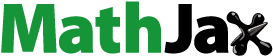
Abstract
Communication of political information is vital for a well-functioning democratic system, and texts are one of the main mediums of politics. Most studies dealing with political text consider how such texts communicate content, rather than the structural characteristics of texts themselves. The current study focuses on complexity as one of the focal structural characteristics of political text. Previous research showed that different types of textual complexity affect learning processes. Such prior studies are, however, limited both conceptually and empirically. This study addresses these gaps by employing a large-scale experimental design (N= 822), investigating how different dimensions of textual complexity affect factual and structural political knowledge, and whether such relationships are mediated through perceived complexity. Results indicate that different levels (high vs. low) and different dimensions of textual complexity (semantic vs. syntactic) influence reader’s perception of text, as well as their factual and structural knowledge. Only semantic complexity has an effect on one’s perceived complexity, which in turn negatively affects factual and structural knowledge. Syntactic complexity directly lowered one’s factual knowledge acquisition, while there was no direct effect of syntactic complexity on structural knowledge. The results suggest that text complexity indeed plays an important role in political information acquisition, and our findings also highlight the importance of perception in mediating the structural effects of the text.
A modern democratic system requires that information about political affairs is freely disseminated to the public, enabling citizens to participate in the political process and exercise democratic control of government (Strömbäck, Citation2005). It is essential that people are informed about politics, understand the political environment communicated by the media, as well as comprehend the messages by political elites (often through media) in order to form opinions about policies that may lead to political action (Delli Carpini & Keeter, Citation1993). News, whether broadcast or print, is (still) the main source of political information; and it is delivered to the audiences largely through language. Therefore, how people perceive, comprehend, and process political information and expand their political knowledge ultimately also depends on the language through which such information is delivered.
An extensive literature shows how news outlets, news articles within each outlet, or even individual frames all differ in the language used to present news, and how these characteristics affect citizens’ comprehension and learning (Liu, Shen, Eveland, & Dylko, Citation2013; Scheufele & Tewksbury, Citation2006). These studies, however, only focus on the content characteristics of texts. Nevertheless, the actual content is not the only feature that distinguishes news coverage. The structure of language is important, and variation in that structure that is used to produce political texts is readily observable. Complexity of text stands out as one of such content-independent, structural features that distinguishes political texts. Tabloids, quality newspapers, and broadcasting media may be reporting on the same topic, drawing on the same frames, but still, using structurally different, more (or less) complex language (Tolochko & Boomgaarden, Citation2019; Baker, Citation2010; Reah, Citation2002). The complexity of (political) text may have important political consequences, since simpler (vs. complex) messages may facilitate further political engagement and learning (e.g., Bischof & Senninger, Citation2018).
However, the literature has not paid sufficient attention to both, differentiations of types of political knowledge and differentiations of types of textual complexity. Starting with the former, prior studies have only considered the relationship between text complexity and factual knowledge (Kleinnijenhuis, Citation1991; Spirling, Citation2016). Such emphasis is based on the reasoning that making informed decisions requires “accurate” information (Delli Carpini & Keeter, Citation1993). However, scholars further differentiate the concept of political knowledge between “factual” and “structural” knowledge (Eveland, Cortese, Park, & Dunwoody, Citation2004; Eveland & Schmitt, Citation2015), asserting that citizens’ political competence also depends on one’s ability to make systematic connections among ideas (Eveland & Schmitt, Citation2015; Graber, Citation2001), and to make a connection between one’s prior preferences and information regarding prospective policy preferences (Bartels, Citation2005; also see Lupia, Citation2016). Yet, the literature has, for the most part, only considered how text complexity affects factual knowledge.
Linguistic complexity is also a multidimensional construct.Footnote1 The metrics used to measure linguistic complexity can be roughly categorized into semantic or syntactic complexity. Semantic complexity relates to the variability of lexical linguistic units such as variations of words and phrases that denote certain meanings, while syntactic complexity relates to the structural characteristics of texts such as clause lengths, compounds, or subordinates. Often, however, prior research on this topic has relied on measures tapping a single aspect of complexity. We argue such approaches are not sufficient to uncover the multifaceted nature of complexity and its consequences.
Finally, studies indicate that “perceived” characteristics of a media message matter, such that mere perception of message complexity is sufficient to trigger different processing strategies and psychological responses (Leroy, Helmreich, & Cowie, Citation2010; Nadkarni & Gupta, Citation2007). Based on general perspectives of the feelings-as-information theory (Schwarz, Citation2011), we conceptualize “perceived complexity” as the subjective experience of processing fluency, which is at least partly a function of objective complexity of a message. We posit this subjective experience of processing messages would serve as an internal metacognitive cue that mediates the influence of objective characteristics of a text on its outcomes.
Against this background, we explore whether, and how, these various factors are interconnected with each other. Do these different complexity dimensions produce different outcomes for political knowledge? Is perception, if at all, relevant to only one or all dimensions of complexity? Are both structural and factual knowledge acquisition affected by different dimensions of complexity? Answering these questions allows researchers to better understand both the consequences of the structural characteristics of political messages, as well as various processes connected to political knowledge acquisition. This study therefore investigates how various dimensions of text complexity affect factual and structural political knowledge, and further, whether such relationship is mediated through perceived complexity. It does so by drawing on an online experiment with a broad sample of the British population incorporating three different types of text stimuli.
Theoretical Framework
Text Complexity; Theory and Measurement
For the most part, studies of linguistic complexity of texts have been confined to linguistics and developmental psychology. In linguistics, text complexity is regarded as an objective characteristic of a text that influences the difficulty of encoding and decoding information that is conveyed in the text (“objective complexity”: Dahl, Citation2004). At the most general and abstract level, the objective characteristics of language can be further broken down into semantics and syntax (Chomsky, Citation1975; Mitkov, Citation2005).Footnote2 Syntax deals with the formal structural components of language; semantics, in contrast, is occupied with meaning. Following this distinction, semantic complexity is related to the variability, or the difficulty, of words and phrases that denote certain meanings (e.g., make use of vs. exploit), while syntactic complexity is related to the variability, or the difficulty, associated with structural characteristics of texts such as clause lengths, compounds, or subordinates (e.g., the cat sat on the mat vs. on the mat sat the cat). Despite this linguistic distinction, definitions of “text complexity” across extant studies often appear ad hoc and more importantly, unidimensional, depending on the actual research interest. In the social sciences – and especially in political science or in communication science – text complexity is most often operationalized (with some exceptions) as simply textual readability (e.g., Spirling, Citation2016). Readability is one of many rough measures of how easy the reader can understand the text. Since readability is essentially based on average number of syllables, words, and sentences in a given text (Flesch, Citation1948), it is only concerned with syntactic variations of textual information. Yet another way to operationalize text complexity, albeit more popular in linguistics and developmental psychology, is the type/token ratio (McCarthy & Jarvis, Citation2010). It is a simple ratio of unique words in a text (“types”) to all words in a text (“tokens”), therefore one of the many metrics that gauge semantic diversity of a text.
Making this distinction is important. First, when researchers claim to measure “text complexity,” more often they are using either only one of the aforementioned family of metrics. Arguably, such operationalizations are somewhat limited; the problem lies not in the fact that these measures are inherently incorrect, but rather that they tend to effectively measure different dimensions (syntactic vs. semantic complexity), therefore are not exactly comparable with each other. Relying merely on a monotonic measure of text complexity invariably leaves out another important aspect of text complexity. Second, previous research has shown how these dimensions (semantics and syntax) vary in divergent ways across types of news outlets (Tolochko & Boomgaarden, Citation2019), in that high levels of syntactic complexity do not necessarily go hand in hand with high levels of sematic complexity. Although prior research shows that text complexity may affect political knowledge (Eveland, Cortese, et al., Citation2004; Kleinnijenhuis, Citation1991), one might further expect variations in its effects on different dimensions of knowledge – especially given that there is a variation in different dimensions of text complexity.
Political Knowledge
Usually, political knowledge is used as an umbrella term for a few related, but not necessarily identical, concepts (Barabas, Jerit, Pollock, & Rainey, Citation2014; Delli Carpini & Keeter, Citation1993). For example, Eveland, Cortese, et al. (Citation2004) suggest that political knowledge is a multidimensional construct with two main facets – factual contents and structural aspects. Factual knowledge assesses whether one correctly “knows” some events and key political figures (e.g., correctly naming Donald Trump as the current president of the U.S.), therefore is very closely related to information recall. Sometimes this “factual” political knowledge can be further divided into how recent the political events or policies are (the “temporal” dimension: current affairs vs. general political knowledge),Footnote3 or whether the factual information is about abstract public policy concerns or the institution/people-specific developments (“policy-specific” vs. “general” facts: Barabas et al., Citation2014). Structural knowledge, on the other hand, refers to the degree to which such factual information is interrelated and organized within one’s cognitive structure (Eveland, Cortese, et al., Citation2004; Eveland & Schmitt, Citation2015). For instance, knowing that Greece has recently agreed on a bailout deal with the EU would constitute “factual” political knowledge; making connections of how Greece’s recent bailout deal is related to its international relation within the EU constitutes “structural” political knowledge. This distinction between “knowing” and “making connections” is also closely related to the notion of integrative complexity – or, differentiation and integration (Suedfeld & Tetlock, Citation1977); differentiation as the process of distinguishing multiple bits of information (and thus relating to factual knowledge), while integration as the process of combining disjoint bits together to form a narrative and causal relations (which directly speaks to the notion of structural knowledge).
Structural knowledge – how one bit of information is connected to another, regardless of its domain or temporal dimensions – plays a profound role in information processing. Making connections between bits of information and creating a coherent cognitive representation is crucial to relevant schema development (Neuman, Citation1986; Rhee & Cappella, Citation1997). In turn, such cognitive organization enables citizens to not only link their prior preferences to new policy information (e.g., Bartels, Citation2005; Lupia, Citation2016), but also generalize prior information to a new situation and lead to a better understanding of nuance in situations at hand (e.g., Sotirovic, Citation2001). Therefore, as Graber (Citation2001) notes, “the ability to reason effectively depends on the ability to make connections among ideas” (p. 14).
While text complexity has been largely sidestepped from prior empirical studies concerning the determinants of political knowledge, it is reasonable to assume that political knowledge may directly relate to text complexity. An information processing perspective undoubtedly suggests that complex information requires more cognitive resources to be processed and retained (e.g., Lang, Park, Sanders-Jackson, Wilson, & Wang, Citation2007). In this regard, text complexity – as the objective characteristics of how a given information is being transmitted in a text – should play an important role in how political texts are processed, therefore further influence the relationship between exposure to political information and political knowledge. For example, the decreased levels of text complexity in British parliamentary speeches has led average readers to better comprehend the message (Spirling, Citation2016). Similarly, Kleinnijenhuis (Citation1991) showed that text complexity in news articles plays an important part in explaining learning from news media – people with higher education benefit from more complex news, while the lower educated are at a disadvantage. Studies concerning message complexity (although not strictly text complexity, but the way how information is presented) also have found that complexity influence structural knowledge (Eveland, Marton, & Seo, Citation2004). Given textually complex news affects recall of factual information while characteristics of how a message is presented also affect one’s structural knowledge, one can expect that text complexity should also have a predictive effect on these two dimensions of political knowledge. Therefore:
H1: Higher degree of text complexity in political texts will have a negative effect on factual (H1a) and structural knowledge (H1b).
To date, empirical research is mainly focused on factual knowledge, while structural knowledge remains mostly in the theoretical domain. Nevertheless, political knowledge is a function of both content and structure of the message (Eveland & Hively, Citation2011). Having a proper differentiation between the dimensions of political knowledge therefore may add precision to our understanding of various types of political knowledge and its determinants. For instance, parsing and re-integration of syntactically complex text (e.g., grammatically complex sentences) may directly impede working memory capacity (Gibson, Citation1998; Gordon, Hendrick, & Levine, Citation2002), which may reduce successful recall of relevant information. In contrast, processing semantically complex text (e.g., ambiguous or abstract words) may require more resources (and therefore negatively affect recall), yet it may facilitate retrieval of thematically relevant information in one’s cognitive structure due to its multiple and complex patterns of semantic priming in one’s memory (Heil, Rolke, & Pecchinenda, Citation2004). Thus, it seems logical to explore how different dimensions the text, namely syntactic complexity and semantic complexity, are associated with different facets of political knowledge. Yet recognizing the lack of firm prior evidence, we ask the following research question:
RQ1: Do different dimensions of text complexity (semantic vs. syntactic) have differential effects on the different knowledge constructs (factual vs. structural)?
Objective vs. Perceptual Complexity and Their Effects
In addition to the manifest, objective characteristics of the media message – whether it is content or structure – a growing body of theoretical and empirical literature is devoted to “perceived” media characteristics and their influence. Processing the same contents may require substantially different levels of cognitive effort depending on message features, and the subjective experience of such processing effort may serve as a cue for further message engagement (for a review, see Petty, Briñol, Tormala, & Wegener, Citation2007). Research based on metacognitions (i.e., “thinking about thinking,” or how one’s own thoughts are associated with cognitive processes) and feelings-as-information theory indicates that people attend to their subjective experience of how easy or difficult it is to process certain information (Schwarz, Citation2011). When information is processed with difficulty, people appear to discount such information, and thus such information become less influential in judgments. Conversely, when the encoding and retrieval process is easy, the perceived value of the information is increased, and people are more likely to rely on such information (Schwarz, Citation2011; Schwarz et al., Citation1991). Based on this idea, we further conceptualize people’s subjective experience of how easy or difficult it is to process text (processing fluency; Winkielman, Schwarz, Fazendeiro, & Reber, Citation2003) as “perceived” complexity of a text.
The concept of fluency in cognitive processing can take a wide variety of forms (for a broad review, see Alter & Oppenheimer, Citation2009). Yet pertinent to our interest here, prior research indicates that the subjective experience of processing fluency is indeed systematically affected by objective linguistic features of a given text. Oppenheimer (Citation2006) showed that lexical difficulty of a word directly influences one’s processing fluency, while Lowrey’s (Citation1998) experiment suggests that certain syntactic structures (e.g., right-branching vs. center-embedded vs. left-branching sentences) can influence processing fluency and subsequent recall. Intuitively, some words are simpler in their meaning than obscure and less familiar words, and some sentences are structured more complicated than others. Presumably, greater semantic and syntactic complexity of text requires more resources for encoding (Lang et al., Citation2007), and such lexical (semantic) and syntactic variations should produce different levels of subjective experience regarding the ease or difficulty to process and encode the information (Alter & Oppenheimer, Citation2009). As a result, respondents should perceive a given textual information that is syntactically or semantically complex to be indeed more “complex” (i.e., high “perceived complexity”). Therefore, we predict:
H2: Higher semantic (H2a) and syntactic (H2b) text complexity of political texts increases perceived complexity of the text.
A large body of research on metacognitions and in particular processing fluency generally agrees that people naïvely “interpret” processing fluency as uniformly positive in nature – if a certain information is easily processed, people (sometimes erroneously) judge that information as more familiar, engage more with such information, and respond to such information more positively (Alter & Oppenheimer, Citation2009; Schwarz, Citation2011). Therefore, the subjective experience of processing fluency, which is a perceived characteristic of a given message, may provide an important mechanism of how objective linguistic features influence one’s knowledge acquisition. In the context of learning, people also appear to perceive their learning to be more successful when processing feels easy (Dunlosky, Baker, Rawson, & Hertzog, Citation2006; Koriat, Ackerman, Lockl, & Schneider, Citation2009), and they are more certain about remembering new information that is easier to process and encode (Koriat et al., Citation2009). Most importantly, processing fluency does tend to positively predict learning, such that items requiring less processing effort were more likely to be recalled later on (e.g., words that were easy to encode are recalled more often than difficult words; Koriat et al., Citation2009; Koriat & Ma’ayan, Citation2005). This is likely to be driven by the fact that perceived complexity in processing new information may directly reduce both motivation and ability for (further) systematic processing of such information. Since political knowledge – both factual and structural – requires relevant information successfully being culled from working memory, any factors limiting one’s motivation or ability to process relevant information (Chaiken, Liberman, & Eagly, Citation1989; Petty & Cacioppo, Citation1986) should negatively influence political knowledge.
A number of existing studies (albeit indirectly) supports this perspective. For instance, Leroy et al. (Citation2010) showed that perceived difficulty of medical texts directly reduces people’s health information comprehension. Similarly, Shulman and Sweitzer (Citation2018) reveal that processing difficulty – induced by different language use – can negatively influence one’s political interest and efficacy, which is shown to be a meaningful predictor of knowledge acquisition. In sum, existing studies on processing fluency undoubtedly point towards the critical role of perceived complexity (i.e. whether people actually judge the text they are reading as easy or difficult to process) in knowledge acquisition. Therefore:
H3: Perceived complexity of a text (i.e., low processing fluency) will have a negative effect on factual (H3a) and structural knowledge (H3b)
Following O’Keefe (Citation2003), discriminating between the effects of objective (e.g., text complexity) vs. subjective media characteristics (e.g., perceived complexity) allows advancing the role of objective text complexity (semantic vs. syntactic complexity) as a variation in intrinsic features of a message, whereas perceived complexity (i.e., a processing fluency experience) as a mediating psychological state that accounts for the observed effect of such objective message features. Similarly, Tao and Bucy’s (Citation2007) discussion of the effect-based paradigm conceptualizes media effects through the psychological states elicited by objective media attributes. Following O’Keefe’s (Citation2003) and Tao and Bucy (Citation2007), we posit that objective levels of text complexity should produce higher level of perceived complexity (i.e., lower processing fluency experience) as a mediating psychological state, in turn further hinder information recall (i.e., factual knowledge) and cognitive integration (i.e., structural knowledge). Therefore, we additionally predict:
H4: The negative effects of text complexity on factual and structural knowledge will be mediated by perceived complexity.
Method
Participants and Design
An online experiment was conducted to test the proposed hypotheses. A total of 822 people was recruited through Survey Sampling International. The sample is comprised of native English speakers and is generally well representing the British population in terms of gender (53% female), education (1.8% no formal education, 45.5% secondary, 30.7% college, 22% university,) and age (13.5% ages from 18 to 25, 18.6% ages from 26 to 35, 19.3% ages from 36 to 45, 19.9% ages from 46 to 55, 28.6% ages 56 and older).Footnote4
In order to test the effects of semantic and syntactic complexity on the readers’ political information acquisition, the present study employed four between-subject conditions, where participants were randomly assigned to one of the following conditions: high semantic complexity (N = 203) vs. low semantic complexity (N = 199) vs. high syntactic complexity (N = 211) vs. low syntactic complexity (N = 209).Footnote5 Yet in order to prevent possible confounding of our text complexity manipulation with a particular choice of the news topic itself (therefore to increase the generalizability of findings), we have created three different versions of stimulus per each complexity condition (i.e., stimulus sampling: Wells & Windschitl, Citation1999), varying the thematic focus of the articles. Topic 1 was about the Greek bailout deal with the EU; Topic 2 was about Italy’s economic crisis; Topic 3 was about a bird flu epidemic on an American university’s campus. This totaled up to 12 different experimental stimuli (i.e., three topics per each of four complexity manipulation conditions), yet participants within each condition were randomly exposed to only one version of the stimulus. The article topic factor was later collapsed for further analysis (also see below “Preliminary Analysis” section). Table A1 in the online appendix provides further details regarding the stimulus sampling within each complexity conditions.
Stimuli
As mentioned above, 12 stimulus categories were created (4 different conditions × 3 different topics) using real New York Times articles.Footnote6 In order to manipulate text complexity of the chosen articles, a two-step iterative process was implemented: researchers created a modified version of the original article to reflect a desired level of one complexity dimension (high vs. low) while aiming to hold the other complexity dimension at its mean level, and subsequently the modified texts were checked by a natural language processing programming script assessing their semantic and syntactic complexity levels. The script was designed to assess following metrics: for semantic complexity, lexical diversity, lexical richness and semantic entropy, all aimed to measure variability of lexicons, words and phrases. For syntactic complexity, the script assessed automated readability index, sentence length, syntactic depth and syntactic dependency, which measure structural characteristics such as clause lengths, compounds, subordinates, etc.
In order to reduce dimensionality of these metrics, we performed a principal component analysis extracting two separate components – representing the semantic and the syntactic dimensions of text – thus creating a separate multi-item scale for both dimensions. We believe this yields a more robust and valid measure than simply relying on a single metric (as many of prior studies purportedly measuring “text complexity”: for a detailed discussion of each measure, see Tolochko & Boomgaarden, Citation2019). We take the two extracted component scores as the separate semantic and syntactic complexity scores. Then, these scores were compared to benchmarks (again, separately for semantic and syntactic dimensions) from a large corpus of English newspaper articles. The researchers iteratively repeated this process until the modified version of the article scored higher (or lower) than 1 standard deviation from the mean (against the full corpus) on the respective complexity dimension, creating high (+1SD) vs. low (−1SD) versions of complexity manipulations per each dimension. As a consequence, high (low) syntactically complex version of the stimuli texts entailed more (less) subordinate clauses, prepositions, verb phrases and compound sentences, etc., while high (low) semantically complex version of stimuli entailed more (less) multiple synonyms and polysemy, had more (less) abstract and ambiguous words, etc. A sample of the manipulation materials can be found in the online appendix.
Procedure
After informed consent was obtained, participants were randomly assigned to one of the four complexity manipulation conditions. In pre-exposure questionnaire, participants were first asked to provide sociodemographic variables (for sampling purpose). Next, participants were asked to fill out the questionnaire asking their level of political interest, along with the need for cognition (NFC) scale (Cacioppo, Petty, & Feng Kao, Citation1984), then presented with the manipulation material. After exposure to the stimulus material, they were asked about the perceived complexity of the article. Next, they were presented with the concept-mapping matrix for structural knowledge measure, followed by a set of factual knowledge questions. Participants were thanked and instructed to exit the study.
Materials and Measures
Perceived Complexity
Our first dependent variable, perceived text complexity was operationalized as a subjective experience of processing fluency, and measured through following three items: “How easy is it to comprehend the (i) general idea, (ii) grammatical structure, and (iii) vocabulary of the text?” (all ranged from “extremely easy” to “extremely difficult” on a 7-point Likert scale). Since Cronbach’s alpha was acceptable ( = .91), all items were averaged (M = 3.00; SD = 1.48). Although this may be seen as an imperfect measure of “processing fluency” in that it does not encompass other dimensions of processing fluency (per Alter & Oppenheimer, Citation2009), yet since we posit subjective experience of such processing fluency would have an effect on political knowledge, we relied on self-reported measures tapping their subjective experience of ease regarding the text.
Structural Knowledge
Based on Eveland, Cortese, et al.’s (Citation2004) and Wang and Liu’s (Citation2000) pairwise concept mapping, participants were presented with a 5 × 5 symmetric matrix, where rows and columns are including concepts and entities from the manipulation materials (e.g., Prime minister, EU presidency, European Central Bank, Italy, and Financial Aid for Italy economic crisis article). For each of the pairs between concepts (e.g., between Italy and EU presidency), participants had to choose whether there was an association between the pairs based on their own understanding of the text. Participants’ structural knowledge (M = 5.41, SD = 3.91) was operationalized as the number of associations that respondents perceived to exist out of 10 unique concept-mapping pairs. This variable is ranged from zero (no associations present) to 10 (all of the concepts are related).Footnote7
Factual Knowledge
For factual knowledge, we have created seven multiple-choice questions (e.g., “what was the general idea of the article?”, “according to the article when did the event start”, and “according to the article which of the following is not true?”, etc.) that were customized for each news topic. Out of four response options, participants were asked to choose only one response for each question, where correct responses were recoded as 1 and incorrect responses were recoded as 0 (including non-response). The proportion of correctly answered questions was taken to represent one’s factual knowledge (0 = no correct answers, to 1 = all of the given answers are correct, M = .43; SD = .25).Footnote8
Analysis
Preliminary Analysis
In the pre-manipulation questionnaire, we included several pre-treatment covariates, including age, education, gender (see “Participants” section for descriptive statistics), NFC, and political interest. NFC was measured using seven items ( = .77, M = 4.41; SD = .93). Political interest was measured by using a 4-item scale from the European Social Survey (
= .96, M= 5.00, SD = 1.59). However, a balance/randomization check indicates there were no significant differences regarding those pre-treatment covariates between the four treatment conditions (see online appendix for a detail).Footnote9 Only the topic of articles (i.e., Greek bailout, Italy’s economic crisis, and the bird flu epidemic) had an effect on perceived complexity, F(2, 819) = 44.99, p < .001 and structural knowledge, F(2, 819) = 9.23, p < .001; therefore, every model controls for the topic of article participants were exposed to.Footnote10 A online supporting information presents detailed information regarding our measurement instruments.
We first start by presenting basic descriptive patterns found in our data. shows the marginal means of our three dependent variables across the four complexity experimental conditions (high semantic vs. low semantic vs. high syntactic vs. low syntactic) where marginal means per condition were averaged across article topics (we present a separate descriptive statistic per topic in the online appendix). Looking at perceived complexity, people exposed to the semantically complex texts (1st column, dark grey bars) perceived the material as being somewhat complex (M = 3.187) than those who were exposed to semantically simple texts (2nd column: M = 2.838). Similarly, those who were exposed to the syntactically complex texts did perceive the text as being more complex (3rd column: M = 3.042) than those exposed to the syntactically simple texts (4th column: M = 2.955).
Figure 1. Estimated marginal means of perceived complexity, factual knowledge, and structural knowledge across complexity manipulations averaged across article topics. Vertical lines represent 95% confidence intervals.
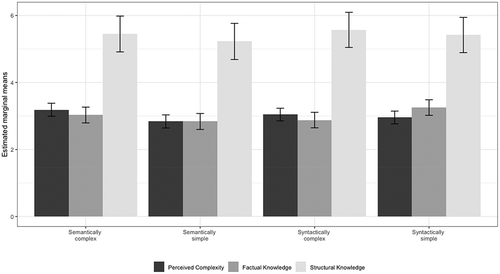
For factual knowledge, people exposed to the semantically complex texts (1st column, grey bars) answered slightly more questions correctly (M = 3.029) than those in the semantically simple texts (2nd column: M = 2.837). However, those who were exposed to the syntactically complex texts did worse in answering factual knowledge questions (3rd column: M = 2.878) than those in syntactically simple texts (4th column: M = 3.252). For structural knowledge, those who were exposed to the semantically complex texts (1st column, light grey bars) indicated slightly more connections among concept pairs (M = 5.448) compared to those who were assigned to semantically simple texts (2nd column: M = 5.225). This pattern was largely identical for the syntactically complex texts (3rd column: M = 5.571) compared to the syntactically simple texts (4th column: M = 5.471).
Effects of Text Complexity on Perceived Complexity
In order to further decompose the effect of text complexity into two different dimensions (i.e., semantic vs. syntactic separately) while controlling for the effect of one factor over the other, we estimated linear regression models with custom contrasts (i.e., complex conditions coded as +0.5 vs. simple conditions coded as −0.5; therefore, the coefficients are interpreted as mean difference across conditions while controlling for the other complexity factor).Footnote11 The results can be seen in the first column of . The result indicated that more semantically complex texts (b = .349, SE = .140, p < .05), but not syntactically complex texts (b = .086, SE = .137, p = .528), significantly increase perceived complexity of the text. Thus, there seems to be a difference in how people process and perceive various texts differently based on specific complexity dimensions. Semantic complexity has a non-trivial direct effect on perceived complexity – whether the text contains more (or less) synonymous, polysemous, abstract, or ambiguous words, etc. However, we do not find any evidence that syntactic complexity also increases processing fluency as well, only partially confirming H2. This may be due to the possibility that processing syntactically complex text may directly lower one’s ability to process such information (Gibson, Citation1998; Gordon et al., Citation2002), while proper recognition of syntactic complexity itself is not an easy task; therefore, people may not necessarily (correctly) attribute their processing difficulties to syntactic variations of texts (Funke, Wieland, Schönenberg, & Melzer, Citation2013).
Table 1 Regression models predicting perceived complexity, factual and structural political knowledge
Effects of Text Complexity and Perceived Complexity on Political Knowledge
Given the data generating process of our factual knowledge variable – binomial distribution with 7 number of trials (i.e., composed of 7 separate Bernoulli trials) – we fitted a binomial logistic regression to predict one’s factual knowledge level based on the text complexity factor one was exposed to, again only controlling for the topic of articles. The result, reported in the second and the third column of , indicates no significant direct effect of semantic complexity on factual knowledge (per H1: b = .111, SE = .076, p = .144). Syntactic complexity, on the other hand, did have a significant negative effect on factual knowledge (b = −.218, SE = .074, p < .01), indicating that people exposed to the more syntactically complex text had a lower chance of answering a factual knowledge question correctly (per RQ1). When perceived complexity is accounted for in the model (the third column of ); however, semantic complexity significantly increased the chance of answering a given factual knowledge question (b = .194, SE = .077, p <. 05), partially contradicting our H1a. However, when we additionally control for pre-treatment covariates (e.g., age, education, interest, and need for cognition) along with perceived complexity in the model, this effect no longer holds (see Table A7 in the online appendix: we briefly discuss its implications in the discussion section). Nevertheless, perceived complexity had a negative effect on factual knowledge (b = −.227, SE = .019, p <. 001), supporting H3a.
Structural knowledge (i.e., the number of connections that respondents perceived to exist) was treated as count data, yet the observed distribution showed evidence of over-dispersion. Therefore, a generalized linear model with quasi-Poisson regression was fitted, again controlling for article types. Results are reported in the last two columns of .
The results indicated that neither syntactic, nor semantic complexity directly influences structural knowledge. Therefore, H1a and H1b were not supported. This null result was largely replicated when perceived complexity was additionally accounted for in the model (the last column). Perceived complexity had a significant negative effect on structural knowledge (b = −.114, SE = .018, p <. 001), supporting H3b.
Mediation Analysis
In line with the literature supporting mediation models for perceived media characteristics (O’Keefe, Citation2003; Tao & Bucy, Citation2007), we additionally predicted that there should be an indirect effect of the text complexity through perceived complexity on both factual and structural knowledge (H4). In order to test this idea, we have relied on the model-based simulation approach to mediation analysis utilizing the R mediation package (Imai, Keele, & Tingley, Citation2010; Tingley, Yamamoto, Hirose, Keele, & Imai, Citation2014) based on 104 quasi-Bayesian Monte Carlo simulations. As can be seen in , the analysis yielded significant mediation effects for semantic complexity on factual (indirect effect = −.019, 95% CIs = [−.0342, −.0038], p < .05) as well as structural knowledge (indirect effect = −.223, 95% CIs = [−.420, −.048], p < .05). In contrast, there was no significant indirect effect of syntactic complexity on both types of political knowledge. Combined with earlier results, the analysis suggests that the effects of semantic variability of political text on knowledge acquisition and integration are likely to operate through one’s perception and processing fluency, while structural characteristics of text (as measured by syntactic complexity) directly hinder one’s factual knowledge acquisition.
Table 2 Effect decomposition from mediation models
Discussion and Conclusion
The present study attempted to investigate the effects of two different dimensions of text complexity on perceived text complexity, factual knowledge (i.e., information recall), and structural knowledge (i.e., interconnections among factual information) while taking into account the mediating role of perceived complexity. Results reveal that higher levels of objective text complexity do indeed have an ultimate consequence on the way people perceive the text and further on one’s political knowledge; however, the results hint at different mechanisms at work for different dimensions of complexity (semantic vs. syntactic), and their differential consequences for different types of knowledge (factual vs. structural). Our results therefore support the notion that textual complexity is an important factor to be considered in political communication, highlighting the critical role of how texts are presented to the audience for their optimal processing of political information.
First, our analyses revealed that only semantic complexity has an effect on one’s perception of the text at hand, with the syntactic dimension having no direct influence. While lexically complex (i.e., more abstract, ambiguous, or less familiar) text may readily provide heuristic cues regarding the difficulty level of a given text, the null results of syntactic complexity may be attributed to the fact that recognizing syntactic structures of the text is a non-trivial task for untrained readers (Funke et al., Citation2013).
Second, our analysis found that semantic complexity has a direct positive effect on factual knowledge, although this effect only holds when controlling for perceived complexity and when pre-treatment covariates are not included in the statistical model. Since this direct effect appears to be much sensitive to a model specification, we do not think this observed effect would reliably represent a (would-be) true effect of interest, yet would still hope future research would take up this question. The mediation analysis, however, indicated that semantic complexity indeed negatively affects factual knowledge through the perception of the text (O’Keefe, Citation2003; Tao & Bucy, Citation2007). Contrary to the results of semantic complexity, syntactic complexity produces an opposite result irrespective of whether the model controls for perceived complexity. Thus, the probability of recalling more factual information decreases (directly) with an increase in syntactic complexity and (indirectly) with the perceived complexity of a text. Interestingly, in case of syntactic complexity, this negative effect on factual knowledge exists without people actually recognizing the structural complexity of the text (since there is no direct evidence of syntactic complexity on perceived complexity).
Lastly, our analysis found no evidence of either the semantic or the syntactic dimension directly affecting structural knowledge. Instead, we found a significant negative indirect effect of semantic complexity on structural knowledge through perceived complexity. This demonstrates that a mediating psychological state accounting for the manifest characteristics of a message may be equally important.
Throughout the study, we have conceptualized perceived complexity as one possible form of metacognitions, in particular the subjective experience of processing fluency. Consistent with the theoretical perspective that one’s subjective experience may influence further message engagements, our results demonstrate that one’s subjective experience of how easy or difficult it is to process text uniquely affect one’s knowledge acquisition (i.e., factual) as well as its integration (i.e., structural knowledge), above and beyond the direct effect of (objectively measured) text complexity. Overall, only semantic complexity had an effect on one’s perceived complexity, which in turn negatively affected factual and structural knowledge. Syntactic complexity directly lowered one’s factual knowledge, while there was neither a direct effect nor an indirect effect of syntactic complexity on structural knowledge. In sum, this demonstrates that different types of text complexity have different mechanisms and consequences for factual vs. structural knowledge, and that it is important to further understand these different mechanisms to arrive at a more comprehensive account of the role of complexity for political learning.
These findings have broader societal implications and may motivate a discussion about today’s media discourse in general. They, for instance, might provide journalists and editors with some guidelines on how to combat declining level of political knowledge and further promote political engagements among public. For example, presenting complex information (i.e., high semantic complexity) in a relatively simple fashion (i.e., low syntactic complexity) might be beneficial for knowledge acquisition. Also, if we take perceptions into account, one must strive to present it in a way that the audience does not immediately perceive a political message as being too complex (e.g., providing accessible and easy-to-understand relevant information along with the main text) in order to communicate the complex messages to the audience and further make connections to existing information.
Additionally, our research suggests few directions for future research. For example, how does one’s choice of a news outlet (e.g., mass market vs. elite) influence the complexity effects? What role does the medium (print, online, interactive) play in this relationship? Our findings, combined with the fact that political texts are presented very differently depending on (for example) the type of a newspaper (e.g., tabloid newspapers vs. broadsheets: Tolochko & Boomgaarden, Citation2018) have significant implications for understanding how citizens’ knowledge acquisition patterns might be differentially affected by their day-to-day media diets.
Apart from the implications regarding political knowledge, we believe that these results may prompt a further discussion about measurement of text as data in communication and political science. Our results point to opposite effects of these two textual complexity dimensions – syntax and semantics. Nevertheless, a vast majority of existing research is measuring textual complexity as simply textual readability, or any other single-item measure. We believe such approaches are not enough to uncover the multifaceted nature of “text complexity” construct and its consequences. One could end up with, inadvertently, opposite conclusions based on the single “complexity measure” one chooses – be it from a family of readability indices, or, for example, a lexical diversity index.
Certainly, our study is not without shortcomings. First and foremost, this study does not draw on a true 2 (high vs. low) by 2 (semantic vs. syntactic) factorial design, but relied on 4 separate between-subjects conditions. Had it been a true 2 by 2 design, the extreme text conditions would either be visibly too complicated (i.e., high semantic and syntactic) or too simplistic (i.e., low semantic and low syntactic), not resembling a real newspaper article anymore, therefore would limit the generalizability of our results. Nevertheless, future studies investigating the effects of textual complexity would benefit from exploring possible interactive effects of semantic and syntactic complexities.
Another limitation is the way we have operationalized the knowledge variables. For instance, both for factual and structural political knowledge, we have exclusively relied on questions concerning recent developments (“surveillance-general facts”) but not about policy-specific questions (Barabas et al., Citation2014), nor about more static political facts (i.e., “general political knowledge”: Barabas et al., Citation2014; Delli Carpini & Keeter, Citation1993). Also, for structural knowledge, we simply relied on respondents’ self-reported associations among concepts and entities while largely ignoring the “valence” or “quality” of such associations (e.g., objectively incorrect associations, negative associations, etc.), which may have important implications for how an individual organizes complex political information in his or her memory. Indeed, a valence measure may better capture the “structural” characteristics of such associations, and future studies would be advisable to employ such a measure in order to better understand the precise nature of observed null results regarding the direct effect of objective complexity on structural knowledge in our study. Likewise, all of our knowledge questions exclusively rely on “verbal” questions rather than visual knowledge (Prior, Citation2014), although this is somewhat natural choice since we are interested in the effect of “language” complexities. While we acknowledge that our operationalizations rely on imperfect measurements of such variables, we strived to simplify the measurement as much as possible for the online panel. Without a doubt, adding such nuances to the measurement would have greatly improved the precision, but would naturally introduce additional complexity that would impede participants’ comprehension of the tasks even further.
Additionally, our analysis revealed significant main effects of the “article types” – that the actual topic of the text matters in peoples’ further evaluation of the text. Indeed, it is quite intuitive to think that some topics (e.g., international finance, as in Greek bailout topic) are naturally more complex than others (“issue complexity”: Kleinnijenhuis, Citation1991). Yet unfortunately, we only had three separate topics in our design, therefore creating a comprehensive taxonomy of topics and their effects on political knowledge would be somewhat futile within the current scope of the analysis.
Despite those concerns, we believe that this study brings a valuable contribution to rapidly expanding literature of text complexity and political knowledge – by accounting for the multidimensional nature of both concepts, and also by introducing one’s perception regarding text complexity as a possibly decisive factor that driving such results. We hope this study provides a first and important step towards these mechanisms, and paves a way for future research on text complexity in political communication in particular, and communication science more generally, further enabling researcher to understand how language structures affect politically relevant outcomes above and beyond the actual contents of the communication.
Supplemental Material
Download ()Supplementary Material
Supplemental data for this article can be accessed on the publisher’s website at https://doi.org/10.1080/10584609.2019.1631919
Disclosure Statement
No potential conflict of interest was reported by the authors.
Additional information
Notes on contributors
Petro Tolochko
Petro Tolochko (PhD, University of Vienna) is a post-doctoral researcher at the Department of Political Science at University of Vienna. His research interests include statistical modeling, statis- tical text analysis, linguistic characteristics of political communication.
Hyunjin Song
Hyunjin Song (PhD, The Ohio State University) is an assistant professor in the Department of Communication at University of Vienna. His research areas include the influence of interpersonal discussion on political engagement and statistical modeling of social networks.
Hajo Boomgaarden
Hajo Boomgaarden (PhD, University of Amsterdam) is Professor for Empirical Social Science Methods with a Focus on Text Analysis at the Department of Communication at University of Vienna. His research interests include the coverage and effects of political information on citizens’ cognitions, attitudes and behaviors in various domains of media and politics, and developments in automated content analysis techniques.
Notes
1. Although important, we do not consider “structural” complexity as in the LC4MP framework (Lang et al., Citation2007) since this notion of structural complexity is focusing on non-textual features of a media message (e.g., speech rates, frames per second, visuals), therefore has little to do with “textual” complexity per se.
2. While the system of language further includes pragmatics, this dimension deals with the use and interpretations of the language, therefore is related to “agent” characteristics rather than the objective characteristics.
3. General political knowledge is not considered here since civic facts (“the structure of a government and how it functions”) seldom change and are best predicted by long-term political socialization and education (Barabas et al., Citation2014; Delli Carpini & Keeter, Citation1993).
4. During the questionnaire respondents were asked a simple “attention check” question (using an instructional manipulation check), where the question reads as “This is an attention check, please choose ‘orange’ as the answer to this question,” followed by three response options (blue, orange, and purple). A subset analysis excluding those who gave an incorrect response (excluded n = 33) did not change the results reported in the main result section. These additional analyses are reported in Table A6 of the online appendix.
5. We did not employ a true 2 × 2 factorial design, since doing so would create too simple (i.e., both score low in syntactic and semantic complexity) or too complex materials (i.e., both score high in syntactic and semantic complexity), which would compromise the external validity of our approach. Since we do not posit any interactive relationship between two complexity factors, we opted to rely on four between-subject conditions instead.
6. Article topics were chosen based on the consideration that the target audience – British general population – would be reasonably, but not too, familiar with the topic. In addition, in order to prevent possible confounding effects of the label of the news source (i.e., participants engaging in biased processing of the message based on the NYT label), we changed the format of the articles to be of a more neutral source (i.e., Reuters). Reuters is considered to be fairly nonpartisan in nature, and frequently being utilized as such in selective exposure research (e.g., Messing & Westwood, Citation2014). In order to increase external validity of our manipulation, articles were presented in form of an online Reuters article, using the same layout and the general look (see online appendix for detail).
7. It is important to note that there is no conceptually “correct” or “wrong” answer to structural knowledge, since this measure is intended to capture the degree of integration regarding one’s understanding of political matters. In addition, we have presented all of the concept pairs that actually appeared together in the article; therefore, in structural knowledge questions there are no “wrong” (i.e., relations that actually were not depicted in the article) concept pairs, in line with Eveland and Hively (Citation2009) or with Wang and Liu (Citation2000).
8. We choose to present factual knowledge questions after the structural knowledge question. Doing so would eliminate the possibility of factual knowledge question would distort the treatment effect on structural knowledge measure (by repeatedly priming the contents in answering structural knowledge question). In addition, answering factual knowledge questions after structural knowledge questions does not likely contaminate the factual knowledge measures, since structural knowledge measures present no additional details other than concepts/entities presented in the articles. For a detailed discussion on this issue, see Kane and Barabas (Citation2019).
9. Controlling those pre-treatment covariates in the models did not change the results or substantial conclusions of the current study.
10. We do not find any interaction effects between article topic and complexity manipulation conditions (for perceived complexity, F(6, 810) = .367, p = .90; for factual knowledge, F(6, 810) = .564, p = .75; for structural knowledge, F(6, 810) = .683, p = .66), indicating that the effects of complexity manipulations were indeed statistically indistinguishable across different topics of articles.
11. This is identical to using effects coding, in a way that the estimated intercept is the overall mean across all conditions. When the effects of semantic and syntactic complexity are separately modeled by splitting the data by types of complexity condition (as if it was a two-study design), the results of such analyses were identical to the main results reported here (see online appendix for the comparison of the results).
References
- Alter, A. L., & Oppenheimer, D. M. (2009). Uniting the tribes of fluency to form a metacognitive nation. Personality and Social Psychology Review, 13, 219–235. doi:10.1177/1088868309341564
- Baker, P. (2010). Representations of Islam in British broadsheet and tabloid newspapers 1999–2005. Journal of Language and Politics, 9, 310–338. doi:10.1075/jlp
- Barabas, J., Jerit, J., Pollock, W., & Rainey, C. (2014). The question(s) of political knowledge. American Political Science Review, 108, 840–855. doi:10.1017/S0003055414000392
- Bartels, L. M. (2005). Homer gets a tax cut: Inequality and public policy in the American mind. Perspectives on Politics, 3, 15–31. doi:10.1017/S1537592705050036
- Bischof, D., & Senninger, R. (2018). Simple politics for the people? Complexity in campaign messages and political knowledge. European Journal of Political Research, 57, 473–495. doi:10.1111/ejpr.2018.57.issue-2
- Cacioppo, J. T., Petty, R. E., & Feng Kao, C. (1984). The efficient assessment of need for cognition. Journal of Personality Assessment, 48, 306–307. doi:10.1207/s15327752jpa4803_13
- Chaiken, S., Liberman, A., & Eagly, A. H. (1989). Heuristic and systematic information processing within and beyond the persuasion context. In J. S. Uleman & J. A. Bargh (Eds.), Unintended thought (pp. 212–252). New York, NY: Guilford.
- Chomsky, N. (1975). The logical structure of linguistic theory. Chicago, IL: University of Chicago Press.
- Dahl, Ö. (2004). The growth and maintenance of linguistic complexity. Amsterdam, Netherlands: John Benjamins Publishing.
- Delli Carpini, M. X., & Keeter, S. (1993). Measuring political knowledge: Putting first things first. American Journal of Political Science, 37, 1179–1206. doi:10.2307/2111549
- Dunlosky, J., Baker, J., Rawson, K. A., & Hertzog, C. (2006). Does aging influence people’s metacomprehension? Effects of processing ease on judgments of text learning. Psychology and Aging, 21, 390–400. doi:10.1037/0882-7974.21.2.390
- Eveland, W. P., Cortese, J., Park, H., & Dunwoody, S. (2004). How Web site organization influences free recall, factual knowledge, and knowledge structure density. Human Communication Research, 30, 208–233. doi:10.1111/j.1468-2958.2004.tb00731.x
- Eveland, W. P., & Hively, M. H. (2009). Political discussion frequency, network size, and “heterogeneity” of discussion as predictors of political knowledge and participation. Journal of Communication, 59(2), 205–224.
- Eveland, W. P., & Hively, M. H. (2011). Political knowledge. In W. Donsbach (Ed.), The international encyclopedia of communication. Malden, MA: Blackwell.
- Eveland, W. P., Marton, K., & Seo, M. (2004). Moving beyond “just the facts”: The influence of online news on the content and structure of public affairs knowledge. Communication Research, 31, 82–108. doi:10.1177/0093650203260203
- Eveland, W. P., & Schmitt, J. B. (2015). Communication content and knowledge content matters: Integrating manipulation and observation in studying news and discussion learning effects. Journal of Communication, 65, 170–191. doi:10.1111/jcom.12138
- Flesch, R. (1948). A new readability yardstick. Journal of Applied Psychology, 32, 221–233. doi:10.1037/h0057532
- Funke, R., Wieland, R., Schönenberg, S., & Melzer, F. (2013). Exploring syntactic structures in first-language education: Effects on literacy-related achievements. L1 Educational Studies in Language and Literature, 13, 1–24.
- Gibson, E. (1998). Linguistic complexity: Locality of syntactic dependencies. Cognition, 68, 1–76.
- Gordon, P. C., Hendrick, R., & Levine, W. H. (2002). Memory-load interference in syntactic processing. Psychological Science, 13, 425–430. doi:10.1111/1467-9280.00475
- Graber, D. A. (2001). Processing politics: Learning from television in the Internet age. Chicago, IL: University of Chicago Press.
- Heil, M., Rolke, B., & Pecchinenda, A. (2004). Automatic semantic activation is no myth: Semantic context effects on the N400 in the letter-search task in the absence of response time effects. Psychological Science, 15, 852–857. doi:10.1111/j.0956-7976.2004.00766.x
- Imai, K., Keele, L., & Tingley, D. (2010). A general approach to causal mediation analysis. Psychological Methods, 15, 309–334. doi:10.1037/a0020761
- Kane, J. V., & Barabas, J. (2019). No harm in checking: Using factual manipulation checks to assess attentiveness in experiments. American Journal of Political Science, 63, 234–249. doi:10.1111/ajps.2019.63.issue-1
- Kleinnijenhuis, J. (1991). Newspaper complexity and the knowledge gap. European Journal of Communication, 6, 499–522. doi:10.1177/0267323191006004006
- Koriat, A., Ackerman, R., Lockl, K., & Schneider, W. (2009). The memorizing effort heuristic in judgments of learning: A developmental perspective. Journal of Experimental Child Psychology, 102, 265–279. doi:10.1016/j.jecp.2008.10.005
- Koriat, A., & Ma’ayan, H. (2005). The effects of encoding fluency and retrieval fluency on judgments of learning. Journal of Memory and Language, 52, 478–492. doi:10.1016/j.jml.2005.01.001
- Lang, A., Park, B., Sanders-Jackson, A. N., Wilson, B. D., & Wang, Z. (2007). Cognition and emotion in TV message processing: How valence, arousing content, structural complexity, and information density affect the availability of cognitive resources. Media Psychology, 10, 317–338. doi:10.1080/15213260701532880
- Leroy, G., Helmreich, S., & Cowie, J. R. (2010). The influence of text characteristics on perceived and actual difficulty of health information. International Journal of Medical Informatics, 79, 438–449. doi:10.1016/j.ijmedinf.2010.02.002
- Liu, Y. I., Shen, F., Eveland, W. P., & Dylko, I. (2013). The impact of news use and news content characteristics on political knowledge and participation. Mass Communication & Society, 16, 713–737. doi:10.1080/15205436.2013.778285
- Lowrey, T. M. (1998). The effects of syntactic complexity on advertising persuasiveness. Journal of Consumer Psychology, 7, 187–206. doi:10.1207/s15327663jcp0702_04
- Lupia, A. (2016). Uninformed: Why people know so little about politics and what we can do about it. New York, NY: Oxford University Press.
- McCarthy, P. M., & Jarvis, S. (2010). MTLD, vocd-D, and HD-D: A validation study of sophisticated approaches to lexical diversity assessment. Behavior Research Methods, 42(2), 381–392. doi:10.3758/BRM.42.2.381
- Messing, S., & Westwood, S. J. (2014). Selective exposure in the age of social media: Endorsements trump partisan source affiliation when selecting news online. Communication Research, 41, 1042–1063. doi:10.1177/0093650212466406
- Mitkov, R. (2005). The Oxford handbook of computational linguistics. Oxford, UK: Oxford University Press.
- Nadkarni, S., & Gupta, R. (2007). A task-based model of perceived website complexity. MIS Quarterly, 31, 501–524.
- Neuman, W. R. (1986). The paradox of mass politics: Knowledge and opinion in the American electorate. Cambridge, MA: Harvard University Press.
- O’Keefe, D. J. (2003). Message properties, mediating states, and manipulation checks: Claims, evidence, and data analysis in experimental persuasive message effects research. Communication Theory, 13, 251–274. doi:10.1111/j.1468-2885.2003.tb00292.x
- Oppenheimer, D. M. (2006). Consequences of erudite vernacular utilized irrespective of necessity: Problems with using long words needlessly. Applied Cognitive Psychology, 20, 139–156. doi:10.1002/(ISSN)1099-0720
- Petty, R. E., Briñol, P., Tormala, Z. L., & Wegener, D. T. (2007). The role of metacognition in social judgment. In A. W. Kruglanski & E. T. Higgins (Eds.), Social psychology: Handbook of basic principles (pp. 254–284). New York, NY: Guilford Press.
- Petty, R. E., & Cacioppo, J. T. (Eds.). (1986). The elaboration likelihood model of persuasion. In Communication and persuasion: Central and peripheral routes to attitude change (pp. 1–24). New York, NY: Springer.
- Prior, M. (2014). Visual political knowledge: A different road to competence? The Journal of Politics, 76, 41–57. doi:10.1017/S0022381613001096
- Reah, D. (2002). The language of newspapers. New York, NY: Routledge.
- Rhee, J. W., & Cappella, J. N. (1997). The role of political sophistication in learning from news: Measuring schema development. Communication Research, 24, 197–233. doi:10.1177/009365097024003001
- Scheufele, D. A., & Tewksbury, D. (2006). Framing, agenda setting, and priming: The evolution of three media effects models. Journal of Communication, 57, 9–20.
- Schwarz, N. (2011). Feelings-as-information theory. In P. van Lange, A. Kruglanski, & E. T. Higgins (Eds.), Handbook of theories of social psychology (Vol. 1, pp. 289–308). Thousand Oaks, CA: Sage.
- Schwarz, N., Bless, H., Strack, F., Klumpp, G., Rittenauer-Schatka, H., & Simons, A. (1991). Ease of retrieval as information: Another look at the availability heuristic. Journal of Personality and Social Psychology, 61, 195–202. doi:10.1037/0022-3514.61.2.195
- Shulman, H. C., & Sweitzer, M. D. (2018). Varying metacognition through public opinion questions: How language can affect political engagement. Journal of Language and Social Psychology, 37(2), 224–237.
- Sotirovic, M. (2001). Effects of media use on complexity and extremity of attitudes toward the death penalty and prisoners’ rehabilitation. Media Psychology, 3, 1–24. doi:10.1207/S1532785XMEP0301_01
- Spirling, A. (2016). Democratization and linguistic complexity: The effect of franchise extension on parliamentary discourse, 1832–1915. The Journal of Politics, 78, 120–136. doi:10.1086/683612
- Strömbäck, J. (2005). In search of a standard: Four models of democracy and their normative implications for journalism. Journalism Studies, 6, 331–345. doi:10.1080/14616700500131950
- Suedfeld, P., & Tetlock, P. (1977). Integrative complexity of communications in international crises. Journal of Conflict Resolution, 21, 169–184. doi:10.1177/002200277702100108
- Tao, C. C., & Bucy, E. P. (2007). Conceptualizing media stimuli in experimental research: Psychological versus attribute-based definitions. Human Communication Research, 33, 397–426. doi:10.1111/j.1468-2958.2007.00305.x
- Tingley, D., Yamamoto, T., Hirose, K., Keele, L., & Imai, K. (2014). Mediation: R package for causal mediation analysis. Journal of Statistical Software., 59, 1–38. doi:10.18637/jss.v059.i13
- Tolochko, P., & Boomgaarden, H. G. (2018). Analysis of linguistic complexity in professional and citizen media. Journalism Studies, 19(12), 1786–1803.
- Tolochko, P., & Boomgaarden, H. G. (2019). Determining political text complexity: Conceptualizations, measurements, and application. International Journal of Communication, 13(21), 1784–1804.
- Wang, K., & Liu, H. (2000). Discovering structural association of semistructured data. IEEE Transactions on Knowledge and Data Engineering, 12, 353–371. doi:10.1109/69.846290
- Wells, G. L., & Windschitl, P. D. (1999). Stimulus sampling and social psychological experimentation. Personality and Social Psychology Bulletin, 25, 1115–1125. doi:10.1177/01461672992512005
- Winkielman, P., Schwarz, N., Fazendeiro, T., & Reber, R. (2003). The hedonic marking of processing fluency: Implications for evaluative judgment. In J. Musch & K. C. Klauer (Eds.), The psychology of evaluation: Affective processes in cognition and emotion (pp. 189–217). Mahwah, NJ: Erlbaum.