ABSTRACT
Social media companies wield considerable power over what people can say and do online, with consequences for freedom of expression and participation in digital culture. Yet we still know little about the factors that shape these companies’ policy decisions. Drawing on data collected from mainstream English-language news sources between 2005-2021 and on a novel dataset of policy documents from the Platform Governance Archive (PGA), we investigate the extent to which policy change at Facebook, Twitter, and YouTube is responsive to negative news coverage. We find that sustained negative coverage significantly predicts changes to platforms’ user policies, highlighting the role of public pressure in shaping the governance of online platforms.
Introduction
On October 30, 2019, Twitter announced it would ban all political advertising on its platform ahead of the 2020 US presidential elections (Conger, Citation2019). The announcement came just days after Meta’s CEO Mark Zuckerberg had defended his own company’s decision not to remove or fact-check ads placed by politicians on its Facebook platform, following growing concerns over potential voter manipulation during the election. Faced with mounting pressure to curb the spread of misinformation circulating on the site ahead of the vote, however, Meta eventually reversed course later that year. In October 2020, the company issued a statement pledging to stop running all political ads for an indefinite period of time after polls had closed (Paul, Citation2020). It revoked the decision in March 2021.
Social media platforms play an increasingly powerful role in shaping online speech, with little public accountability. Today, companies like Meta and Twitter set the terms for how billions of people communicate and interact worldwide and – as this sequence of events clearly shows – their policy decisions have important consequences for political expression and participation. Even minor tweaks to platforms’ policies and terms of services can dramatically constrain whose voices can be heard, and by whom, and directly influence the quality and type of information their users are exposed to (DeNardis & Hackl, Citation2015; Gillepsie, Citation2018; Klonick, Citation2017; Srnicek, Citation2016). When Meta banned all Australian news content from its site in 2021, it inadvertently took down hundreds of pages belonging to charities and public health services, leaving thousands of people without access to critical public information (Shead, Citation2021). This outcome is especially troubling considering how reliant public actors are on these sites as political communication tools (Kreiss & Mcgregor, Citation2018).
Platforms’ power over our digital lives has often brought comparisons to that of nation states. Mark Zuckerberg himself said he thought Facebook was more “like a government than a traditional company” (Foer, Citation2017). Yet, unlike governments, social media firms are not held to democratic standards of legitimacy and accountability. Platforms regularly update their own rules without consulting or properly notifying users, leaving them without safeguards or meaningful ways to contest these decisions (Barrett & Kreiss, Citation2019; Kreiss & McGregor, Citation2019; Suzor, Citation2018); even less so when their livelihoods are tied up to the platform (Caplan & Gillespie, Citation2020; Duffy & Meisner, Citation2022). And while the last few years have seen growing regulatory efforts to address these pitfalls (such as Germany’s NetzDG law and the EU’s Digital Services Act), in practice these companies still exercise considerable discretion over when and how to enforce their own standards, and often do so in inconsistent ways (Kreiss & McGregor, Citation2019; Suzor, Citation2019; Tobin et al., Citation2017).
Given these far-reaching implications, it is imperative for scholars to better understand the forces that shape platforms’ policy decisions. In this article, we examine one of the factors that might exert influence on these processes: media coverage. Drawing on English-language newspaper data collected between 2005 and 2021 and leveraging a range of natural language processing techniques, we conduct a large-scale content and longitudinal analysis of media coverage of Facebook, Twitter, and YouTube-three of the most widely used social media platforms worldwide. Using a recurrent events’ survival analysis approach, accounting for potential correlation between event times due to heterogeneity, we then model how negative news coverage impacts policy change on these platforms. Our findings show that negative news coverage of these platforms by the US and UK-based outlets has increased significantly since 2018 and is a significant predictor of changes to platform user policies overall. These findings underscore the fact that, as powerful as they may be, platforms do indeed respond and adapt to public and media pressures.
The remainder of the paper is structured as follows. In the next section, we first situate our inquiry within the field of “platform governance,” before drawing on theories of agenda-setting and the strategic management literature to formulate hypotheses about the role media coverage might play in shaping platforms’ policy activities. The third section introduces our data and methodology. In the last section, finally, we present the results from our analyses and discuss their implications for scholars of political communication.
Platform Governance
Platform governance scholars have increasingly studied how platforms set the rules and norms governing online life. Previous research has shown that platforms’ policy-making process is largely concealed from the public. Social media companies deploy a range of strategies and tools to moderate problematic speech (Caplan, Citation2021; Gillepsie, Citation2018; Gillespie, Citation2022), most of which involve a mix of automation and human curation (Gorwa et al., Citation2020) and rely heavily on outsourced labor from moderators (S. T. Roberts, Citation2019). While platforms maintain public-facing policy documents, such as terms of services and community guidelines, that articulate their policy preferences to users and external stakeholders (Popiel, Citation2022), their systems and moderation practices remain mostly opaque and illegitimate to users (Haggart & Keller, Citation2021; Suzor, Citation2018). Barrett and Kreiss (Citation2019) and Katzenbach and Gollatz (Citation2020) have argued that the “transient” and reflexive nature of platforms’ policies further contributes to this lack of transparency, compromising the ability of lawmakers and the public to keep platforms accountable (Suzor, Citation2019). Additionally, critical tech scholars note that platforms’ standards mostly reflect US-centric norms of speech and harm, which they apply to the detriment of marginalized groups (DeCook et al., Citation2022; Hallinan et al., Citation2022; Siapera & Viejo-Otero, Citation2021; York, Citation2022).
Despite this growing scholarly interest in “governance by platforms” (Gorwa, Citation2019), the mechanisms and forces that inform platforms’ policy decisions are still poorly understood. For Klonick (Citation2017), policy development is inextricably tied to these companies’ business interests, and perceptions of their role and responsibility toward users. Because major social media platforms derive most of their profits from advertising revenue, to stay economically viable, they must carefully balance private and public interests; ensuring their sites remain attractive to advertisers whilst meeting users’ changing expectations and norms around speech (Medzini, Citation2021). In practice, platforms therefore solicit input from multiple external stakeholders when designing and formulating policies. Expert interviews with Meta and Google employees have shown that policy development at these companies generally follows a multi-step process that involves gathering feedback on societal trends and engaging with academics and political practitioners (Kettemann & Schulz, Citation2020; Kreiss & McGregor, Citation2019).
Given the black boxed nature of platforms’ internal operations, existing studies provide important insights on some of the organizational dynamics involved in the platforms’ policy development. However, by focusing largely on internal rulemaking, these studies overlook the broader institutional context platforms are embedded in as corporate actors and how this environment may shape their strategic decisions. One aspect that has thus far received little scholarly attention in particular is the role of the media in creating normative pressures on technology companies. As social media has taken central stage in people’s lives, journalists and civil society groups have increasingly taken on a watchdog role, scrutinizing platforms’ practices and exposing failures and corporate oversights, such as the misuse of users’ data by the political consulting firm Cambridge Analytica or the role of Meta’s negligence in triggering genocidal violence against Rohingya Muslims in Myanmar (Napoli, Citation2021). Some scholars have characterized these episodes as public shocks – critical moments that spark public outrage and concern about the negative impacts of platforms, gain widespread media visibility, and challenge their owners to do more to protect users from these harms (Ananny & Gillespie, Citation2016; Caplan, Citation2017, Hofmann et al., Citation2016 & Napoli), summing up to a “techlash” (Hemphill, Citation2019). There is ample, though largely anecdotal, evidence to suggest that these incidents can create pressure on platforms to take corrective action. For example, the #FreeTheNipple campaign on Instagram led Meta to update its nudity rules following public outcry and collective mobilization over the company’s inconsistent enforcement of its policies (West, Citation2017). Similarly, the deplatforming of Donald Trump in early 2021 has been linked to a public outcry over his role in the January 6 insurrection (Alizadeh et al., Citation2022). However, empirical studies on these relationships remain scarce.
The Role of Media Coverage in Corporate Governance
There are good reasons to believe that negative media coverage might impact platforms’ policy activities. One reason for this has to do with the agenda-setting function of the media. The core tenet of agenda-setting theory is that news coverage plays an important role in bringing issues to people’s attention (McCombs, Citation2005; McCombs & Shaw, Citation1972), especially around “focusing events” and crises (Birkland, Citation1998). Research shows that the salience of certain topics and issues in the news influences not only their prominence on the public agenda but also how much attention they receive and how quickly they are addressed by policy-makers (see McCombs (Citation2005) and Wolfe et al. (Citation2013) for reviews). Journalists’ editorial choices can shape the public’s perception and understanding of political events and impact their evaluations of political candidates and government officials (Carroll & McCombs, Citation2003; Iyengar & Kinder, Citation1987; Sheafer, Citation2007). For communication scholars, these issue definitions and framing effects are key to understanding the relationship between media reporting and policy-making (Wolfe et al., Citation2013).
Although research on the media’s influence on policy-making has primarily focused on public policy, insights from strategic management literature suggest that these mechanisms may also extend to platform governance (Carroll & McCombs, Citation2003). One way in which media reporting could impact a company’s policy activities is by bringing issues to their attention that they might have otherwise been unaware of. Corporations tend to privilege the status quo (Hambrick et al., Citation1993) – especially when they are performing well – and only resort to making strategic changes when a problem becomes salient to company leadership (Greve, Citation1998; Lant et al., Citation1992). At the first level of agenda-setting, if journalists frequently and prominently report on a platform’s internal operations and policies, the issues raised by their reporting may therefore be seen as important by company executives, who may be prone to address them. The valence of media coverage matters in this regard. Negative events and information are more likely to become salient than positive or neutral news (Baumeister et al., Citation2001; Rozin & Royzman, Citation2001).
In addition to bringing issues to their attention, media reporting can also affect external stakeholders’ beliefs about a firm, with consequences for its public reputation, legitimacy, and financial performance (see Deephouse (Citation2000) and Graf-Vlachy et al. (Citation2020) for reviews). This is especially relevant for platforms, as their economic viability depends on user engagement with their services. Positive media coverage can positively affect a company’s financial performance by granting its legitimacy among investors (Deephouse, Citation2000; Pollock & Rindova, Citation2003). In contrast, negative news coverage has been shown to harm CEOs’ public image (Wiesenfeld et al., Citation2008), dampen consumer confidence (Liu & Shankar, Citation2015), and incentivize government action against a firm (Tang & Tang, Citation2016), all of which can drive down its market value. To protect their reputation and avoid further sanctions, firms are thus more inclined to take concrete action – from issuing pro-social statements (McDonnell & King, Citation2013) to implementing major strategic changes (Bednar et al., Citation2013) – in response to negative coverage. This is especially true in “crisis contexts,” where media reporting exposes real or perceived wrongdoings by a company (Graf-Vlachy et al., Citation2020). For example, studies find that companies tend to divest from controversial industries, such as nuclear power, following critical coverage about their involvement in those industries (Durand & Vergne, Citation2015; Piazza & Perretti, Citation2015). Researchers have also identified a firm’s financial performance as a moderating factor that may accentuate or dampen the effects of news coverage on these outcomes (Graf-Vlachy et al., Citation2020).
Based on this survey of the literature, we formulate the following hypotheses:
H1:
Negative media coverage is positively associated with platform policy change.
H2:
The relationship between negative media coverage and policy change is moderated by a platform’s financial performance.
H3:
The effect of negative media coverage on policy change is stronger when coverage is critical of a platform’s policy and strategic decisions.
Several factors might also impact our dependent variable. Platforms’ policy activities may be sensitive to peer-pressure, as companies seek to align themselves with emerging industry standards or to stay competitive with rival platforms that have already made similar changes (Caplan & Boyd, Citation2018). They may also be responsive to external pressures from governments and policy-makers trying to shape their content moderation practices (Stockmann, Citation2022). Previous research has also shown that high media visibility in general may impact a firm’s favorability and therefore its subsequent strategic decisions (Carroll & McCombs, Citation2003). We identify these as potential confounding variables.
Materials and Methods
Data
Our goal in this study is twofold: to analyze how news coverage of Facebook, Twitter, and YouTube has evolved over time and to explore how negative news coverage impacts platform policy change. To track media coverage of these platforms over time, we collected news articles from 26 influential English-language outlets published between January 1, 2005 – shortly after Facebook was launched – and January 1, 2021 from the LexisNexis database using the following search terms: (‘facebook’ OR ‘twitter’ OR ‘youtube’) AND (‘user*’ OR ‘rule*’ OR ‘policies’ OR ‘policy’ OR ‘guideline*’ OR ‘standard*’ OR ‘data’ OR ‘action*’). To ensure the quality and relevance of the data and exclude irrelevant examples, we iteratively refined our list of search terms to focus on governance by our three platforms of interest, including these platforms’ strategic actions and policy activities. This process reduces LexisNexis data collection to a manageable size and ensures balanced classification data.Footnote1 News outlets to query were sourced from MIT Media Cloud’s collection of “Top US Mainstream News” and “Top US Sources” (Pew Research Center, Citation2021; H. Roberts et al., Citation2021) and consist of a mix of the US and UK digital and print outlets from across the ideological spectrum. News outlets were included if they were available in the LexisNexis database and their ideological distribution of news outlets was determined by identifying them in Media Bias Check.
We draw our data on changes to platforms’ user policies recorded in the Platform Governance Archive (PGA) (Katzenbach et al., Citation2023). The PGA tracks changes to platforms’ public-facing policy documents through automatic and manual scraping of time-stamped web pages stored on the Internet Archive’s Wayback Machine (WBM) (see Appendix for detailed collection procedure). For this study, we captured changes to Facebook and Twitter’s Terms and Services, Community Guidelines and Privacy Policies and to YouTube’s Terms of Services and Privacy Policies between Q1/2007 and Q1/2021.
Measures
To qualify and quantify how media coverage of Facebook, Twitter, and YouTube has evolved over time, we rely on a combination of qualitative content and targeted sentiment analysis. Our first measurement task was to prune out any irrelevant articles from our news corpus and to classify them by topic. Since our study is mainly concerned with the effects of negative coverage on platforms’ policy activities, it was important for us to be able to distinguish articles that referred to platforms as corporate entities with decision-making power (e.g., “Facebook launches new policy on health misinformation”) from the ones that simply mentioned them as the sites of specific events (e.g., “Donald Trump has joined Twitter”). In our case, only the former cases are relevant. Beyond that, to test our hypotheses we also needed to identify articles that specifically report on a platform’s policy and strategic decisions.
To this end, we developed a qualitative coding scheme aimed at capturing the full range of topics present in our corpus. Following an inductive and iterative approach (Wolfe et al., Citation2013), three members of the team independently identified common themes across a random subsample of 50 news articles. Initial codes were then shared and reviewed by team members for validation before being consolidated into broader categories. This process was repeated three times, culminating in a total of eight topic categories: (1) Business, (2) Policy, (3) Products & Features, (4) Society, (5) Legal, (6) Government & Regulation, (7) Employees, and (8) Miscellaneous ( below provides a detailed description of each topic category, with working examples). According to this coding scheme, all articles assigned to one of the first seven topic categories would be considered “relevant” as they deal with matters related to Facebook, Twitter, or YouTube as corporate entities, whereas all articles labeled as Miscellaneous would be considered “irrelevant.”
Table 1. Codebook for article classification.
In a second step, we trained and tested four binary classifiers to identify and remove irrelevant cases from our dataset. To construct the training set, a team of two coders were extensively trained on the coding scheme and given detailed instructions on how to annotate articles. Specifically, coders were instructed to focus on an article’s headline, subtitle, and lead paragraph when labeling texts, as news articles tend to follow an inverted pyramid structure, with key information about the story (the “who did what,” “when,” “where,” “why,” and “how”) summarized in the first few paragraphs (Norambuena et al., Citation2020). Following two rounds of test coding on a random sample of 250 articles, both coders achieved acceptable intercoder reliability on all coding categories (Cohen’s kappa = 0.75) and proceeded to label another 2,000 articles randomly sampled from our dataset.
Out of the four binary classifiers we tested (Logistic Regression, Random Forest, XGBoost, and BERT), we achieved a maximum accuracy of 0.95 on 20% unseen out-of-sample test data for the XGBoost classifier, using article headlines as our unit of analysis. Using this technique, we discarded 7,820 news articles as “irrelevant.” In a final step, we trained and compared three multi-class Logistic Regression, Random Forests, and XG Boost classifiers to assign each news article to a specific topic category. In this case, the regularized multinomial logistic regression model performed best, achieving the highest accuracy (0.99) on the same unit of analysis. All performance metrics are included in section S2 of the SI Appendix. Given that our interest is in targeted media coverage of social media platforms, we further restrict our sample to articles that explicitly mention Facebook, Twitter, or YouTube in their headlines. In those cases (4.45% of all cases), we considered the first platform mentioned as the article’s main target. After this last step, our final dataset consists of 19,560 articles.
Negative Coverage. Following similar work in this area, we operationalize negative media coverage as the expression of negative value judgments about a firm’s actions and policies (Deephouse, Citation2000). Conceptually, this includes articles that explicitly criticize or condemn a platform’s strategic choices and actions – or lack thereof – on a given issue through its author’s choice of words, for example, or the events or individuals it chooses to report on.
To identify instances of negative coverage, we deploy a targeted sentiment classification model (GRU-STC) pre-trained on a dataset of over 11,000 manually annotated news articles about policy developments in the US, thus displaying high domain similarity (Hamborg & Donnay, Citation2021). Following standard pre-processing steps, preliminary tests were conducted on a random sample of 100 articles from our corpus to assess model performance out-of-the-box. The classifier performed moderately well on the negative class (F 1 = 0.54), with the best results produced when applying the model to the article’s first five sentences (i.e., the headline, subtitle, and lead paragraph). As a follow-up step, we fine-tuned the model on a set of 1,035 domain-specific sentences randomly sampled from our own news corpus. Each sentence was manually labeled by three team members, including the lead author, as expressing either positive, negative, or no value judgment toward the target platform identified through coreference resolution (see Section S2 of the SI Appendix for a more detailed description of this procedure). Any disagreements were thoroughly discussed and resolved by the authors. After fine-tuning, we achieved a significantly higher performance on the negative (F 1 = 0.72) and non-negative classes (F 1 = 0.84), with an overall accuracy of 0.80 (a = 0.80).
Policy Change. We operationalize policy change as any substantial changes to a company’s community guidelines, privacy policies, or terms of services. This excludes cosmetic changes and any other artifacts resulting from the data scraping process, such as the inclusion of ads. Drawing on the Platform Governance Archive database between January 2007 and 2021, we record a total of 252 policy changes to platforms’ policies (N = 126 for Facebook, N = 90 for Twitter and N = 36 for YouTube; cf. rugs in ).Footnote2
Financial Performance. Finally, we measure a firm’s financial performance based on quarterly net income data from Meta, Twitter, and Alphabet, collected between Q1/2011 and Q3/2021. These data were sourced from the companies’ own websites and through external aggregators of financial metrics including Statista and MacroTrends (see Table S6 in the SI Appendix for detailed breakdown per platform).
Analysis
Evolution of Media Coverage
We begin our analysis by examining how media coverageFootnote3 of our three platforms of interest has evolved over time. below shows the volume of articles published about Facebook, Twitter, and YouTube by the news outlets in our sample between 2005 and 2021, broken down by topic category, while shows the proportion of negative coverage received by each platform over that same period. Both figures show that news coverage of these three platforms substantially increased over our observation period. Early coverage focused predominantly on business news, new features, and product releases, and platforms’ operations. A clear spike in business reporting could be observed in 2012, corresponding to the year Facebook went public via IPO. Since 2015, however, journalists have increasingly reported on government actions toward social media companies, as well as platforms’ strategic decisions and policy activities, with the latter category making up the largest part of the media coverage in our dataset overall (see Table S5 in the SI Appendix).
Figure 1. Prevalence of different categories of media coverage.
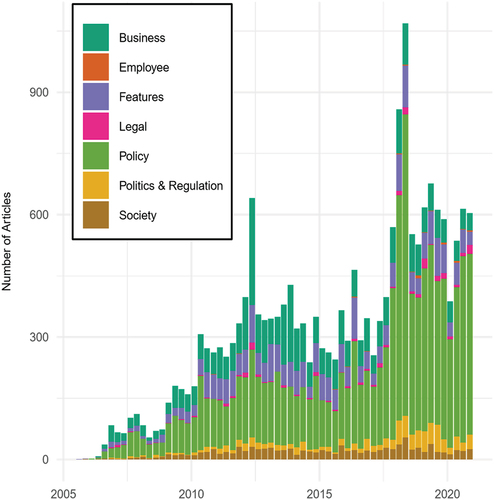
Figure 2. Proportion of negative media coverage received by Facebook, Twitter, and YouTube. Percentage of negative articles published about each platform. Thirty weeks rolling average. The rugs indicate recorded policy changes.
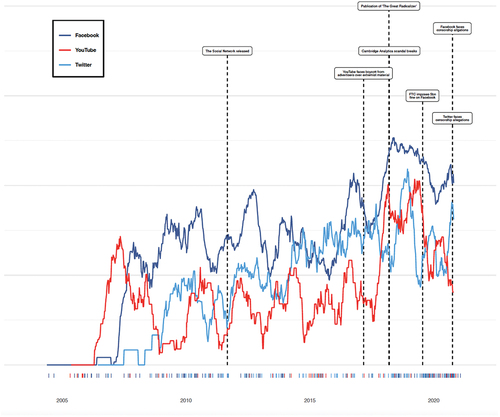
As summarized in , around a third (34%) of the news articles in our dataset were classified as expressing negative judgment toward Facebook, Twitter, and YouTube. Of these, the great majority were targeted at Facebook – far beyond the other two platforms. Interestingly, there was a noticeable increase in negative news coverage post-2018, following disclosure of the illegal harvesting and misuse of Facebook users’ personal data by the consulting firm Cambridge Analytica in The Guardian and The New York Times. Similar spikes in negative coverage broadly map onto other events that brought social media’s influence on politics to the fore, including the revelations of Russia’s interference into the 2016 US Presidential elections and a first wave of large fines against Google and Facebook for violating antitrust laws.
Table 2. Proportion of negative and non-negative coverage.
Impact of Negative Coverage on Policy Change
Next, we assess evidence for the main research question, namely that exposure to negative coverage makes it more likely for platforms to implement policy changes. While previous research has collected anecdotes and case-studies of media and discourse's impact on policy changes, we investigate this nexus systematically here, yet without attention to the specific content of such changes. Drawing inspiration from research in epidemiology (Checkoway & Rice, Citation1992; Richardson et al., Citation2008; Thomas, Citation1988), we argue that the responsiveness of platforms to negative coverage does not depend on a singular exposure, but rather on their exposure history. In other words, while one critical news article about their platforms might not be enough to compel Meta, Twitter, and Alphabet into action on their own, sustained negative coverage is more likely to pressure a company to respond. In this view, once a change has been made, it effectively “releases” the public pressure and resets a platform’s exposure history. We follow common practice in epidemiology (de Vocht et al., Citation2015; White et al., Citation2008) and operationalize cumulative negative coverage as the integral of negative articles published against a platform since their last policy change.
Given the nature of our data and the research question at hand, to estimate the impact of negative coverage on platform policy change, we employ a PWP-Gap model – a conditional recurrent event model (Box-Steffensmeier et al., Citation2007; Prentice et al., Citation1981) that considers the sequential ordering of policy changes, and controls for potential correlation between event times due to heterogeneity. This type of model is fitting when it is reasonable to assume that the effect of covariates on the hazard of policy change is not constant but may vary between events (Amorim and Cai, Citation2015). We model the effect of negative coverage on the hazard of future policy changes in gap time, i.e., from the time since the previous change.
Based on our prior analysis of the literature, we further control for the following covariates: (1) regular (non-negative) coverage of a given platform, (2) total news coverage, and (3) regulatory pressure – measured as the cumulative number of news articles mentioning regulatory action against a specific platform – during the risk interval, and (4) a binary variable indicating whether any policy change was implemented by a competitor platform during the same risk interval. All continuous measures are lagged by 1 week to account for the fact that platforms cannot respond to media coverage instantaneously but need some time to consider and eventually approve new policy changes (Kettemann & Schulz, Citation2020). This choice provides sufficient time for platforms to incorporate critique while preventing the effects of negative press and policy response to be falsely regarded as contemporaneous.
Model 1 in addresses our first hypothesis (H1), namely that negative coverage would be positively associated with policy change. Results from Model 1 show that being the target of negative coverage is indeed associated with a greater risk of introducing policy changes (HR = 1.06, p < .001). Specifically, for every unit that increases in negative coverage since its last policy change, the likelihood that a platform will update its user policies increases by 6%. We report marginal effects by platform in the Supplementary Appendix. H1 is thus confirmed. Interestingly, regulatory pressure (HR = 0.87, p < .001), overall media visibility (HR = 0.98, p < .001), non-negative media coverage (HR = 0.97, p < .001), and recent changes in competitors’ user policies (HR = 0.68, p < .001) all seem to negatively impact the likelihood of new policy activity.
Table 3. Effect of cumulative negative coverage on future policy change.
Moving on to our second hypothesis (H2), we assess whether the relationship between negative coverage and policy change is moderated by financial performance by adding an interaction term to Model 1. Results from this model are reported in Model 2 of . These show that the interaction between these two variables is not statistically significant: we therefore cannot reject the null hypothesis that financial performance moderates the effects of negative coverage on our dependent variable. Finally, our third hypothesis (H3) expected that the impact of negative coverage on policy change to be greater if that coverage specifically focused on a company’s policy and strategic decisions. We test this assumption by restricting the analysis to media reporting that we had previously classified as belonging in the “Policy” category (see Model 3 of ). We find that policy-specific negative coverage is also significantly associated with a greater risk of policy change (H = 1.05, p < .01), but its effect is not stronger than that of negative coverage in general. Comparing the coefficients of both variables in the same model indicates that the difference in the magnitudes of these effects is not significant (see Supplementary Appendix). There is therefore no strong evidence to support H3.
Diagnostic tests show that the proportional hazard assumption is violated for all three models, indicating that the effects of our independent variable are not perfectly constant over time. Plotting the evolution of the estimate from Model 1 over time indeed shows that the positive effect of negative coverage on policy change lasts until the 32-week mark since the last policy change, with the strongest part of the effect concentrated in the first 3 months. After that time, the negative effect becomes even stronger, though our model cannot predict it with the same degree of confidence (see Figure S1 in the Appendix). To test the potential reverse effect of policy changes on negative press coverage, we also run additional analyses modeling negative press coverage as a function of implemented policy changes (see Table 8 in the Appendix). Using lags of 1−4 weeks, the results show that policy changes do not have any significant effect on negative press coverage.
Discussion
This study documented the evolution of media discourse around Facebook, Twitter, and YouTube over a 15-year period and investigated the extent to which policy change at these platforms is responsive to negative news coverage. Several findings stand out from our analyses. First, we find empirical support for the baseline assumption of the “techlash” phenomenon – namely, a spike in negative sentiment and critical new reporting toward major Big Tech companies (Hemphill, Citation2019). Platforms’ outsized power and influence over public life have brought widespread criticisms since 2016, and these are clearly reflected in the increasingly critical tone of tech coverage over that period.
Beyond documenting this phenomenon, we find evidence, consistent with the existing literature (Klonick, Citation2017; West, Citation2017), that platforms are more likely to make changes to their user policies as a result of sustained negative news coverage, but that this effect is not especially more pronounced when that coverage focuses on a platform’s policy decisions. In other words, platforms only seem to be generally reactive to media critiques that challenge their legitimacy and authority or implicate them in being responsible for harming society or not taking sufficient action to protect users.
Against our initial expectations, however, we do not find evidence that this relationship is moderated by a firm’s financial performance. While the reasons for this result can only be speculated, one explanation for this finding might be that positive financial performance for these platforms is itself contingent on being endorsed, and therefore widely used, by the public at large. While platforms may set the rules about who uses their networks and how, as hybrid actors who must reconcile user preferences with public interests, they are inevitably bound by and ultimately reliant upon public acceptance of these rules to operate. Public pressure may therefore work as an independent mechanism that propels platform policy change, irrespective of these companies’ revenues.
Contrary to the belief that platforms have simply become too dominant or too powerful to abide by public demands, our findings also underscore the vital role that the news media plays in shaping the institutional environment in which these companies operate by providing a platform for institutional and cultural narratives that frame how the appropriateness of firms’ actions are perceived and evaluated (Bednar et al., Citation2013). Decades of agenda-setting research have shown that journalists can exert strong normative pressures on public and private actors by amplifying public concerns about their practices and drawing attention to corporate misdeeds. Our results extend our findings to the platform governance literature by demonstrating that social media platforms (as politically powerful as they are) are also receptive to these pressures.
These findings come with several limitations, which should be acknowledged. In this study, we restricted our analysis to newspaper data from influential, mainstream, English-language news sources, as it is reasonable to expect that the most powerful US-based technology companies would pay attention to reporting from these outlets. However, the impact of media reporting on social media firms might vary by platform and socio-political context. It remains to be seen, for example, whether our results extend to geographies where businesses, governments, and special interest groups heavily lean on the media to advance their own interests, and to “secondary markets,” where platform companies might be less inclined to respond to bad press. Likewise, the reputation and clout of a media outlet for its reporting might also impact how negative media coverage impacts platform policies. Future studies could thus usefully extend our findings by testing how these relationships hold across multiple countries and types of news sources.
It is also important to acknowledge that factors other than negative press coverage may contribute to platform policy changes as well. Platform policy-making is a dynamic process, shaped by complex relationships between multiple actors including lawmakers, individual staff members, journalists, academics, and civil society groups. News coverage of social media itself, for example, is not entirely exempt from some degree of influence from platform companies (Napoli, Citation2021, Schiffrin, Citation2021). Other possible explanations for our findings include the fact that platforms may be reacting to novel or unexpected events or other sources of institutional inputs. However, our model controls for these possibilities by including covariates for total news coverage and peer-pressure. Journalists report prominently on novel or unexpected events and co-orientation within an industry is also likely to be highest during such events, as a lack of precedent or sufficient information can lead firms to imitate each other’s policies (see for e.g. Lieberman & Asaba, Citation2006). Yet, we find that peer pressure and overall news coverage have a negative impact on the likelihood of policy change, suggesting that the novelty of an issue is not the driving force behind the observed policy changes. Another potential critique that could be leveled at our analysis is that policy changes implemented by platforms may themselves generate negative press coverage, creating a reverse effect. However, additional robustness checks also indicate that this is not the case. While the scope of our observational study is necessarily limited – as it does not permit us to draw causal conclusions about the role of negative media reporting – we therefore nevertheless view it as an important and robust empirical contribution to the field of platform governance and a descriptive foundation for other communication scholars to build on. Further research and alternative methods, such as process tracing and causal approaches, will be needed to examine how this constellation of actors influences each other, whether they frame similar issues differently, and how the nature of their relationships ultimately informs and shapes platforms’ policy activities.
Supplemental Material
Download MS Word (100 KB)Acknowledgments
The authors thank Yptach Lelkes, Shannon McGregor, Robert Gorwa, Fabrizio Gilardi, and the participants of ICA and APSA 2022 for their thoughtful comments on earlier versions of this manuscript. We also thank Fabio Melliger and Paula Moser for their excellent research assistance.
Disclosure Statement
No potential conflict of interest was reported by the author(s).
Supplementary material
Supplemental data for this article can be accessed on the publisher’s website at https://doi.org/10.1080/10584609.2024.2377992.
Additional information
Funding
Notes on contributors
Nahema Marchal
Nahema Marchal is a posdoctoral research fellow in the Department of Political Science at the University of Zurich. Her research integrates approaches from computational social science, media, communication and political science to explore the implications of digital technology for politics and society.
Emma Hoes
Emma Hoes is a posdoctoral research fellow in the Department of Political Science at the University of Zurich. Her research focuses on the challenges that came about with the advancement of digital technologies, including misinformation, micro-targeting, online content moderation, AI and LLMs, and the role of social media in our daily media-diet.
K. Jonathan Klüser
K. Jonathan Klüser is a postdoctoral researcher at the Chair of Policy Analysis at the University of Zurich. His research focuses on agenda setting and party competition, with a dominant focus on how the involved actors behave on social media.
Felix Hamborg
Felix Hamborg is a senior researcher at the Humboldt University of Berlin, Germany. His research focuses on the automated identification of media bias in news articles and combines approaches from deep learning, natural language processing, information visualization, and political science.
Meysam Alizadeh
Meysam Alizadeh is a senior researcher at the Digital Democracy Lab within the Department of Political Science at the University of Zurich. His research focuses broadly around framing theory, information operations, opinion dynamics, social influence, and intergroup relationships.
Mael Kubli
Mael Kubli is a doctoral researcher in the Department of Political Science at the University of Zurich, working on digital democracy and computational social science. His research focuses on digitalization, its emergence as a political issue and its framing in various contexts.
Christian Katzenbach
Christian Katzenbach is Professor of Media and Communication at the University of Bremen where he heads the “Platform Governance, Media, and Technology” lab and Associated researcher at the Humboldt Institute for Internet and Society (HIIG). His research and teaching addresses the interrelationships of communication, technology and politics in the context of the digitalisation of society.
Notes
1. Please note that the number of articles per outlet varies. This discrepancy can be attributed to differences in publication frequency, varying degrees of coverage of our selected topic, and the completeness of the records of each newspaper’s publications in LexisNexis. We rely on LexisNexis to ensure that all sources are uploading data to their archive.
2. For a detailed overview of all recorded policy changes, please refer to the Platform Governance Archive database (Katzenbach et al., Citation2023).
3. Please note that our definition of “(negative) media coverage” is limited to articles that mention words such as “user,” “guideline,” or “data.” This means that our findings may not reflect all general media coverage about platforms.
References
- Alizadeh, M., Gilardi, F., Hoes, E., Klüser, K. J., Kubli, M., & Marchal, N. (2022). Content moderation as a political issue: The twitter discourse around Trump’s ban. Journal of Quantitative Description: Digital Media, 2, 1–44. https://doi.org/10.51685/jqd.2022.023
- Amorim, L. D., & Cai, J. (2015). Modelling recurrent events: A tutorial for analysis in epidemiology. International Journal of Epidemiology, 44(1), 324–333.
- Ananny, M., & Gillespie, T. (2016). Public platforms: Beyond the cycle of shocks and exceptions. The internet, policy & politics conference. http://blogs.oii.ox.ac.uk/ipp-conference/sites/ipp/files/documents/anannyGillespie-publicPlatforms-oii-submittedSept8.pdf
- Barrett, B., & Kreiss, D. (2019). Platform transience: Changes in Facebook’s policies, procedures, and affordances in global electoral politics. Internet Policy Review, 8(4). 1–22 https://doi.org/10.14763/2019.4.1446
- Baumeister, R. F., Bratslavsky, E., Finkenauer, C., & Vohs, K. D. (2001). Bad is stronger than good. Review of General Psychology, 5(4), 323–370. https://doi.org/10.1037/1089-2680.5.4.323
- Bednar, M. K., Boivie, S., & Prince, N. R. (2013). Burr under the saddle: How media coverage influences strategic change. Organization Science, 24(3), 910–925. https://doi.org/10.1287/orsc.1120.0770
- Birkland, T. A. (1998). Focusing events, mobilization, and agenda setting. Journal of Public Policy, 18(1), 53–74. https://doi.org/10.1017/S0143814X98000038
- Box-Steffensmeier, J., De Boef, S., & Kyle, J. (2007). Event dependence and heterogeneity in duration models: The conditional frailty model. Political Analysis, 15(3), 237–256. https://doi.org/10.1093/pan/mpm013
- Caplan, R. (2021). The artisan and the decision factory: The organizational dynamics of private speech governance. In L. Bernholz, H. Landemore, & R. Reich (Eds.), Digital technology and democratic theory (pp. 167–186). University of Chicago Press.
- Caplan, R., & Boyd, D. (2018). Isomorphism through algorithms: Institutional dependencies in the case of facebook. Big Data & Society, 5(1), 205395171875725. https://doi.org/10.1177/2053951718757253
- Caplan, R., & Gillespie, T. (2020). Tiered governance and demonetization: The shifting terms of labor and compensation in the platform economy. Social Media + Society, 6(2), 205630512093663. https://doi.org/10.1177/2056305120936636
- Carroll, C., & McCombs, M. (2003). Agenda setting effects of business news on the public’s images and opinions about major corporations. Corporate Reputation Review, 6(1), 36–46. https://doi.org/10.1057/palgrave.crr.1540188
- Checkoway, H., & Rice, C. H. (1992). Time-weighted averages, peaks, and other indices of exposure in occupational epidemiology. American Journal of Industrial Medicine, 21(1), 25–33. https://doi.org/10.1002/ajim.4700210106
- Conger, K. (2019, October 30). Twitter will ban all political ads, C.E.O. Jack Dorsey says. The New York Times. https://www.nytimes.com/2019/10/30/technology/twitter-political-ads-ban.html
- DeCook, J. R., Cotter, K., Kanthawala, S., & Foyle, K. (2022). Safe from “harm”: The governance of violence by platforms. Policy & Internet, 14(1), 63–78. https://doi.org/10.1002/poi3.290
- Deephouse, D. L. (2000). Media reputation as a strategic resource: An integration of mass communication and resource-based theories. Journal of Management, 26(6), 1091–1112. https://doi.org/10.1177/014920630002600602
- DeNardis, L., & Hackl, A. M. (2015). Internet governance by social media platforms. Telecommunications Policy, 39(9), 761–770.
- de Vocht, F., Burstyn, I., & Sanguanchaiyakrit, N. (2015). Rethinking cumulative exposure in epidemiology, again. Journal of Exposure Science & Environmental Epidemiology, 25(5), 467–473. https://doi.org/10.1038/jes.2014.58
- Duffy, B. E., & Meisner, C. (2022). Platform governance at the margins: Social media creators’ experiences with algorithmic (in)visibility. Media Culture & Society, 45(2), 285–304. https://doi.org/10.1177/01634437221111923
- Durand, R., & Vergne, J. P. (2015). Asset divestment as a response to media attacks in stigmatized industries. Strategic Management Journal, 36(8), 1205–1223.
- Foer, F. (2017, September 19). Facebook’s war on free will. The guardian. https://www.theguardian.com/technology/2017/sep/19/facebooks-war-on-free-will
- Gillepsie, T. (2018). Custodians of the internet: Platforms, content moderation, and the hidden decisions that shape social media. Yale University Press.
- Gillespie, T. (2022). Do not recommend? Reduction as a form of content moderation. Social Media + Society, 8(3), 20563051221117550. https://doi.org/10.1177/20563051221117552
- Gorwa, R. (2019). What is platform governance? Information Communication & Society, 22(6), 854–871. https://doi.org/10.1080/1369118X.2019.1573914
- Gorwa, R., Binns, R., & Katzenbach, C. (2020). Algorithmic content moderation: Technical and political challenges in the automation of platform governance. Big Data & Society, 7(1), 2053951719897945. https://doi.org/10.1177/2053951719897945
- Graf-Vlachy, L., Oliver, A. G., Banfield, R., König, A., & Bundy, J. (2020). Media coverage of firms: Background, integration, and directions for future research. Journal of Management, 46(1), 36–69. https://doi.org/10.1177/0149206319864155
- Greve, H. R. (1998). Performance, aspirations, and risky organizational change. Administrative Science Quarterly, 43(1), 58–86. https://doi.org/10.2307/2393591
- Haggart, B., & Keller, C. I. (2021). Democratic legitimacy in global platform governance. Telecommunications Policy, 45(6), 102152. https://doi.org/10.1016/j.telpol.2021.102152
- Hallinan, B., Scharlach, R., & Shifman, L. (2022). Beyond neutrality: Conceptualizing platform values. Communication Theory, 32(2), 201–222. https://doi.org/10.1093/ct/qtab008
- Hamborg, F., & Donnay, K. (2021). NewsMTSC: A dataset for (multi-)target-dependent sentiment classification in political news articles. In Proceedings of the 16th Conference of the European Chapter of the Association for Computational Linguistics: Main Volume (pp. 1663–1675). Association for Computational Linguistics (ACL). https://doi.org/10.18653/v1/2021.eacl-main.142
- Hambrick, D. C., Geletkanycz, M. A., & Fredrickson, J. W. (1993). Top executive commitment to the status quo: Some tests of its determinants. Strategic Management Journal, 14(6), 401–418. https://doi.org/10.1002/smj.4250140602
- Hemphill, T. A. (2019). Techlash’, responsible innovation, and the self-regulatory organization. Journal of Responsible Innovation, 6(2), 240–247. https://doi.org/10.1080/23299460.2019.1602817
- Hofmann, J., Katzenbach, C., & Gollatz, K. (2016). Between coordination and regulation: Finding the governance in internet governance. New Media & Society, 19(9), 1406–1423. https://doi.org/10.1177/1461444816639975
- Iyengar, S., & Kinder, D. R. (1987). News that matters: Television and American opinion. University of Chicago Press.
- Katzenbach, C., & Gollatz, K. (2020). Platform governance as reflexive coordination – mediating nudity, hate speech and fake news on Facebook[preprint]. SocArXiv. https://doi.org/10.31235/osf.io/aj34w
- Katzenbach, C., Kopps, A., Magalhaes, J. C., Redeker, D., Sühr, T., & Wunderlich, L. (2023). The platform governance archive v1 – a longitudinal dataset to study the governance of communication and interactions by platforms and the historical evolution of platform policies. Centre for Media, Communication and Information Research (ZeMKI), University of Bremen. https://doi.org/10.26092/elib/2331
- Kettemann, M. C., & Schulz, W. (2020). Setting rules for 2.7 billion: A (first) look into Facebook’s norm-making system; results of a Pilot study. Leibniz-Institut für Medienforschung | Hans-Bredow-Institut (HBI). https://doi.org/10.21241/ssoar.71724
- Klonick, K. (2017). The new governors: The people, rules, and processes governing online speech. Harvard Law Review, 131, 1598–1670. https://heinonline.org/HOL/LandingPage?handle=hein.journals/hlr131&div=73&id=&page=
- Kreiss, D., & Mcgregor, S. C. (2018). Technology firms shape political communication: The work of Microsoft, Facebook, Twitter, and Google with campaigns during the 2016 U.S. presidential cycle. Political Communication, 35(2), 155–177. https://doi.org/10.1080/10584609.2017.1364814
- Kreiss, D., & McGregor, S. C. (2019). The “arbiters of what our voters see”: Facebook and Google’s struggle with policy, process, and enforcement around political advertising. Political Communication, 36(4), 499–522. https://doi.org/10.1080/10584609.2019.1619639
- Lant, T. K., Milliken, F. J., & Batra, B. (1992). The role of managerial learning and interpretation in strategic persistence and reorientation: An empirical exploration. Strategic Management Journal, 13(8), 585–608. https://doi.org/10.1002/smj.4250130803
- Lieberman, M. B., & Asaba, S. (2006). Why do firms imitate each other?Academy of Management Review, 31(2), 366–385.
- Liu, Y., & Shankar, V. (2015). The dynamic impact of product-harm crises on brand preference and advertising effectiveness: An empirical analysis of the automobile industry. Management Science, 61(10), 2514–2535. https://doi.org/10.1287/mnsc.2014.2095
- McCombs, M. (2005). A look at agenda-setting: Past, present and future. Journalism Studies, 6(4), 543–557. https://doi.org/10.1080/14616700500250438
- McCombs, M., & Shaw, D. L. (1972). The agenda-setting function of mass media. Public Opinion Quarterly, 36(2), 176–187. https://doi.org/10.1086/267990
- McDonnell, M.-H., & King, B. (2013). Keeping up appearances: Reputational threat and impression management after social movement boycotts. Administrative Science Quarterly, 58(3), 387–419. https://doi.org/10.1177/0001839213500032
- Medzini, R. (2021). Enhanced self-regulation: The case of Facebook’s content governance. New Media & Society. https://doi.org/10.1177/1461444821989352
- Napoli, P. (2021). The platform beat: Algorithmic watchdogs in the disinformation age. European Journal of Communication, 36(4), 376–390. https://doi.org/10.1177/02673231211028359
- Napoli, P., & Caplan, R. (2017). Why media companies insist they’re not media companies, why they’re wrong, and why it matters. First Monday, 22(5). https://doi.org/10.5210/fm.v22i5.7051
- Norambuena, B. K., Horning, M., & Mitra, T. (2020). Evaluating the inverted pyramid structure through automatic 5W1H extraction and summarization (pp. 1–7). https://faculty.washington.edu/tmitra/public/papers/5W1H-C_J_2020.pdf
- Paul, K. (2020, October 8). Facebook announces plan to stop political ads after 3 November. The guardian. https://www.theguardian.com/technology/2020/oct/07/facebook-stop-political-ads-policy-3-november
- Pew Research Center. (2021, July 27). State of the news media methodology. Pew research Center’s journalism project. https://www.pewresearch.org/journalism/2021/07/27/state-of-the-news-media-methodology/
- Piazza, A., & Perretti, F. (2015). Categorical stigma and firm disengagement: Nuclear power generation in the United States, 1970–2000. Organization Science, 26(3), 724–742.
- Pollock, T. G., & Rindova, V. P. (2003). Media legitimation effects in the market for initial public offerings. Academy of Management Journal, 46(5), 631–642. https://doi.org/10.2307/30040654
- Popiel, P. (2022). Digital platforms as policy actors. In T. Flew & F. R. Martin (Eds.), Digital platform regulation: Global perspectives on internet governance (pp. 131–150). Springer International Publishing.
- Prentice, R. L., Williams, B. J., & Peterson, A. V. (1981). On the regression analysis of multivariate failure time data. Biometrika, 68(2), 373–379. https://doi.org/10.1093/biomet/68.2.373
- Richardson, D. B., Terschüren, C., Pohlabeln, H., Jöckel, K.-H., & Hoffmann, W. (2008). Temporal patterns of association between cigarette smoking and leukemia risk. Cancer Causes & Control: CCC, 19(1), 43–50. https://doi.org/10.1007/s10552-007-9068-7
- Roberts, H., Bhargava, R., Valiukas, L., Jen, D., Malik, M. M., Bishop, C., Ndulue, E., Dave, A., Clark, J., Etling, B., Faris, R., Shah, A., Rubinovitz, J., Hope, A., D’Ignazio, C., Bermejo, F., Benkler, Y., & Zuckerman, E. (2021). Media cloud: Massive open source collection of global news on the open web. Media Cloud: Massive Open Source Collection of Global News on the Open Web [Preprint] arXiv, 15, 1034–1045. https://doi.org/10.48550/arXiv.2104.03702
- Roberts, S. T. (2019). Behind the screen. Yale University Press.
- Rozin, P., & Royzman, E. B. (2001). Negativity bias, negativity dominance, and contagion. Personality and Social Psychology Review, 5(4), 296–320. https://doi.org/10.1207/S15327957PSPR0504_2
- Schiffrin, A. (Ed.). (2021). Media capture: How money, digital platforms, and governments control the news. Columbia University Press.
- Shead, S. (2021, February 18). Facebook blocked charity and state health pages in Australia news ban. CNBC. https://www.cnbc.com/2021/02/18/facebook-blocked-charity-and-state-health-pages-in-australia-news-ban.html
- Sheafer, T. (2007). How to evaluate it: The role of story-evaluative tone in agenda setting and priming. Journal of Communication, 57(1), 21–39. https://doi.org/10.1111/j.0021-9916.2007.00327.x
- Siapera, E., & Viejo-Otero, P. (2021). Governing hate: Facebook and digital racism. Television & New Media, 22(2), 112–130. https://doi.org/10.1177/1527476420982232
- Srnicek, N. (2016). Platform capitalism. Wiley.
- Stockmann, D. (2022). Tech companies and the public interest: The role of the state in governing social media platforms. Information Communication & Society, 26(1), 1–15. https://doi.org/10.1080/1369118X.2022.2032796
- Suzor, N. (2018). Digital constitutionalism: Using the rule of law to evaluate the legitimacy of governance by platforms. Social Media + Society, 4(3), 205630511878781. https://doi.org/10.1177/2056305118787812
- Suzor, N. (2019). Lawless: The secret rules that govern our digital lives. Cambridge University Press.
- Tang, Z., & Tang, J. (2016). Can the media discipline Chinese firms’ pollution behaviors? The mediating effects of the public and government. Journal of Management, 42(6), 1700–1722. https://doi.org/10.1177/0149206313515522
- Thomas, D. (1988). Models for exposure-time-response relationships with applications to cancer epidemiology. Annual Review of Public Health, 9(1), 451–482. https://doi.org/10.1146/annurev.pu.09.050188.002315
- Tobin, A., Varner, M., & Angwin, J. (2017, December 28). Facebook’s uneven enforcement of hate speech rules allows vile posts to stay up. ProPublica. https://www.propublica.org/article/facebook-enforcement-hate-speech-rules-mistakes
- West, S. M. (2017). Raging against the machine: Network gatekeeping and collective action on social media platforms. Media and Communication, 5(3), 28–36. https://doi.org/10.17645/mac.v5i3.989
- White, E., Armstrong, B. K., Saracci, R., White, E., Armstrong, B. K., & Saracci, R. (2008). Principles of exposure measurement in epidemiology: Collecting, evaluating and improving measures of disease risk factors (Second ed.). Oxford University Press.
- Wiesenfeld, B. M., Wurthmann, K. A., & Hambrick, D. C. (2008). The stigmatization and devaluation of elites associated with corporate failures: A process model. Academy of Management Review, 33(1), 231–251. https://doi.org/10.5465/amr.2008.27752771
- Wolfe, M., Jones, B. D., & Baumgartner, F. R. (2013). A failure to communicate: Agenda setting in media and policy studies. Political Communication, 30(2), 175–192. https://doi.org/10.1080/10584609.2012.737419
- York, J. C. (2022). Silicon values: The future of free speech under surveillance capitalism. Verso Books.