ABSTRACT
Two laboratory studies (N = 240) were designed to explain and predict how people make decisions in low-information political environments. Guided by feelings-as-information theory, it was argued that when direct democracy ballot issues do not receive any campaign expenditures and are not about moral/civic issues, voters are likely to encounter these ballots for the first time in the voting booth. And when this is the case, how these ballots are written should affect vote choice. In support of study hypotheses, it was found that the difficulty of the words on the ballot affected people’s processing fluency, defined as the ease with which people processed the information presented. In turn, self-reports of processing fluency influenced vote choice. Specifically, easier texts were more likely to be supported and difficult texts were more likely to be opposed or abstained from voting on. As hypothesized, this relationship was mediated through self-reports of processing fluency. Additionally, to demonstrate the external validity of this process, it was found that the voting results obtained in the two laboratory studies replicated real-world election results 86% of the time. These results offer communicative and psychological insight into how communication affects information processing, and how these processing experiences inform political decisions of consequence to everyday life.
How voters arrive at decisions when they have little to no information about the decision at hand is a critical question for democracy. Indeed, a substantial amount of research in communication, political science, and beyond (e.g., Berinsky et al., Citation2020; Bowler, Citation2015; Downs, Citation1957; Lupia, Citation1994; Nicholson, Citation2005) has examined how under conditions of low information people use decision aids, such as partisan cues, endorsements, or the physical attributes of candidates, to guide vote choice. Notably, this line of research has helped researchers to better understand the power of partisanship as a social identity (Green et al., Citation2002) and the (ir)rationality of various voter decisions (e.g., Nai, Citation2015). Despite these advancements, the context of direct democracy is a different sort of instance where voters are asked to make decisions on policies that are often complicated, nonpartisan, and receive little media attention. As shown by Burnett (Citation2019), voter knowledge of ballots about nonsocial issues, that also tend to receive little media attention, lags behind all other forms of political knowledge. Thus, focusing on ballots that do not concern social/moral issues, and do not receive campaign expenditures (a proxy for media attention), offers the opportunity to study political decision-making in a very low, yet very common, information environment. We presume, and empirically test, our claim that in these low information environments, voters are first exposed to these ballots in the voting booth and, as such, the way these ballots are written should affect vote choice. Thus, identifying how ballot wording affects voting decisions is an important democratic pursuit and interesting communication inquiry.
Guided by the literature in metacognition (Petty et al., Citation2007; Shulman & Bullock, Citation2019) and feelings-as-information theory (Schwarz, Citation2011), we find that the metacognitive feelings associated with processing difficulty predict how people vote on ballots. We also offer support for the external validity of this prediction by demonstrating across two lab studies using registered voters, and 64 ballots, that the decisions arrived at in the lab correspond with past and future election outcomes. Taken together, the goals of this work are to offer communicative and psychological insight into how word choice affects information processing and how these processing experiences inform decisions of consequence to everyday life.
Low Information Direct Democracy Elections
Ballot referenda, also known as initiatives, measures, propositions, and amendments, are a form of democracy that allows voters to vote directly on a piece of policy or legislation. If the measure is passed, it goes into law, and if it fails, it doesn’t. Direct democracy efforts are widespread: currently, in the U.S., all 50 states allow for some form of ballot measures (e.g., popular and/or legislative referendum; Bowler et al., Citation2020), as do 113 countries around the world (Ballotpedia, Citationn.d.-a). Despite the proliferation of direct democracy voting, there is substantial variation in the ballot topics, media attention, and campaign spending directed toward these ballots. This variability presents a challenge for making broad inferences about how people engage with these sorts of decisions. Understandably, much of the existing work on direct democracy has focused on issues that have been able to capture media attention, citizen interest, deliberation (Suiter & Reidy, Citation2020), and noteworthy political endorsements (e.g., same-sex marriage, Shi, Citation2016). In other words, much of the research in this area has focused on ballots in which ballot-relevant attitudes are expected to exist prior to Election Day (e.g., climate change or taxing corporations, see, Goldberg & Carmichael, Citation2017). Consistent with this expectation, research in this vein has convincingly demonstrated that, much like high information elections, the partisan framing of ballot issues and/or campaign spending in support of, or in opposition of, various ballots are strongly predictive of election outcomes (Bowler, Citation2015; Bowler & Donovan, Citation1998; Branton, Citation2003; Burnett, Citation2019; Burnett & Kogan, Citation2015; Damore & Nicholson, Citation2014; Lupia, Citation1994; Nicholson, Citation2003, Citation2005). What is less understood, however, is how voters make decisions when they are likely to possess little to no ballot-relevant attitudes prior to entering the voting booth (e.g., non-attitudes, Converse, Citation2006). Here, we define contexts in which people are likely to hold weak or non-attitudes about the decision under consideration as low information environments. One common and reoccurring low information political environment is low-salience ballot voting. Here, low-salience ballots are defined as ballots that receive zero dollars in campaign expenditures (and thus receive little to no media attention), are not about social/moral issues, nor are obviously partisan (Damore & Nicholson, Citation2014; Lupia, Citation1994). By focusing on how voters make decisions without the more commonly studied set of voting decision aids, new insights regarding political decision-making becomes possible.
Before reviewing research on direct democracy voting in general, our decision to focus on low-salience ballots merits investigation because, practically speaking, these types of initiatives are quite common. According to Ballotpedia (Citationn.d.-a), a nonpartisan digital encyclopedia of American elections, in 2018, of the 167 state-certified ballots, 30.5% classify as low-salience under our criteria. And, if local ballots were included in this count, this percentage would be much higher as many local issues surrounding bonds, sales tax rates, and local health ballots, for example, would qualify as low salience. Thus, given the prevalence of this low-information environment, an opportunity arises for communication scholars to offer insight into how word choice informs a common, and consequential, type of political behavior.
Research on direct democracy generally finds that the media environment, ballot topics, and citizen deliberation substantially affect voter awareness and subsequent vote choice (e.g., Carlin & Carlin, Citation1989; Suiter & Reidy, Citation2020). Nicholson’s (Citation2003) work, for example, finds that campaign expenditures can increase awareness of a ballot measure by an average of 16%. This same work also finds that social/moral issues, on average, have awareness rates that are 18% higher than nonsocial issues (defined as revenues and taxes, environment and resources, business regulation, education, welfare, and health). Furthermore, Burnett’s (Citation2019) study similarly found that voter knowledge of ballot initiative facts was substantially higher for moral issues and ballots that were well-financed and, accordingly, better publicized. To better contextualize these results, foundational work from Lupia (Citation1994) argued that voters use “shortcuts” including partisan cues, past behavior, and interest group campaigns, as a way to approximate real preferences when voting. Thus, taken together, this literature reveals that the type and amount of information voters hold about these ballots predictably impacts vote choice (see, Morisi et al., Citation2021). What is less understood, however, is how voters make decisions when the typical slate of informational cues is unavailable. As such, the goal of this study is to uncover a less considered source of influence within these environments: word difficulty.
Although research in direct democracy does not typically focus on low-salience ballots, there is some work that supports the claim that ballot wording could be a decision aid that guides vote choice under these conditions. Burnett and Kogan’s (Citation2015) survey experiment, for example, found that although the way ballots were framed initially influenced vote choice in favor of the frame, the presence of additional information – meant to emulate media attention in the real world – mitigated any framing effects. In addition to research on media effects, there has also been work examining the role of ballot language difficulty, given that many ballots contain words that are legalistic and likely unfamiliar to the average voter (Bowler & Donovan, Citation1998; Goldberg & Carmichael, Citation2017; Milita, Citation2015; Reilly & Richey, Citation2011; Shockley & Fairdosi, Citation2015). This research has found that language difficulty affects aggregate behavior, such as the likelihood of referenda passing (Milita, Citation2015), and individual behavior, such as voter roll-off and abstention (Reilly & Richey, Citation2011). Thus, taken together, experimental and cross-sectional work has shown that a) as media attention decreases, the influence of ballot wording increases, and b) ballot language difficulty can affect vote choice.
Though these findings inform the current investigation, to our knowledge no studies have examined these processes using real ballot measures and deploying methods that could isolate the communicative and psychological explanations that underlie these relationships. As such, the purpose of this investigation is to address these gaps in the literature and enhance our theoretical understanding of political decision-making in the process.
Word Difficulty and Processing Fluency
To enhance our understanding of political decision-making in these contexts, we set up a lab-based study that could test a psychological explanation for why vote choice is affected by word difficulty using metacognition. To theorize about this relationship, it is important to distinguish between two kinds of cognitions. The first, called primary cognition, refers to the declarative information and attitudes a person has in their memory (Petty et al., Citation2007; Schwarz, Citation2015; Shulman & Bullock, Citation2019). Primary cognitions affect our decision-making. When a ballot measure is introduced that has clear social or moral implications, people are able to access their existing value systems and decide whether to vote in support of the measure. So, for example, decision-making about ballots related to same-sex marriage should lead people to access primary cognitions such as religious beliefs, beliefs about civil rights, self-interests, etc. Declarative-based models of information processing state that people render a judgment based on their global assessment of their accessed primary cognitions. Although primary cognitions, or declarative-based models, are frequently used to explain why individuals arrive at particular decisions, in a low information environment, the availability of useful declarative information should be lacking. Under these circumstances, experience-based models may be more influential. Experience-based models are composed of secondary cognitions, also known as metacognition (Petty et al., Citation2007; Schwarz, Citation2015).
Metacognition refers to the thoughts people have about their thoughts or thought process (Schwarz, Citation2015). In other words, metacognition reflects how people feel about their information processing experience. Although there are many forms of metacognitive feelings, including emotions and moods while processing information (Schwarz, Citation2015), here we operationalize metacognition through the concept of processing fluency. Processing fluency can be defined as how easy or difficult information processing is experienced (Schwarz, Citation2015), or, put differently, how easy or difficult it feels to access primary cognitions. To convey how processing fluency is experienced, imagine being asked a difficult question. The feelings associated with something being hard (or easy) to understand and respond to reflects processing fluency.
Difficult or complex language has been reliably shown to affect processing fluency, such that as language gets more difficult, in terms of syntax, semantics, and/or word typicality (e.g., jargon), people report less fluent processing (Goldberg & Carmichael, Citation2017; Markowitz & Shulman, Citation2021; Shockley & Fairdosi, Citation2015; Shulman et al., Citation2020; Tolochko et al., Citation2019). For example, experimental work on the impact of scientific jargon revealed that the presentation of more specialized and less frequently used words produced lower processing fluency than when these words were replaced with more common or frequently used terms (Shulman et al., Citation2020). Within the political realm, three different papers by Sweitzer and Shulman (Citation2018, Shulman & Sweitzer (Citation2018b), Citation2018a) demonstrated that public opinion questions written with easier words produced higher reports of processing ease than the difficult version of these questions. And finally, Shockley and Fairdosi’s (Citation2015) experimental work applied these relationships to ballot referenda by randomly assigning participants to evaluate a complex or simple version of a hypothetical ballot measure. Consistent with expectations, those in the complex condition reported lower levels of processing ease than those in the simple condition (see also, Goldberg & Carmichael, Citation2017). In this study, we aim to complement and advance existing research by replicating the results from these experiments using unmanipulated ballot measures. Specifically, given that the presence of difficult, uncommon, or unfamiliar words (Milita, Citation2015) is both common within ballot measures and also reliably shown to affect processing fluency, an opportunity is presented to assess whether the known association between word difficulty and processing fluency generalizes to this consequential, real-world context.
H1: The presence of easier words on ballots will be positively associated with processing fluency.
Feelings-as-Information Theory and Vote Choice
In a low information environment, the declarative content voters can draw upon should be limited. This is where experience-based models, and specifically processing fluency experiences, might offer a more useful explanation for how people make decisions in these circumstances. To this end, feelings-as-information theory (FIT; Schwarz, Citation2011) can guide predictions for how processing fluency experiences will influence whether people support, oppose, or abstain from voting on low-salience ballots.
The first proposition of FIT states that people use metacognitive information in the same way as declarative information and that “different types of [metacognitive] feelings provide different types of information” (Schwarz, Citation2011, p. 32). The second postulate of FIT states that, “People usually experience their feelings as being ‘about’ whatever is in the focus of attention; this fosters the perception that incidental feelings are relevant” (Schwarz, Citation2011, p. 32). Together, these postulates predict that processing fluency, induced through the presence of common or uncommon words, will be attributed toward the ballot at hand. As such, existing research about the outcomes produced by a (dis)fluent processing experience should be informative.
One of the most reliable findings in the literature on processing fluency is that easier processing feels good and a difficult experience feels bad (for reviews see, Petty et al., Citation2007; Schwarz, Citation2015). Guided by proposition two of FIT, people should misattribute these positive or negative feelings toward the subject of one’s attention (Schwarz, Citation2011). To illustrate this Shulman and Sweitzer (Citation2018a, Citation2018b) observed that participants randomly assigned to public opinion questions written with simpler language reported more interest, knowledge, and efficacy in politics than those randomly assigned to the difficult language condition. For the current study, theory and evidence suggests that language difficulty should affect one’s processing experience, and the feelings compelled by this experience should be directed toward one’s vote choice in the direction consistent with the hedonic nature of processing fluency. Thus, one should expect that positive feelings, provoked by an easy experience, should engender support and negative feelings, provoked by a difficult experience, should lead to opposition (see also, Bowler & Donovan, Citation1998).
There is, however, an alternative way to interpret predictions guided by FIT. The second proposition of FIT further specifies that processing fluency provides information about how informed people are about the subject at hand (Schwarz, Citation2011). Specifically, an easy processing experience augments, and a difficult processing experience discounts, the perceived value of information. The perceived value of information refers to the perceived utility of the declarative information people are able to retrieve about a topic. An easier experience leads people to assume, with more confidence, that they possess adequate knowledge to proffer a decision (Schwarz, Citation2011). This is because an easy experience augments one’s perceived understanding of the issue. Conversely, a difficult experience leads to the discounting of information. When this is the case, a person may conclude that they do not possess enough quality information on the topic (Schwarz, Citation2011), and should be more likely to abstain because they don’t know which way to vote (Bowler & Donovan, Citation1998; Sweitzer & Shulman, Citation2018). Notably, this would lead to a slightly different expectation than the easy experience = support and difficult experience = oppose outcome. This work strives to offer a first step toward understanding the relationship between vote choice under conditions of easy, moderate, and difficult processing, and whether any of these conditions is more likely to result in a decision to abstain. Specifically, we assess whether an abstain vote better reflects a state between two affective poles (support and oppose) in ways similar to ambivalence or neutrality (H2, Bowler & Donovan, Citation1998; Song & Ewoldsen, Citation2015; Sweitzer & Shulman, Citation2018), or the feeling that one is not knowledgeable enough to vote (RQ1). Thus, because we are unsure whether low levels of processing fluency will result in a vote to oppose (H2), or a decision to abstain, the following hypothesis and research question is advanced:
H2: The relationship between word difficulty and vote choice will be mediated by processing fluency, such that an increase in the presence of easier words will lead to greater processing fluency which, in turn, will increase support for the ballot measure.
RQ1: What is the relationship between levels of processing fluency and outcomes of opposition and abstention?
Method
Participants
Participants for this study were 120 registered voters from Ohio.Footnote1 Participants were recruited through ResearchMatch, a volunteer service sponsored by the National Institutes of Health, and their voter registration was verified using publicly available voter records (60 females; Age M = 34.99, SD = 16.19, Range = 18–79; Race: White = 97, Black = 9, Latina/o/Hispanic = 3, Asian = 4, Mixed = 6, Other = 1; Partisan Affiliation: Democrat = 71, Independent = 37, Republican = 12). All subjects were compensated $40 for taking part in this study.
Materials
The stimuli for this study consisted of 40 statewide ballots drawn from 21 states (see supplementary materials for examples). Several ballots were used in accordance with a message-sampling approach (see, Slater et al., Citation2015)Footnote2 to better ensure that the scope of ballot topics (14 different topics were represented by this sample), and the range of word frequency scores, used in this sample was generalizable to the population of real low-salience ballots.
The 40 ballots chosen were selected from a larger data set of all statewide ballots (excluding ballots from Ohio) that were voted on during the 2012 general (n = 22) and 2014 midterm (n = 18) elections. These years were chosen to increase the likelihood that participants had not seen, remembered, nor previously voted on, these ballots. All stimuli were sourced from state boards of elections, the National Conference of State Legislatures (a nonpartisan advocacy organization), and Ballotpedia. This initial sampling frame included 307 statewide ballots from 43 states and was reduced to 40 ballots using the sampling procedure described below. All of the selected ballots are provided in the online supplement.
Stimuli Selection
The two goals of this sampling procedure were to (1) reduce the data set to only low salience ballots, operationalized as ballots that received zero dollars in campaign spending, and (2) to maximize variance in word difficulty.Footnote3 Of the 307 ballots collected, 191 received zero dollars (62.21%), thus qualifying as low-salience issues. With the remaining ballots, we removed any ballots with a word count greater than 125 words (n = 48) to ensure that the full text of the measure could be shown on a single screen during the lab study procedure. This left 143 ballots that were included in the next stage of stimuli selection.
Word Difficulty
Although we acknowledge that the latent construct of language difficulty can be operationalized in a variety of ways, we opted to use word frequency measures, which assess how commonly or frequently a word is used in the English language using the 2012 Google Ngram English fiction corpus (Google, Citation2013; see also, Michel et al., Citation2011). For this study, we decided to use the Google Ngram measure for three reasons. The first was that recent work (e.g., Benoit et al., Citation2019b; Richey & Taylor, Citation2020) advocates for the content validity of this measure over other commonly used metrics (e.g., Flesch-based measures of reading ease, see, Benoit et al., Citation2019b). Second, given that we were interested in information processing experiences resulting from written, rather than spoken, word, we used a measure obtained from a sample of books rather than transcriptions from voice recordings. Finally, other work examining the effects of language difficulty (Milita, Citation2015; Shulman et al., Citation2020) has found that processing difficulty arises out of the usage of unfamiliar and technical terms (i.e., semantic difficulty) as opposed to syntactical difficulty (as indexed in Flesch-based measures, see, Tolochko et al., Citation2019). Thus, using a measure that assesses word frequency – as a proxy for word familiarity – is a valid, contemporary, and likely to be influential measure of language difficulty.Footnote4
A word’s Ngram score is a count of the number of times that word appears in Google’s entire corpus of electronic books. Words with lower Ngram scores appear less frequently in English literature and should thus be less familiar to readers than words with higher scores (see, Benoit et al., Citation2019b). To produce an Ngram score for each ballot, we calculated the median Ngram score for the title and text of each remaining ballot. To maximize variance in this independent variable, the 20 “easy” ballots used in this study had an Ngram score above the median Ngram score, whereas the 20 “difficult” ballots selected had Ngram scores that were below the median. We then recalculated the Ngram scores for each ballot after removing counts for stop words, such as “the” or “of,” which have outlying Ngram scores, and which also contribute little to the meaning of the ballot.Footnote5 The average Ngram score, rescaled to the order of millions of uses, for each ballot was used in the analyses that follow. Overall, Average Ngram scores varied considerably in our stimuli: M = 2.00 million uses, SD = 1.16, range = 0.83–5.59 (all ballots used in this investigation are available in supplementary materials).
Pretest Survey
Because the stimuli for this study were actual ballots whose content varied in ways beyond word difficulty, we conducted a pretest to assess these differences. To do so, we administered a survey to a separate set of 101 registered voters from Ohio , also recruited through ResearchMatch and compensated $10 for their participation. Findings revealed that easy and difficult ballots differed significantly from one another for perceived familiarity, F (39, 1877) = 8.04, p < .001, interest, F (39, 1874) = 9.50, p < .001, and importance, F (39, 1877) = 8.34, p < .001. Given that the independent variable, word frequency, covaried with these ballots, we opted to control for the effects of familiarity (M = 2.30, SD = 0.54), interest (M = 3.22, SD = 0.53), and importance (M = 3.60, SD = 0.49) by including the ballot-level means from this pretest as covariates for hypothesis testing.Footnote6
Procedure and Measures
This study employed a repeated measures research design with word frequency serving as the independent variable. Thus, each participant was exposed to all 40 ballots that varied from easy to difficult in the ways discussed above. The order in which each ballot appeared was randomized, thus controlling for ballot order effects on the aggregate. Participants in the in-person lab study were tested individually in a quiet room placed in front of a computer screen. Participants were told at the beginning of the study that they would be reading and voting on ballots that were currently being considered in Ohio to enhance the relevance of the task. Participants were instructed to imagine that they were in a voting booth, to read each initiative carefully, and then vote. Participants were shown ballot measures on the computer screen in front of them. They held a controller that enabled them to advance to the next screen, which was where they made their vote choice. The screen after the ballot measure contained the words “Support,” “Oppose,” and “Abstain.” The order of these Vote Choices on the screen and their corresponding button locations were counterbalanced to ensure that observed effects were not due to a specific choice/button configuration. After reading and voting on all of the ballots, participants were asked to complete a follow-up survey of self-report items. Participants read each ballot measure again and responded to a three-item Processing Fluency scale (M = 4.86, SD = 1.57, α = .91; Shulman & Sweitzer, Citation2018bCitation2018),Footnote7 wherein higher scores reflect an easier processing experience. Participants then reported demographic information. All of our stimuli, measures, and data can be found on our open science framework page.
Study 1 Results
To test H1, H2, and RQ1, the structural equation models illustrated in were used. These mediation models were estimated using MPlus, which permits a number of specification options that pertain to this data. First, because our study uses repeated measures, we elected to cluster standard errors at the subject-level. Second, the dependent variable in tests of H2 and RQ1 (vote choice; oppose, abstain, or support) was treated as an ordinal categorical variable because the differences between abstain and oppose outcomes (and their relative ordering) was not yet known. Third, the mediating variable (processing fluency) was measured using three items; rather than creating a mean index of these items, structural equation models permit the construction of a latent processing fluency variable, allowing for mathematical inferences that reflect the abstraction of psychological processes (e.g., processing fluency).
Figure 1. Structural equation models of in-lab voting decisions on ballot measures by average Ngram score and reported processing fluency – Study 1. Vote choice was coded ordinally in both models; the left panel shows the oppose (0), abstain (1), support (2) coding scheme, while the right panel shows the abstain (0), oppose (1), support (2) coding scheme. Estimates, standard errors, confidence intervals, and effect sizes were calculated with maximum likelihood estimation. Standard errors were clustered at the subject-level (N = 120). The path between NGram scores and Fluency supports the hypothesized relationship from H1 and that the indirect path between NGram scores and Vote Choice is consistent with H2 regardless of the ordering of the Vote Choice DV.
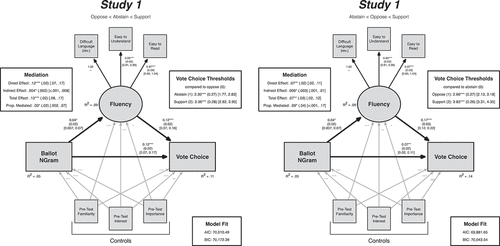
An Increase in Word Frequency Is Associated with an Increase in Processing Fluency (H1)
To test H1 – that word frequency and processing fluency would be positively associated – the path coefficient between word frequency and participants’ self-reported Processing Fluency was estimated. More specifically, this analysis included Average Ngram values of the ballots, on the scale of millions of uses, as the independent variable, and mean Familiarity, Interest, and Importance scores were included as controls. As presented in both models within , we found significant evidence in the predicted direction for the positive relationship between word frequency and processing fluency: B = 0.04, SE = 0.02, p < .05, R2 = .09. Thus, when ballots included more frequently used words, self-reports of processing fluency increased as well.
Processing Fluency Mediates the Relationship between Word Frequency and Vote Choice (H2)
To address H2 – that processing fluency would mediate the relationship between word frequency and voting decisions – we estimated the mediation model depicted in the left panel of . In this model, the dependent variable Vote Choice was coded as follows: support [2], abstain [1], and oppose [0]. Importantly, mediation effects (i.e., indirect effect, total effect, proportion of effects mediated) were estimated in the same structural equation modeling framework in MPlus. As shown in the left-panel of this figure, the indirect effect was significant and positive (B = .004, SE = .002, p < .05, 95% CI = [<.001, .008], R2 = .11), suggesting that word frequency is associated with a vote in support of a ballot as a result of increased processing fluency. The direct effect was also significant and positive in this model, indicating that the effect is not exclusively mediated through processing fluency. In sum, these findings support H2.
Word Frequency Affects Vote Choice Primarily through Processing Fluency at the Level of Support (RQ1)
To address research question one, we estimated the same mediation model as above, but recoded the ordinal dependent variable Vote Choice (support [2], oppose [1], and abstain [0]). These results are depicted in the right panel of . The results here are substantively similar: the indirect effects were significant and positive among lab self-reports (B = .006, SE = .003, p < .05, 95% CI = [.001, .01], R2 = .14). Moreover, in both models, the confidence intervals of the indirect effects overlap the estimates in the other model. These converging results may indicate that word frequency affects voting decisions through processing fluency at the level of support, but that the decision between abstention and opposition may depend on some as-yet-unmeasured characteristic of the ballot or the voter.
Figure 2. Structural equation modeling results with real-world voting outcomes for Studies 1 (left panel) and 2 (right panel). Processing fluency was measured among lab participants in these models. Estimates shown with standard errors in parentheses and 95% confidence intervals in brackets. As these figures indicate, the indirect path between ballot wording and real-world passage rates, mediated through fluency, was supported across both studies.
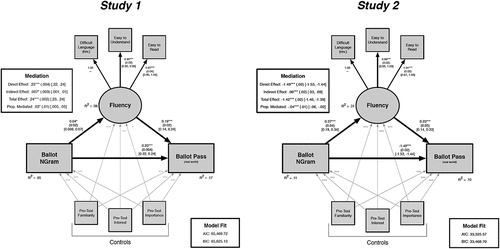
Aggregate Votes in the Lab Predicted Real-World Aggregate Votes
Finally, to understand whether these results replicate, and in turn generalize, to the real-world elections these ballots were chosen from, we ran two analyses. The first provided a global assessment of whether the “election” results from our in-lab study produced the same result as the real-world election using the appropriate state-level election rules (Ballotpedia, Citationn.d.-b). To do so, we converted support rates into a pass/fail categorical variable for both in-lab responses and real-world elections.Footnote8 We then conducted a chi-squared test to determine if the observed rates of similar results (i.e., ballots that pass/fail in real-world elections also pass/fail among lab participants) occur above and beyond chance. The results of this test were significant, χ2(1) = 10.21, p < .01. Of the 40 ballots, 34 (85%) had the same result (28 pass, 6 fail) in both the lab “election” and in their real-world election. Just 6 ballots (15%) had different outcomes: 4 passed in the lab but failed in the real-world, and 2 failed in the lab but passed in the real-world. In addition to this analysis, we also estimated the same mediational model used to test H1, H2, and the RQ1 (described in detail below) but replaced laboratory outcomes with real-world ballot passage rates (0: fail, 1: pass) as the dependent variable. This allowed us to test whether a ballot’s NGram score and aggregated processing fluency score (obtained using the lab participants), could be used to explain ballot passage rates in the real-world election. In sum, the results from this analysis revealed that word frequency, with processing fluency as the mediator, predicted real-world ballot passage rates, B = .007, SE = .003, p < .05, 95% CI = [.001, .01]. Together, these analyses demonstrate the generalizability of these relationships.
Study 1 Discussion
The purpose of study 1 was to understand whether word difficulty could affect vote choice through processing fluency. The results from our laboratory study revealed that ballots written with more frequently used words were also easier to process. And, when ballots were easy to process, participants were more likely to support the measure. Furthermore, we provide evidence that the processes observed in the lab appear to emulate the processes that occur in real elections. Together, this evidence is both practically important and theoretically compelling.
Theoretically, examining political decision-making within this context offered a broader opportunity to study the decision aids people use to make decisions when little prior, or outside, information is available. Some research on direct democracy has revealed that endorsements (e.g., Lupia, Citation1994), the presence of scientific evidence (e.g., Stucki et al., Citation2018), or perceptions of argument quality (e.g., Nai, Citation2015) affect voting decisions. The findings obtained here contribute to this literature by adding a theoretical explanation for why word difficulty can be another influential decision aid within low-information environments.
Finally, it is noteworthy that the influence of word difficulty on vote choice (through fluency) persisted across 40 different ballots that varied in ways beyond word difficulty. Thus, despite an abundance of alternative explanations for why a person would support or oppose a ballot (that should manifest as error in our design), word difficulty, and the mediating effect of processing fluency, still produced a statistically significant relationship and strong to medium sized effects (.09 < R2 < .14). Given the important practical and theoretical utility of this finding, Study 2 was conducted to replicate this effect.
Study 2
Study 1 provided initial evidence that subjective experiences while processing difficult versus easily worded information informs decision-making. The aims of Study 2 were to try and replicate this effect using different ballots, and to shed more light on the processes that underlie an abstain versus opposition vote choice. Thus, hypotheses one and two, along with research question one were tested again using a different sample of ballots from an upcoming election using a new sample of participants.
Method
The method for Study 2 was identical to Study 1, with the exceptions discussed below.
Participants
Participants for Study 2 were 120 registered voters from Ohio recruited through ResearchMatch. Participants from Study 1 and pretest survey participants were ineligible to participate in Study 2 (60 females; Age M = 33.98, SD = 18, Range = 18–73; Race: White = 103, Black = 9, Asian = 4, Mixed = 3, Other = 1; Partisan Affiliation: Democrat = 61, Independent = 29, Republican = 30).
Materials
The stimuli for this study consisted of 24 statewide ballots from 11 states (see Appendix A for an example). These 24 ballots were selected from a larger data set, sourced by the researchers using the same procedure described in Study 1. In total, there were 142 statewide ballots from 35 states that were available to the public at the time of this data collection (Summer 2018) and set to be voted on during the 2018 midterm election. These 142 ballots were reduced to 24 in accordance with the sampling procedure described in Study 1. Ballots that received financial support or that contained more than 125 words were removed first, leaving 58 ballots. A median Ngram score was determined for the entire data set and then 12 ballots which had a median Ngram score below the set’s median were selected for use as difficult ballots, and 12 ballots whose median Ngram score was above the set’s median were selected for use as easy ballots. As in Study 1, we then recalculated Average Ngram scores – again, on the scale of millions of uses – after removing stop words. Notably, although the ballots used in Study 2 contained somewhat more obscure words compared to the stimuli in Study 1, M = 1.68 million uses, SD = 0.61, range = 0.51–2.74, Ngram scores were not significantly different between Studies 1 and 2, t (62) = 1.26, p = .21, r2 = .02.
Pretest
Once again, a pretest survey was used to assess whether these ballots varied in ways beyond word difficulty. To make this assessment, 102 registered voters in Ohio assessed the selected 24 ballots (see supplement). Participants were compensated $10 for their participation. Survey findings revealed that the easy ballots scored significantly higher in perceived familiarity, F (23, 2315) = 17.59, p < .001, interest, F (23, 2317) = 19.13, p < .001, and importance, F (23, 2312) = 16.79, p < .001, than difficult ballots. As such, we elected to include ballot-level means for familiarity (M = 2.90, SD = 0.55), interest (M = 3.69, SD = 0.51), and importance (M = 3.99, SD = 0.46), from our pretest as covariates in hypothesis testing.
Procedure and Processing Fluency
The design and procedures from study 1 were replicated in study 2. Once again, this study used word frequency as the independent variable across 24 ballots. Participants read each ballot on a screen and were asked to make a Vote Choice between support, oppose, or abstain. Ballot order and decision position were counterbalanced between subjects. Following voting decisions for all 24 ballots, participants read each ballot again on a separate survey and responded to the same three-item Processing Fluency scale (M = 4.57, SD = 1.73, α = .94).
Study 2 Results
An Increase in Word Frequency Is Associated with an Increase in Processing Fluency (H1)
The statistical tests for Study 2 were the same as those from Study 1. To test H1, we estimated a structural equation model in which (among the other modeled paths; see, ) participants’ mean Processing Fluency evaluation of each initiative served as the dependent variable, and Average Ngram values on the scale of millions of uses served as the independent variable. Familiarity, importance, and interest were included as controls, and a subject identifier was used to cluster standard errors. Consistent with expectations, there was a significant and positive relationship between word frequency and processing fluency; B = 0.27, SE = 0.04, p < .001, 95% CI = [0.19, 0.34], R2 = .31. These results offer support for H1 and replicate the findings obtained from Study 1.
Figure 3. Structural equation models of in-lab voting decisions on ballot measures by average Ngram score and reported processing fluency – Study 2. Vote choice was coded ordinally in both models; the left panel shows the oppose (0), abstain (1), support (2) coding scheme, while the right panel shows the abstain (0), oppose (1), support (2) coding scheme. Estimates, standard errors, confidence intervals, and effect sizes were calculated with maximum likelihood estimation. Standard errors were clustered at the subject-level (N = 120). The path between NGram scores and Fluency supports the hypothesized relationship from H1 and that the indirect path between NGram scores and Vote Choice is consistent with H2 regardless of the ordering of the Vote Choice DV.
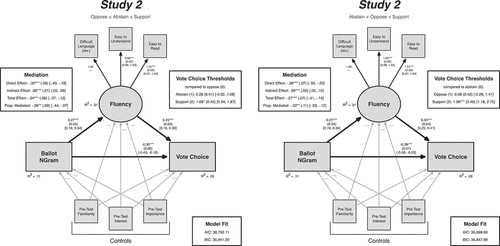
Processing Fluency Mediates the Relationship between Word Frequency and Vote Choice (H2)
Hypothesis two was tested using the same full structural equation model used in Study 1. Importantly, the dependent variable – Vote Choice – was coded ordinally with opposition assigned the lower value (0) relative to abstention (1). The results of this model are shown in the left-panel of . The indirect effect was positive and significant, supporting our hypothesis of mediated effects (B = .06, SE = .01, p < .001, 95% CI = [.03, .09], R2 = .05). Interestingly, the direct effect of Average Ngram on Vote Choice was significant and negative after accounting for the mediated effect through Processing Fluency. This could be the biproduct of a few important differences between Study 1 and Study 2. Namely, the indirect effect is much stronger in Study 2 than in Study 1, and this difference should influence the direct effect between word frequency and vote choice. Moreover, ballot word frequency was slightly more difficult in Study 2 than Study 1, which may also account for these differences. Taken together, however, the relationship posited by H2 was supported and replicates the finding from Study 1.
Word Frequency Affects Vote Choice Primarily through Processing Fluency at the Level of Support (RQ1)
To address RQ1, we again reversed the order of the abstain (0) and oppose (1) Vote Choices and estimated the equivalent models to those used in the test of H2. The results are presented in the right panel of . Once more, the results of these tests are substantively similar to our tests of H2 and show a significant indirect effect in the expected direction (B = .09, SE = .02, p < .001, 95% CI = [.05, .12], R2 = .09). In fact, all of the confidence intervals of the estimates in the model for RQ1 overlap with the estimates in the H2 model, indicating no significant differences in the indirect effects between dependent variable specifications. This bolsters the results from Study 1: the effects of word frequency and processing fluency on voting decisions are likely at the level of support and not at the level of abstain versus oppose.
Aggregate Votes in the Lab Predicted Real-World Aggregate Votes
And finally, to understand the generalizability of these relationships we again examined whether the lab results replicate real-world election results by converting voting percentages into a pass/fail categorical measure for both real-world elections and our lab participants. Again, we found that the rates of co-occurring results (e.g., pass/pass and fail/fail) significantly differed from chance: χ2(1) = 5.05, p < .05. Specifically, among the 24 ballots in Study 2, 21 (87.5%) had the same outcome in both the real-world election and in the lab election: 18 passed and 3 failed. Of the 3 ballots (12.5%) which had differing results, 2 passed in the lab and failed in the real-world, and 1 passed in the real-world while failing to pass in the lab. In addition to these analyses, we also estimated a mediational model with real-world ballot passage rates (0: fail, 1: pass) as the dependent variable. The results from this analysis revealed support for the positive indirect effect between word frequency and real-world ballot passage rates, B = .06, SE = .02, p < .001, 95% CI = [.03, .09], with processing fluency as the mediator. These results are presented in full in the right panel of . Together, these results support the generalizability of the focal relationships such that the same ballots that tend to pass (fail) in our study also tend to pass (fail) in the real world, and these pass and failure rates can be explained by ballot word difficulty and in-lab reports of processing fluency.
General Discussion
The purpose of these studies was to apply theory in practice and, in doing so, better understand how people make decisions in low information political environments. In addition to providing communicative and psychological explanations for how these types of decisions are made, we also tested these ideas within the context of a consequential and commonly occurring low information environment – direct democracy voting. The results from two carefully controlled laboratory studies, and across 64 ballots, offer compelling evidence for the role of experience-based models of decision-making within these contexts. Notably, we find that feelings of difficulty or ease, evoked by word difficulty, offer incidental information that is attributed toward the ballot being read. Across two studies, we found support for the notion that ballots written with more frequently used words (i.e., easier) compelled an easier experience that led to a higher likelihood of ballot support. Moreover, the decisions arrived at using this experience-based model produced decisions identical to both past and future elections 86% of the time, despite our use of a small, non-representative sample. These findings provide support for the role of communication and processing fluency in explaining how people make decisions.
Of note, the obtained associations between word difficulty, processing fluency, and voting, were supported across 64 ballots that spanned 11 different issue topics that varied greatly in substance and content. In fact, pretests revealed that participants reported that the substance of these ballots were differentially interesting, familiar, and important. Yet nevertheless, even when controlling for these important informational differences, a consistent pattern of participants relying on their experience was still observed. This pattern supports FIT (Schwarz, Citation2011) in theoretically important ways. Specifically, the notion that feelings are privileged over declarative information provides a powerful demonstration of the guiding role of our experiences when informational value is discounted. Recall that in FIT the discounting effect occurs when participants discount the utility of their declarative information. Under these conditions, people feel less certain, less confident, and more ambivalent about their opinions (Schwarz, Citation2011), whereas under conditions of easy processing people are more likely to use their positive affect as information. The results from our experiments support these processes. Namely, under conditions of easy processing, people offered greater support for ballot measures than under difficult processing.
Although this work was tested within the context of direct democracy ballots, it merits mentioning that the processes observed here should generalize to other low-information contexts, political and beyond. We found here that word difficulty evokes a predictable experience: An easy experience promotes a more positive experience, and a difficult experience engenders negativity (all else being equal). When a person is asked to render a judgment (political or otherwise) and does not possess much information about the subject of this judgment, our data (and FIT) propose that people use their information processing experience to guide their decision. There is a rich literature on political decision making aids (see, Downs, Citation1957; Green et al., Citation2002; Lupia, Citation1994) because scholars have long been fascinated with the cues people use to make complicated decisions. Although decision making aids have been well-studied, an examination of the types of cues people rely upon in low-information contexts is not as well understood. Specifically, in high-profile elections, and thus high-information political environments, people rely upon common cues such as endorsements, source cues, partisan cues, and status quo biases (Branton, Citation2003; Damore & Nicholson, Citation2014; Lupia, Citation1994; Morisi et al., Citation2021; Nicholson, Citation2005). When these cues are unavailable, however, we found that experience-based feelings, evoked by word frequency, could guide decisions instead. Although this work suggests that word difficulty serves as a cue that guides behavior for low-salience ballots, moving forward, it is important to contextualize the direction and magnitude of this effect in contrast to more well-known processes such as voting behavior in high-salience elections. Given that we only tested low-salience ballots, the degree of difference between these contexts remains unknown and thus merits future investigation.
Another benefit of more direct comparisons between voting behavior under conditions of high versus low information, or strong versus weak preexisting attitudes, is the ability to understand how the findings obtained here comport with related work. Goldberg and Carmichael’s (Citation2017) experiment, for instance, similarly examined the role of ballot language complexity on processing ease and policy favorability. Unlike the current study, however, Goldberg and Carmichael (Citation2017) considered the moderating role of preference-consistency on the relationship between language complexity and policy support. Similar to the results obtained here, Goldberg and Carmichael (Citation2017) found that when participants expressed no preference (e.g., non-attitudes, Converse, Citation2006), simply worded ballots were more likely to be supported than complex ballots (see also, Bowler & Donovan, Citation1998). Interestingly, however, when the impact of one’s initial policy preferences were taken into consideration, the effect of language complexity and processing ease became more nuanced. Specifically, in the preference-consistent condition it was found that the simply worded version of the ballot measure was more supported than the complex version. In the preference-inconsistent condition, however, the simply worded ballot was rated more negatively than the complex version. Notably, this pattern of results can also be explained by FIT (Schwarz, Citation2011) which proposes that the value of information (i.e., the ballot information under consideration) is augmented under conditions of fluent processing and discounted under conditions of disfluent processing. Thus, simpler forms of language can produce more “accurate,” or more preference-consistent, decisions in ways similar to other, more commonly studied, political heuristics (see also, Lupia, Citation1994; Morisi et al., Citation2021; Shulman & Sweitzer, Citation2018a).
When integrating these ideas, existing work suggests that in low-information environments the impact of word difficulty seems to predict, through fluency, whether ballots pass or fail, and in high-information environments, word difficulty can positively impact people’s ability to vote their preferences (Bowler & Donovan, Citation1998). Thus, word difficulty can affect political decision-making in a variety of ways, some of which are normatively positive for democracy, some of which might not be. Although work on language complexity as a decision-aid is relatively new, hopefully these ideas inspire future thinking on the role of communication, and more specifically word difficulty, in consequential decision-making environments.
Despite these contributions, there were some limitations and areas that could benefit from further research. First, like all observational studies, endogeneity is a concern that prevents us from having a strong causal interpretation of our results. For example, it is possible that unmeasured ballot characteristics such as an ideological lean (see, Branton, Citation2003; Damore & Nicholson, Citation2014), or unmeasured environmental characteristics, such as notable endorsements or general support, could have been driving the relationships under investigation. Although we attempted to minimize these possibilities methodologically, by limiting our sample to low-salience ballots from across the country, and statistically by including control variables reflecting general support, our inability to control for these possibilities reflects the trade-off inherent in our decision to use real, as opposed to hypothetical or manipulated, ballot measures. This decision could reflect why, despite the fact we found support for our mediated voting models as predicted, direct effects were observed as well. This suggests that there are still unmeasured properties of these ballots that account for vote choice beyond word difficulty, processing fluency, familiarity, interest, importance, and party identification and future researchers may wish to use alternative designs to investigate these processes.
Second, as previously mentioned, the antecedents for an oppositional vote and an abstention vote remain unclear. Future research would be well served to better understand what communication and psychological phenomena account for these different behavioral decisions. Third, we acknowledge that the causal effects we propose remain conjectural due to our lack of a between-subjects experimental method and our methodological decision to have participants offer a vote before reporting on processing fluency. Although the causal relationship we hypothesized was predicated upon theory, our pretest data, and an abundance of experimental work in this domain (for a review see, Shulman & Bullock, Citation2019), future work should utilize different study designs to offer stronger methodological support for these causal claims. Fourth, these studies relied on only one, semantic-based, measure of language difficulty. Although recent work (e.g., Tolochko et al., Citation2019) has observed that semantic-based measures, such as Ngram, are more influential than syntactic-based measures (e.g., Flesch-based measures), future work would be benefited by a more robust understanding of how different features of language (including other operationalizations of difficulty) affect the relationship between ballot language and vote choice. And finally, we acknowledge that some of the methodological decisions guiding this study hindered our ability to make broadly generalizable claims. Specifically, our decision to use low-salience ballots impaired our ability to make broader claims about how people make decisions for high-salience ballots. Additionally, we did not take any steps toward ensuring that our lab sample was politically representative of the U.S. as a whole. Still, we found that our lab results mirrored election results even without a representative sample and without modeling influential political variables such as partisanship, political knowledge and participation. Nevertheless, future work should endeavor to replicate and extend our understanding of these processes by using a more politically representative sample and by modeling potentially important individual differences.
In conclusion, this research strove to integrate ideas within the communication, metacognition, and direct democracy literatures to better understand how people behave in low information environments. We found compelling support for the notion that metacognitive feelings guide decision-making within this domain and that communication features, independent of communication content, can guide these experiences. We hope these findings can be used to inspire future work into how communication and processing fluency can explain how people make decisions that affect everyday life.
Open Scholarship
This article has earned the Center for Open Science badge for Open Materials. The materials are openly accessible at https://osf.io/2q7cw/?view_only=15f95ed1a4a476099d0244b7dfba919.
Supplemental Material
Download MS Word (42.6 KB)Disclosure Statement
No potential conflict of interest was reported by the author(s).
Data availability statement
The data described in this article are openly available in the Open Science Framework at https://osf.io/2q7cw/?view_only=15f95ed1a4a476099d0244b7dfba919.
Supplementary Material
Supplemental data for this article can be accessed on the publisher’s website at https://doi.org/10.1080/10584609.2022.2092920
Additional information
Funding
Notes on contributors
Hillary C. Shulman
Hillary C. Shulman, Ph.D. is an Associate Professor in the School of Communication at The Ohio State University. Her work examines how communication can be used to stimulate engagement in the areas of politics, health, and science.
Matthew D. Sweitzer
Matthew D. Sweitzer, Ph.D. is a recent graduate of the Ohio State University School of Communication and a postdoctoral fellow at Sandia National Laboratories. His research explores the spread of information in social networks through a variety of computational methods. Note: this paper and associated research are in no way affiliated with Sandia National Laboratories; any views expressed here are solely those of the authors.
Olivia M. Bullock
Olivia M. Bullock, Ph.D. is an Assistant Professor in the Department of Organizational Sciences and Communication at George Washington University. Her research focuses on message design strategies that can reduce the ideologically motivated processing of political, science, and health information.
Jason C. Coronel
Jason C. Coronel, Ph.D. is an Associate Professor in the School of Communication at The Ohio State University. His work examines the psychological processes that underlie political decision making.
Robert M. Bond
Robert M. Bond, Ph.D. is an Associate Professor in the School of Communication at The Ohio State University. His research interest is in political behavior and attitudes with a specific interest in how our social networks influence our political behavior and communication.
Shannon Poulsen
Shannon Poulsen is a doctoral candidate in the School of Communication at The Ohio State University. She is broadly interested in how people become, and stay, politically misinformed and the role of humor as a source of, and solution to, misinformation.
Notes
1. This study is part of a larger project examining the effect of ballot language on voting decisions. This is the second manuscript from this project. Other data are not reported here and are reported in a separate article (Coronel et al., Citation2021).
2. The message-sampling approach refers to technique in which researchers use messages sampled from the population of real messages as stimuli for their study (see, Slater et al., Citation2015). As argued by authors, the goal of this approach is to address generalizability concerns in communication research, while also being able to infer with more confidence how a particular feature of message design impacts audiences using a variety of instantiations of this feature and within a variety of settings. Consistent with this approach, the current study selected a sample of real low-salience ballots from the population of ballot proposals of this kind. Please see the cited work for more information on the utility of this methodological approach.
3. Because the ballots chosen varied by topic (11 subtopics were represented) alongside word difficulty, we ran a chi-square to assess whether our primary variable (word difficulty: easy, hard) covaried with topic in ways that could impact our primary relationship. The results of this analysis found that there was no significant association between topic category and word difficulty (Study 1: F (6, 33) = .93, p = .49; Study 2: F (9, 14) = 1.28, p = .33), indicating that topic categories were randomly distributed across our word difficulty measure.
4. We ran all of our primary analyses using Flesch reading ease, Flesch-Kincaid grade level, and SUBTLEX-US measures as well (results from the reading ease analyses are presented in the online supplement). However, comparing and contrasting results across these analyses is not straight-forward due to our removal of stop-words for the Ngram measure (Benoit et al., Citation2019a), and the lack of availability of words in the SUBTLEX-US dictionary. In sum, though the results from analyses using SUTBLEX-US as the independent variable replicate the findings presented here, we did not find support for our hypotheses using reading ease and grade level measures as the independent variable. These divergent results, however, are not particularly surprising given that Ngram can be considered a semantic-measure of language difficulty whereas Flesch-based measures are syntactically based, and these metrics have been shown to perform differently in studies on political information processing (see, Tolochko et al., Citation2019).
5. We used the SMART stop word dictionary available in the “stopwords” package in the R statistical software (Benoit et al., Citation2019a).
6. More information about this pretest is included in the supplemental materials.
7. For consistency with the survey responses, we also elected to remove the item “A lot of the information presented was new to me.” from the processing fluency scale. Doing so provided a more reliable scale of processing fluency (α = .91) compared to the same scale with this item included (α = .81). The three retained items were: 1) The ballot measure I just read was easy to read; 2) Overall, I found the language used in this ballot measure to be difficult (reverse-coded); 3) It was easy for me to understand the information presented.
8. In most cases, “pass” equates to greater than 50% of the votes cast in support of a measure. However, ballots in Colorado (n = 1) require >55% of support to pass, while ballots in both Florida (n = 2) and Illinois (n = 1) require >60% of support to pass (Ballotpedia, Citationn.d.-b). These ballots were coded accordingly. Results of the same test in which all ballots are considered to have passed if more than 50% of the votes support the measure produced substantively similar results.
References
- Ballotpedia. (n.d.-a). Ballot measure campaign finance, 2018. Retrieved October 14, 2019, from https://ballotpedia.org/Ballot_measure_campaign_finance,_2018
- Ballotpedia. (n.d.-b). Supermajority requirement. Retrieved July 15, 2019, from https://ballotpedia.org/Supermajority_requirement
- Benoit, K., Muhr, D., & Watanabe, K. R Foundation for Statistical Computing, Vienna, Austria. (2019a Stopwords: The R package). (). https://stopwords.quanteda.io/
- Benoit, K., Munger, K., & Spirling, A. (2019b). Measuring and explaining political sophistication through textual complexity. American Journal of Political Science, 63(2), 491–508. https://doi.org/10.1111/ajps.12423
- Berinsky, A. J., de Benedictis-Kessner, J., Goldberg, M. E., & Margolis, M. F. (2020). The effect of associative racial cues in elections. Political Communication, 37(4), 512–529. https://doi.org/10.1080/10584609.2020.1723750
- Bowler, S., & Donovan, T. (1998). Demanding choices: Opinion, voting, and direct democracy. University of Michigan Press.
- Bowler, S. (2015). Information availability and information use in ballot proposition contests: Are voters over-burdened? Electoral Studies, 38, 183–191. https://doi.org/10.1016/j.electstud.2015.02.002
- Bowler, S., Dobbs, R., & Nicholson, S. (2020). Direct democracy and political decision making. In Oxford research encyclopedias. Oxford University Press.
- Branton, R. P. (2003). Examining individual-level voting behavior on state ballot propositions. Political Research Quarterly, 56(3), 367–377. https://doi.org/10.1177/106591290305600311
- Burnett, C. M., & Kogan, V. (2015). When does ballot language influence voter choices? Evidence from a survey experiment. Political Communication, 32(1), 109–126. https://doi.org/10.1080/10584609.2014.894160
- Burnett, C. M. (2019). Information and direct democracy: What voters learn about ballot measures and how it affects their votes. Electoral Studies, 57(November), 223–244. https://doi.org/10.1016/j.electstud.2018.12.001
- Carlin, D. P., & Carlin, J. (1989). A typology of communication functions in ballot issue campaigns. Political Communication, 6(4), 229–248. https://doi.org/10.1080/10584609.1989.9962877
- Converse, P. E. (2006). The nature of belief systems in mass publics (1964). Critical Review, 18(1–3), 1–74. https://doi.org/10.1080/08913810608443650
- Coronel, J. C., Bullock, O. M., Shulman, H. C., Sweitzer, M. D., Bond, R. M., & Poulsen, S. (2021). Eye movements predict large-scale voting decisions. Psychological Science, 32(6), 838–848. https://doi.org/10.1177/0956797621991142
- Damore, D. F., & Nicholson, S. P. (2014). Mobilizing interests: Gorup participation and competition in direct democracy elections. Political Behavior, 36(3), 535–552. https://doi.org/10.1007/s11109-013-9252-2
- Downs, A. (1957). An economic theory of democracy. Addison Wesley.
- Goldberg, M. H., & Carmichael, C. L. (2017). Language complexity, belief-consistency, and the evaluation of policies. Comprehensive Results in Social Psychology, 2(2–3), 1–17. https://doi.org/10.1080/23743603.2017.1404427
- Google. (2013). Google Books: Ngram Viewer. http://storage.googleapis.com/books/ngrams/books/datasetsv2.html
- Green, D., Palmquist, B., & Schickler, E. (2002). Partisan hearts & minds: Political parties and the social identities of voters. Yale University Press.
- Lupia, A. (1994). Shortcuts versus encyclopedias : Information and voting behavior in California insurance reform elections. American Political Science Review, 88(1), 63–76. https://doi.org/10.2307/2944882
- Markowitz, D. M., & Shulman, H. C. (2021). The predictive utility of word familiarity for online engagements and funding. Proceedings of the National Academy of Sciences of the United States of America, 118(18), 1–7. https://doi.org/10.1073/pnas.2026045118
- Michel, J. B., Shen, Y. K., Aiden, A. P., Veres, A., Gray, M. K., Team, T. G. B., Aiden, E. L., Clancy, D., Norvig, P., Orwant, J., Pinker, S., Nowak, M. A., & Aiden, E. L. (2011). Quantitative analysis of culture using millions of digitized books. Science, 331(6014), 176–182. https://doi.org/10.1126/science.1199644
- Milita, K. (2015). Election laws and agenda setting: How election law restrictiveness shapes the complexity of state ballot measures. State Politics and Policy Quarterly, 15(2), 119–146. https://doi.org/10.1177/1532440015575901
- Morisi, D., Colombo, C., & De Angelis, A. (2021). Who is afraid of a change? Ideological differences in support for the status quo in direct democracy. Journal of Elections, Public Opinion and Parties, 31(3), 309–328. https://doi.org/10.1080/17457289.2019.1698048
- Nai, A. (2015). The maze and the mirror: Voting correctly in direct democracy. Social Science Quarterly, 96(2), 465–486. https://doi.org/10.1111/ssqu.12154
- Nicholson, S. P. (2003). The political environment and ballot proposition awareness. American Journal of Political Science, 47(3), 403–410. https://doi.org/10.1111/1540-5907.00029
- Nicholson, S. P. (2005). Voting the agenda: Candidates, elections, and ballot propositions. Princeton University Press.
- Petty, R. E., Brinol, P., Tormala, Z. L., & Wegener, D. T. (2007). The role of metacognition in social judgment. Social Psychology: Handbook of Basic Principles, 2(1994), 254–284. https://doi.org/10.1016/j.eplepsyres.2008.03.002
- Reilly, S., & Richey, S. (2011). Ballot question readability and roll-off: The impact of language complexity. Political Research Quarterly, 64(1), 59–67. https://doi.org/10.1177/1065912909349629
- Richey, S., & Taylor, J. B. (2020). Google Books Ngrams and political science: Two validity tests for a novel data source. PS - Political Science and Politics, 53(1), 72–78 doi:https://doi.org/10.1017/S1049096519001318.
- Schwarz, N. (2011). Feelings-as-information theory. In P. Van Lange, A. Kruglanski, & E. T. Higgins (Eds.), Handbook of Theories of Social Psychology: Volume 1 (pp. 289–308). Sage Publications. https://doi.org/10.4135/9781446249215.n15
- Schwarz, N. (2015). Metacognition M. Mikulincer, P. R. Shaver, E. Borgida, J. A. Bargh . In APA Handbook of Personality and Social Psychology: Vol. 1 Attitudes and Social Cognition (American Psychological Association) (pp. 203–229). https://doi.org/10.1037/14341-006
- Shi, Y. (2016). Cross-cutting messages and voter turnout: Evidence from a same-sex marriage amendment. Political Communication, 33(3), 433–459. https://doi.org/10.1080/10584609.2015.1076091
- Shockley, E., & Fairdosi, A. S. (2015). Power to the people? Psychological mechanisms of disengagement from direct democracy. Social Psychological and Personality Science, 6(5), 579–586. https://doi.org/10.1177/1948550614568159
- Shulman, H. C., & Sweitzer, M. D. (2018a). Advancing framing theory: Designing an equivalency frame to improve political information processing. Human Communication Research, 44(2), 155–175. https://doi.org/10.1093/hcr/hqx006
- Shulman, H. C., & Sweitzer, M. D. (2018b). Varying metacognition through public opinion questions: How language can affect political engagement. Journal of Language and Social Psychology, 37(2), 224–237. https://doi.org/10.1177/0261927X17707557
- Shulman, H. C., & Bullock, O. M. (2019). Using metacognitive cues to amplify message content: A new direction in strategic communication. Annals of the International Communication Association, 43(1), 24–39. https://doi.org/10.1080/23808985.2019.1570472
- Shulman, H. C., Dixon, G. N., Bullock, O. M., & Colón Amill, D. (2020). The effects of jargon on processing fluency, self-perceptions, and scientific engagement. Journal of Language and Social Psychology, 39(5–6), 579–597. https://doi.org/10.1177/0261927X20902177
- Slater, M. D., Peter, J., & Valkenberg, P. (2015). Message variability and heterogeneity: A core challenge for communication research. Annals of the International Communication Association, 39(1), 3–31. https://doi.org/10.1080/23808985.2015.11679170
- song, H., & Ewoldsen, D. R. (2015). Metacognitive model of ambivalence: The role of multiple beliefs and metacognitions in creating attitude ambivalence. Communication Theory, 25(1), 23–45. https://doi.org/10.1111/comt.12050
- Stucki, I., Pleger, L. E., & Sager, F. (2018). The making of the informed voter: A split-ballot survey on the use of scientific evidence in direct-democratic campaigns. Swiss Political Science Review, 24(2), 115–139. https://doi.org/10.1111/spsr.12290
- Suiter, J., & Reidy, T. (2020). Does deliberation help deliver informed electorates: Evidence from Irish referendum votes. Representation, 56(4), 539–557. https://doi.org/10.1080/00344893.2019.1704848
- Sweitzer, M. D., & Shulman, H. C. (2018). The effects of metacognition in survey research: Experimental, cross-sectional, and content-analytic evidence. Public Opinion Quarterly, 82(4), 745–768. https://doi.org/10.1093/poq/nfy034
- Tolochko, P., Song, H., & Boomgaarden, H. (2019). “That looks hard!”: Effects of objective and perceived textual complexity on factual and structural political knowledge. Political Communication, 36(4), 609–628. https://doi.org/10.1080/10584609.2019.1631919