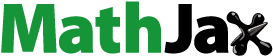
ABSTRACT
Changes in a company’s pricing strategy have the potential to influence profitability. Data from choice-based conjoint questionnaire were used to estimate revenue-maximizing dynamic prices accounting for both demand shifting and market expansion. The results show that dynamic prices induce greater demand and could increase revenue by 0.5% to 7.5%. Moreover, skiers have a strong preference and higher willingness-to-pay for good weather-related skiing conditions, suggesting that skiers prefer skiing during the midweek at a higher price, instead of on the weekend, if the skiing conditions are better in the midweek. Relevant implications are provided for practitioners.
Introduction
Changes in a company’s pricing strategy have the potential to influence profitability more than any other instrument of the marketing mix (Liozu et al., Citation2012). In the tourism industry, the first signs of an innovative approach to pricing can be seen in airline deregulation that occurred in the late 1970s. Until the 1980s, airline tickets were essentially sold like peanut butter: one product (i.e. one route), one price. Revenue management revolutionized airline ticket pricing and contributed to increasing the profitability of the industry, by several hundred million US$ per year (Cross, Citation1997). Revenue management – matching supply with demand by using dynamic pricing – is now practiced in a large number of other industries as well (Hinterhuber & Liozu, Citation2014): tourism (hotels, rental cars companies, cruise lines), leisure services (restaurants, golf courses, sport clubs, entertainment venues), industrial services (freight transportation, advertising time), consumer services (equipment rental), and, finally, in industrial manufacturing (chip manufacturing). As of this writing, several ski resorts in Switzerland and the US have begun to implement dynamic pricing. Such pricing is risky: revenues could collapse if large numbers of customers with a high willingness-to-pay (WTP) switch to lower-priced offers; revenues could increase if customers who would otherwise not have consumed are drawn into the market.
Clearly, managers and researchers need data on the expected benefits related to changes in a company’s pricing strategy. Surprisingly, the revenue management literature is largely silent on quantifying the effects of dynamic pricing strategies primarily due to the complexity of obtaining data (Abrate et al., Citation2019). Consulting companies publicize services in price consultancy by promising quick returns (Liozu & Hinterhuber, Citation2014) without providing the data that would allow independent verification. In the first textbook on revenue management, Cross (Citation1997) proposes that the implementation of dynamic pricing increases revenues by 3% to 7%. This figure is widely quoted in the revenue management literature (Skugge, Citation2004), yet empirical studies on the consequences of introducing dynamic pricing are, as of today, scarce.
In this context, the present study focuses on alpine skiing industry that has a potential for implementing more dynamic pricing practices (Berman, Citation2005), but have received little attention both from academics and practitioners (Denizci Guillet & Mohammed, Citation2015). Most ski resorts charge a constant price for various ski lift ticket passes over the entire winter season, but provide group pricing, different prices to children, adults and senior citizens. However, in today’s very competitive environment and demographics, the traditional pricing approaches, such as cost- and competition-based pricing, are no longer sufficient to ensure profitable alpine skiing businesses (Hinterhuber & Liozu, Citation2014; Vanat, Citation2020). Although the dynamic pricing has been lately implemented in some ski resorts in Europe with great success (see for instance, Arosa Lenzerheide in Switzerland), it is still viewed with a touch of scepticism and not yet widely adopted (Vanat, Citation2020).
Alpine skiing is a service offering that cannot be stored for future consumption, and demand is continuously fluctuating. Moreover, the weather conditions have a significant influence on the alpine skiing experience and consequently also the number of visitors on a particular day (Malasevska et al., Citation2017b); therefore, we choose to examine how the implementation of dynamic pricing that is based on weather- and weekday-related factors affects the ski resort’s revenue and demand. Accordingly, we address the following research questions:
- What are the revenue-maximizing prices for various skiing day scenarios?
- What is the share of high WTP customers who will switch to lower-price offers in case of dynamic pricing?
- What is the share of new customers, who otherwise would not have visited, attracted by the dynamic pricing?
We apply the choice-based conjoint (CBC) method to estimate skiers’ choices from among relevant skiing-day scenarios. Although this method is able to forecast customer choices in a way that is very similar to the actual marketplace, it has not been widely used in previous research on demand- and price-modelling in the skiing industry. An additional advantage of the CBC method is that it allows us to model the demand-shifting fraction directly. Finally, this method also enables us to evaluate the role of dynamic prices in generating new demand and additional revenue. The questionnaire we used was developed based on insights from previous research and from qualitative interviews with active skiers.
To sum up, we use data from a choice-based conjoint survey collected among skiers in the inland region of Norway to provide new empirical evidence of the impact of dynamic pricing on a company’s financial performance. Managers of ski resorts can use the insights presented here to increase profitability by segmenting visitors by their perceived value of various skiing days.
The rest of the article is organized as follows. The next sections provide a brief overview of the literature and introduce the methodology. The final sections present the empirical results and give the conclusion and managerial implications arising from our findings.
Literature review
The major part of research regarding dynamic pricing and revenue management has been done within traditional revenue management industries, such as airlines, hotels, and rental car companies (Denizci Guillet & Mohammed, Citation2015; Wang et al., Citation2015). Nevertheless, a number of studies have covered the non-traditional industries, such as attractions (e.g., Heo & Lee, Citation2009; Hoseason, Citation2006; Leask et al., Citation2013, Citation2000), national parks (e.g., Mejía & Brandt, Citation2015; Schwartz et al., Citation2012), cruise and ferry lines (e.g., Hoseason, Citation2000; Li et al., Citation2014; Namin et al., Citation2020; Sun et al., Citation2011), resorts (e.g., Pinchuk, Citation2006), golf clubs (e.g., Licata & Tiger, Citation2010; Noone et al., Citation2019), entertainment (e.g., Baldin & Bille, Citation2018; Baldin et al., Citation2018; Choi et al., Citation2015; Volpano, Citation2003), and sporting events (e.g., Barlow, Citation2000; Courty & Davey, Citation2020; Drayer et al., Citation2012; Shapiro & Drayer, Citation2012, Citation2014; Shapiro et al., Citation2016).
Comparatively, pricing research within the alpine skiing industry has received little academic attention. The study by Perdue (Citation2002) is one of the first that examines revenue management in the context of alpine skiing. The author studies the effect of the introduction of heavily discounted ski season passes (75% discount) on skier behaviour and concludes that such a discount program has a positive effect on skiers’ loyalty (days spent at the respective ski resort) as well as on overall ski resort revenue. Holmgren and McCracken (Citation2014) investigate the effect price discrimination has on demand for different groups of skiers (half-day, local, multiday, college, season pass, and student) at one ski resort. The results indicate that half-day skiers and students are more price-sensitive and are likely to switch to a nearby ski resort to obtain a discount. Holmgren et al. (Citation2016) examine price discrimination at a ski resort from season pass perspective and suggest that skiers who have purchased the season pass will ski enough times to receive at least a 50% discount than the daily ticket price. Falk and Scaglione (Citation2018) find that the introduction of the reduced seasonal ski pass price leads to a notable increase in domestic overnight stays in Swiss winter sports destinations. Still, the findings of Falk and Scaglione (Citation2020) emphasize that the positive effect of discounted season passes has to be perceived critically as it could be temporary until the competitors do not introduce a similar pricing practice.
Regarding one-day ski passes, empirical pricing studies have been conducted by Malasevska and Haugom (Citation2018) and Malasevska et al. (Citation2017b). Both studies have used contingent valuation method to estimate WTP in alpine skiing industry. Malasevska and Haugom (Citation2018) estimate optimal prices for ski-lift tickets for various weekdays and concluded that lower prices for midweek days (Monday to Friday) could lead to a substantial increase in the number of visitors and in total revenue of the ski resort. Malasevska et al. (Citation2017b) find that ski resort revenues could be increased by offering weather-related discounts when skiing quality is reduced because of unpleasant weather conditions. There are some previous studies that have applied conjoint analysis (rating-based) to estimate perceived value of ski resort qualities (e.g., price, accessibility, the number and variability of ski slopes, weather condition) (see e.g., Carmichael, Citation1996; Haugom & Malasevska, Citation2019; Haugom et al., Citation2020; Siomkos et al., Citation2006; Won et al., Citation2008; Won & Hwang, Citation2009). Recently, Haugom et al. (Citation2020) examine the profit-maximizing one-day ski pass prices for various capacity levels at a ski resort, as well as for various waiting lines at the main ski lift based on a conjoint framework (rating based).
Despite this emerging interest in innovative pricing in the alpine skiing industry, no one has, to the best of our knowledge, controlled for demand shifting or incorporated weather- and weekday-related factors simultaneously in their scenarios for a skiing day when estimating skiers’ WTP and optimal dynamic prices for a ski lift ticket for a particular day. The present paper aims to fill this gap and to further develop research done by Malasevska and Haugom (Citation2018) and Malasevska et al. (Citation2017b) using the CBC method. The CBC method is widely used in marketing studies concerning optimal design and pricing of new products, selecting a target market, as well as competitive reactions (see e.g., Rao, Citation2014). However, there is a lack of studies using the CBC method within the alpine skiing industry, and particularly in pricing.
Methodology
Estimation of willingness-to-pay with discrete-choice analysis
There are various methods for estimating consumers’ WTP (see e.g., Breidert et al., Citation2006; Miller et al., Citation2011). Although all these methods have advantages and disadvantages, the indirect survey approaches such as conjoint and discrete-choice analysis (also known as CBC analysis or the stated-choice experimental method) are usually preferred. Because, first, CBC models imitate real-world market situations, and second, the discrete choice is based on well-accepted utility-maximization concepts in economic theory (Paczkowski, Citation2018).
The CBC approach allows us to predict customer choices in a way that is very similar to the actual marketplace (Ben-Akiva & Lerman, Citation1991; Rao, Citation2014, p. 128). The choice theory assumes that an individual acts rationally so that the utility of the alternative chosen exceeds the utility of all other alternatives (e.g., various scenarios for a skiing day) under consideration (Rao, Citation2014, p. 129). Each of the alternatives has a price, and each potential customer has a vector of WTP, one for each alternative. Different WTPs represent the different monetary values that consumers assign to the attribute levels associated with each alternative – a more desirable alternative will have a higher associated WTP (Phillips, Citation2005).
The utility function of a chosen alternative can be defined as follows:
where is a function of the attribute levels of the alternative
is a random error term (attributes that cannot be perfectly measured),
is a vector of attribute levels of the alternative
, and
is a vector of estimated coefficients that are weights associated with each attribute level.
Accordingly, assuming that follows an extreme-value distribution (McFadden, Citation1973), the choice probability (also called preference-share or market share)Footnote1 obtained by an alternative i among a set of j alternatives can be defined by the following logit model:
In other words, the probability of choosing alternative is a function of both the attribute levels of alternative
and the attribute levels of all other alternatives under consideration.
In a real-life situation, consumers have the option to refrain from purchase; therefore, a none option should be included in the choice sets. The none option does not have a systematic utility, since it has no attributes. Accordingly, EquationEquation 2(2)
(2) has to be slightly modified to handle a none alternative by adding a “1” to the denominator (Paczkowski, Citation2018). This is equivalent to adding a systematics utility component that is zero, since
. The preference-share rates including a none alternative are calculated as follows:
A preference-share function has the following characteristics: (1) the preference share of each alternative is between 0 and 1, (2) every consumer chooses some alternative, (3) an increase of a product price decreases its preference share, and (4) an increase in a product price leads to an increase of the preference share of its substitute product (Phillips, Citation2005).
Accordingly, to estimate the price-response function for a particular alternative, we use market simulations to generate relative preference-share curves. This approach involves holding all other alternatives and their attribute levels (including price) constant while changing the price of a single alternative and recording the relative preference share at different price points for that particular alternative. Moreover, such discrete-choice simulations can reveal differential substitutability (e.g., demand shifting) between different features of an alternative (Orme, Citation2010). For ski resorts, various alternatives are substitutable to some degree and therefore may cause demand shifting – also called cannibalization (Meredith & Maki, Citation2001; Phillips, Citation2005). Demand shifting can be defined as a market phenomenon whereby a less desirable skiing day at a lower price takes the market share from a more desirable skiing day at a higher price.
Given a preference-share function and a total demand D, the quantity of an alternative i demanded is given by
Price optimization
Dynamic pricing is a pricing strategy whereby the price of a good or service varies based on different factors. Assuming that each customer has a separate WTP for each product alternative (i.e. skiing day under various weather-related scenarios), it is expected that a customer would compare their WTP with the given price and purchase the alternative that provides the highest utility. The art of price differentiation lies in finding a way to divide the market into different segments such that higher prices can be charged for more desirable products or services and lower prices for less desirable products or services to improve a company’s profitability (Phillips, Citation2005).
If we assume that demand for different product alternatives (i.e. skiing days under various weather-related scenarios) at a ski resort are independent and that a ski resort has a marginal cost of zero per customer, the optimal ski-pass prices can be estimated by solving independent optimization problems for each product alternative (Phillips, Citation2005):
subject to
where is the optimal price for alternative
and
is the price-response function for alternative
, and C is the total daily capacity of the ski resort. summarizes the price optimization process using data from CBC questionnaire.
Survey design
In this study we combined qualitative and quantitative research methods. First, we conducted face-to-face semi-structured interviews with 32 active skiers and 12 potential skiers to gain insight on what are considered the most important attributes of a desirable alpine skiing experience (see Appendix A). Interviews took place in January 2019 at various resorts in Norway and Austria. Then based on these qualitative findings and review of previous studies in the alpine skiing industry (e.g., Falk, Citation2015; Holmgren & McCracken, Citation2014; Klenosky et al., Citation1999; Konu et al., Citation2011; Malasevska et al., Citation2017a; Won et al., Citation2008) we created a CBC questionnaire that simulates the real decision-making process. The attributes and attribute levels included in CBC questionnaire are presented in .
Table 1. Attributes and attribute levels
Because attributes with more levels tend to achieve higher importance than those with fewer levels (Wittink et al., Citation1990), the final CBC survey design included almost the same number of levels for each attribute. After choosing the attributes and attribute levels, we use orthogonal main effect plan to generate the hypothetical skiing day alternatives for evaluation by respondents. Orthogonal design controls that each level of one attribute occurs with each level of another attribute with proportional frequencies to ensure statistical efficiency (Rao, Citation2014). An orthogonal array for our study with 5 attributes with 4 levels and 1 attribute with 3 levels consists of 48 profiles.
Choice sets were designed using the shifting method that ensures statistical efficiency (for more details see Rao, Citation2014, p. 140). A total of 48 choice sets were created and divided into six questionnaires consisting of eight different choice sets each, because 48 choice sets are too many to be included in one questionnaire (see Appendix B). Thus, each respondent had to evaluate only eight choice sets. Each choice set contained three different scenarios in addition to the no-purchase option (see Appendix C for an example of one CBC question). Respondents were asked to assume that they were in an actual situation buying of a ski pass where they had to choose between different hypothetical scenarios of a skiing day or option not to buy. The no-purchase option was included because it allows us to estimate the purchase threshold (Gensler et al., Citation2012) and renders the experimental setting more realistic (Parker & Schrift, Citation2011). When pre-defining the price levels, we took into account that the price for a one-day ski-lift ticket at Norwegian ski resorts ranges from NOK 250 at very small ski resorts to NOK 495 at the largest ski resorts for winter season 2018/2019. Accordingly, the price levels included in the questionnaire can be defined as realistic from a managerial point of view (Rao, Citation2014, p. 44), and they also overlap the consumers’ WTP range and help prevent extreme response behaviour by respondents (Gensler et al., Citation2012). Extreme response behaviour occurs if the price is too high and the respondent always chooses the no-purchase option because this alternative provides the highest consumer surplus. At the same time, if the price is too low, the respondent will never choose the no-purchase option, because the consumer surplus is higher for choosing a product alternative at a very low price. The questionnaire was specifically designed to be answered online using a computer, tablet, or mobile device. In addition, respondents were informed that choice experiment is valid for regular weeks and not for holiday weeks.
Before conducting the final survey, we pre-tested a web-based pilot survey collecting answers from 95 respondents. The main purpose of the pilot survey was to ensure that respondents were not overly burdened by providing preference judgements on different profiles (Johnson & Orme, Citation1996) and that the questions were clear and understandable. Based on respondents’ feedback, we changed the verbal description of the ski resorts’ size attributes (e.g., total length of ski slopes and share of different-difficulty slopes) to a visual presentation (ski maps) of three Norwegian ski resorts of different sizes. This made it possible for respondents to quickly see the similarities and differences between resorts. Additionally, we illustrated weather-related-attribute levels with symbols commonly used by meteorologists in weather forecast reports and added a photorealistic representation of crowdedness level to more realistically represent a hypothetical scenario (Jansen et al., Citation2009). There are both advantages and disadvantages, however, to adding visual illustrations to a conjoint analysis. A picture can generate a more realistic representation of the alternatives to be chosen but may also de-emphasize attributes that are described textually (Jansen et al., Citation2009). Therefore, we combined pictures with symbols and short texts to compensate for the potential loss of focus.
Sampling and data collection and analysis
The data were collected online among skiers in the inland region of Norway from February 4 to March 17 2019. Survey respondents were recruited via (1) face-to-face interviews, (2) social media (e.g., Facebook and Instagram), and (3) flyers accessible at the ski resorts. A total of 722 survey forms were completed. For analysis we used only data obtained from respondents who were 18 years old or older, who were interested in alpine skiing, and who did not possess a seasonal ski pass. A total of 415 respondents met these criteria. Approximately 89% of respondents indicated that they usually purchase a one-day ski pass. Each respondent was asked eight choice experiment questions, and the sample size was calculated by multiplying the number of respondents by the number of questions per respondent (see e.g., Hensher et al., Citation2005). The sample size of 3,320 (415 × 8 = 3,320) in this study fulfils the minimum requirements to ensure reliable results of the CBC analysis (Orme, Citation2010). presents the background variables of the sample.
Table 2. Sample characteristics (n = 415)
In this study, we focused on the effect of characteristics (levels) of the alternatives rather than the characteristics of the individuals making the choice. Alternatives are assumed to be distinct and weighted independently in the eyes of potential skiers. Accordingly, a conditional logit model that is based on the random utility theory was used for data analysis (McFadden, Citation1973). In our case, we found that the alternative levels “Monday–Wednesday” and “Thursday–Friday” as well as “Saturday” and “Sunday” were in close proximity on a preference scale. Therefore, we nested them in two groups: (1) midweek days (Monday–Friday) and (2) weekend days (Saturday–Sunday) to avoid overestimating the attribute levels of individual weekdays (Paczkowski, Citation2018). Additionally, the price and size of the ski resort (the total length of ski slopes) attributes were included in the model as continuous variables, as the Akaike’s information criterion (AIC) and the Bayesian information criterion (BIC) indicated that such a model fits the data best.
Results and discussion
Results from the estimation using the conditional logit model are presented in Appendix D and indicate that all attributes are statistically significant and that all attribute levels have the expected sign. Based on the estimated parameters, we calculated how much an average visitor would be willing to pay for the selected level of an attribute. In other words, the estimated marginal WTP shows how a change in price would cause an equivalent shift in utility due to a change in the level of the attribute in question from the reference level. presents the marginal WTP for a particular level of an attribute. We see that an average consumer would be indifferent between skiing on a sunny day and paying approximately NOK 133 less and skiing on a rainy day. In the case of different weekdays, we see that an average consumer would be willing to pay up to approximately NOK 51 to ski on a weekend instead of a midweek day, holding all other characteristics fixed. The results also indicate that skiers are willing to pay approximately NOK 4 for each additional km of ski slope.
Table 3. Marginal WTP for a particular level of an attribute
For further analysis, we incorporated weather- and weekday-related factors in four various week scenarios at two ski resorts that differ in size in order to estimate how skiers’ WTP differ among various skiing day alternatives. In this study, we chose to focus only on two possible weather conditions that we define as desirable and undesirable weather conditions for a skiing day. Based on findings by Haugom and Malasevska (Citation2019) and information obtained from the semi-structured interviews with skiers we defined desirable skiing day as a sunny day with no wind and the air temperature around minus two degrees Celsius. Undesirable skiing day is defined as a day with precipitations, moderate wind, and the air temperature around five degrees Celsius. In addition, we assume that all four scenarios are for regular weeks and the slopes at ski resorts are a little crowded.
Further, the estimated attribute coefficients (Appendix D) is used to predict how customers would choose among those skiing day alternatives for each week scenario at a fixed one-day ski pass price (for more details about conjoint market simulations see Orme (Citation2010)). The ski resorts in inland Norway use fixed price for a one-day ski-lift ticket and the average market price at these ski resorts for winter season 2018/2019 was NOK 425. Therefore in this study we chose to use this average ski pass price as a reference price to illustrate the share of preferences for each scenario.
The results of the predicted preference shares at one fixed ski pass price () is consistent with previous studies (see e.g., Holmgren & McCracken, Citation2014; Malasevska et al., Citation2017a) and show that in the case of the same weather conditions during the midweek and the weekend, skiers tend to choose to visit a ski resort on the weekend. However, if the weather conditions are better on midweek days, consumers tend to choose midweek days over weekends to ensure a better skiing experience. This substantiates previous findings by Dawson et al. (Citation2011) that if there are insufficient weather-related skiing conditions are present and that skiing on a particular day will not be worthwhile, skiers, especially frequent visitors, are likely to change their skiing behaviour by choosing to ski on another day or replacing skiing with another leisure activity. Moreover, when bad weather conditions are expected during the whole week (scenario 2), the share of respondents who will choose not to visit the ski resort at all is larger when compared to other scenarios that emphasizes the strong link between demand and weather conditions. The results of the predicted preference shares hold true both for a relatively small and large ski resorts.
Table 4. The predicted share of preferences at NOK 425
In order to estimate a price response function for each alternative, we calculated the preference shares for a given alternative in a given scenario under various price levels while holding the price level for other alternatives constant at an average market price. Following the suggestion of Bodea and Ferguson (Citation2014) and Phillips (Citation2005) we focus on the logit price-response functional form that has a reversed-S shape and reflects real customer behaviour in a reasonable way. At low price levels, demand is expected to be a very high and changes slowly as the price increases. The demand is very sensitive to price around the so-called “market price” when small changes in market price can lead to significant changes in demand. As price increases past the market price, the demand curve flattens again and changes slowly at high prices. lists the price elasticities at average market price NOK425 for each scenario, indicating that we should expect customers to react differently to change in price at various scenarios and that one fixed ski-lift ticket price for a whole season is not optimal. Appendix E summarizes the parameters of the estimated price-response functions.
Table 5. The estimated price elasticities at NOK 425
The estimated price response functions allow us to calculate revenue-maximizing prices for each skiing day scenario. present the results of the price optimization for various regular week scenarios in the case of dynamic prices. In addition, using market simulation, we estimated the approximate percentage of demand shifting and market expansion for each scenario. Results suggest that revenue-maximizing prices for undesirable weather conditions are significantly lower than for good weather conditions at both ski resorts. This corresponds to the findings of Malasevska et al. (Citation2017b). Compared with the actual average price (NOK 425), we see that dynamic prices provide an opportunity to generate additional revenue even in the case of demand shifting to lower prices. Previous research has shown that demand tends to be higher during the weekends (e.g., Holmgren & McCracken, Citation2014) and that optimal prices should therefore be higher during these peak periods (Malasevska & Haugom, Citation2018). However, our findings indicate that if midweek weather conditions are noticeably better than what is forecast for the weekend, customers tend to choose midweek days instead of weekend days, even if the price for the former is higher. In turn, if the weather conditions are better on the weekend, and in the case of an actual average price for ski-lift tickets (NOK 425), approximately half the potential customers choose to visit the ski resort on the weekend. This situation could cause overcrowded ski slopes and/or long waiting lines, reducing customer satisfaction (see e.g., Fonner & Berrens, Citation2014; Zehrer & Raich, Citation2016). These results suggest that implementing dynamic pricing could reduce overall crowdedness on the most desirable weekend days by shifting more price-sensitive customers to cheaper and less desirable days and simultaneously increase revenues (see scenario 4 in ).
Table 6. Revenue-maximizing dynamic prices for various scenarios at a small ski resort with a total slope length of 10 km
Table 7. Revenue-maximizing dynamic prices for various scenarios at a large ski resort with a total length of slopes of 50 km
For comparison, we followed (Malasevska & Haugom, Citation2018) and estimated profit-maximizing variable prices taking into account only various days of the week (see ). The findings emphasize that variable pricing based only on time-related variables induces a lower increase in revenues than when weather-related variables are incorporated in pricing decisions: a 0.6% to 1.3% increase and a 0.5% to 7.5% increase, respectively. In addition, demand shifting and the market-expansion rate for such intra-weekly variable pricing are lower than in the more complex dynamic-pricing approach.
Table 8. Revenue-maximizing variable prices for various weekdays
Conclusion and managerial implications
The decision to visit a ski resort is based mostly on visitor preferences and availability of time for skiing activities. The results of CBC analysis suggest that dynamic pricing opens an innovative way for ski resorts to improve their profitability and to attract new customers because customers’ WTP differs among various weather- and time-related skiing-day scenarios, with a great preference for good weather conditions when skiing. In addition, our results complement previous studies by Malasevska and Haugom (Citation2018) and Malasevska et al. (Citation2017b) with new empirical evidence that dynamic-pricing decisions that simultaneously incorporate multiple attributes that are important for consumers provide better outcomes. This study, therefore, makes an important contribution to the literature and lends support to a widely quoted proposal by Cross (Citation1997) that more dynamic pricing can increase a company’s revenue by 0.5% to 7.5%.
Bendle et al. (Citation2016) have emphasized that any company considering introducing a more dynamic pricing strategy should estimate the amount of potential cannibalization beforehand to determine how the pricing strategy should be adjusted. If the price difference between desirable and less desirable products or services becomes too great, increasing numbers of high-WTP customers could switch to cheaper products or services and could, therefore, exceed the benefits of less desirable demand induction and higher prices for more desirable products or services. Our findings suggest that the fraction of higher-WTP skiers who choose to buy at lower prices in the case of dynamic pricing is greater when the difference in ski-lift ticket prices is larger due to significant variance in weather conditions between midweek and weekend. In such cases, the market-expansion rate is higher as well. Moreover, if the midweek weather conditions are noticeably better than those forecast for the weekend, customers tend to choose midweek days instead of the weekend, even if the midweek price is higher. Awareness of such a customer reaction allows ski resorts to use a dynamic-pricing approach to shift demand from more desirable periods to less desirable periods and to reach a new group of potential skiers.
Ski resorts could use CBC data to segment the market into market segments that have similar preferences. However, given that skiers’ preferences are not evenly distributed, ski resort managers have to find effective ways to communicate the value of a skiing experience on a particular day based on customer needs and preferences and understandably justify why prices vary among the various days. Because customers claim to prefer easily understandable pricing schemes that are potentially available for all (see e.g., Choi et al., Citation2015; Kimes & Wirtz, Citation2003; Lynn, Citation1990).
The results presented in this paper are rather exploratory and a simplification of reality. Stated preferences can often overstate the actual situation (Schmidt & Bijmolt, Citation2019). For instance, customers might state that they would visit a ski resort if prices were lower. In reality, however, as a result of other factors unobserved in this study (for instance, holiday time), customers might possibly make a choice that differs from the predicted. Future research should examine the revealed preferences by using field experiments, as well as accounting for seasonality and a possible reaction of competitors.
Additional research that examines how the implementation of dynamic prices for one-day ski passes affects the sale of season passes would be beneficial and provide more detailed explanations and understanding of the overall impact dynamic pricing on ski resort’s financial performance. Possibly many of the season pass holders that are not frequent skiers would consider purchasing one-day ski passes at lower prices instead of the season pass. Because skiers usually purchase a season pass with the belief that the total cost will be less expensive than the purchase of one-day ski passes over the entire season (Holmgren et al., Citation2016).
Furthermore, this study is restricted to one country and cannot be generalized to ski resorts in other countries, as ski resorts vary widely in their characteristics. Therefore, future studies may be extended to other countries.
Acknowledgments
We acknowledge Torgeir Vikane Stemsverk for creating the images for attribute levels used in the conjoint survey.
Disclosure statement
No potential conflict of interest was reported by the authors.
Additional information
Funding
Notes
1. In this article, we use the term “preference shares” (as suggested in Chapman and Feit (Citation2015)) instead of “market shares” because we estimate the predicted shares based on survey data rather than real retail data; therefore, estimates might not translate directly to sales in the marketplace.
References
- Abrate, G., Nicolau, J. L., & Viglia, G. (2019). The impact of dynamic price variability on revenue maximization. Tourism Management, 74, 224–233. https://doi.org/10.1016/j.tourman.2019.03.013
- Baldin, A., & Bille, T. (2018). Modelling preference heterogeneity for theatre tickets: A discrete choice modelling approach on Royal Danish Theatre booking data. Applied Economics, 50(5), 545–558. https://doi.org/10.1080/00036846.2017.1335386
- Baldin, A., Bille, T., Ellero, A., & Favaretto, D. (2018). Revenue and attendance simultaneous optimization in performing arts organizations. Journal of Cultural Economics, 42(4), 677–700. https://doi.org/10.1007/s10824-018-9323-7
- Barlow, G. L. (2000). Capacity management in the football industry. In A. Ingold, U. McMahon-Beattie, & I. Yeoman (Eds.), Yield management: Strategies for the service industries (pp. 303–314). Continuum.
- Ben-Akiva, M., & Lerman, S. R. (1991). Discrete choice analysis. The MIT press.
- Bendle, N., Farris, P., Pfeifer, P., & Reibstein, D. (2016). Marketing metrics: The manager’s guide to measuring marketing performance (3rd ed.). Pearson Education, Inc.
- Berman, B. (2005). Applying yield management pricing to your service business. Business Horizons, 48(2), 169–179. https://doi.org/10.1016/j.bushor.2004.10.015
- Bodea, T., & Ferguson, M. (2014). Segmentation, revenue management and pricing analytics. Routledge.
- Breidert, C., Hahsler, M., & Reutterer, T. (2006). A review of methods for measuring willingness-to-pay. Innovative Marketing, 2(4), 8–32.
- Carmichael, B. A. (1996). Conjoint analysis of downhill skiers used to improve data collection for market segmentation. Journal of Travel & Tourism Marketing, 5(3), 187–206. https://doi.org/10.1300/J073v05n03_02
- Chapman, C., & Feit, E. M. (2015). R for marketing research and analytics. Springer International Publishing.
- Choi, C., Jeong, M., & Mattila, A. S. (2015). Revenue management in the context of movie theaters: Is it fair? Journal of Revenue and Pricing Management, 14(2), 72–83. https://doi.org/10.1057/rpm.2014.30
- Courty, P., & Davey, L. (2020). The impact of variable pricing, dynamic pricing, and sponsored secondary markets in major league baseball. Journal of Sports Economics, 21(2), 115–138. https://doi.org/10.1177/1527002519867367
- Cross, R. G. (1997). Revenue management: Hard-core tactics for market domination. Broadway Books.
- Dawson, J., Havitz, M., & Scott, D. (2011). Behavioral adaptation of alpine skiers to climate change: Examining activity involvement and place loyalty. Journal of Travel & Tourism Marketing, 28(4), 388–404. https://doi.org/10.1080/10548408.2011.571573
- Denizci Guillet, B., & Mohammed, I. (2015). Revenue management research in hospitality and tourism: A critical review of current literature and suggestions for future research. International Journal of Contemporary Hospitality Management, 27(4), 526–560. https://doi.org/10.1108/IJCHM-06-2014-0295
- Drayer, J., Shapiro, S. L., & Lee, S. (2012). Dynamic ticket pricing in sport: An agenda for research and practice. Sport Marketing Quarterly, 21(3), 184–194. http://search.ebscohost.com.ezproxy.inn.no/login.aspx?direct=true&db=s3h&AN=82306237&site=ehost-live.
- Falk, M. (2015). The demand for winter sports: Empirical evidence for the largest French ski-lift operator. Tourism Economics, 21(3), 561–580. https://doi.org/10.5367/te.2013.0366
- Falk, M., & Scaglione, M. (2018). Effects of ski lift ticket discounts on local tourism demand. Tourism Review, 73(4), 480–491. https://doi.org/10.1108/TR-08-2017-0133
- Falk, M., & Scaglione, M. (2020). Effects of price model copycats in the ski industry. Tourism Analysis, 25, 2–3. https://doi.org/10.3727/108354220X15950120083867
- Fonner, R. C., & Berrens, R. P. (2014). A hedonic pricing model of lift tickets for US alpine ski areas: Examining the influence of crowding. Tourism Economics, 20(6), 1215–1233. https://doi.org/10.5367/te.2013.0338
- Gensler, S., Hinz, O., Skiera, B., & Theysohn, S. (2012). Willingness-to-pay estimation with choice-based conjoint analysis: Addressing extreme response behavior with individually adapted designs. European Journal of Operational Research, 219(2), 368–378. https://doi.org/10.1016/j.ejor.2012.01.002
- Haugom, E., & Malasevska, I. (2019). The relative importance of ski resort- and weather-related characteristics when going alpine skiing. Cogent Social Sciences, 5(1), 1681246. https://doi.org/10.1080/23311886.2019.1681246
- Haugom, E., Malasevska, I., & Lien, G. (2020). Optimal pricing of alpine ski passes in the case of crowdedness and reduced skiing capacity. Empirical Economics. https://doi.org/10.1007/s00181-020-01872-w
- Hensher, D. A., Rose, J. M., & Greene, W. H. (2005). Applied Choice Analysis: A Primer. Cambridge University Press.
- Heo, C. Y., & Lee, S. (2009). Application of revenue management practices to the theme park industry. International Journal of Hospitality Management, 28(3), 446–453. https://doi.org/10.1016/j.ijhm.2009.02.001
- Hinterhuber, A., & Liozu, S. (2014). Is innovation in pricing your next source of competitive advantage? Business Horizons, 57(3), 413–423. https://doi.org/10.1016/j.bushor.2014.01.002
- Holmgren, M. A., & McCracken, V. A. (2014). What affects demand for “the greatest snow on earth?”. Journal of Hospitality Marketing & Management, 23(1), 1–20. https://doi.org/10.1080/19368623.2012.746212
- Holmgren, M. A., McCracken, V. A., & McCluskey, J. J. (2016). Should I ski today? The economics of ski resort season passes. Leisure/Loisir, 40(2), 131–148. https://doi.org/10.1080/14927713.2016.1219964
- Hoseason, J. (2000). Capacity management in the cruise industry. In A. Ingold, U. McMahon-Beattie, & I. Yeoman (Eds.), Yield management: Strategies for the service industries (pp. 289–302). Continuum.
- Hoseason, J. (2006). Revenue management in visitor attractions: A case study of the EcoTech Centre, Swaffham, Norfolk. In F. Sfodera (Ed.), The spread of yield management practices (pp. 83–98). Springer.
- Jansen, S., Boumeester, H., Coolen, H., Goetgeluk, R., & Molin, E. (2009). The impact of including images in a conjoint measurement task: Evidence from two small-scale studies. Journal of Housing and the Built Environment, 24(3), 271–297. https://doi.org/10.1007/s10901-009-9149-x
- Johnson, R. M., & Orme, B. K. (1996). How many questions should you ask in choice-based conjoint studies. Paper presented at the Art Forum, Beaver Creek.
- Kimes, S. E., & Wirtz, J. (2003). Has revenue management become acceptable? Findings from an international study on the perceived fairness of rate fences. Journal of Service Research, 6(2), 125–135. https://doi.org/10.1177/1094670503257038
- Klenosky, D. B., Gengler, C. E., & Mulvey, M. S. (1999). Understanding the factors influencing ski destination choice: A means-end analytic approach. Consumer Behavior in Travel and Tourism, 25(4), 59–80. https://doi.org/10.1080/00222216.1993.11969934
- Konu, H., Laukkanen, T., & Komppula, R. (2011). Using ski destination choice criteria to segment Finnish ski resort customers. Tourism Management, 32(5), 1096–1105. https://doi.org/10.1016/j.tourman.2010.09.010
- Leask, A., Fyall, A., & Goulding, P. (2000). Revenue management in Scottish visitor attractions. In A. Ingold, U. McMahon, & I. Yeoman (Eds.), Yield management: Strategies for the service industries (2nd ed., pp. 211–232). Thomson.
- Leask, A., Fyall, A., & Garrod, B. (2013). Managing revenue in Scottish visitor attractions. Current Issues in Tourism, 16(3), 240–265. https://doi.org/10.1080/13683500.2012.667070
- Li, Y., Miao, Q., & Wang, B. X. (2014). Modeling a cruise line revenue management problem. Journal of Revenue and Pricing Management, 13(3), 247–260. https://doi.org/10.1057/rpm.2013.44
- Licata, J. W., & Tiger, A. W. (2010). Revenue management in the golf industry: Focus on throughput and consumer benefits. Journal of Hospitality Marketing & Management, 19(5), 480–502. https://doi.org/10.1080/19368623.2010.482837
- Liozu, S., & Hinterhuber, A. (2014). The ROI of pricing: Measuring the impact and making the business case. Routledge.
- Liozu, S., Hinterhuber, A., Perelli, S., & Boland, R. (2012). Mindful pricing: Transforming organizations through value-based pricing. Journal of Strategic Marketing, 20(3), 197–209. https://doi.org/10.1080/0965254X.2011.643916
- Lynn, M. (1990). Choose your own price: An exploratory study requiring an expanded view of price’s functions. Advances in Consumer Research, 17(1), 710–715. https://scholarship.sha.cornell.edu/articles/1137
- Malasevska, I., & Haugom, E. (2018). Optimal prices for alpine ski passes. Tourism Management, 64, 291–302. https://doi.org/10.1016/j.tourman.2017.09.006
- Malasevska, I., Haugom, E., & Lien, G. (2017a). Modelling and forecasting alpine skier visits. Tourism Economics, 23(3), 669–679. https://doi.org/10.5367/te.2015.0524
- Malasevska, I., Haugom, E., & Lien, G. (2017b). Optimal weather discounts for alpine ski passes. Journal of Outdoor Recreation and Tourism, 20, 19–30. https://doi.org/10.1016/j.jort.2017.09.002
- McFadden, D. (1973). Conditional logit analysis of qualitative choice behavior. In P. Zarembka (Ed.), Frontiers of econometrics,105–142. New York, NY: Academic Press.
- Mejía, C. V., & Brandt, S. (2015). Managing tourism in the Galapagos Islands through price incentives: A choice experiment approach. Ecological Economics, 117, 1–11. https://doi.org/10.1016/j.ecolecon.2015.05.014
- Meredith, L., & Maki, D. (2001). Product cannibalization and the role of prices. Applied Economics, 33(14), 1785–1793. https://doi.org/10.1080/00036840010015769
- Miller, K. M., Hofstetter, R., Krohmer, H., & Zhang, Z. J. (2011). How should consumers’ willingness to pay be measured? An empirical comparison of state-of-the-art approaches. Journal of Marketing Research, 48(1), 172–184. https://doi.org/10.1509/jmkr.48.1.172
- Namin, A., Gauri, D. K., & Kwortnik, R. J. (2020). Improving revenue performance with third-degree price discrimination in the cruise industry. International Journal of Hospitality Management, 89, 102597. https://doi.org/10.1016/j.ijhm.2020.102597
- Noone, B. M., Enz, C. A., & Canina, L. (2019). The uniqueness of revenue management approaches in nontraditional settings: The case of the Golf industry. Journal of Hospitality & Tourism Research, 43(5), 633–655. https://doi.org/10.1177/1096348019828094
- Orme, B. K. (2010). Getting started with conjoint analysis: Strategies for product design and pricing research (2nd ed.). Research Publishers, LLC.
- Paczkowski, W. R. (2018). Pricing analytics: Models and advanced quantitative techniques for product pricing. Routledge.
- Parker, J. R., & Schrift, R. Y. (2011). Rejectable choice sets: How seemingly irrelevant no-choice options affect consumer decision processes. Journal of Marketing Research, 48(5), 840–854. https://doi.org/10.1509/jmkr.48.5.840
- Perdue, R. R. (2002). Perishability, yield management, and cross-product elasticity: A case study of deep discount season passes in the Colorado ski industry. Journal of Travel Research, 41(1), 15–22. https://doi.org/10.1177/0047287502041001003
- Phillips, R. L. (2005). Pricing and revenue optimization. Stanford University Press.
- Pinchuk, S. G. (2006). Applying revenue management to palapas: Optimize profit and be fair and consistent. Cornell Hotel and Restaurant Administration Quarterly, 47(1), 84–91. https://doi.org/10.1177/0010880405283512
- Rao, V. R. (2014). Applied conjoint analysis. Springer.
- Schmidt, J., & Bijmolt, T. H. (2019). Accurately measuring willingness to pay for consumer goods: A meta-analysis of the hypothetical bias. Journal of the Academy of Marketing Science, 48(3), 499–518. https://doi-org.ezproxy.inn.no/10.1007/s11747-019-00666-6
- Schwartz, Z., Stewart, W., & Backlund, E. A. (2012). Visitation at capacity-constrained tourism destinations: Exploring revenue management at a national park. Tourism Management, 33(3), 500–508. https://doi.org/10.1016/j.tourman.2011.05.008
- Shapiro, S. L., & Drayer, J. (2012). A new age of demand-based pricing: An examination of dynamic ticket pricing and secondary market prices in Major League Baseball. Journal of Sport Management, 26(6), 532–546. https://doi.org/10.1123/jsm.26.6.532
- Shapiro, S. L., & Drayer, J. (2014). An examination of dynamic ticket pricing and secondary market price determinants in Major League Baseball. Sport Management Review, 17(2), 145–159. https://doi.org/10.1016/j.smr.2013.05.002
- Shapiro, S. L., Drayer, J., & Dwyer, B. (2016). Examining consumer perceptions of demand-based ticket pricing in sport. Sport Marketing Quarterly, 25(1), 34–46. https://doi-org.ezproxy.inn.no/10.1007/s11747-019-00666-6
- Siomkos, G., Vasiliadis, C., & Lathiras, P. (2006). Measuring customer preferences in the winter sports market: The case of Greece. Journal of Targeting, Measurement Analysis for Marketing, 14(2), 129–140. https://doi.org/10.1057/palgrave.jt.5740175
- Skugge, G. (2004). Growing effective revenue managers. Journal of Revenue & Pricing Management, 3(1), 49–61. https://doi.org/10.1057/palgrave.rpm.5170093
- Sun, X., Gauri, D. K., & Webster, S. (2011). Forecasting for cruise line revenue management. Journal of Revenue and Pricing Management, 10(4), 306–324. https://doi.org/10.1057/rpm.2009.55
- Vanat, L. (2020). International report on Snow & Mountain Tourism. Overview of the key industry figures for ski resorts (Report No. 12). https://www.vanat.ch/RM-world-report-2020.pdf
- Volpano, L. J. (2003). A proposal to rationalise entertainment ticket pricing using price discrimination. Journal of Revenue and Pricing Management, 1(4), 379–382. https://doi.org/10.1057/palgrave.rpm.5170041
- Wang, X. L., Yoonjoung Heo, C., Schwartz, Z., Legohérel, P., & Specklin, F. (2015). Revenue management: Progress, challenges, and research prospects. Journal of Travel & Tourism Marketing, 32(7), 797–811. https://doi.org/10.1080/10548408.2015.1063798
- Wittink, D. R., Krishnamurthi, L., & Reibstein, D. J. (1990). The effect of differences in the number of attribute levels on conjoint results. Marketing Letters, 1(2), 113–123. https://doi.org/10.1007/BF00435295
- Won, D., Bang, H., & Shonk, D. (2008). Relative importance of factors involved in choosing a regional ski destination: Influence of consumption situation and recreation specialization. Journal of Sport & Tourism, 13(4), 249–271. https://doi.org/10.1080/14775080802577185
- Won, D., & Hwang, S. (2009). Factors influencing the college skiers and snowboarders’ choice of a ski destination in Korea: A conjoint study. Managing Leisure, 14(1), 17–27. https://doi.org/10.1080/13606710802551197
- Zehrer, A., & Raich, F. (2016). The impact of perceived crowding on customer satisfaction. Journal of Hospitality and Tourism Management, 29, 88–98. https://doi.org/10.1016/j.jhtm.2016.06.007
Appendix A.
Appendix B.
Choice sets included in each questionnaire
Appendix C.
An example of one CBC question (text in the picture in Norwegian)
Q: Below are various ski day scenarios. The scenarios are based on different weather conditions, day in a regular week, type of resorts, amount of people on the ground and price. Choose the option you prefer. If you would not have been skiing/snowboarding in any of the scenarios, select: “None of the options (in Norwegian Ingen av alternative)
Appendix D.
Estimation results using a conditional logit model
Appendix E.
Logit price-response functions: Summary of the parameter estimates and model fit