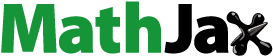
Abstract
Our paper argues that Agent Based Modelling (ABM) can play an important role in evaluating interventions for counter radicalization and recruitment. Its advantages are due to three realities of research and practice in this area of study. First, field research on radicalization and recruitment raises significant ethical and human subjects dilemmas beyond traditional criminological and social research that are not present to the same degree in ABM experiments. Second, given the lack of existing studies and especially existing evaluation studies, ABMs provide a mechanism for identifying which programs or practices should be focused upon in field experiments, thus allowing researchers to focus in on interventions which have most promise of success. Third, ABM allows researchers to examine very large populations of individuals, which can solve the base rate problem for evaluating interventions focused on rare events, for example on recruitment to terrorism or terrorist attacks. We illustrate these advantages of ABM studies for assessing programs and policies in the context of an ABM model developed in Proton (an Horizon 2020 project). We focus in particular on how ABMs can be developed in ways that provide valid outcome results. In this context, we emphasize the importance of a strong theoretical foundation for ABM studies, creating a realistic landscape with actual data, and testing the ABM model for its fit to outcomes in the real world.
Radicalization and recruitment to terrorism remain key social problems across the world. Surveys suggest that large numbers of individuals in Western countries support the use of violence in pursuit of political goals.Footnote1 In many cities between 5 and 10% of residents endorse the use of political violence,Footnote2 though the actual number of people who become terrorists and participate in violent activities remains very small.Footnote3 Even with this small number of people that move from belief to action, the threat and the harm of terrorism, and the reality of high-stakes terrorist attacks in many Western countries, has made counter radicalization programs, and programs to prevent recruitment to terrorism, a major policy focus.
While there has been very wide adoption of such programs,Footnote4 there has been little rigorous evaluation research of whether they have the desired impact of reducing radicalization and recruitment to terrorism.Footnote5 As such, counter-radicalization policies have proliferated with little scientific guidance as to what programs are most effective, or which programs have potential to cause harm. Instead, policies and practices are often more reflective of local history, politics, and social norms.Footnote6
A key question is why an area of such government interest has yielded so few rigorous outcome evaluations. One reason is that counter radicalization and recruitment programs raise strong ethical and human subjects concerns. This often begins with who is identified to receive programmatic interventions. Focusing on specific populations, especially disadvantaged minority populations, which may be most vulnerable to radicalization and recruitment, raises concerns regarding profiling and unfair labelling of racial, ethnic, or religious groups for government intervention. From the perspective of researchers, studies of interventions that target young people, who may benefit most from counter-radicalization programs, are subject to strict human subjects protections, making the research process all the more difficult. In turn, counter radicalization often involves intervening with groups that may be assessed to be high risk but who have not as yet been involved in radical activities. Gaining cooperation of representative samples and access to intervention data in such contexts is especially difficult.Footnote7
A second barrier to rigorous evaluation studies in the area of counter-radicalization and recruitment is that there are a large number of programs presently being implemented.Footnote8 Rigorous evaluation studies are expensive, and there is often relatively little funding for such work. But even with larger funding mechanisms available, it would not be possible to conduct rigorous evaluations on all such programs. Wolfowicz and colleagues analyzed 64 distinct programs presently being implemented across European countries, and found that these programs target a wide range of different risk and protective factors.Footnote9 Adding programs from the US, South America, Asia, and Africa, to this list, makes the application of rigorous evaluation to every intervention a very challenging task. Which programs should be chosen for evaluation given the limited resources available?
A final problem applies more to recruitment than the problem of radicalization. As we already noted, radicalization is fairly common across Western contexts. But recruitment is relatively rare. It is estimated that only a small number of radicalized individuals are ever recruited to terrorism.Footnote10 In practical terms this means that very large numbers of people must be studied to gain statistically powerful designs.
In this article we illustrate the use of Agent-Based Modelling (ABM) as a tool for addressing these barriers to evaluation research, and as a first stage method for developing rigorous experimental field studies. ABM is a computational model for simulating real world environments and behaviors allowing for examination of dynamic outcomes of interventions. ABM is particularly well suited to situations in which the opportunities to conduct field experiments are limited by ethical challenges.Footnote11 It is a simulation approach and therefore does not require actual human subjects to be involved. It also provides an opportunity to assess the potential for success of a large number of programs in a laboratory setting. While a simulation cannot wholly substitute for field studies, it can identify which specific programs have enough promise to warrant field experiments. Finally, ABM allows the examination of large populations. This, as noted above, is particularly important in the context of recruitment to terrorism and terrorist events, which are rare phenomena. Of course, no research approach is without limitations, and as we will discuss, ABM experiments, while providing solutions to key problems in the development of rigorous research in this area, have specific limitations. In concluding, we will argue that the combination of ABM and field experiments provides an important opportunity to advance rigorous research about outcomes of programs.
We use the example of our recent ABM model on radicalization and recruitment to terrorism developed as part of an Horizon-2020 funded Proton project.Footnote12 The Proton ABM modeled prevailing research knowledge of radicalization and recruitment and tested the effects of existing counter radicalization initiatives common in major European cities. The model included a heterogenous population, within which was embedded a small population of high-risk agents (radicalized agents) susceptible to recruitment. Agents engaged in routine activities and socialization (including both online and offline) modelled using an opinion-dynamics approach.Footnote13 In this model, routine activities shape and determine socialization opportunities and patterns, which influence risk via changes in dynamic, opinion-based risk and protective factors, as well as responsiveness to the endeavors of recruiter agents who target susceptible, high-risk agents. Below we expand on the model and highlight the advantages, as well as limitations, of ABM in modelling the complex, dynamic features of radicalization and recruitment.
Counter-Radicalization Interventions
Since the attacks on the United States of America (USA) on September 11th, 2001, the “war on terror” has featured among the top priorities of virtually all Western states. However, the nature of this war has undergone significant changes. The initial stages of the war focused primarily on military interventions overseas, and the bolstering of hard security at home. However, in the years following, Western states were witness to new developments in the terrorist threat. One development came in the form of so called “homegrown terrorists,” residents or citizens of a country who radicalized into terrorists and attacked domestically. Simultaneously, attention was turned towards the threat from so called “lone wolf” terrorism. Lone wolf terrorists were considered to be an especially dangerous threat since they were more difficult to identify, lacking the type of infrastructure that leaves groups more vulnerable to intelligence efforts. Another emerging threat came in the form of foreign fighters, citizens who would travel to join groups such as Al-Qaeda and ISIS. There have been ongoing concerns that returning foreign fighters could post a serious threat to their countries of origins.Footnote14
As these threats emerged and developed, so too did the perspectives and policy approaches of western countries. While in the earlier years there was more of a concern about the so called “root causes” and “drivers” of terrorism, more recent years gave rise to a new approach that focused on pathways and routes to terrorism.Footnote15 As research and political perspectives devoted more attention to this perspective, a new paradigm emerged that focused on radicalization. In 2005, The European Commission provided one of the early operational definitions of radicalization as being “the phenomenon of people embracing opinions, views and ideas, which could lead to acts of terrorism.”Footnote16 While the EU did not specify what “opinions, views and ideas” it was referring to, a general consensus has emerged that considered positive evaluations of terrorism as representing radicalization. It is in line with this assumption that significant investment was made to develop strategies and interventions that would stymie or otherwise mitigate the development of radicalization in the population. According to this logic model, lower levels of radicalization would lead to lower risk of recruitment.
However, the selection of which factors should be targeted in order to effectively counter radicalization has remained somewhat elusive. Some of the most commonly targeted factors pertain to social inclusion and assimilation, based on the view that radicalization is primarily an outcome of social alienation and a lack of identification with the state, society, and culture.Footnote17 However, by targeting such factors, counter-radicalization may inevitably single out certain communities or groups. Doing so may, in turn, lead to stigmatization and contribute to the very alienation that it seeks to combat. Additionally, it remains questionable whether such factors are even significantly related to radicalization at all.Footnote18 The selection of these or other factors for targeting by counter-radicalization has often been based more on local history, politics and culture rather than evidence.Footnote19 In fact, the entire development and proliferation of the counter-radicalization paradigm preceded the emergence of an evidence-base concerning the risk factors for radicalization.Footnote20
A recent systematic review of counter-radicalization programs in OECD countries shows the large number of counter radicalization and recruitment prevention programs that have developed since the turn of the century.Footnote21 Fully 21 of the 36 countries in the OECD have national policies and programs to counter radicalization. These programs differ widely in terms of their identification of risk factors to target, though social integration was the most commonly targeted risk factor. Additionally, 11 municipalities in the OECD were found to have independent counter terrorism programs. Again, the most commonly targeted risk factor was social integration. But a varied group of risk factors are targeted overall with some programs targeting as many as two dozen risk factors and others only 1 or 2. Similarly, while social integration was the most common risk factor, a large number of other risk factors were targeted across programs ranging from socio-economic conditions to mental health, with almost 50 separate risk factors featuring in this group of programs.
Despite the strong interest in counter-radicalization programs, and the large number of counter-radicalization programs that have been developed to reduce recruitment, there is little knowledge about whether such programs actually have the desired impact. In a review of counter-radicalization programs, Jugl et al. identify six evaluations of programs that focus primarily on efforts to reduce radicalization.Footnote22 These programs generally focus on targeting attitudinally oriented factors, with the goal of reducing radicalization. Overall, the programs were found to be effective, though the methodological quality of the evaluations was generally weak, with five of the nine evaluations scoring the lowest rating on the Maryland Scientific Methods scale.Footnote23 Only two of the studies had a control condition compared to the intervention. Importantly, none of these studies examined recruitment to terrorism. It remains the case that we simply do not know whether counter-radicalization interventions are achieving their desired goals.
Below we focus upon ABM as a tool for increasing knowledge about the impacts of counter-radicalization programs. We first introduce ABM as a method, and then describe the ABM we developed as part of the Proton Horizon 2020 project.Footnote24 We focus particular attention on how ABM can help to advance counter-radicalization research and policy, and the steps necessary to take to successfully develop realistic ABM models.
What Can ABMs Tell us?
An ABM is a simulation model that mimics the mechanisms operating in the real world. In some sense it allows the researchers to “play God” by creating a world in which agents, or people, live everyday lives and interact with each other and a dynamic environment. The researcher in this case builds that environment and identifies the agents. The ABM is driven by a series of rules about how agents interact and how such interactions may affect outcomes such as their attitudes or behaviors. An advantage of ABMs over traditional modelling techniques is that they are dynamic and changing across time. It is one thing to say that if A and B occur the likelihood of C occurring increases, which is how models in social science often develop. It is another to say that we will allow actors in a dynamic environment to develop and change over time. This is especially pertinent to the issues of attitudinal or opinion change and emergence of behaviors not previously displayed.
ABM results are generated by running the set of rules against the initial conditions; they might include emerging properties in the sense of complex science – that is, properties that arise from the interactions of simpler components in ways that cannot be directly deduced from those components, or properties that aren’t explicitly encoded or predictable at the agent level but manifest at a higher level of organization due to non-linear interactions. In testing interventions in our ABM we gained outcomes that were not expected based on simple extensions of parameters of the model. For example, while as expected increasing the number of community workers decreased radical attitudes, it did not have strong impact on recruitment. At the same time, increasing employment among high risk agents had little effect on radicalization, but the strongest impact of the interventions tested on recruitment. These outcomes emerged from the model, as ordinary citizen agents interacted in a dynamic environment with recruiter agents (see later), and allowed us to identify the tremendous potential of opportunity reducing programs in this area.Footnote25 With advanced agent design, emergent properties can be recognized by the agents inside the simulation and manipulated in a cognitive way, as in the case of norms.Footnote26
In the case of simulated radicalization and recruitment outcomes, ABM represents a bottom-up approach that can closely approximate how attitudes and behaviors emerge from interpersonal interactions in the real world.Footnote27 Crucially, ABMs comprise a theoretically informed approach to ask the question: “If this process is a reasonable reflection of reality, then what is the expected outcome?”Footnote28
ABM in (Counter)Radicalization Research
We are by no means the first to recognize the potential for ABM to be used in the study of radicalization. Several studies have used ABM to model the emergence of radicalization in different ways, including polarization, opinion extremism, extremist groups who carry out violenceFootnote29 and terrorism.Footnote30 However, these studies have mostly been limited to examining single mechanisms and focus on emergence of the phenomenon and rarely experiment with policy changes.
For example, in one of the earlier applications of ABM in this field, Butler and Bryson examined radicalization and recruitment to the extremist Animal Liberation Front (ALF) as a function of social interaction both directly and through mass media exposure.Footnote31 Their experimental conditions were limited to testing the effects of “replenishing” the pool of potential recruits, manipulating the size of the model’s main geographic units, so that restrictions would be placed on social interaction, and varying degrees of mass media exposure. As such, whilst the study demonstrated the effects of mechanisms contributing to the outcomes, it did not test any interventions per se.
Some studies have included multiple mechanisms. For example, Neumann modelled radicalization in Yugoslavia.Footnote32 His model included multiple components and mechanisms and several sources of real-world empirical data were used to inform the development of the base model. However, the “radicalization” assessed in this study referred to nationalistic ideology adoption in the form of increasing popularity of certain political leaders over others, rather than the type of violent radicalization associated with terrorism that is the focus of the radicalization/violent extremism/terrorism literature that we are more familiar with. Importantly, ABM models have also been used to identify how radicalization emerges.Footnote33 However, we are not aware of any studies that have used ABM models to study recruitment to terrorism. We argue below that ABMs are particularly useful for developing a rigorous program for advancing assessments of policies and practices relating to counter-radicalization and reduction of recruitment to terrorism.
Identifying and Implementing the Theoretical Model
Key to the development of ABMs is the identification of one or more theoretical model that reflects behavior in the real world. Clear identification of the theoretical approach helps reduce the risk of ad-hoc modeling choices. Proton was guided by the prevailing conceptions of radicalization that distinguish between the cognitive outcomes of radicalization (e.g. radical attitudes), and the behavioral outcomes of radicalization (e.g. radical behaviors). We began the development of the Proton ABM with the assumption that core criminological theories would be appropriate for modelling radicalization and recruitment outcomes. Our model was informed particularly by the risk and protective factors approach to understanding involvement in criminal activities.Footnote34
Like risk and protective factors for other criminal and criminal-analogous outcomes, risk factors for radicalization can generally be split into categories of static and dynamic factors.Footnote35 Static risk factors are the stable, often immutable factors that are distributed among individuals in a population, manifested as background characteristics or propensities usually ingrained in individual psychological or personality-related traits.Footnote36 Dynamic factors are malleable and often change over time. Among dynamic factors, attitudinal risk factors are often seen as offering the greatest opportunity for targeting by interventions. This is because such factors are open to formation and change through the individual’s experiences and exposures. Taken together, “risk status” and dynamic factors determine levels of radicalization and whether an individual would be vulnerable to actual recruitment at a given point in time.Footnote37
We note that the Proton ABM was based on a systematic review of risk factors for radicalization and recruitment to terrorism.Footnote38 We think that such careful reviews are key to developing an ABM that accurately reflects the real world. We also benefited from the ability of Proton to commission new studies where there were significant gaps in the literature.Footnote39 These included studies on recidivism in the context of terrorism,Footnote40 resilience against radicalization,Footnote41 psychological and socio-economic influences differentiating terrorist offenders from the general population,Footnote42 the effects of policing practice and procedural justice on radicalization and recruitment to terrorism,Footnote43 and the relationship between the internet and radicalization and recruitment.Footnote44
In constructing our ABM, we sought to identify a small group of static and dynamic risk factors that have been found to be consistent correlates of radicalization. In this context, authoritarian and fundamentalist personality are generally considered key risk factors or propensities related to radicalization.Footnote45 Most studies examine this construct using validated measures which include components measuring deference to established authorities, demonstration of prejudice and aggression toward out-groups, and support for traditional values. In Proton, we drew on the European Values Survey (EVS) and items that have previously been used to create a proxy scale for authoritarian/fundamentalist personality, and which measure child rearing attitudes, and attitudes concerning the relationship between religion, state, and authority over citizens. Given that these items are measured on a likert scale, the weighting of the factor, derived from the meta-analysis,Footnote46 was assigned based on categories of one and two standard deviations from the population mean.
Other static risk factors that have been consistently found to influence likelihood of radicalization are gender and age.Footnote47 With respect to gender, the weighting of the factor, derived from a meta-analysis, was applied dichotomously. With respect to age, we followed previous research on the age-terror curve, which similarly to the age-crime curve shows that the mean age for involvement in terrorism is early-mid 20’s. In doing so, we applied the weighting for age, as derived from the meta-analysis, to agents under the age of 25. Two other factors that were included were criminal history and employment status. While these factors will vary across the life course, they represent key background factors influencing radical attitudes at the initiation of the Proton ABM simulation. With respect to employment status, meta-analysis results only provided estimates for employed-unemployed.Footnote48 This meant that we were limited in being able to explore a broader range of employment statuses, such as part-time employment, or homemaker. As such, the weighting for employment was applied dichotomously based on a collapsed employment category reported in the census data. With respect to criminal history, meta-analysis findingsFootnote49 similarly were unable to distinguish between different types of histories (e.g. drug, violent, property offences) and offence severity, only providing a summary estimate. As such, we were limited to applying the weighting for this factor dichotomously.
Based on the systematic review and meta-analysis, as well as the commissioned studies, we developed two key equations for determining radicalization. The first was to model the propensity for radicalization at the outset of the model. The factors included in this equation were agents’ age, gender, employment status, criminal history, and authoritarian/fundamentalist personality. All these factors are considered to be static, with the exception of employment status which was subject to change as a result of one of the interventions we tested.Footnote50 “W” represents the weights applied in the equations.
The second equation describes the dynamic aspects of the model, focusing on the additive role of dynamic factors in addition to propensity, in modelling the radicalization risk status of agents in the model. This determined the level of radicalization of an individual as well as potential to be recruited to terrorist groups. This equation was made up of the initial propensity (see above) and the floating scores of three different, dynamic risk-protective factors. The scores for these factors, and as such the overall risk score, were updated at each tick (see below) of the model. As noted above, all of these factors have been shown to be important to radicalization both theoretically and empirically. Perhaps most importantly, they represent the types of factors commonly targeted by real-world counter-radicalization programs.Footnote51
The best ABM models are likely to be developed through inter-disciplinary collaboration.Footnote52 The Proton ABM was created through a collaboration between criminologists at the Institute of Criminology at the Hebrew University, and computational social scientists from the Institute for Cognitive Sciences and Technologies in Rome. The degree of precision that is required for developing an ABM requires the transformation of an informally described theory or an integrated set of theories into a formal model. Computational social scientists may lack sufficient understanding of the theory in order to accomplish this task, and the criminologist or radicalization expert may lack the necessary technical know-how.
For example, in developing our ABM, the criminology team raised the importance of routine activities and socialization in any model of radicalization, and in particular, as the mechanisms through which dynamic risk factors are subject to change. These are mechanisms that are difficult to model using traditional statistical techniques, and traditional instruments such as self-report surveys. It was at this stage that the computer science team suggested the most appropriate options for modelling these mechanisms, focusing in particular on opinion dynamics functions for modelling the type of socialization envisioned by the team (see the section below on “Creating realistic agents in the model”).
Additionally, over the course of the translation and formulation process, inevitably various situations will arise in which decisions will need to be made concerning modelling choices that will be characterized by a need to achieve some sort of parsimony between the theory and the complexity of the model. In such circumstances, reformulation may be required, and in other cases abandonment or replacement with alternatives may be called for. As these decisions will have significant impacts on how the results from the ABM are ultimately interpreted, collaboration is of the utmost importance.
Throughout the course of our collaboration there were ongoing meetings in which we discussed what we wanted to model and how our ideas could be implemented. There were also ongoing discussions about what types of data would be needed in order to translate our ideas into a formal model. Rather than being multidisciplinary, the best computational social science, or computational criminology, comes from interdisciplinary work.Footnote53 Failure to take this approach has led to poorly developed ABMs.Footnote54
ABM and Ethical and Human Subjects Concerns
The first advantage of ABMs for critically assessing the outcomes of programs is that they are simulations of the real world and do not require actual interactions with human subjects. This of course removes many of the ethical and human subjects concerns that have hindered terrorism research. Agents in ABMs are not actual people and therefore there is no need to define human subjects protocols. Simulated groups may be targeted without concern regarding how the intervention may impact them. This was how our ABM in the Proton study supported by the Horizon 2020 program began.Footnote55 Concerns regarding testing programs meant to prevent or reduce recruitment in the real world led us to assess whether it would be possible to assess such programs in a simulated world.
But it is important to note at the outset that ethical concerns do not disappear with the use of simulation modelling of this type. There remains the more general question of whether the interventions that would be tested meet in general terms ethical requirements. In an ABM, experiments can be developed for any intervention. For example, one could test the use of intrusive identification techniques, or programs that apply pressure to people in the simulated system. Accordingly, while the direct concerns of interactions with human subjects are overcome in ABMs, ethical questions regarding the interventions being tested remain. Of course, even in this case it could be argued that it is useful to understand whether and how those interventions impact outcomes and that ABM provides a method for doing that without real world impacts on subjects.
In Proton, the reviewers raised the question of whether information gained from ABMs may cause societal harm simply from their being available for use. For example, an ABM might focus on a specific disadvantaged minority population. In this case, the question was whether the testing of outcomes through an ABM would lead to stigmatization of such populations by governments. Put differently, does the focus, for example, on Muslim radicals, direct consumers of the research to focus undue attention on such groups? We suspect that such ethical concerns will vary from context to context, but it is a mistake to assume at the outset that they will not be raised in relationship to an ABM just because it is a simulation.
In Proton, and in line with the Horizon 2020 program, an independent review team was established to assess the ethical and human subjects concerns of the project overall, and specifically regarding the ABM. Indeed, initial concerns about the focusing on minority Muslim populations in European cities led us to review whether in fact it would be necessary to develop ABMs separately for this population. From our meta-analytic work that was conducted as part of the project, we found that left wing, right wing, and Muslim radicalization involved very similar risk factors. In fact, the magnitude of the effects for the various risk and protective factors were very similar. Based on this, we concluded that our ABM did not need to focus on any specific group.Footnote56 This allowed us to respond to the concerns raised by reviewers, without any loss of validity. Such solutions may not be realistic in other contexts, but our experience suggests the potential for ethical questions to be raised in ABMs in this area.
The importance of Defining Measurable Outcomes
One of the most important steps in developing an ABM is determining what the outcomes of interest are. In counter-radicalization research we may be interested in a range of factors, such as cognitive radicalization, behavioral radicalization, recruitment, terrorism events, or any combination of these. We may also be interested in secondary outcomes such as agents’ opinions on a range of factors, or changes to psychological factors, such as authoritarianism.
When defining the outcomes of interest, it is also important to consider and formalize how they are to be measured. The choice of measurement should reflect the way in which these outcomes are measured in the real world, according to some sort of discrete or continuous scale, or sometimes as binary outcomes of present/not present, or having occurred/not occurred.
It is also possible to have more than one outcome, and for the outcomes to have reciprocal effects. In the Proton experiments we were interested in both radicalization and recruitment. Following radicalization models, radicalization was viewed as an essential prerequisite for recruitment in the Proton model—only radicalized individuals could be recruited. Agents with high risk scores (which were dynamic and changed throughout the model), were eligible for recruitment (when they reached a specific threshold). This served to reflect the reality in which 1) only a small proportion of radicals are recruited, and 2) not all recruited individuals are necessarily the most radicalized or high risk.Footnote57 Recruitment was treated as a general outcome, or a state of being (either recruited or not recruited). Relatedly, just as the model did not pertain to a specific ideology, it also did not differentiate between different types of terrorism (e.g. lone wolves, foreign fighters etc.), as this was not the focus of the project. Striving to reflect the best evidence on radicalization and recruitment, it was necessary to ensure that an agent could become recruited following relatively brief interactions (though this would happen very rarely), whereas another agent may not be recruited until they reached a threshold of hundreds of hours of socializing with a recruiter (also a rare process). The Proton ABM dealt with this issue by implementing a normal distribution into the recruitment function, with an empirically informed mean and standard deviation, and governed by a stochastic factor.Footnote58
Creating a Realistic Landscape
We think the development of realistic models requires creating ABM landscapes that represent accurately the focus of the research. Though there is always a balance between parsimony and detail, we think to date researchers have erred in developing overly generalized models that fail to accurately reflect the reality of the real world.Footnote59 Indeed, in some sense, the more an ABM simulation can look like the real world, the easier it will be for consumers of the ABM to accept that it is believable. An ABM that fails to approximate characteristics of the real world will always be vulnerable to be challenged on the basis that some factors not modelled are important for understanding outcomes.
At the same time, models that are overly complex will be difficult to run, demanding significant processing resources, though such constraints are being overcome as computing power is significantly increased over time. Modelers will note that there is no need for making models overly complex if a simpler model can adequately represent real world outcomes.Footnote60 And from this perspective, the simpler the model, and the smaller the number of parameters it defines, the better. Our experience however is that models that seek to support or inform policy must reflect a world that is recognizable to those it is meant to influence.
In defining the landscape for the Proton ABM we sought to identify a borough, or section of a city, whose landscape and population of vulnerable individuals was similar to other communities in Europe or elsewhere facing problems of radicalization and recruitment. Having considered a number of possible locations from which to model the ABM, and consulting with local experts, we identified Neukölln, Berlin, Germany as a suitable site. Neukölln, one of Berlin’s largest districts, is home to a diverse population of about 350,000 residents. Importantly, Neukölln experienced violent attacks from right-wing, left-wing, and Islamist radicals.Footnote61
Neukölln also served as a suitable test case on account of it containing four distinct adjacent neighborhoods (Neukölln, Britz/Buckow, Gropiusstadt, and Buckow Nord/Rudow) that differed in their characteristics, and therefore provided a diverse set of communities for our model. For example, the neighborhoods differed in terms of the makeup of their populations, as well as both the number and types of places (e.g. community centers, parks, religious buildings, public spaces, workplaces, cafes, etc.) relevant for recruitment to terrorism. We used Google Maps to identify and populate the model with these places. We also relied on local research findings for identifying the number of places that could be characterized as risk decreasing (e.g. community centers) or increasing (e.g. recruitment hotspots), and their locations.Footnote62
We followed previous applications of ABM and implemented an abstract geographic landscape.Footnote63 Accordingly, while we sought to create a realistic landscape more generally, we made the decision that it was unnecessary for our purposes to define a street network which reflected the reality of space in the city. Instead we used the common ABM designation of “patches” which may be thought of as a kind of abstract physical space similar to a census block but without physical distance within the patch. Importantly, we also decided that our agents would not transverse the landscape by foot, or mechanized transportation. Rather, they were “teleported” from one patch to another, within a definite range based on their routine activities, established and developing preferences, and stochastic factors. These are examples of assessing when a model must conform to the reality of the world, and when it is possible to simplify that reality for the purposes of the simulation. An example where the street network was critical to the operation of an ABM is found in an ABM experiment which examined police patrol at crime hot spots. In that model, the realistic movement of potential offenders and victims through streets was critical to assessing whether and where robberies would occur.Footnote64 In the Proton model, as we modeled the interactions that would affect recruitment scores as happening only in specific places, modeling movement was unnecessary.
Creating Realistic Agents in the Model
Creating agents that reflect actors in the real world is equally important for developing a realistic ABM. The primary agents in the Proton model were ordinary people, “citizens,” who lived in the simulated landscape. We initialized their characteristics using data from the Berlin Central Bureau of Statistics (CBS) for each of Neukölln’s four neighborhoods. In order to generate and assign opinion scores to agents, we resampled the 2008 European Values Survey (EVS) for Germany, cross-tabulated the census factors with the relevant opinions that we wished our agents to possess, and then distributed the shared set of characteristics to each individual agent in the population based on their characteristics derived from the census data. The result of this approach was that each citizen agent represented a (re-sampled) survey respondent. We think that in the creation of realistic policy evaluation models, such data are crucial to create a believable ABM.
Following prior research, for computational purposes we scaled down our simulated borough to 40,000 agents.Footnote65 We noted earlier that one of the benefits of ABM is that it allows one to overcome the problems of assessing low base rate outcomes. In our case, in assessing recruitment we knew that an exceptionally small part of the population would ever be recruited to terrorism. Achieving a sample of 40,000 subjects is certainly something that would be difficult to replicate in any field study. At the same time, ABMs to date are constrained regarding the size of the population that can be examined due to computational limitations. Larger populations inherently require longer computing times at all stages. This is another factor that the researcher must balance when considering the development of an ABM model. What size sample is required to observe the effects examined?
To some extent this question may become irrelevant as computing processing becomes more powerful. The best approach would be to include the full population of the study’s target of interest. However, this is not realistic in today’s computing environment, which makes the identification of required sample size an important focus. We do have some evidence that small population ABMs will adequately represent scaled up populations. Bosse, Elffers, and Gerritsen examined directly whether small-scale ABM models produce results similar to larger simulations.Footnote66 These authors doubled the size of their model’s population and noted: “[T]he effect of scaling up the size of the society [on crime] turned out to be small”.Footnote67 Of course, this assumes that the distribution of factors, at scale, is still of a meaningful frequency. For example, in our case, if one were to scale down a population to such a size that there would only be one high-risk agent, then it would be impossible to model the outcomes of interest. In the Proton model, the chosen scale included a sufficient number of agents for the distributions of characteristics to remain meaningful. Modelers must consider the issue of scale carefully when determining the most appropriate population size.
Citizens in the Proton model engaged in routine activities, moving around the landscape and visiting different “places.” The likelihood that an individual would visit a given place, or be exposed to a particular setting, was a function of social and self-selection processes. Relevant factors that conditioned routine activities included (1) place of residence, (2) employment status and place of employment, (3) activity history, (4) personal preferences, and (5) randomness. All agents were assigned an 8-h period for sleeping at home each day. As nothing occurs during this period, we did not model it explicitly. Employed agents spent 8-h per day at work. Outside of these hours, agents could decide to (1) stay at home, (2) visit different locations, or (3) spend time on the internet. These factors, combined, give rise to heterogenous, differential opportunities for socialization among agents.Footnote68
The primary activity that the Proton model examined was inter-agent interaction, of which there were three categories: (1) speaking, (2) listening, and (3) experiencing. It is through these interactions that opinion-related dynamic factors are changed, and through which an individual can come to be recruited.Footnote69 In this model, when two agents converge at a location at the same time, they are given the opportunity to interact. We modeled three topics that agents could choose to discuss when they interact and that pertain to the dynamic risk factors described earlier: (i) trust in/legitimacy of institutions, (ii) integration, and (iii) subjective deprivation.Footnote70 Agents could also communicate about their experiences at specific places. Importantly, to better capture social learning dynamics,Footnote71 agents would develop preferences for returning to places in which successful interactions occurred. It was through these interactions that agents changed their risk levels in the model. Interacting with a recruiter agent in our model, for example, would be likely to lead to increases in each of three risk factors noted above, which are key components of the risk equation defined above. As noted earlier, when an agent reached a specific level of risk of radicalization in the model, they were then capable of being recruited.
As we have highlighted, we sought to create a simulation that was reflective of the real world. As such, we found it important to incorporate into agents’ routines the ability to interact via online communications. In our models, online communications operated in the same way as offline interactions, although they differed in three ways. First, agents did not have to come into direct contact with each other physically in order to interact. While interactions occurred with other agents in the model, the heterogenous population of agents served to reflect the spectrum of potential online interactions. Second, based on data concerning the amount of time Germans spend on the internet,Footnote72 we limited agents to spending up to a maximum of 25% of their leisure time using the internet. Third, the magnitude of the effects of successful online communications were calibrated to being half of those of offline interactions, with the relative effect sizes based on the findings of the aforementioned meta-analysis.Footnote73 This is an example of the type of detail that a researcher must focus upon to develop ABMs that will present valid outcomes.
Recruiter Agents
In evaluating counter-radicalization and recruitment prevention programs in the context of an ABM it is likely that researchers will need to also include “recruiter agents.” Recruiters differ from ordinary citizens in that they are already attached to terrorist groups, and have a specific role in that they are dedicated to actively trying to radicalize and recruit ordinary citizens. In the Proton ABM, recruiter agents maintained normative routine activities and only spent part of their time actively engaged in recruiting activities (6 h). Recruiters were also able to passively engage in recruiting if they converged with susceptible agents randomly during times in which they were not actively engaged in recruiting activities.
Recruiter agents had maximum values on all risk factors, and therefore played a role also as sources of radicalization. We chose to restrict recruiter agents’ opinions so that they were not open to change. This was achieved by setting recruiter agents to only be able to be speakers, and not listeners, in inter-agent interactions.
Given that current evidence points to the existence of “recruitment hotspots,” places where recruitment activities tend to be concentrated, and where they are most successful,Footnote74 we assigned recruiter agents to spend a portion of their time at such hot spots. However, not all recruitment occured at such places—although it often did—and recruitment can also occur through chance encounters during routine activities.Footnote75 In the real world, terrorism recruiters specifically seek to identify and “target” high-risk agents, or otherwise “go out of their way” to interact with them. They identify such individuals in various ways, such as through word of mouth, or their own experiences and interactions.Footnote76 In order to model this important feature of recruitment, we imbued recruiter agents with an ability to “perceive” the risk (radicalization) status of citizen agents upon coming into contact with them. Similar to citizen agents, recruiter agents also developed a preference for places in which they have successfully interacted with high-risk agents, increasing the likelihood that they would repeatedly come into contact with agents with whom they had previously had successful interactions.
The Importance of Defining Interventions and Intervention Agents
To implement ABM experiments that reflect real world programs, it is important to clearly define what the interventions are and who would carry them out. In one type of intervention, the modeler simply imposes the intervention on the agents in the simulation, a sort of an “act of God,” in which agent characteristics are manipulated in order to reflect the receipt of “treatment.” This was the approach in one of the experiments we tested in Proton, which considered the impacts of an employment program. For this experiment, we identified “high-risk” individuals who were also unemployed and assigned them to be employed. There was no program of activities in this case. In this sense our experiment followed other applications of ABM, representing an “efficacy” experiment in which the interventions were studied under ideal conditions.Footnote77 It would be possible to define attrition rates of employment based on known data for example, but in this type of ABM experiment in counter-radicalization and prevention of recruitment, there is no program per se, but rather an imposition of a specific condition on agents.
Sometimes, it is necessary to define agents who implement such programs. The Proton study simulated two policies that focused on special agents who are “implementers” of programs. One such policy focused on increasing the number of community workers operating at community centers. Reflecting again a well implemented program, all community center workers could communicate positive counter-radicalization values to people who visit these centers. In the second experiment, the effects of a community policing policy on radicalization and recruitment to terrorism were tested. Proton modeled a scenario in which 50% of police officers in the simulated community were trained in community policing. While these police officers were similar to ordinary police, in that they engaged in random patrol and random interactions with citizens, community police officers were programmed to communicate positive legitimacy values to people they have contact with. In these two cases, community workers and police agents existed in the base model, however the experiments focused on increasing their numbers, and altering their characteristics respectively, representing two different intervention approaches.
These examples show the importance of defining the key elements of a program, and how agents may be required to carry them out. However, they also reflect the “ideal” world circumstances common to ABM models. This is one reason why ABM experiments cannot replace actual field experiments. In ABM experiments the researchers can create programs with high fidelity, without attrition and other barriers to implementation. This allows the experiment to examine what would happen in a “perfect” world, but the field environment is often not perfect. As noted above, the ABM can build constraints into program implementation, but there would need to be sufficient data at the outset to implement those. Ordinarily, such data are not available, and indeed this is why data from process evaluations of field research can be useful in building intervention experiments in ABM.
The Potential for Large Scale Programs of Evaluation
An advantage of ABM that we noted earlier is that a large number of “evaluations” can theoretically be carried without leaving the laboratory, a benefit similar to laboratory experiments more generally. At the same time, it would be overly simplistic to assume that ABM experiments could be carried out on a large number of interventions without significant effort and cost. Although ABMs are simulations, and do not require real-world resources and implementation, as we noted earlier they are resource intensive in terms of programming and computer time needed. Still, we think that one of the most important benefits of ABM experiments is that once an ABM model has been defined, tested and found to be a realistic portrayal of outcomes in the real world (see below), they can be applied to a large number of programs and policies with some cost, but with relatively little expense compared with field experiments.
The benefits of ABMs accordingly are twofold. In the first place they allow us to assess the “logic model” of any specific program. As noted earlier, ABMs provide a simulation model in which we can assess whether a program will have the desired outcomes proposed under the assumptions made by the modeler. Rather than asking whether “the program works,” the ABM asks whether the program has “potential to be effective.” Accordingly, ABMs provide an important tool for assessing specific programs and practices in the real world.
But as a second benefit of ABMs, we have the potential for reviewing a large number of programs to identify which of these has most promise and should be subject to evaluation. We noted above the large number of de-radicalization programs now present across the world. But even if the number of programs was small, there may be a large group of interventions that have theoretical promise of success. ABMs provide potential for a program of evaluations that would focus in on those that have most potential for success. This would be an important advance over the serendipitous nature of selection of programs for evaluation that is often present today. Whether a program or policy is evaluated has much to do with researcher interest, or whether government or NGOs have interest in a specific intervention. Once an ABM has been developed it is possible to observe the full range of actual and potential programs, and to identify which of these have most potential for success. We think this provides for the possibility of a more systematic and cost-effective approach to field experimentation.
To date, there has not been governmental or foundational support for such an approach, but it has long been recognized that ABM experiments could provide a filter through which programs could be identified that have strong promise of success.Footnote78 Counter-radicalization programs clearly provide an example of where such a filtering approach would be beneficial. There are simply too many potential programs and policies to examine using expensive field research evaluation methods. ABM would allow the identification of which programs have enough promise that they would warrant the expense and risk of a field evaluation.
The Importance of Calibration and Validation
As we have so far suggested, building a valid ABM requires significant basic research knowledge about underlying mechanisms, and significant effort in modelling those underlying set of mechanisms, the broader landscape, and citizen characteristics and attitudes associated with the problem under study. But whatever the effort that has gone into building the ABM, for it to play a role in evaluations of programs and policies, it must be shown that the underlying model reflects what occurs in the real world. There are two key steps to establishing “believability” in ABM models: calibration and validation.
Calibration
Calibration entails the setting of the model’s parameters and structures according to values that serve to reflect some real-world system. That is, calibration is the stage in which the mechanisms underlying the model are fine-tuned in order for them to simulate the particular dimension of the reality, and its characteristics, that is being modelled. This stage therefore typically requires integrating some level of data on the micro-level processes that the agent-based model is based upon, and theoretically informed assumptions.Footnote79 The goal is not to see if the model works in a certain way to produce a certain outcome but rather to constrain the model’s parameters and rules so that it will operate in a certain way.
A key mechanism underpinning our model was opinion dynamics, which we used to model the transference or influence of opinions during social interactions. There is a long history of the relevance of social interaction in the development of criminal or radical ideas. Sutherland’s differential association theory in criminology, for example,Footnote80 has long been seen as key to understanding how associations with offenders are likely to lead to criminality. More recently, differential associations have been identified as a key theory for understanding radicalization and terrorism.Footnote81 In the case of opinion dynamics, we calibrated our model to find an appropriate tolerance level, or range of plausible changes in opinions, that were reasonable given theory and data in this area.Footnote82 We initially ran the model with higher tolerance levels, which are often used in basic opinion dynamics models. Then, we gradually lowered the value until, the average opinion change fell within a realistic range on our time scale. Real-world opinions, as observed in surveys like the EVS, don’t undergo drastic changes from one year to the next.
However, in our simulations, there could be significant yearly shifts, likely due to reinforcing feedback effects. To match this, we progressively reduced the alpha or tolerance range values until the average change aligned with a threshold of about a 10% annual change. In this regard, our model also entailed calibration of parameters such as the number of patches and the spatial dimension that they represent, distance traveled by agents, the time that each tick of the model represents, and other features, so that the model accurately represented the reality that it sought to simulate.
Validation
Validation is key to creating a believable ABM. It asks whether the ABM is producing outcomes that are consistent with real world outcomes.Footnote83 In the Proton model, we validated the model using three approaches. First, we sought to examine whether the base model produced realistic outcomes in terms of change over time in justifications of terrorism. Various cross-national surveys show relatively little fluctuation in justification of terrorism over time within populations. As such, we expected that in running the ABM for the 6-month and 12-month study periods without any interventions, there would be little impact on overall radicalization (risk) for the citizen agents—because there is little change in survey outcomes over these time periods. Indeed, the average change in radicalization across 40 runs of the ABM was only 0.8%, demonstrating that our base model followed the patterns observed in real-world data.
A second approach was to test whether the base model produced a realistic number of recruited individuals. An ABM is intended to provide a portrait of the real world, that goes beyond what is normally observed or measured by the type of statistics known to suffer from undercounting. In the case of our model, the goal was to model the actual level of recruitment, not the level captured by official data. As such, we operated from the point of departure that official statistics on offending serve as an “at least this much” estimate.Footnote84 Our approach was to take statistics from the Berlin PoliceFootnote85 on the number of politically motivated criminal defendants recorded in Neukölln for 2017 (divided by first and second halves of the year) as an “at least this much” estimate of recruitment. We then applied a conservative dark figure estimate of 50% and scaled the estimate down to our population of 40,000.Footnote86 Based on this, we arrived at an estimate of 80 recruited individuals at 6 months and 140 at 1 year. In our base model, across 40 simulations, we found an average of 82 recruited agents at 6 months and 157 at 1 year. We took this as an indication that our model was producing estimates approximating those in the real world.
Finally, we manipulated key factors in the model to see whether they led to expected and consistent changes in outcomes.Footnote87 When a model is operating as it should, extreme manipulations of underlying variables ought to produce commensurate changes to the model’s outcomes—which was the case in our models. For example, we increased the ratio of males to females, in increments of 5%, the proportion of unemployed agents by increments of 10%, and the proportion of agents with criminal history by 5%, up to a maximum of 20%. In all of these manipulations, our main outcomes, namely radicalization and recruitment, increased in a linear fashion, as would be expected from our theoretical model and prior research.
One way to validate the model outcomes in an evaluation study is to examine whether dosage of an intervention follows an expected outcome pattern given the overall results of the model. In the Proton experiments we found that the employment and community policing interventions had little impact on average radicalization of agents in the model at the dosages employed in each intervention. In contrast, the community workers intervention (at the dosage proscribed in the experiment) had a significant impact on radicalization. In validating our model we wanted to see whether the outcomes followed these patterns when we applied very low or very high dosages of treatment. As is apparent from , irrespective of treatment dosage the employment and community policing interventions produced relatively small changes in average radicalization/risk levels of agents in the model. In contrast, the community workers intervention showed a relatively linear pattern with increasing dosage producing decreasing radicalization.
Conclusions
Our paper has described how ABM can aid in developing a rigorous body of knowledge about the impacts of programs and policies to reduce radicalization and recruitment. This is the case in part because ABMs allow researchers to overcome key barriers to experimental field research in this area. Ethical requirements in research make it difficult to develop rigorous field trials of these interventions, in part because they deal with sensitive questions and often involve marginalized populations, and sometimes youth populations. ABM is a simulation model and accordingly does not face directly such problems, though as we have noted, ethical questions do not disappear with ABM interventions since the nature of the interventions and who they focus upon may impact policy makers and the public. ABMs also allow the involvement of large populations of subjects with modest increases in costs, thus allowing investigation of outcomes such as recruitment to terrorism which are rare. Finally, because ABMs are essentially laboratory experiments without actual human subjects, it is possible in theory to examine a large number of programs once a valid base model has been produced.
We have emphasized in our paper the importance of developing ABMs that reflect the mechanisms underlying radicalization and recruitment to terrorism. This means using strong theory, and defining the landscapes and populations examined based on data from the real world. Our experience is that the more the ABM looks like real life, the more believable it will be to policy makers and the public. But beyond this we illustrate how such an approach can produce realistic outcomes. We have described the importance of calibration in ABMs, as well as the necessity of examining the validity of such models through comparisons of simulation outcomes with real world outcomes, and examination of the sensitivity of outcomes examined to meaningful changes in parameters. The Proton ABM is a good example, we believe, of the use of real world data both in informing the parameters of the model, and in examining whether it produces realistic outcomes.
Of course, ABMs are in the end only simulations. They tell us whether, in a dynamic modelling framework, programs or policies will have the desired outcomes given the assumptions of our model. But they are not real-world experiments, and they do not replace field evaluations. Additionally, ABMs are necessarily an abstraction of reality, and as described above, require various decisions to be made to reach a parsimonious model. This means that aspects of the complexity of the real world will by necessity be missing in the models.
However, as suggested by a number of other researchers,Footnote88 ABMs can be used as a “first step” in a successful program of evaluation. Once a valid ABM is developed it can be used to test a large number of specific interventions. This is particularly important in the case of programs and policies for deradicalization since there are, as we described above, many different interventions that have developed in many different contexts. With a relatively small investment of research funds toward ABM programs it would be possible to provide a first test of efficacy of a large number of interventions. ABMs can tell us whether programs have significant promise, and accordingly can be used as a way of identifying a small group of programs for experimental testing in the real world. We think this is a much better approach than the serendipitous approach to selecting programs for evaluation common today. In this way ABMs can play a key role in advancing evaluation research in radicalization and recruitment to terrorism.
Disclosure Statement
No potential conflict of interest was reported by the authors.
Notes
1 For example, see Gabriel Zohar, Joan Domènech-Abella, Free De Backer, and Koen Lombaerts, “The Relationship between Level of Education and Household Income in the Justification of Political Violence in the EU,” Political Research Exchange 4, no. 1 (2022): 2031233.
2 We base this on our analysis of nationally representative surveys such as the European Values Survey and the World Values Survey. We note that the surveys ask whether respondents could in specific circumstances support the use of political violence. It does not ask whether they support political violence now.
3 Clark McCauley and Sophia Moskalenko, “Understanding Political Radicalization: The Two-pyramids Model,” American Psychologist 72, no. 3 (2017): 205.
4 Derek M.D. Silva and Mathieu Deflem, eds. Radicalization and Counter-radicalization (Emerald Publishing Limited, 2020).
5 Michael Wolfowicz, Yael Litmanovitz, David Weisburd, and Badi Hasisi, “Cognitive and Behavioral Radicalization: A Systematic Review of the Putative Risk and Protective Factors,” Campbell Systematic Reviews 17, no.3 (2021): e1174; Michael Wolfowicz, David Weisburd, and Badi Hasisi. Counter-radicalization Interventions: A Review of the Evidence (Institute for Futures Studies, 2022).
6 Keiran Hardy, “Comparing Theories of Radicalisation with Countering Violent Extremism Policy,” Journal for Deradicalization, 15 (2018), 76–110; Daniela Pisoiu, Islamist Radicalisation in Europe: An Occupational Change Process (Routledge, 2012); Silva and Deflem, Radicalization and Counter-radicalization.
7 Michael J. Williams and Steven M. Kleinman, “A Utilization-focused Guide for Conducting Terrorism Risk Reduction Program Evaluations,” Behavioral Sciences of Terrorism and Political Aggression 6, no. 2 (2014): 102–46; Michael J.Williams, Preventing and Countering Violent Extremism: Designing and Evaluating Evidence-based Programs (Routledge, 2021).
8 Wolfowicz, Weisburd, and Hasisi, Counter-radicalization Interventions.
9 Ibid.
10 McCauley and Moskalenko, “Understanding Political Radicalization”.
11 Elizabeth Groff and Lorraine Mazerolle, “Simulated Experiments and Their Potential Role in Criminology and Criminal Justice,” Journal of Experimental Criminology 4, no. 3 (2008): 187–93.
12 David Weisburd, Michael Wolfowicz, Badi Hasisi, Mario Paolucci, and Giulia Andrighetto, “What is the Best Approach for Preventing Recruitment to Terrorism? Findings from ABM Experiments in Social and Situational Prevention,” Criminology & Public Policy 21, no. 2 (2022): 461–85.
13 Rainer Hegselmann and Ulrich Krause, “Opinion Dynamics and Bounded Confidence Models, Analysis, and Simulation,” Journal of Artificial Societies and Social Simulation 5, no. 3 (2002).
14 Edwin Bakker and Beatrice De Graaf, “Preventing Lone Wolf Terrorism: Some CT Approaches Addressed,” Perspectives on Terrorism 5, no. 5/6 (2011): 43–50; Julian Richards, “Down But Not Out? Revisiting the Terror Threat From Foreign Fighters,” The International Journal of Intelligence, Security, and Public Affairs 22, no. 2 (2020): 61–81.
15 Randy Borum, “Radicalization into Violent Extremism I: A Review of Social Science Theories,” Journal of Strategic Security 4, no. 4 (2011): 7–36; John Horgan, “From Profiles to Pathways and Roots to Routes: Perspectives from Psychology on Radicalization into Terrorism,” The ANNALS of the American Academy of Political and Social Science 618, no. 1 (2008): 80–94; David Weisburd, Ernesto U. Savona, Badi Hasisi, and Fransesco Calderoni, “Introduction,” in Understanding Recruitment to Organized Crime and Terrorism, ed. David Weisburd, Ernesto U. Savona, Badi Hasisi, and Franceso Calderoni (Cham: Springer, 2020), 1–24.
16 European Commission, “Communication from the Commission to the European Parliament and the Council Concerning Terrorist Recruitment: Addressing the Factors Contributing to Violent Radicalisation”. 2005. https://eur-lex.europa.eu/legal-content/GA/TXT/?uri=CELEX:52005DC0313.
17 Wolfowicz, Weisburd, and Hasisi, Counter-radicalization Interventions.
18 Daniela Pisoiu, Islamist Radicalisation in Europe: An Occupational Change Process (Routledge, 2012); Sadeq Rahimi and Raissa Graumans, “Reconsidering the Relationship between Integration and Radicalization,” Journal for Deradicalization 5 (2015): 28–62.
19 Hardy, “Comparing Theories of Radicalisation with Countering Violent Extremism Policy”; Rahimi and Graumans, “Reconsidering the Relationship between Integration and Radicalization.”
20 Silva and Deflem, eds., Radicalization and Counter-radicalization.
21 Wolfowicz, Weisburd, and Hasisi, Counter-radicalization Interventions.
22 Irina Jugl, Friedrich Lösela, Doris Bender, and Sonja King, “Psychosocial Prevention Programs Against Radicalization and Extremism: A Meta-analysis of Outcome Evaluations,” European Journal of Psychology applied to legal context 13, no. 1 (2020): 37–46.
23 David P. Farrington, Rolf Loeber, and Maria M. Ttofi, “Risk and Protective Factors for Offending,” in The Oxford Handbook of Crime Prevention, ed. Brian C. Welsh and David P. Farrington (New York: Oxford University Press, 2012): 46–69.
24 See Weisburd, Savona, Hasisi, and Calderoni, “Introduction.”.
25 David Weisburd, Michael Wolfowicz, Badi Hasisi, Mario Paolucci, and Giulia Andrighetto, “What is the Best Approach for Preventing Recruitment to Terrorism? Findings from ABM Experiments in Social and Situational Prevention,” Criminology & Public Policy 21, no. 2 (2022): 461–85.
26 Giulia Andrighetto, Rosaria Conte, and Paolo Turrini, “Emergence in the Loop: Simulating the Two Way Dynamics of Norm Innovation,” Dagstuhl Seminar Proceedings. Schloss Dagstuhl-Leibniz-Zentrum für Informatik, 2007; Cristiano Castelfranchi, “Simulating with Cognitive Agents: The Importance of Cognitive Emergence,” International Workshop on Multi-agent Systems and Agent-based Simulation (Berlin, Heidelberg: Springer Berlin Heidelberg, 1998).
27 For example, see Rosaria Conte and Mario Paolucci, “On Agent-based Modeling and Computational Social Science,” Frontiers in Psychology 5 (2014): 668.
28 John E. Eck and Lin Liu, “Contrasting Simulated and Empirical Experiments in Crime Prevention,” Journal of Experimental Criminology 4, no. 3 (2008): 195–213.
29 For example, see Steven Butler and Joanna J. Bryson, “Effects of Mass Media and Opinion Exchange on Extremist Group Formation,” Proceedings of the 4 th Conference of the European Social Simulation Association (September 10-14, 2007), 455.
30 For example, see William M. Bulleit and Matthew W. Drewek, “Agent-Based Simulation for Human-Induced Hazard Analysis,” Risk Analysis: An International Journal 31, no. 2 (2011): 205–17.
31 Butler and Bryson, “Effects of Mass Media and Opinion Exchange on Extremist Group Formation.”
32 Martin Neumann, “The Escalation of Ethnonationalist Radicalization: Simulating the Effectiveness of Nationalist Ideologies,” Social Science Computer Review 32, no. 3 (2014): 312–33.
33 Pepys, Bowers, and Bouhana (2020) developed a model that shared many common features with the Proton model. At the same time, it was limited to examining only radicalization, and included a population of only 500 agents. See Rosemary Pepys, Robert Bowles, and Noémie Bouhana, “A Simulation Model of the Radicalisation Process based on the IVEE Theoretical Framework,” Journal of Artificial Societies and Social Simulation 23, no. 3 (2020).
34 Farrington, Loeber, and Ttofi, “Risk and Protective Factors for Offending.”
35 Friedrich Lösel, Doris Bender, Irina Jugl, and Sonja King, “Resilience against Political and Religious Extremism, Radicalization, and Related Violence: A Systematic Review of Studies on Protective Factors,” in Understanding Recruitment to Organized Crime and Terrorism, ed. David Weisburd, Ernesto U. Savona, Badi Hasisi, and Franceso Calderoni (Cham: Springer, 2020), 55–84; Wolfowicz et al., “Cognitive and Behavioral Radicalization: A Systematic Review of the Putative Risk and Protective Factors.”
36 Per-Olof H. Wikström and Noémie Bouhana, “Analyzing Radicalization and Terrorism: A Situational Action Theory.” The Handbook of the Criminology of Terrorism, ed. Gary La Free and Joshua D. Freilich (Wiley Blackwell, 2017), 175–86.
37 Kevin S. Douglas, and Jennifer L. Skeem, “Violence Risk Assessment: Getting Specific About Being Dynamic.” Psychology, Public Policy, and Law 11, no. 3 (2005): 347.
38 Wolfowicz et al., “Cognitive and Behavioral Radicalization: A Systematic Review of the Putative Risk and Protective Factors.”
39 These studies served to fill gaps in the literature identified in the PROTON project, and were integrated into the aforementioned systematic review and meta-analysis.
40 Badi Hasisi, Tomer Carmel, David Weisburd, and Michael Wolfowicz, “Crime and Terror: Examining Criminal Risk Factors for Terrorist Recidivism,” Journal of Quantitative Criminology 36, no. 3 (2020): 449–72.
41 Lösel et al., “Resilience against Political and Religious Extremism, Radicalization, and Related Violence: A Systematic Review of Studies on Protective Factors.”
42 Vanja Ljujic, Inge Versteegt, Frank Weerman, Fabienne Thijs, Jan-Willem van Prooijen, Fatima El Bouk, and Steve van de Weijer, “Testing a Threat Model of Terrorism: A Multi-method Study about Socio-economic and Psychological Influences on Terrorism Involvement in the Netherlands,” in Understanding Recruitment to Organized Crime and Terrorism, ed. David Weisburd, Ernesto U. Savona, Badi Hasisi, and Franceso Calderoni (Cham: Springer, 2020), 147–71.
43 Justice Tankebe (2020), “Unintended Negative Outcomes of Counter-terrorism Policing: Procedural (in)Justice and Perceived Risk of Recruitment into Terrorism” in Understanding Recruitment to Organized Crime and Terrorism, ed. David Weisburd, Ernesto U. Savona, Badi Hasisi, and Franceso Calderoni (Cham: Springer, 2020), 105–19.
44 MichaelWolfowicz, Simon Perry, Badi Hasisi, and David Weisburd, “Faces of Radicalism: Differentiating between Violent and Non-violent Radicals by their Social Media Profiles,” Computers in Human Behavior 116 (2021): 106646; Wolfowicz, Michael, David Weisburd, and Badi Hasisi, “Examining the Interactive Effects of the Filter Bubble and the Echo Chamber on Radicalization,” Journal of Experimental Criminology 19, no. 1 (2023): 119–41.
45 Pisoiu, Islamist Radicalisation in Europe.
46 Wolfowicz et al., “Cognitive and Behavioral Radicalization: A Systematic Review of the Putative Risk and Protective Factors.”
47 Ibid.
48 Ibid.
49 Ibid.
50 Weisburd, “What is the Best Approach for Preventing Recruitment to Terrorism? Findings from ABM Experiments in Social and Situational Prevention.”
51 Wolfowicz, Weisburd, and Hasisi, Counter-radicalization Interventions.
52 Groff et al., “State of the Art in Agent-based Modeling of Urban Crime: An Overview.”
53 Conte and Paolucci, “On Agent-based Modeling and Computational Social Science.”
54 David Anzola, “Knowledge Transfer in Agent-based Computational Social Science,” Studies in History and Philosophy of Science Part A 77 (2019): 29–38.
55 Weisburd et al., “Introduction.”
56 David Weisburd, Michael Wolfowicz, Badi Hasisi, Mario Paolucci, and Giulia Andrighetto, “What is the Best Approach for Preventing Recruitment to Terrorism?”
57 McCauley and Moskalenko, “Understanding Political Radicalization.”
58 Weisburd et al., “What is the Best Approach for Preventing Recruitment to Terrorism?”
59 Edmonds, Bruce, and Scott Moss. "From KISS to KIDS–an ‘anti-simplistic’modelling approach." In International workshop on multi-agent systems and agent-based simulation, pp. 130–144. Berlin, Heidelberg: Springer Berlin Heidelberg, 2004.
60 Arnald Puy, Pierfrancesco Beneventano, Simon A. Levin, Samuele Lo Piano, Tommaso Portaluri, and Andrea Saltelli, “Models with Higher Effective Dimensions Tend to Produce More Uncertain Estimates.” Science Advances 8, no. 42 (2022): eabn9450.
61 Weisburd et al., “What is the Best Approach for Preventing Recruitment to Terrorism?”
62 See for more details: Weisburd et al., “What is the Best Approach for Preventing Recruitment to Terrorism?”.
63 Groff et al., “State of the Art in Agent-based Modeling of Urban Crime: An Overview.”
64 Weisburd, Anthony A. Braga, Elizabeth R. Groff, and Alese Wooditch, “Can Hot Spots Policing Reduce Crime in Urban Areas? An Agent-Based Simulation.” Criminology 55, no.1 (2017): 137–73. Even with this modelling choice, encounters between agents occur accidentally and at random, although with a higher chance of such encounters between agents in close proximity and whose routine activities have a higher chance of convergence in time and space. As in real-world routine activities, patterns are determined by agents’ activity spaces, preferences, and elements of randomness. There are of course various other ways of modelling “travelling” in such models, and these modelling decisions do influence the opportunities for agents to converge with each other. Modelers should consider choices that most accurately reflect the dynamics they are attempting to simulate.
65 Ibid.; Groff et al., “State of the Art in Agent-based Modeling of Urban Crime: An Overview.”
66 Tibor Bosse, Henk Elffers, and Charlotte Gerritsen, “Simulating the Dynamical Interaction of Offenders, Targets and Guardians,” Crime Patterns and Analysis 3, no. 1 (2010): 51–66.
67 Ibid., 65.
68 See for more details on the routine activities of these agents in the Proton model, Weisburd, David, Michael Wolfowicz, Badi Hasisi, Mario Paolucci, and Giulia Andrighetto, “What is the Best Approach for Preventing Recruitment to Terrorism? Findings from ABM Experiments in Social and Situational Prevention,” Criminology & Public Policy 21, no. 2 (2022): 461–85.
69 See Guillaume Deffuant, David Neau, Frederic Amblard, and Gérard Weisbuch, “Mixing Beliefs among Interacting Agents,” Advances in Complex Systems 3, no. 1(2000): 87–98; Guillaume Deffuant, Frederic Amblard, Gérard Weisbuch, G., and Thierry Faure, “How can Extremism Prevail? A Study Based on the Relative Agreement Interaction Model,” Journal of Artificial Societies and Social Simulation 5, no. 4 (2002), 27; Andreas Flache; Michael Mäs, Thomas Feliciani, Edmund Chattoe-Brown, Guillaume Deffuant, Sylvie Huet, and Jan Lorenz, “Models of Social Influence: Towards the Next Frontiers,” Journal of Artificial Societies and Social Simulation 20, no. 4 (2017): 2; Hegselmann and Krause, “Opinion Dynamics and Bounded Confidence Models, Analysis, and Simulation.”
70 Agents were also able to discuss other, undefined topics which are not modelled.
71 Ronald L. Akers, Social Learning and Social Structure: A General Theory of Crime and Deviance (Boston, MA: Northeastern University Press, 1998).
72 Global Web Index, “Social: Global Web Index’s Flagship Report on the Latest Trends in Social Media. (Globalwebindex.com, 2018).
73 Wolfowicz et al., “Cognitive and Behavioral Radicalization: A Systematic Review of the Putative Risk and Protective Factors.”
74 European Commission, “Communication from the Commission to the European Parliament and the Council Concerning Terrorist Recruitment.”; Vidino, Lorenzo, Francesco Marone, Eva Entenmann. Fear thy Neighbor: Radicalization and Jihadist Attacks in the West. Ledizioni, 2017.
75 Noémie Bouhana, “Recruitment of Terrorists,” in Capacity Building in the Fight Against Terrorism, ed. U. Gürbuz (IOS Press, 2013), 70–3; Sageman, Marc, Understanding Terror Networks (University of Pennsylvania Press, 2004).
76 Noémie Bouhana, “Recruitment of Terrorists”; Thomas Hegghammer, “Should I Stay or Should I go? Explaining Variation in Western Jihadists’ Choice between Domestic and Foreign Fighting,” American Political Science Review 107, no. 1 (2013): 1–15.
77 For example, see John E. Eck and Lin Liu, “Contrasting Simulated and Empirical Experiments in Crime Prevention”; Weisburd et al., “Can hot spots policing reduce crime in urban areas? An agent-based simulation.”
78 For example, see Eck and Liu, “Contrasting Simulated and Empirical Experiments in Crime Prevention”; Groff & Mazerolle, “Simulated Experiments and heir Potential Role in Criminology and Criminal Justice”; Weisburd et al., “Can Hot Spots Policing Reduce Crime in Urban Areas? An agent-based simulation.”
79 Floriana Gargiulo, Sȏnia Ternes, Sylvie Huet, and Guillaume Deffuant, “An Iterative Approach for Generating Statistically Realistic Populations of Households,” PloS One 5, no. 1 (2010): e8828. https://doi.org/10.1371/journal.pone.0008828; Maxime Lenormand and Guillaume Deffuant, “Generating a Synthetic Population of Individuals in Households: Sample-free vs Sample-based Methods,” Journal of Artificial Societies and Social Simulation, 16, no. 4 (2012), 12. https://doi.org/10.18564/jasss.2319; Sylvie Huet, Nicolas Dumoulin, and Guillaume Deffuant, “A Calibration to Properly Design a Model Integrating Residential Mobility and Migration in a Rural Area,” In Advances in Social Simulation 2015, ed. Wander Jager, Rineke Verbrugge, Andreas Flache, Gert de Roo, Lex Hoogduin, and Charlotte Hemelrijk (Springer International Publishing, 2017), 163–79.
80 Sutherland, Edwin (1947), Principles of Criminology. Lippincott, 1947.
81 Gary LaFree, Toward a Criminology of Terrorism (Cambridge University Press, 2023).
82 Deffuant et al., “Mixing Beliefs among Interacting Agents”; Deffuant et al., “How can Extremism Prevail?”.
83 Leigh Tesfatsion and Kenneth L. Judd, eds., Handbook of Computational Economics: Agent-based Computational Economics (Elsevier, 2006); Carlo Bianchi, Pasquale Cirillo, Mauro Gallegati, and Pietro A. Vagliasind, “Validating and Calibrating Agent-based Models: A Case Study,” Computational Economics 30, no. 3 (2007): 245–64.
84 Janne Flyghed, “Policing of Political Protest. The Security Police’s Control of the Extra-Parliamentary Opposition in Sweden during and after the Cold War,” Journal of Scandinavian Studies in Criminology and Crime Prevention 14, Sup. 1 (2013): 81–99.
85 Polizei Berlin [Berlin Police], Ausgewählte Fallzahlen der Politisch motivierten Kriminalität in Berlin, 2018; Polizei Berlin [Berlin Police], Lagedarstellung Politisch Motivierte Kriminalität in Berlin 2017 (data set), 2018.
86 See Weisburd et al., “What is the Best Approach for Preventing Recruitment to Terrorism?”.
87 Gerritsen, Charlotte, “Agent-based Modelling as a Research Tool for Criminological Research,” Crime science 4, no. 1 (2015): 1–12; Gilbert, Nigel, Agent-Based Models (SAGE Publications, 2008).
88 Eck and Liu, “Contrasting Simulated and Empirical Experiments in Crime Prevention”; Groff & Mazerolle, “Simulated Experiments and heir Potential Role in Criminology and Criminal Justice”; Weisburd et al., “Can Hot Spots Policing Reduce Crime in Urban Areas? An Agent-Based Simulation.”