutic alliance was stronger when the patient was more motivated to attend their therapist’s communication (via facial expression).
The effect of log of time has also reached significance in addition the interdependence effects. The positive significant effect of log of time suggested that alliance tended to increase in the early stages of therapy, and remain strong throughout it. This finding is supported by the therapeutic alliance literature, which emphasizes the importance of the early stages of treatment in building and establishing the working alliance (Horvath & Luborsky, Citation1993), as well as the literature suggesting that the psychotherapy process can follow a logarithmic trajectory (Gallop & Tasca, Citation2009; Nissen-Lie et al., Citation2015; Tasca & Gallop, Citation2009).
In interpreting the results, it is important to remember that facial expressions can serve many communicative functions, sometimes even simultaneously (Chovil, Citation1991; Fridlund & Russell, Citation2006). Some of these functions can be emotionally relevant, like raising one’s eyebrows to signal surprise. Other functions can be more neutral, like opening one’s mouth to signal their motivation to begin talking, or to signal action readiness in general (Frijda & Tcherkassof, Citation1997). Thus, the phenomena of reactivity and stability in facial expressions that are discussed in this study can be an equifinal manifestation of different processes of nonverbal communicative events that occur between patients and therapists. Future studies may distinguish different communicative functions of facial expressions when investigating reactivity and stability in psychotherapy.
Reported findings should be considered carefully, however, as there are some limitations to the methodology that was implemented. First, this study introduces a new data-driven approach to measuring facial behavior. While this approach allowed measurement of the amount of facial expressiveness, it is still unclear what the dynamics of facial expressiveness mean. For example, therapist stability in facial expressiveness requires further empirical investigation to achieve a better grasp of the underlying experience it indicates. Second, facial expressions were analyzed continuously, even when patients and therapists were talking, which may have influenced the results. While it can be argued that facial behavior is worth analyzing while the person is engaged in talking, it may be beneficial to separate moments of inherent facial movement (e.g., talking) from moments in which the face could remain still (e.g., listening). One possible way to do that is to fuse facial data with acoustic data within the same analysis. This may allow a more nuanced multimodal analysis which taps into different modes of reactivity and stability. Third, while the reported methodology was intentionally designed with minimal assumptions about the dynamics of facial behavior, future studies may also distinguish between different properties of the different facial muscles. For example, facial expressions can encompass larger facial areas (e.g., a smile) or smaller ones (e.g., a blink), they can also be longer or shorter. Some action units tend to activate together as well (e.g., eyebrows and eyelids in a surprised expression). One way to address these different dynamics is by adopting an event-focused approach. For example, a facial event can be a happy expression or a brief, offended expression. Facial events have onset, duration, and termination, as well as a group of facial muscles, which can all be predefined theoretically and assessed statistically, to help distinguish between signal and noise or to explore specific facial behaviors. Fourth, this study has relied upon a component conceptualization of emotions (Moors, Citation2009; Scherer, Citation2005). Furthermore, within the component conceptualization this study has focused on the motor manifestation of emotion (i.e., facial expressions). Investigating changes in other components of emotion, or relying upon a different conceptualization of emotion altogether, may yield different results. Fifth, this study focused on patients diagnosed with major depressive disorder. The literature links depression with lower emotional expression in facial behavior (Berenbaum & Oltmanns, Citation1992) and impaired attribution of emotion in facial behavior of others (Demenescu et al., Citation2010). Both findings can heavily influence nonverbal communication via facial expressions in therapy of patients with depression. Analyses of therapy with patients from different populations may lead to different results. Sixth, the reported analysis in this study adopted a non-overlapping windows approach. While this approach seems to be more common in the literature (e.g., Bar-Kalifa et al., Citation2019; Tschacher & Meier, Citation2020), it also introduces an implicit overlap between the duration of facial behavior measured and the time difference in which a reaction in the other is assessed. This is important as different methodologies were shown to tap into different aspects of nonverbal interdependence (for example with physiological data, see Tschacher & Meier, Citation2020). Future studies may help broaden the knowledge in this field by suggesting more approaches to data segmentation when assessing interdependence. Seventh, the analysis reported had a constant temporal structure for emotional dynamics (i.e., time spans analyzed in each version of data remained constant). Future studies can select some dynamic criteria by which to temporally segment the clinical material (e.g., by fusing acoustic analysis of talking turns; e.g., Bryan et al., Citation2018). This may lead to tapping into different mechanisms of communication between patients and therapists.
This study explored emotional reactivity and stability between patients and therapists, as they manifest in their facial expressions. Therapist reactivity and stability, as well as patient stability, were positively associated with the strength of the therapeutic alliance. This study demonstrates the great potential of exploring emotional dynamics between patients and therapists by empirically supporting their importance to the therapy process. Researchers may also explore a link with therapy outcome in future studies. If replicated, these findings suggest not only the potential benefit of the emotionally reactive therapist, but also the benefit of the emotionally stable therapist, who integrates their own personality, their presence, into their practice.
tpsr-2023-0149-File004
Download MS Word (43.7 KB)tpsr-2023-0149-File003
Download MS Word (438 KB)tpsr-2023-0149-File001
Download MS Word (22.6 KB)Acknowledgements
We thank Dr. Ilan Shimshoni (University of Haifa) for establishing the infrastructure required to use OpenFace 2.0.
Disclosure Statement
No potential conflict of interest was reported by the author(s).
Supplemental Data
Supplemental data for this article can be accessed online at https://doi.org/10.1080/10503307.2024.2311777.
Additional information
Funding
References
- Atzil-Slonim, D., Soma, C. S., Zhang, X., Paz, A., & Imel, Z. E. (2023). Facilitating dyadic synchrony in psychotherapy sessions: Systematic review and meta-analysis. Psychotherapy Research, 33(7), 898–917.
- Baltrusaitis, T., Zadeh, A., Lim, Y. C., & Morency, L. P. (2018). Openface 2.0: Facial behavior analysis toolkit. In 2018 13th IEEE International Conference on Automatic Face & Gesture Recognition (FG 2018) , Xi'an, China, 2018 (pp. 59–66). IEEE. https://doi.org/10.1109/FG.2018.00019
- Bänninger-Huber, E. (1992). Prototypical affective microsequences in psychotherapeutic interaction. Psychotherapy Research, 2(4), 291–306. https://doi.org/10.1080/10503309212331333044
- Bar-Kalifa, E., Prinz, J. N., Atzil-Slonim, D., Rubel, J. A., Lutz, W., & Rafaeli, E. (2019). Physiological synchrony and therapeutic alliance in an imagery-based treatment. Journal of Counseling Psychology, 66(4), 508. https://doi.org/10.1037/cou0000358
- Berenbaum, H., & Oltmanns, T. F. (1992). Emotional experience and expression in schizophrenia and depression. Journal of Abnormal Psychology, 101(1), 37.
- Bolger, N., & Laurenceau, J. P. (2013). Intensive longitudinal methods: An introduction to diary and experience sampling research. Guilford Press.
- Bordin, E. S. (1979). The generalizability of the psychoanalytic concept of the working alliance. Psychotherapy: Theory, Research & Practice, 16(3), 252. https://doi.org/10.1037/h0085885
- Bosker, R., & Snijders, T. A. (2011). Multilevel analysis: An introduction to basic and advanced multilevel modeling (pp. 1–368). Sage.
- Bradley, M. M. (2009). Natural selective attention: Orienting and emotion. Psychophysiology, 46(1), 1–11. https://doi.org/10.1111/j.1469-8986.2008.00702.x
- Bryan, C. J., Baucom, B. R., Crenshaw, A. O., Imel, Z., Atkins, D. C., Clemans, T. A., Leeson, B., Burch, T. S., Mintz, J., & Rudd, M. D. (2018). Associations of patient-rated emotional bond and vocally encoded emotional arousal among clinicians and acutely suicidal military personnel. Journal of Consulting and Clinical Psychology, 86(4), 372. https://doi.org/10.1037/ccp0000295
- Butler, E. A. (2011). Temporal interpersonal emotion systems: The “TIES” that form relationships. Personality and Social Psychology Review, 15(4), 367–393. https://doi.org/10.1177/1088868311411164
- Calder, A. J., Burton, A. M., Miller, P., Young, A. W., & Akamatsu, S. (2001). A principal component analysis of facial expressions. Vision Research, 41(9), 1179–1208. https://doi.org/10.1016/S0042-6989(01)00002-5
- Chovil, N. (1991). Discourse-oriented facial displays in conversation. Research on Language & Social Interaction, 25(1–4), 163–194. https://doi.org/10.1080/08351819109389361
- Dahl, H. S. J., Høglend, P., Ulberg, R., Amlo, S., Gabbard, G. O., Perry, J. C., & Christoph, P. C. (2017). Does therapists’ disengaged feelings influence the effect of transference work? A study on countertransference. Clinical Psychology & Psychotherapy, 24(2), 462–474. https://doi.org/10.1002/cpp.2015
- Delgadillo, J., Saxon, D., & Barkham, M. (2018). Associations between therapists’ occupational burnout and their patients’ depression and anxiety treatment outcomes. Depression and Anxiety, 35(9), 844–850. https://doi.org/10.1002/da.22766
- Demenescu, L. R., Kortekaas, R., Den Boer, J. A., & Aleman, A. (2010). Impaired attribution of emotion to facial expressions in anxiety and major depression. PLoS One, 5(12), e15058.
- Deres-Cohen, K., Dolev-Amit, T., Peysachov, G., Ramseyer, F. T., & Zilcha-Mano, S. (2021). Nonverbal synchrony as a marker of alliance ruptures. Psychotherapy, 58(4), 499. https://doi.org/10.1037/pst0000384
- Dimberg, U., Thunberg, M., & Elmehed, K. (2000). Unconscious facial reactions to emotional facial expressions. Psychological Science, 11(1), 86–89. https://doi.org/10.1111/1467-9280.00221
- Ekman, P. (1992). An argument for basic emotions. Cognition & Emotion, 6(3–4), 169–200. https://doi.org/10.1080/02699939208411068
- Ekman, P. (1993). Facial expression and emotion. American Psychologist, 48(4), 384. https://doi.org/10.1037/0003-066X.48.4.384
- Ekman, P., & Friesen, W. V. (1969). The repertoire of nonverbal behavior: Categories, origins, usage, and coding. Semiotica, 1(1), 49–98. https://doi.org/10.1515/semi.1969.1.1.49
- Ekman, P., & Friesen, W. V. (1977). Manual for the facial action coding system. Consulting Psych. Press.
- Ekman, P., & Oster, H. (1979). Facial expressions of emotion. Annual Review of Psychology, 30(1), 527–554. https://doi.org/10.1146/annurev.ps.30.020179.002523
- Elkin, I., Falconnier, L., Smith, Y., Canada, K. E., Henderson, E., Brown, E. R., & Mckay, B. M. (2014). Therapist responsiveness and patient engagement in therapy. Psychotherapy Research, 24(1), 52–66. https://doi.org/10.1080/10503307.2013.820855
- Falkenström, F., Solomonov, N., & Rubel, J. A. (2020). Do therapist effects really impact estimates of within-patient mechanisms of change? A Monte Carlo simulation study. Psychotherapy Research, 30(7), 885–899. https://doi.org/10.1080/10503307.2020.1769875
- Figley, C. R. (2002). Compassion fatigue: Psychotherapists’ chronic lack of self care. Journal of Clinical Psychology, 58(11), 1433–1441. https://doi.org/10.1002/jclp.10090
- Flückiger, C., Del Re, A. C., Wampold, B. E., Symonds, D., & Horvath, A. O. (2012). How central is the alliance in psychotherapy? A multilevel longitudinal meta-analysis. Journal of Counseling Psychology, 59(1), 10. https://doi.org/10.1037/a0025749
- Ford, J. D. (2021). Crises as a challenge to therapist self-regulation. In J. D. Ford (Ed.), Crises in the psychotherapy session: Transforming critical moments into turning points (pp. 139–169). American Psychological Association. https://doi.org/10.1037/0000225-007
- Frank, M. G., Ekman, P., & Friesen, W. V. (1993). Behavioral markers and recognizability of the smile of enjoyment. Journal of Personality and Social Psychology, 64(1), 83. https://doi.org/10.1037/0022-3514.64.1.83
- Freud, S. (1910). Future prospects of psychoanalytic therapy. In J. Strachey (Ed.), The standard ed. of the complete works of Sigmund Freud (pp. 139–151). Hogarth Press.
- Freud, S. (1915). Observations on transference-love. SE (12), 157–171.
- Fridlund, A. J., & Russell, J. A. (2006). The functions of facial expressions: What's in a face? In V. Manusov & M. L. Patterson (Eds.), Sage handbook of nonverbal communication (pp. 299–319). Sage Publications.
- Frijda, N. H., & Tcherkassof, A. (1997). Facial expressions as modes of action readiness. In J. A. Russell & J. M. Fernández-Dols (Eds.), The psychology of facial expression (pp. 78–102). Cambridge University Press.
- Gallop, R., & Tasca, G. A. (2009). Multilevel modeling of longitudinal data for psychotherapy researchers: II. The complexities. Psychotherapy Research, 19(4–5), 438–452. https://doi.org/10.1080/10503300902849475
- Gehricke, J. G., & Shapiro, D. (2000). Reduced facial expression and social context in major depression: Discrepancies between facial muscle activity and self-reported emotion. Psychiatry Research, 95(2), 157–167. https://doi.org/10.1016/S0165-1781(00)00168-2
- Gentsch, K., Grandjean, D., & Scherer, K. R. (2015). Appraisals generate specific configurations of facial muscle movements in a gambling task: Evidence for the component process model of emotion. PLoS One, 10(8), e0135837. https://doi.org/10.1371/journal.pone.0135837
- Graham, F. K. (2021). Distinguishing among orienting, defense, and startle reflexes. In The orienting reflex in humans (pp. 137–167). Routledge.
- Hamilton, M. A. X. (1967). Development of a rating scale for primary depressive illness. British Journal of Social and Clinical Psychology, 6(4), 278–296. https://doi.org/10.1111/j.2044-8260.1967.tb00530.x
- Harris, C. R., Millman, K. J., van der Walt, S. J., Gommers, R., Virtanen, P., Cournapeau, D., Wieser, E., Taylor, J., Berg, S., Smith, N. J., & Kern, R. (2020). Array programming with NumPy. Nature, 585(7825), 357–362. https://doi.org/10.1038/s41586-020-2649-2
- Hayes, J. A., Gelso, C. J., & Hummel, A. M. (2011). Managing countertransference. Psychotherapy, 48(1), 88. https://doi.org/10.1037/a0022182
- Horvath, A. O., & Greenberg, L. S. (1989). Development and validation of the Working Alliance Inventory. Journal of Counseling Psychology, 36(2), 223. https://doi.org/10.1037/0022-0167.36.2.223
- Horvath, A. O., & Luborsky, L. (1993). The role of the therapeutic alliance in psychotherapy. Journal of Consulting and Clinical Psychology, 61(4), 561. https://doi.org/10.1037/0022-006X.61.4.561
- Johnson, R. (1986). A triarchic model of P300 amplitude. Psychophysiology, 23(4), 367–384. https://doi.org/10.1111/j.1469-8986.1986.tb00649.x
- Kenny, D. A., Kashy, D. A., & Cook, W. L. (2006). Dyadic data analysis. Guilford Press.
- Kimerling, R. E., Zeiss, A. M., & Zeiss, R. A. (2000). Therapist emotional responses to patients: Building a learning-based language. Cognitive and Behavioral Practice, 7(3), 312–321. https://doi.org/10.1016/S1077-7229(00)80089-9
- Kleinbub, J. R. (2017). State of the art of interpersonal physiology in psychotherapy: A systematic review. Frontiers in Psychology, 8, 2053. https://doi.org/10.3389/fpsyg.2017.02053
- Kleinbub, J. R., Talia, A., & Palmieri, A. (2020). Physiological synchronization in the clinical process: A research primer. Journal of Counseling Psychology, 67(4), 420. https://doi.org/10.1037/cou0000383
- Koole, S. L., & Tschacher, W. (2016). Synchrony in psychotherapy: A review and an integrative framework for the therapeutic alliance. Frontiers in Psychology, 7, 862. https://doi.org/10.3389/fpsyg.2016.00862
- Kramer, U., & Stiles, W. B. (2015). The responsiveness problem in psychotherapy: A review of proposed solutions. Clinical Psychology: Science and Practice, 22(3), 277. https://doi.org/10.1111/cpsp.12107
- Leibovich, L., McCarthy, K. S., & Zilcha-Mano, S. (2020). How do supportive techniques bring about therapeutic change: The role of therapeutic alliance as a potential mediator. Psychotherapy, 57(2), 151. https://doi.org/10.1037/pst0000253
- Leibovich, L., Nof, A., Auerbach-Barber, S., & Zilcha-Mano, S. (2018). A practical clinical suggestion for strengthening the alliance based on a supportive-expressive framework. Psychotherapy, 55(3), 231–240. https://doi.org/10.1037/pst0000195
- Levenson, H. (1995). Time-limited dynamic psychotherapy. Basic Books.
- Littell, R. C., Milliken, G. A., Stroup, W. W., Wolfinger, R. D., & Oliver, S. (2006). SAS for mixed models. SAS Publishing.
- Luborsky, L. (1984). Principles of psychoanalytic psychotherapy. A manual for supportive-expressive treatment. Basic Books.
- Luborsky, L., Mark, D., Hole, A. V., Popp, C., Goldsmith, B., & Cacciola, J. (1995). Supportive-expressive dynamic psychotherapy of depression: A time-limited version. In J. P. Barber & P. Crits-Christoph (Eds.), Dynamic therapies for psychiatric disorders (Axis I) (pp. 13–42). Basic Books.
- Main, A., Paxton, A., & Dale, R. (2016). An exploratory analysis of emotion dynamics between mothers and adolescents during conflict discussions. Emotion, 16(6), 913. https://doi.org/10.1037/emo0000180
- Matsumoto, D., Keltner, D., Shiota, M. N., O’Sullivan, M., & Frank, M. (2008). Facial expressions of emotion. Handbook of Emotions, 3, 211–234.
- McParlin, Z., Cerritelli, F., Friston, K. J., & Esteves, J. E. (2022). Therapeutic alliance as active inference: The role of therapeutic touch and synchrony. Frontiers in Psychology, 13, 329. https://doi.org/10.3389/fpsyg.2022.783694
- Merten, J. (2003). Context-analysis of facial-affective behavior in clinical populations. In M. Katsikitis (Ed.), The human face: Measurement and meaning (pp. 131–147). Springer.
- Merten, J. (2005). Facial microbehavior and the emotional quality of the therapeutic relationship. Psychotherapy Research, 15(3), 325–333. https://doi.org/10.1080/10503300500091272
- Merten, J., & Brunnhuber, S. (2004). Facial expression and experience of emotions in psychodynamic interviews with patients suffering from a pain disorder. Psychopathology, 37(6), 266–271. https://doi.org/10.1159/000081982
- Moors, A. (2009). Theories of emotion causation: A review. Cognition and Emotion, 23(4), 625–662. https://doi.org/10.1080/02699930802645739
- Namba, S., Sato, W., Osumi, M., & Shimokawa, K. (2021). Assessing automated facial action unit detection systems for analyzing cross-domain facial expression databases. Sensors, 21(12), 4222. https://doi.org/10.3390/s21124222
- Nieuwenhuis, S., De Geus, E. J., & Aston-Jones, G. (2011). The anatomical and functional relationship between the P3 and autonomic components of the orienting response. Psychophysiology, 48(2), 162–175. https://doi.org/10.1111/j.1469-8986.2010.01057.x
- Nissen-Lie, H. A., Havik, O. E., Høglend, P. A., Rønnestad, M. H., & Monsen, J. T. (2015). Patient and therapist perspectives on alliance development: Therapists’ practice experiences as predictors. Clinical Psychology & Psychotherapy, 22(4), 317–327. https://doi.org/10.1002/cpp.1891
- Pfohl, B., Blum, N., & Zimmerman, M. (1997). Structured interview for DSM-IV personality: Sidp-IV. American Psychiatric Pub.
- Ramseyer, F., & Tschacher, W. (2014). Nonverbal synchrony of head-and body-movement in psychotherapy: Different signals have different associations with outcome. Frontiers in Psychology, 5, 979. https://doi.org/10.3389/fpsyg.2014.00979
- Rasting, M., & Beutel, M. E. (2005). Dyadic affective interactive patterns in the intake interview as a predictor of outcome. Psychotherapy Research, 15(3), 188–198. https://doi.org/10.1080/10503300512331335039
- Reich, C. M., Berman, J. S., Dale, R., & Levitt, H. M. (2014). Vocal synchrony in psychotherapy. Journal of Social and Clinical Psychology, 33(5), 481–494. https://doi.org/10.1521/jscp.2014.33.5.481
- Russell, J. A. (1980). A circumplex model of affect. Journal of Personality and Social Psychology, 39(6), 1161. https://doi.org/10.1037/h0077714
- SAS Institute Inc. (2015). SAS/STAT® 14.1 user’s guide.
- Scherer, K. R. (2005). What are emotions? And how can they be measured? Social Science Information, 44(4), 695–729. https://doi.org/10.1177/0539018405058216
- Schmidt, K. L., Ambadar, Z., Cohn, J. F., & Reed, L. I. (2006). Movement differences between deliberate and spontaneous facial expressions: Zygomaticus major action in smiling. Journal of Nonverbal Behavior, 30(1), 37–52. https://doi.org/10.1007/s10919-005-0003-x
- Sels, L., Ceulemans, E., Bulteel, K., & Kuppens, P. (2016). Emotional interdependence and well-being in close relationships. Frontiers in Psychology, 7, 283. https://doi.org/10.3389/fpsyg.2016.00283
- Sheehan, D. V., Lecrubier, Y., Sheehan, K. H., Amorim, P., Janavs, J., Weiller, E., Hergueta, T., Baker, R., & Dunbar, G. C. (1998). The Mini-International Neuropsychiatric Interview (MINI): The development and validation of a structured diagnostic psychiatric interview for DSM-IV and ICD-10. Journal of Clinical Psychiatry, 59(20), 22–33.
- Sloan, D. M., & Kring, A. M. (2007). Measuring changes in emotion during psychotherapy: Conceptual and methodological issues. Clinical Psychology: Science and Practice, 14(4), 307. https://doi.org/10.1111/j.1468-2850.2007.00092.x
- Sutton, S., Braren, M., Zubin, J., & John, E. R. (1965). Evoked-potential correlates of stimulus uncertainty. Science, 150(3700), 1187–1188. https://doi.org/10.1126/science.150.3700.1187
- Tal, S., Bar-Kalifa, E., Kleinbub, J. R., Leibovich, L., Deres-Cohen, K., & Zilcha-Mano, S. (2023). A multimodal case study utilizing physiological synchrony as indicator of context in which motion synchrony is associated with the working alliance. Psychotherapy, 60(1), 86. https://doi.org/10.1037/pst0000465
- Tasca, G. A., & Gallop, R. (2009). Multilevel modeling of longitudinal data for psychotherapy researchers: I. The basics. Psychotherapy Research, 19(4–5), 429–437. https://doi.org/10.1080/10503300802641444
- Terhürne, P., Schwartz, B., Baur, T., Schiller, D., Eberhardt, S. T., André, E., & Lutz, W. (2022). Validation and application of the non-verbal behavior analyzer: An automated tool to assess non-verbal emotional expressions in psychotherapy. Frontiers in Psychiatry, 13, Article 1026015. https://doi.org/10.3389/fpsyt.2022.1026015
- Tourunen, A., Kykyri, V. L., Seikkula, J., Kaartinen, J., Tolvanen, A., & Penttonen, M. (2020). Sympathetic nervous system synchrony: An exploratory study of its relationship with the therapeutic alliance and outcome in couple therapy. Psychotherapy, 57(2), 160. https://doi.org/10.1037/pst0000198
- Tschacher, W., & Meier, D. (2020). Physiological synchrony in psychotherapy sessions. Psychotherapy Research, 30(5), 558–573. https://doi.org/10.1080/10503307.2019.1612114
- Van Rossum, G., & Drake, F. L. (2009). Python 3 reference manual. CreateSpace.
- Yokotani, K., Takagi, G., & Wakashima, K. (2020). Nonverbal synchrony of facial movements and expressions predict therapeutic alliance during a structured psychotherapeutic interview. Journal of Nonverbal Behavior, 44(1), 85–116. https://doi.org/10.1007/s10919-019-00319-w
- Zilcha-Mano, S., Dolev, T., Leibovich, L., & Barber, J. P. (2018). Identifying the most suitable treatment for depression based on patients’ attachment: Study protocol for a randomized controlled trial of supportive-expressive vs. supportive treatments. BMC Psychiatry, 18(1), 1–9. https://doi.org/10.1186/s12888-018-1934-1
- Zilcha-Mano, S., Goldstein, P., Dolev-Amit, T., Ben David-Sela, T., & Barber, J. P. (2021). A randomized controlled trial for identifying the most suitable treatment for depression based on patients' attachment orientation. Journal of Consulting and Clinical Psychology, 89(12), 985.