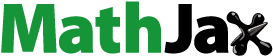
ABSTRACT
Standardized observation systems seek to reliably measure a specific conceptualization of teaching quality, managing rater error through mechanisms such as certification, calibration, validation, and double-scoring. These mechanisms both support high quality scoring and generate the empirical evidence used to support the scoring inference (i.e., that scores represent the intended construct). Past efforts to support this inference assume that rater error can be accurately estimated from a few scoring occasions. We empirically test this assumption using two datasets from the Measures of Effective Teaching project. Results show that rater error is highly complex and difficult to measure precisely from a few scoring occasions. Typically, designed rater monitoring and control mechanisms likely cannot measure rater error precisely enough to show that raters can distinguish between levels of teaching quality within the range typically observed. We discuss the implications for supporting the scoring inference, including recommended changes to rater monitoring and control mechanisms.
Introduction
Directly measuring instruction is important for educational research (Ball & Hill, Citation2009; Steinberg & Donaldson, Citation2015). Observation systems are increasingly prominent tools for such measurement (Praetorius & Charalambous, Citation2018). They are designed to measure a specific, theoretical conceptualization of teaching quality (i.e., the intended construct), which is explicitly operationalized in terms of observable classroom behaviors by the observation rubric. Scoring routines and procedures are included to ensure that observation systems precisely measure their intended construct (Bell et al., Citation2019; Hill et al., Citation2012; Liu et al., Citation2019). The purported key benefits of observation systems come from measuring the specified conceptualization of teaching quality, which allows scores to be comparable across settings (since the same construct is measured regardless of rater or setting), supporting the accumulation of empirical evidence about that conceptualization of teaching quality (Bell et al., Citation2019; Hill & Grossman, Citation2013; Klette, Citation2020). These two features in turn support the many uses of observation systems (e.g., comparing instruction across settings, teacher evaluation, teacher training; Hill et al., Citation2012; T. J. Kane et al., Citation2012).
In contrast to this promise,Footnote1 some have raised critiques about the capacity of observation systems to consistently measure teaching quality across studies and settings (e.g., Liu et al., Citation2019). Rater error may be a threat to the claim that observation scores represent the intended construct. We define rater error as any variation in scores resulting from raters not perfectly applying the rubric (i.e., the gap between the rubric-intended score and the rater-assigned score; OECD, Citation2002). Rater error introduces construct-irrelevant variation that can undermine the interpretation of observation scores as representing the intended construct.
Due to the importance of measuring the intended construct, observation systems have mechanisms designed to manage rater error, including the recruitment, systematic training, and certification of raters, calibration, validation, and double scoring. These rater monitoring and control mechanisms are vital for ensuring the quality of scores, leading researchers to use the term “observation system” to describe empirical efforts to measure teaching quality through observation (Hill et al., Citation2012; Liu et al., Citation2019). These mechanisms, along with the data they generate, serve as the basis for supporting the inference that observation scores reflect the intended construct (e.g., Bell et al., Citation2012; Hill et al., Citation2012).
The goal of this paper is to evaluate how well observation systems support the inference that scores represent the intended construct, which we call the scoring inference. We begin by reviewing previous formal and informal attempts to support the scoring inference, which highlights a high degree of uniformity in how researchers support the scoring inference. Core to the empirical evidence used to support the scoring inference is the assumptionFootnote2 that raters’ overall capacity to score accurately and consistently can be estimated by observing their scoring performance on a few scoring occasions. Stated in more traditional terms, this is the assumption that the construct of average rater error can be reliably measured with a few items (i.e., scoring occasions). We call this the “ease of measuring rater error” assumption.
The centrality of the “ease of measuring rater error” assumption in efforts to support the scoring inference led us to empirically test whether average rater error can be precisely estimated from only a few scoring occasions. Our findings suggest that rater error is highly complex and difficult to measure. Across the two observation systems and two data sets assessed in the main body of this paper, we find that measuring rater error on a few scoring occasions does not result in precise estimates of average levels of rater error. In appendices, we replicate these findings using overall scores from two more observation systems, a second research project, and for every rubric item in each observation system. These findings suggest that the “ease of measuring rater error” assumption is false, undermining the majority of empirical evidence used to support the scoring inference. This does not rule out the possibility that the “ease of measuring rater error” assumption is true in other observation systems, but the replicability of findings suggests that any claims making the “ease of measuring rater error” assumption must be supported by strong empirical evidence of the assumptions reasonableness.
Our findings do not imply that the scoring inference is wrong or unsupportable. They imply instead that the evidence commonly put forward to support the scoring inference offers little support for that inference; the evidence may instead reflect an institutionalized ritual that operates under a logic of confidence (c.f., White & Stovner, Citation2023). This finding is important for those seeking to support the scoring inference because it highlights significant limitations of current standard approaches to empirically support the scoring inference, pointing toward the need to adopt or develop new approaches. The finding also has implications for those seeking to interpret or use observation scores because (1) the costly efforts to adopt and implement observation systems, as opposed to allowing raters to provide idiosyncratic opinions, are generally justified based on the belief that an observation system will measure the intended construct, a belief that requires supporting the scoring inference, and (2) the scoring inference may not be as well supported as one might hope.
Literature review
Validity arguments around observation systems
There are an increasing number of formal, extensive validity arguments being made to support the use of observation systems (e.g., Gleason et al., Citation2017; Johnson et al., Citation2020; Liu et al., Citation2019; Lyon et al., Citation2020; Walkowiak et al., Citation2018; White et al., Citation2022), which are largely based upon two prominent, early exemplar validity arguments (Bell et al., Citation2012; Hill et al., Citation2012). The space required for these formal validity arguments is prohibitive, however. Thus, most uses of observation systems are not supported by formal validity arguments, but by methodological descriptions of study procedures and references to institutionalized practices that are meant to convey the trustworthiness of scores (White & Stovner, Citation2023). We term these “informal validity arguments” because the authors use these descriptions with the goal of convincing readers of the validity of a paper’s conclusions, though the arguments tend to be incomplete, implicit, and/or undeveloped. Importantly, both validity argument types often referenceFootnote3 the same data (e.g., double-scores, training procedures; Bostic et al., Citation2019; Wilhelm et al., Citation2018).
Across these formal and informal validity arguments, documenting the accuracy and consistency of raters’ scoring emerges as a key challenge to support interpretations of observation scores as capturing the intended construct (M. T. Kane, Citation2006). Rater monitoring and control mechanisms are the main process and source of evidence to support the accuracy and consistency of scoring (e.g., Bell et al., Citation2012; Hill et al., Citation2012; Wilhelm et al., Citation2018). In fact, the guidance provided under Standard 6.9 of the Standards for Educational and Psychological Testing (AERA, APA, NCME, 2014) emphasizes the importance of the sort of rater monitoring and control mechanisms that are prominent in observation systems. There is, then, a great deal of agreement that such mechanisms that we discuss below are important for supporting the scoring inference. However, little critical examination of how well these rater monitoring and control mechanisms support the scoring inference and the assumptions inherent in these mechanisms exists, at least for observation systems. We discuss the specific contributions each mechanism makes to supporting the scoring inference below.1
Situating this work relative to the rater effects literature
Given our focus on the threat of rater error for uses of observation systems, it is important to situate this paper relative to the rater effects literature. The rater effects literature studies the impact that raters can have on scores in rater mediated assessments, including cataloging possible rater effects, measuring the size and impact of rater effects, and adjusting observed scores for rater effects (Myford & Wolfe, Citation2003). Often, this involves modeling scores from rater-mediated assessments using item-response theory (IRT) models, as different parameters in IRT models can be understood as capturing specific rater effects (Engelhard & Wind, Citation2018).
Three notes on this literature are relevant. First, most rater effects are defined as average levels of rater error across a number of ratings (Myford & Wolfe, Citation2003). For example, leniency is the average extent to which a rater gives higher scores than other raters. In this paper, we are not interested in measuring average rater error, but in the rater error contained in each score, as accurate and consistent scoring requires low rater error in each assigned score. Second, rater effects studies typically focus on specific types of well-known rater effects (e.g., leniency, restriction of range) rather than studying rater error as a whole. In this paper, we are interested in total rater error, which must be low for accurate and consistent scoring. Third, the rater effects literature has generally focused on modeling scores directly, which necessitates modeling rater effects as differences between raters. To the extent that raters make the same errors, these are invisible in most rater effects studies. This critique led to the creation of the rater accuracy model (Engelhard, Citation1996), which has not been widely used. The rater accuracy model takes the distance between the observed score and a criterion score (i.e., a proxy measure of the true score) as the focus of measurement, which precludes examining differences in how raters use a rating scale, limiting the number of rater effects that can be examined (Engelhard et al., Citation2018). Due to the long apprenticeship of observation (Lortie, Citation2002) that could create shared sources of error across raters, this paper, like the rater accuracy models, focuses on directly modeling rater error rather than comparing differences in ratings across raters.
Other rater effects studies use Generalizability theory (GTheory, Brennan, Citation2001) to explore rater error. GTheory starts with the goal of generalizing scores to a pre-specified universe, examining how features of the testing contextFootnote4 (e.g., the rater scoring the assessment) affect one’s ability to generalize. Specifically, GTheory studies decompose the variation in observed scores to understand how each feature of the testing context (termed facets) affects the observed scores, which enables planning for how to most efficiently obtain scores that can be reliably generalized to represent the construct in the universe of generalizability (Brennan, Citation2001). As such, IRT studies often provide more complete examinations of the rater effects contained within the analyzed data set, while GTheory studies often emphasize planning for future data collection efforts. Since this study focuses on generalizing observed measurements of rater error to the universe of the instruction scored by raters in the study, GTheory approaches are more applicable to our research focus.
Rater monitoring and control mechanisms that feature in scoring inference
This section reviews how each rater monitoring and control mechanism supports the scoring inference. First, though, we provide some definitions. These definitions assume that there is a true score that would be assigned to each sequence of instruction if the rubric were perfectly applied. These true scores reflect teaching quality as seen through the rubric’s lens, which may or may not be consistent with other understandings of teaching quality. True scores are operationalized as the “master scores” assigned by rubric developers. Rater error is the distance between the true score and the rater-assigned score. This is the statistical error in a score (OECD, Citation2002) that is attributable to raters. This is sometimes called rater inaccuracy in the rater effects literature (Myford & Wolfe, Citation2003). Here, we consider rater error from the perspective of construct validity, with rater error introducing construct-irrelevant variation to observation scores that may or may not be correlated with other measures of teaching quality (Hoyt & Kerns, Citation1999). Rater consistency is the extent to which raters agree with each other. Importantly, consistency does not imply accuracy, so rater consistency does not guarantee raters are scoring the intended construct (i.e., accuracy), but a lack of consistency implies rater error.
Second, the structure of rater monitoring and control mechanisms varies widely, and each is not always present in every observation system (Praetorius & Charalambous, Citation2018). Further, the structure of these mechanisms can impact how well they support the scoring inference, so care must be taken to consider how each mechanism is structured and implemented (Liu et al., Citation2019). We next draw upon the limited existing research to discuss how each mechanism supports the scoring inference, discussing how variations in their structure could impact how well they support the scoring inference.
Certification
Rater certification generally occurs at the end of training and involves raters scoring a standardized set of master scored videos (often 4–5; Joe et al., Citation2013). The difference between a rater’s scores and the master scores on these videos is taken as a measure of rater error, which is compared to a certification standard (Joe et al., Citation2013). Raters with lower levels of error than the standard are certified to use the observation rubric. The specific measure of error (e.g., distance from master score; percent non-agreement; Cash et al., Citation2012) and the standard to which raters are held vary across observation systems. Both of these factors could impact how well certification supports the scoring inference (White, Citation2018).
The goal of certification is to provide an estimate of a rater’s ability to accurately use the observation rubric (or alternatively rater error) so that raters exhibiting high levels of error do not score. This often excludes a minority of potential raters from scoring. For example, the MET project had certification passing rates (after two attempts) between 56% and 83% across rubrics (T. J. Kane et al., Citation2012), which is not uncommon (e.g., Cash et al., Citation2012; Hill et al., Citation2012) though some studies have almost perfect certification passing rates (e.g., OECD, Citation2020; Reyes et al., Citation2012). Consequences for failing certification range from none to loss of employment. It is important to acknowledge here that most practice-based settings rely on existing staff and so have limited capacity to exclude uncertified raters (Herlihy et al., Citation2014). Further, some observation systems do not conduct certification (Praetorius & Charalambous, Citation2018).
Certification is used to support the scoring inference in two ways. First, authors describe having conducted certification according to the observation system’s standard procedure as a way of documenting study procedures, implying that this demonstrates that raters can score accurately (e.g., Bell et al., Citation2012; Reyes et al., Citation2012). Second, rater error estimates from certification tests are reported as evidence that raters can score accurately (e.g., Hill et al., Citation2012; Lyon et al., Citation2020; OECD, Citation2020; Wilhelm et al., Citation2018). Both approaches assume that a rater’s scoring performance on a few occasions provides a precise estimate of their overall ability to score. To the extent that rater error is video-specific and/or that rater accuracy estimates from certification are imprecise, rater certification provides less trustworthy evidence to support the scoring inference.
Minimal empirical evidence explores how precisely rater error estimates from certification predict future scoring accuracy, though simulations have questioned the stability of estimates (White, Citation2018). The one study we could find that empirically examined this issue concluded that a sizable minority of participants had unstable certification estimates across consecutive years, but this study examined only rater leniency, a single type of rater error (i.e., a rater effect) rather than overall rater error (Jones & Bergin, Citation2019).
Calibration
Calibration refers to ongoing efforts to ensure raters continue to score accurately after scoring begins.2 This usually takes the form of evaluating raters’ scoring performance on specifically chosen calibration videos, often with group calibration meetings to discuss the video and broader scoring issues. Calibration can improve the quality of observation scores in two ways (Joe et al., Citation2013). First, raters’ scoring performance on calibration videos is evaluated by comparing rater scores to master or consensus scores to estimate rater error (Hill et al., Citation2012). Rater error is then either compared to an explicit performance standardFootnote5 (e.g., MET; T. J. Kane et al., Citation2012) or examined informally (e.g., Casabianca et al., Citation2015; White et al., Citation2022) so that low-quality raters can be removed from scoring and/or provided with additional support in order to preserve score quality across the scoring period (Joe et al., Citation2013). Second, calibration meeting discussions provide opportunities for ongoing rater training and space to discuss other challenges (Joe et al., Citation2013; McClellan et al., Citation2012). Both processes assume that observing a rater’s performance on the calibration video will provide insight into the quality of their scores more broadly, such that scoring problems can be identified and corrected.
Similar to certification, calibration is used to support the scoring inference in two different ways. First, estimates of rater error from the calibration sessions are used as evidence that raters scored accurately (e.g., Bell et al., Citation2012; Bieda et al., Citation2020; Gitomer et al., Citation2014; Hill et al., Citation2012; Lyon et al., Citation2020; OECD, Citation2020). This assumes that raters’ scoring performance on the (often few and non-representative) calibration videos can be used to accurately estimate raters’ scoring performance more broadly. Second, the calibration process is described (often superficially) and stated (or implied) to have served to effectively monitor and control rater error (e.g., T. J. Kane et al., Citation2012; Reyes et al., Citation2012; Tong et al., Citation2019; see also papers reviewed in Liu et al., Citation2019). Both arguments assume that rater performance on the calibration videos can be used to make reliable predictions of raters’ broader scoring performance. The second argument rests on the assumption that simply enacting calibration procedures will support the scoring inference without providing empirical evidence to this effect.
Validation
Validation serves much the same purpose as calibration (OECD, Citation2020). The main difference between calibration and validation is that raters are not told when they are scoring validation videos, whereas they know when they score calibration videos. Additionally, validation does not include group discussions of videos and the associated opportunities to provide ongoing feedback to raters that such discussions provide. Validation videos appear to raters just like any other video, but the videos have master scores, and raters’ scoring performance on these videos is tracked and evaluated, just like in calibration. The goal of validation is the same as calibration and the use of validation to support the scoring inference is likely to be the same. We say “likely to be the same” because validation is far less common than the other mechanisms we discuss. We have only observed it in the MET study (T. J. Kane et al., Citation2012) and the Global Teaching Insights Study (OECD, Citation2020).
Double-scoring videos
The practice of double-scoring measures rater consistency (Myford & Wolfe, Citation2009). Double-scoring occurs when multiple raters score the same observation and is the most common practice for examining rater effects in observation scores (Wilhelm et al., Citation2018). It usually serves the goal of providing an overall, summative measure of the consistency of observation scores across raters. Generally, raters are not aware of which videos are double-scored and double-scoring occurs for a random subset of observations. Then, double-scoring should provide an unbiased (but maybe very noisy) estimate of rater consistency that reflects actual rater performance during scoring (i.e., many challenges connected to generalizing estimates of rater error across types of videos do not apply for double-scoring).
Estimates of rater agreement from double-scoring data are often presented as evidence for the scoring inference (e.g., Bell et al., Citation2012; Liu et al., Citation2019; Lyon et al., Citation2020). The limitation of double-scoring in supporting the scoring inference is that it examines only rater consistency rather than rater accuracy. In many empirical papers, as opposed to formal validity arguments, this limitation remains apparently unnoticed (e.g., Gleason et al., Citation2017; Johnson et al., Citation2020; Wilhelm et al., Citation2018; Wind et al., Citation2019). Given the importance of measuring the intended construct, this is a major limitation. Additionally, since double scoring often occurs for only a (typically small) subset of videos, it may not provide precise estimates of rater consistency, especially as consistency estimates can vary widely across different pairs of raters (Stemler, Citation2004).
Research question
Despite the diversity in rater monitoring and control mechanisms, there is an overarching similarity in how they support the scoring inference. Each measures a rater’s ability to accurately score on a few occasions to estimate raters’ average capacity to score accurately. This is true prospectively for certification, calibration, and validation where current scoring performance is assumed to predict future scoring quality and used to restrict who can score. It is also true retrospectively for calibration, validation, and double-scoring where rater error estimates are used to reflect back on the accuracy of existing scores. Then, underlying the majority of empirical evidence that is brought to bear to support the scoring inference is the “ease of measuring rater error” assumption that average rater error can be precisely measured by observing rater performance across a few scoring occasions.
Given the role of the “ease of measuring rater error” assumption in empirically supporting the scoring inference, research must empirically test the plausibility of this assumption. The paper provides such a test, empirically exploring how precisely average rater error can be measured by observing rater performance across a small number of scoring occasions. Specifically, we ask the following questions: how precise are rater error estimates when observing rater error across a number of scoring occasions? and, given these estimates of precision, how much empirical support do rater monitoring and control mechanisms provide for the scoring inference?
Methods
Data sets
This paper uses two different data sets from the MET study (T. J. Kane et al., Citation2012). Here, we describe the unique characteristics of each data set. shows a summary of the data sets. Note that we also provide an online replication appendix (Appendix B) that presents substantively equivalent findings for the Understanding Teaching Quality (UTQ; Casabianca et al., Citation2015) calibration data. Unfortunately, little data exist to clarify who the raters were in the MET study, except to note that all raters were current or former teachers (White et al., Citation2018).
Table 1. Description of data sets.
MET Calibration Data (METc). The first data set consists of the calibration data from the MET project (T. J. Kane et al., Citation2012). The calibration data come from scoring phases II and III (see White et al., Citation2018). Calibration videos were from Math and ELA grades 4–8. shows counts for videos and raters. As mentioned above, MET raters conducted daily high-stakes calibration, where raters only worked and were paid if they passed calibration. Then, there is some risk with these data that raters use atypical scoring practices. Raters received only a pass/fail indicator and nonspecific feedback from calibration exercises. Further, due to the limited number of calibration videos, MET raters saw the same calibration video multiple times (average time between viewings was 17 days), allowing us to distinguish between errors that raters consistently made when scoring a given video (which we might consider biases) and errors that raters inconsistently made (which we might consider to be random error).
MET Validation Data (METv). The second data set consists of the MET validation data. Raters were not aware of when they were scoring validation videos, preventing concerns that raters adapted their scoring behavior. Further, raters who participated in both phase II and III scoring (see White et al., Citation2018) scored the same validation videos in each phase, allowing us to examine inconsistencies in scoring across scoring occasions. However, the METv data are much smaller than the METc data and contain fewer cases of raters scoring the same video multiple times.
Observation rubrics
This study uses data from two subject-neutral observation rubrics. An online appendix replicates the substantive findings in two additional, subject-specific rubrics (see Online Appendix C). The first rubric is the Classroom Assessment and Scoring System (CLASS; Pianta et al., Citation2007). Data come from the CLASS-secondary (grades 6–8) and the CLASS-upper elementary (grades 4–5) rubrics, which we combined. CLASS consists of 12 dimensions. Videos were divided into 15-min segments, and CLASS scores were independently assigned to each segment. The second rubric is the Framework for Teaching (FFT; Danielson, Citation2000). Only domains 2 and 3 (Classroom Environment and Instruction), the two domains focused on enacted instruction, were coded.3 Calibration videos for FFT were 15 min in length, and validation videos were 30 min in length. All raters went through the training and certification protocols that were standard in 2009 or 2010 for each rubric they scored.
Generalizability theory
We use Generalizability Theory (G Theory) as an analytic framework for this paper (Brennan, Citation2001). G Theory observes that every measurement is affected by specific aspects of where and how that measurement took place, which are termed facets. Then, every score assigned by a rater on a segment of instruction can be modeled as a function of the grand-mean average score and variation attributable to different facets of the scoring context, including the rater, the segment of instruction, the scoring occasion, and other facets. G Theory examines the variation in scores attributable to each facet, providing insights into the measurement process and allowing estimates of score reliability under different measurement designs.
Applied here, we seek to understand the rater error exhibited by an individual rater when scoring an observation, exploring the extent to which videos and scoring occasions contribute to the measurement of rater error. Stably estimating average levels of rater error from a few observations, as rater monitoring and control mechanisms do, requires levels of rater error to be stable across videos and scoring occasions.
We focus on rater error in estimates of average teaching quality (i.e., the average score across rubric dimensions). The goal of these analyses is to explore the precision with which rater error can be estimated. Since estimates of average teaching quality aggregate rater error across rubric dimensions, they contain lower overall levels of rater error (i.e., random rater errors are aggregated out). This should make it easier to precisely estimate rater error in average teaching quality than in dimension- or domain-specific teaching quality (since measurement precision is proportionally related to the reliability of measurement and the standard deviation of rater error, see below). Focusing analyses on rater error in average teaching quality estimates, then, represents (on average) a best-case scenario, where rater error should be estimated most precisely. However, it may be the case that rater error in other estimates of teaching quality (e.g., domain or dimension scores), can be precisely estimated even if rater error in average teaching quality estimates cannot be precisely estimated. We then provide an Online Appendix E that replicates the findings reported here for each rubric dimension. Findings reported here for rater error in average teaching quality replicate across individual rubric dimensions, suggesting that our findings are highly robust to the choice of focusing on rater error in average teaching quality.
In order to operationalize rater error, we use the square of the distance between the rater’s score and the master score (averaging scores across all rubric dimensions). We use a distance measure rather than a dichotomous indicator of agreement based on recommendations from past work (c.f., Cash et al., Citation2012) and focus on the squared distance to more heavily penalize higher levels of rater error since larger errors are more problematic (c.f., Wilhelm et al., Citation2018). We present replications of our figures using the absolute distance in an online appendix (Appendix D), which shows broadly similar patterns.
In our GTheory models, the dependent variable is video-level squared distance from the master score (. We consider the facets of video (
), rater (
), and scoring occasion (o). Note that no teacher has more than one video in our data set and each video consists of one segment. This leads to the following model:
where is the global average squared rater error. Generally,
refers to deviations from this mean error resulting from unique combinations of facets
and
. Thus,
captures differences in error across raters caused by some raters being better scorers than others. This is the average level of error possessed by each rater, after centering.
captures differences in error due to some videos being easier or harder to score than others.
captures the extent to which raters consistently have more or less error than other raters on specific videos; and
captures residual errors. Since
captures all variability in rater error that is consistent across scoring occasions, the residual must capture variability in rater error that is inconsistent across scoring occasions. All error facets,
, are modeled as normal distributions with mean 0 and variance
. We run all analyses in the R statistical language (R Core Team, Citation2018) using the lme4 package (Bates et al., Citation2015). GTheory models, then, are run as linear mixed-effects models (Putka et al., Citation2008).
Our models fundamentally differ from standard GTheory approaches that model observation scores themselves (due to the difference in the dependent variable). Our models should not be compared to other GTheory findings unless those other approaches also model rater error. We are unaware of other studies with such models but note that the rater accuracy model effectively takes the same approach within a Rasch framework (Engelhard, Citation1996).
Decision study
As described above, rater monitoring and control mechanisms make the “ease of measuring rater error” assumption (i.e., rater error is precisely measured from a few scoring occasions). A decision study formally examines this assumption (Brennan, Citation2001). Decision studies allow one to specify measurement designs and determine how reliably these designs measure rater error. For example, a certification test might have raters score five videos. A decision study can determine the reliability with which rater error will be measured in such a test and explore how more precisely rater error might be measured if the test were extended to six or seven videos. Then, a decision study allows us to explore how precisely each of the rater monitoring and control mechanisms might measure rater error. The decision study, however, cannot explore the generalizability of rater error across types of videos, so analyses here represent a sort of “best case” scenario where differences between certification and calibration videos and operational videos have no effect on estimates of rater error.
Specifically, taking as the estimated variance of generic facet f, we estimate the reliability of the measurement of rater error (
) after that rater has watched V videos4 using the following formula:
Here, the variance attributable to the rater facet is the target of measurement, while the variance attributable to the video, videos-by-rater, and residual facet are error terms. We assume that scoring a video is a single scoring occasion and each video is not scored more than once, the more common scenario. In averaging estimates of rater error across V videos, the contribution of each error facets is reduced, providing a more reliable estimate of average rater error.
We focus not on the reliability of rater error estimates, but on their precision (i.e., standard error of measurement), where precision is effectively a way of mapping reliability onto the original score metric to aid in interpreting results. Equation 3 shows a formula for converting reliability to precision (i.e., standard error of measurement).
where is the variance of the observed rater error, using the absolute value of the distance between the rater’s score and the master score as the measure of rater error. Here we use this alternative metric for rater error to ensure that our estimate of the standard error of the measure of rater error is on the same scale as the observation rubric, which allows us to compare the uncertainty in rater error estimates to the variability in observation scores.
Quantifying the size of rater error
Here, we briefly discuss issues related to the interpretation of rater error. Supporting the scoring inference requires showing that levels of rater error are low enough that observation scores can be interpreted as representing the intended construct. We take the position that this, at a minimum, means that raters can reliably judge low-quality instruction as distinct from high-quality instruction within the range of instruction that raters typically encounter. Thus, it is important to interpret levels of rater error from the standpoint of the range of observed teaching quality. We use the standard deviation of the average observed scores across all operational videos as a measure of the spread of teaching quality that raters encountered (SDTQ; see ). Assuming normality, one SDTQ is the difference between the observation score of a video at the 50th percentile and one at the 84th or 16th percentile. We use SDTQ as a standard to help interpret the size of rater error, noting that rater error should be precisely measured to be smaller than one SDTQ (and probably much smaller for some uses of observation scores) to support the scoring inference.
Table 2. Average squared rater error, variance of rater error, and standard deviation of day-level teaching quality.
Two notes on this standard are important. First, SDTQ is calculated using the observation scores assigned by raters. Thus, it contains variance due to rater errors. If we could measure teaching quality without rater error and estimate SDTQ, it would be smaller, potentially much smaller (e.g., MET found ~50% of the variance in observation scores was due to stable differences across classrooms, so SDTQ would be ¼ as large if measured without rater error; T. J. Kane et al., Citation2012). This makes our standard of rater error quite conservative. We are biased toward concluding that rater error is not large and monitoring mechanisms can generate precise enough estimates of rater error. Second, this approach is different than using the range of the observation rubric’s scale to explore rater error. The distribution of observation scores is often narrow compared to the scales used by observation rubrics. For example, in the MET project, the FFT rubric has four score points, but some dimensions had up to 90% of scores assigned to one of two score categories (usually either two or three; T. J. Kane et al., Citation2012).
Interpreting results
As mentioned above, our paper models rater error rather than observation scores, making our G Theory models uncomparable to typical models and requiring some upfront comments on model interpretations. First, the global mean from the G Theory models (i.e., ) captures the average level of rater error in the data set. Knowing nothing about a rater, this is our best initial estimate of the error exhibited by any given rater on any given scoring occasion. The smaller the global average rater error, the less problematic rater error will be for the interpretation of scores. Low average error (i.e.,
) would then directly imply high support for the scoring inference within a given study, but average error says little about the measurement of rater error.
Second, examining EquationEquation (3)(3)
(3) , the precision of the rater error estimate is higher if the variance of observed rater error is lower. That is, when rater error is less variable across raters, videos, and scoring occasions, it is more precisely estimated with data from fewer scoring occasions and videos. This is the basis for our previous claim that focusing on rater error in average teaching quality estimates will create a best-case scenario for precisely measuring rater error (i.e., since rater error gets reduced by aggregating across dimensions).
Third, we should expect to see more variance attributable to the residual facet than in standard GTheory models because the variation captured by master scores is removed from the scores (i.e., ). Residual variance here means that rater error is (so far as we can tell) random, whereas variance attributable to the video or rater facets means that rater error is systematically associated with those facets. Then, residual error is ideal as it will not systematically bias observation scores, while error attributable to the video facet could bias observation scores. That said, the more variance attributable to the rater facet, the more precisely we can measure rater error and the greater the difference in scoring quality across raters, which implies the greater the benefit of identifying specific problematic raters and so the more useful are rater certification, calibration, and validation.
Then, the average level of rater error can be more precisely estimated, supporting the “ease of measuring rater error” assumption, to the extent to which (1) rater error is narrowly dispersed across videos and raters and (2) rater error is attributable in large degree to the rater facet (i.e., it is the result of some raters systematically scoring more accurately and consistently than others).
Results
We start by considering the average levels of rater error and the dispersion of rater error by comparing the observed rater error to SDTQ. We then turn to analyses using the G Theory models and decision studies described above, which more precisely highlight the precision with which rater error is measured. Throughout, we discuss implications and limitations.
Average rater error and variances
shows information on the distribution of rater error, standard rater agreement statistics and SDTQ, our standard for understanding the size of rater error (see discussion above). Note that SDTQ is the same for both data sets since this variable is estimated from all scoring done by raters. As a first step, we examine the size of the average observed rater error. Because our models used the squared error, the global average error we observed (first column of ) is on the wrong scale so we focus on the square root of this error (second column of ). We compare this with SDTQ to understand the relative size of observed rater error in relation to the distribution of observed teaching quality in the MET study. The rightmost column of shows the ratio of the square root of the average squared error and SDTQ, which is where we focus our attention.
Overall, shows that the rater error is quite high in all three data sets. Average levels of rater error are about the same size as one SDTQ or larger across rubrics and data sets. Consider, for instance, the average error to be equal to one SDTQ (the standard deviation of observed teaching quality), which is less than the average error seen in . Since this is the average level of error, the error is approximately half the time larger than this and half the time smaller than this. The implication of this level of rater error is that videos with a true score at the 50th percentile in teaching quality will, on average, have error high enough such that the observed score is at the 16th or 84th percentile. The observed average error levels then suggest that raters are not reliably distinguishing between high- and low-quality teaching within the range of teaching quality observed in MET. This highlights potential problems with the scoring inference, raising questions about the reasonableness of the interpretation that scores represent the intended construct. Then, strong evidence must be marshaled to combat this challenge.
Appendix Table 2.1E and 2.3E shows that this same high level of rater error (in relation to the observed standard deviation of assigned scores) is present at the item level of CLASS and FFT for all items, as well as present for all items in the Understanding Teaching Quality data set, where we replicated analyses and for all items (see Appendix B and E), for the other two MET observation instruments (See Appendix C). Then, regardless of the level of analysis (i.e., item-level or average-teaching quality level), observation rubric, or study, there is a consistent finding that average rater error is high enough to call into question the scoring inference.
Generalizability theory analyses
The GTheory analyses decompose rater error across measurement facets, providing insight into the sources of rater error. summarizes the G Theory results in the form of a variance decomposition showing the percentage of the variance of the squared rater error attributable to each facet (with 95% CI). Across data sets and rubrics, the rater facet accounts for very little of the variance in rater error, implying that observation scores are a noisy measure of how much error a rater makes. Looking at , the rater facet accounts for less than 10% of the variance in observed rater error. Importantly, this does not mean that there are no differences in average rater quality across raters, as rater effects are not small (see ; e.g., T. J. Kane et al., Citation2012). This does, though, mean that the average level of error exhibited by a rater will be very difficult to precisely measure and that most raters exhibit average levels of error similar to the average level of rater error discussed in the last section. This finding also implies that all raters have videos and/or scoring occasions where they exhibit high levels of error, so removing or remediating individual raters, a key pathway for certification/calibration/validation, may not have a large impact on score quality (conditional on the MET study procedures).
Figure 1. Variance decomposition of rater error for MET data sets.
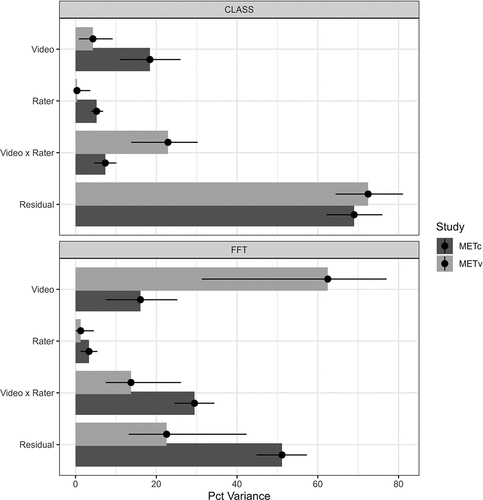
On the other hand, the residual facet is the largest facet across all data sets, accounting for over half of the variance for most rubrics and data sets.5 Our models include a Video-by-Rater facet, which should capture all variability associated with raters making the same errors across scoring occasions. Then, the remaining variability (and so the residual) is associated with raters making inconsistent errors across scoring occasions, which includes the Video-by-Rater-by-Scoring Occasion facet and other more complex errors. The size of the residual facet, then, suggests that raters are not consistently applying observation rubrics (see also Bell et al., Citation2018). That is, the data suggests that the majority of rater error is driven neither by systematic biases against teachers, classrooms, or student characteristics nor a preference for specific instructional approaches, but, so far as we can determine, is closer to random error in the statistical sense. This, of course, does not rule out such systematic biases.
The video facet is unique as it explains errors made consistently by all raters. This has particular implications for efforts to measure rater consistency, since these errors are hidden from consistency measures (Myford & Wolfe, Citation2009). Then, double-scoring will under-estimate levels of rater error and will do so in proportion to the size of the video facet. Additionally, recent efforts have focused on having more raters score each video in order to average rater error across more raters with the goal of reducing the impact of rater error on final scores (e.g., OECD, Citation2020). The error attributable to the video facet is systematic, so this approach of averaging across more raters will not reduce the impact of this source of error. The video facet accounts for between 5% and 60% of the variance (though see footnote 5, which suggests that between 5% and 15% is a more reasonable range). Then, estimates of rater consistency will be 85–95% as large as estimates of rater error, highlighting the important distinction between consistency and accuracy. Importantly, this implies that studies examining only rater consistency will be positively biased when estimating the reliability of scores.
Last, it is important to point out that the video and video-by-rater facets reflect systematic sources of rater error. If raters were biased against specific types of classrooms (e.g., due to sociodemographic features; Campbell & Ronfeldt, Citation2018) or specific types of instructional practice (e.g., due to personal preferences), these biases would manifest in these facets. These facets systematically distort the measurement of the intended construct of teaching quality, and they account for up to 30% of the variance in rater error. This error could systematically inflate or deflate validity coefficients, making observation scores appear to be more or less related to other constructs (Hoyt & Kerns, Citation1999). This systematic bias could undermine validity arguments beyond the scoring inference (e.g., through biasing estimates of concurrent validity used to validate scores), though such effects go beyond the scope of this paper.
In sum, the results just presented highlight the difficulty of estimating average levels of rater error. The small percentage of error attributable to the rater facet means that most raters’ expected error-level will be near the global mean, which was about one SDTQ, a large level of rater error. It also means that expected levels of rater error will be imprecisely measured since expected levels of rater error differ widely across videos and scoring occasions. In the next section, we explore exactly how precisely rater error will be measured across different scoring designs.
Appendix E confirms these results for nearly all dimensions. Namely, (1) the rater facet generally accounts for a very small percentage of the variance of rater error; (2) the residual facet is the largest contributor to the variance of rater error; and (3) the Video and Video-x-Rater facets are modestly large, often larger than the rater facet. The only exceptions are for the Negative Climate dimension of CLASS in the METc dataset, where the video facet dominates due to the lack of any evidence for Negative Climate in the majority of calibration videos (i.e., floor effects); Behavior Management in CLASS for the validation data set, where all facets are approximately equally sized for unknown reasons; and several FFT dimensions in the METv dataset, which had large video facets due to the single strange validation video (see footnote 5). While space constraints prevent more in-depth analyses of dimension-level results, there is a remarkable consistency in findings between dimension-level and average teaching quality-level analyses.
Precision of rater error estimate
This section directly examines the precision with which rater error can be measured from raters’ scores on a small number of videos. The monitoring mechanisms discussed earlier are based, in part, on the premise that scores from a relatively small number of videos can provide good estimates of rater error levels. shows the standard error of the measure of average levels of rater error after scoring different numbers of videos. The dotted line shows the value of SDTQ for comparison. The standard error would have to be about half the size of one SDTQ to ensure that the confidence intervals around rater error estimates do not include one SDTQ (only the upper bound of the confidence interval is meaningful).
Figure 2. Estimates for standard error of rater error estimate across number of video scores.
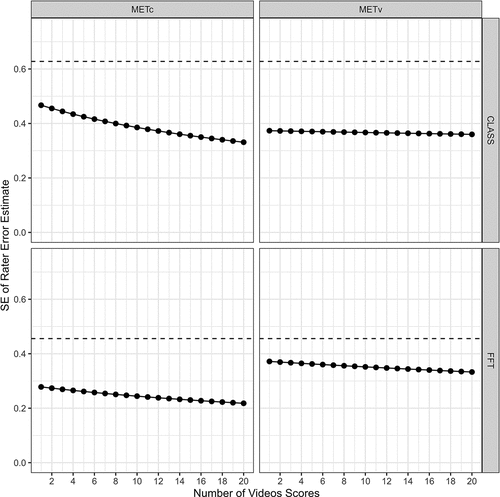
shows that the standard error of an estimate of rater error is roughly two-thirds of SDTQ in CLASS and between one-half and two-thirds of SDTQ for FFT. Certification tests typically average across about five videos; a calibration session often uses a single video; and the total number of calibration and/or validation videos is typically less than 10 (e.g., OECD, Citation2020). As a result, most of the mechanisms just discussed will provide estimates of rater error that are imprecise, making it difficult to determine whether or not a given rater is using the observation rubric accurately and/or consistently. For example, imagine a CLASS certification test consisting of five videos concludes that a given rater has near zero levels of rater error. Given the uncertainties suggested by , the uncertainty in this rater error estimate of ~0 would be about ±0.4 or ±2/3 SDTQ and so the confidence interval around the estimate would be [0 – ~(2*[2/3]) *SDTQ = 1.33*SDTQ]. That is, even a rater with near perfect performance on certification could not confidently (with 95% CI) be claimed to have levels of rater error than are low enough to accurately score teaching quality in the range within which it is typically encountered. Note that this is especially true when we recall that the estimate of SDTQ that we use is conservative, potentially four times larger than it should be (T. J. Kane et al., Citation2012).
also suggests that adding more videos is unlikely to improve the precision of estimates very much, especially for the validation data. This occurs because the rater facets are so small that, even when averaged across a large number of videos, they are still much smaller than the error terms. In this case, the magnitude of the standard error is driven by the standard deviation of the observed rater error (see EquationEquation 3(3)
(3) ), as nearly all of this variability is error.
The dimension-specific results in Appendix E (Tables 3.1E–3.4E) show very few deviations from the main findings. In most cases, rater error is imprecisely measured (relative to SDTQ) and adding additional videos does not improve the precision of measurement much, which is driven by the fact that rater facet is typically a minor source of the variation in observed rater error. In cases where rater error is estimated more precisely (e.g., Behavior Management for CLASS in Table 3.1E in the METv data) or where adding videos does improve precision a fair amount (e.g., Productivity for CLASS in Table 3.1E in the METv data), the same pattern does not repeat across the examined data sets. Still, given a cutoff, such as 0.5 SDTQ, examples of cases where rater error can be precisely measured across a relatively small number of videos can be found. For example, Behavior Management for CLASS in the MET validation data set (see Table 3.1E) requires just four videos to get so precise an estimate of rater error. Again, though, these cases where rater error can be precisely measured are quite rare and do not replicate across data sets or across similar dimensions in different observation rubrics. Thus, the item-level data in Appendix E suggest that there are no dimensions for which we can confidently conclude (and replicate across data sets) that scoring only a handful of videos (i.e., less than 10) would be sufficient to judge that a rater is scoring accurately and consistently.
Differences across the studies, data sets, rubrics, and dimensions are difficult to interpret. The calibration data should provide more precise estimates due to its larger size, but raters know they are being observed. In the validation data, raters do not know if they are being observed. This would certainly create differences in scoring behaviors, especially given the high-stakes nature of MET calibration, but Figure B2 in Appendix B shows that the equivalent graphs from calibration data in UTQ (where stakes are low) look very similar to the MET validation data graphs. Similarly, FFT has a 4-point scale and CLASS has a 7-point scale, which certainly plays a role in the differences across observation systems, but Figure C2 in Appendix C shows that two other observation systems with 4-point scales can look different from FFT. We simply cannot determine from the limited data we have which features of observation systems might be driving the observed patterns. Rather, the use of several data sets and rubrics should be viewed from a replication perspective, showing that the main findings are widely replicable, even though some details of analyses vary across data sets, rubrics, and dimensions.
Discussion
Standardized observation systems are designed to generate observation scores that reflect a specific conceptualization of teaching quality, as operationalized by the rubric (Bell et al., Citation2019; Hill et al., Citation2012; Klette & Blikstad Balas, Citation2018). To achieve this goal, those who design such systems have developed mechanisms to manage rater error, including certification, calibration, validation, and double-scoring. These mechanisms are designed to both improve the quality of scores and/or generate evidence to support the scoring inference (Bell et al., Citation2012; Hill et al., Citation2012; Liu et al., Citation2019; Wilhelm et al., Citation2018). Certification measures performance on a few (non-representative) videos to determine who should be allowed to score. Calibration and validation both attempt to identify if any raters need to be removed from scoring or targeted for remediation, often based on scores from a single video. Double-scoring is meant to estimate average levels of consistency in scoring for post-hoc evaluation of the quality of scores. Within each of these mechanisms is the “ease of measuring rater error” assumption (i.e., a rater’s performance on a few occasions allows for precise measurement of their ability to score accurately). Based on this assumption, rater error estimates from these mechanisms serve as a key source of empirical evidence for the scoring inference (e.g., Bell et al., Citation2012; Hill et al., Citation2012).
The results of this paper suggest that the “ease of measuring rater error” assumption is faulty, undermining the empirical evidence used to support the scoring inference. Rater error is generally high on average and little of the variation in rater error stems from specific raters being, on average, better or worse raters than others. Rather, raters tend to display different levels of error across different videos and scoring occasions. This makes rater error difficult to measure precisely. Our estimates suggest that it often takes many observations (>20) before any estimate of rater error could (with 95% confidence interval) conclude that raters can accurately distinguish between levels of teaching quality within the range that it is typically encountered (i.e., distinguish between two videos 1 SDTQ apart). Due to the time demands of scoring videos, this is more videos than is feasible to observe during certification and more videos than is typical for calibration and validation. Double scoring could potentially generate enough data to provide precise estimates of rater consistency but cannot estimate rater accuracy. Recall that rater consistency may be only 85% as large as rater error. The takeaway, then, is that mechanisms such as certification, calibration, validation, and double-scoring likely will not generate estimates of rater error that are precise enough to empirically support the scoring inference.
These findings represent a serious challenge to interpretations that observation scores accurately represent the intended construct. However, our findings need further confirmation and exploration. Although we provide online appendices that replicate these findings in the UTQ study (Casabianca et al., Citation2015; see Appendix B) using two other MET observation systems (see Appendix C), and for individual dimensions (see Appendix E), further confirmation using additional data sources and other observation systems is needed. Moreover, as we have highlighted, the structure and design of rater monitoring and control mechanisms within observation systems varies widely, which likely impacts the effectiveness of these mechanisms (Liu et al., Citation2019). Despite these limitations, we believe skepticism around the scoring inference is warranted, unless and until strong evidence for the scoring inference that does not rely on the “ease of measuring rater error” assumption is provided.
Current efforts to support the scoring inference rely on the “ease of measuring rater error” assumption because they emphasize estimating standard indices (e.g., Cohen’s kappa) and comparing them to rules of thumb (Wilhelm et al., Citation2018). However, knowing that Cohen’s Kappa is, for example, 0.8 says little about whether or how rater error impacts a specific study conclusion or score use. Future efforts to explore rater error should more clearly connect considerations of rater error to substantive study conclusions or specific uses of observation scores (c.f., White, Citation2023). Simulations could be an important avenue to further explore the relationship between these mechanisms and rater error in observation systems. Simulations could start from some assumed level of rater error, a hypothesized distribution of true scores for teaching quality, and specific structures for rater monitoring mechanisms. Then, simulations could examine how well rater monitoring mechanisms work to identify and remediate problematic raters and examine the influence of rater error on substantive conclusions (e.g., mean differences in scores across groups). By simulating raters with increasing levels of rater error and rater monitoring mechanisms that are increasingly stringent, we can examine how well these mechanisms work to ensure that rater error is not having a disproportionate impact on substantive conclusions that a study might wish to make.
This paper focuses on how observation systems support the scoring inference, not on the entire validity argument. Validity arguments also address the inferences needed to generalize observation scores, to extrapolate scores to represent theoretical constructs, and to examine the implications of uses of observation scores (Bell et al., Citation2012). It is beyond the scope of this paper to detail these other inferences. Then, we cannot make conclusions about the validity of observation scores for any given use of those scores. However, we believe that the support provided for the scoring inference must be carefully considered in any holistic consideration of a validity argument.
The scoring inference can influence other parts of the validity argument. Specifically, weaknesses in the scoring inference can also undermine other aspects of validity arguments in ways that are not easily detectable. For example, the systematic sources of variation in rater error (i.e., the video, rater, and rater-by-video facets) can bias (up or down) estimates of the concurrent and predictive validity of observation scores (Hoyt & Kerns, Citation1999) and adding more raters will not always address this bias (i.e., adding raters does not reduce the impact of the video error facet). Further, the variance in rater error across scoring occasions (which was captured in the residual facet) could serve as a hidden facet (Brennan, Citation2001) that biases estimates of the generalizability of observation scores (i.e., score reliability estimates).
Connecting to the rater effects literature
Here, we briefly connect our results to the rater effects literature. First, our goal was to measure the level of total rater error (or inaccuracy) within a single assigned observation score. Most rater effects, on the other hand, are defined as the average level (across many observations) of a specific type of error made by a rater (e.g., Myford & Wolfe, Citation2003, Citation2009; Wind & Jones, Citation2019; Wind et al., Citation2019). Our goal, then, is much more difficult than the goal in the rater effects literature, just as estimating an expected value for a single individual is more difficult than measuring the mean for a population. The non-zero video-facet highlights the importance of considering models, such as the rater accuracy model (Engelhard, Citation1996), when examining rater error in observation systems, as this error is effectively invisible under most typical rater effects models that look only at consistency across raters.
Further, the Video-by-Rater facet has important implications for Rasch approaches that focus on invariant measurement (Engelhard & Wind, Citation2018), as it implies that raters are not consistently (or invariantly) scoring different videos, violating assumptions of Rasch measurement models, such as the FACETS model (Myford & Wolfe, Citation2003). It is not clear if this is large enough to invalidate the use of such models, as research using those models in other observation data often find acceptable fit (e.g., Wind et al., Citation2019). It could be that there are not enough double-scored data in typical observational data to identify these effects. While simulations have suggested that jointly coding relatively few videos can effectively allow for the estimation of rater effects (e.g., Wind & Jones, Citation2018, Citation2019), these simulations do not appear to have explored the effect of high Video-by-Rater facets or other complex forms of model misfit. More research, then, is needed to explore the appropriateness of invariant measurement models (Engelhard & Wind, Citation2018) with observation scores where the Video-by-Rater facet is large (see also White, Citation2017 for further evidence for a large Video-by-Rater facet and Kelcey, Citation2015 for item-response theory models that relax Rasch assumptions).
Recommendations for rater certification
Our results suggest that rater certification imprecisely measures a rater’s ability to score accurately, at least under typical designs and conditional on extant rubrics and training. This means that rater certification cannot serve as an adequate gatekeeper to restrict entry into scoring, as it is supposed to. In fact, combining our results on the unreliability of measurement with concerns about low standards for raters (White, Citation2018) and multiple opportunities to pass certification leads to the conclusion that certification will have high levels of false-positives – certifying raters who cannot score teaching quality accurately and consistently within the range it is observed, which might explain the high levels of observed rater error in this and past studies (e.g., Bell et al., Citation2014). At the same time, certification failure rates are not always low (e.g., Bell et al., Citation2015; Cash et al., Citation2012), creating a real trade-off between finding raters to complete scoring and only allowing raters who can score accurately and consistently to participate in scoring. The solution to this trade-off needs greater exploration, including studies examining how different rater certification standards might impact levels of achieved rater error. The solution to this challenge may be to make rubrics easier to score and/or improve the quality of rater training where possible.
There are a few potential changes that might help certification tests to more precisely measure rater error and so better serve as gatekeepers to scoring. Based on our estimates, scoring more videos is unlikely to be feasible, given the time demands of scoring (see ). Rather, certification tests could expand what they measure, moving beyond measuring raters’ ability to accurately score. For example, the FFT sometimes includes multiple-choice questions examining raters’ knowledge about the rubric dimensions (Patrick et al., Citation2020). Further, certification tests sometimes test raters’ ability to accurately notice and categorize rubric-relevant behavioral evidence (Bell et al., Citation2016). To the extent that these alternative measures correlate with a rater’s ability to score accurately (or to other relevant rater capacities), their inclusion in certification tests is beneficial (Archer et al., Citation2016; McClellan et al., Citation2012). Unfortunately, initial evidence suggests that these alternative measures can be independent of a rater’s ability to score accurately (Bell et al., Citation2016). In that case, the inclusion of these elements may add construct-irrelevant noise, further reducing the certification tests’ effectiveness as a gatekeeper. Certification testing, then, should work to increase the range of what is tested, but ensure that any new components are empirically associated with a rater’s ability to accurately score in order to improve test precision while keeping tests short.
Recommendations for rater calibration and validation
Our findings suggest changes to rater calibration and validation. Namely, the high-stakes usage of these mechanisms seems unjustified, as poor raters are not reliably identified. However, our results do not suggest eliminating calibration and validation altogether. Both mechanisms could productively surface and address rater misunderstandings, improving the quality of observation scores. Further, calibration meetings are a useful source of continual rater learning. Further research should explore the benefits of these less easily measured aspects of calibration and validation. Such future work should include a focus on how these mechanisms can serve as evidence for the scoring inference, as they do not invoke the faulty “ease of measuring rater error” assumption.
One avenue of research would be to explore the impacts that calibration and validation might have on rater cognitive processes when scoring (e.g., Bell et al., Citation2018). In fact, rather than judging the specific scores assigned by raters, it might be more beneficial to examine thought processes and reasoning behind scores, as even highly experienced raters can misunderstand rubrics (Bell et al., Citation2014). Last, calibration could lead raters to have shared, but incorrect understandings of a rubric, which could lead to the shared video and segment-level variance components we observed. This seems especially likely if calibration videos do not have master scores, but instead rely on consensus scoring. Those leading calibration should strive to adhere closely to rubric scoring rules to avoid this problem, and rubric developers should expand the number of videos with master scores that can be used for calibration.
Recommendations for double-scoring
Our results highlight the need for extensive double-scoring to understand rater consistency. In fact, our analyses likely underestimate the difficulty in reliably measuring rater error using double scoring because we examined raters’ scores relative to the fixed reference of a master score, whereas double-scoring compares the scores of two raters, which introduces additional uncertainty. Further, our analyses suggest that double scoring will under-estimate levels of rater error, which highlights the need to treat double-scoring as just one of multiple approaches to understand and control for rater error.
Limitations
This study has limitations. First, the conclusions are potentially dependent on the design of the observation systems studied. Specifically, the MET study had strong controls over rater recruitment and certification, which could have excluded the worst raters, leading to a small rater error facet. If this were true, we would generally have expected to see much lower levels of overall rater error than we found. Despite our attempts to replicate the findings in Appendix B and C, there remains a need to replicate our empirical findings. Second, much prior research relies on MET data. Researchers must, therefore, acknowledge that much of what we “know” about observation systems could be idiosyncratic features of the MET study, which again highlights the need for replication.
Third, we again note that this study focused on the scoring inference rather than the quality of observation scores more broadly, the overall validity of observation scores for specific uses, or exploring types of rater error. This has important implications for what we can and cannot conclude, limiting our conclusions to those evaluating the quality of the evidence brought to bear to support the scoring inference. There is a need for research to consider the nature of rater error more broadly using models and approaches designed to do this (e.g., Myford & Wolfe, Citation2009; Wind & Jones, Citation2019).
Conclusions
Scores from observation systems are meant to reflect the conceptualization of teaching quality specified by the observation rubric, which leads many interpretations of scores to draw the scoring inference (i.e., raters scored accurately and consistently). Evidence to support the scoring inference comes from rater monitoring and control mechanisms, including certification, calibration, validation, and double-scoring. The empirical evidence for the scoring inference that these mechanisms generate relies on the “ease of measuring rater error” assumption (i.e., that rater error observed on a few occasions can be used to precisely estimate average rater error). The analyses in this paper, which were replicated across two studies, three data sets, four observation rubrics, and most dimensions of each observation rubric, suggest that the “ease of measuring rater error” assumption is faulty. Rather, rater error is highly complex and very difficult to precisely measure. This undermines most evidence that is typically used to support the scoring inference. Then, there may be a lack of strong empirical support for the scoring inference for most observation systems, suggesting that researchers should be careful about interpreting observation scores as representing the intended construct.
Acknowledgments
We would like to thank the editors and anonymous peer reviewers for helpful feedback on the paper.
Disclosure statement
No potential conflict of interest was reported by the author(s).
Additional information
Funding
Notes
1. It is important to acknowledge here that one could, in principle, use observation scores without drawing the scoring inference, though we have not observed this. For example, one could base the validity argument for a teacher observation system entirely on the consequences of the scores (e.g., the “right” teachers are identified for dismissal and overall teaching quality increases). The reader should remember that this paper deals specifically with the scoring inference and how that inference is supported. It does not evaluate validity arguments nor the validity of specific uses of observation scores.
2. Terminology here is not wholly consistent across studies and fields. Oftentimes, calibration is used to refer to the certification testing process (e.g., Cash et al., Citation2012). Other studies use the term calibration for exercises similar to those we describe, but which happen entirely before operational scoring (e.g., Patrick et al., Citation2020).
3. MET scored reduced versions for many of the rubrics. For FFT, MET did not score “Organizing Physical Space” and “Demonstrating Flexibility and Responsiveness.”
4. Our data does not distinguish between segments and videos, so here we must assume that there is 1 video per segment. Often, raters score entire lessons that can be 3 or more segments per video. Thus, we will underestimate the reliability for designs with more than 1 segment per video. The reader can treat V as the number of segments scored instead, which will overestimate reliability to the extent that segments within a video tend to have similar levels of rater error.
5. The low residual error for the FFT rubric in the METv data is driven by the large variance of the video facet. The large video facet, in turn, is driven by a single video (of 11 total) with exceptionally high error for all raters. Removing this one video makes the FFT METv variance decomposition look very much like the FFT METc variance decomposition. While no other single video has such an outsized effect and the results are fairly stable to removing any other video, this goes to show the complexity of this question and potential dependence of all findings on the specific videos examined. Note that the dependence of the results on the specific videos analyzed actually supports our main conclusion that drawing broad conclusions about rater error from a small number of scoring occasions (and so a small number of videos) is a difficult and highly fraught enterprise.
References
- American Educational Research Association [AERA], American Psychological Association [APA], & National Council on Measurement in Education [NCME]. (2014). Standards for Educational and Psychological Testing. American Educational Research Association. http://www.apa.org/science/programs/testing/standards.aspx
- Archer, J., Cantrell, S., Holtzman, S. L., Joe, J. N., Tocci, C. M., & Wood, J. (2016). Better feedback for better teaching: A practical guide to improving classroom observations. Jossey-Bass.
- Ball, D. L., Hill, H. C. & Gitomer, D. (2009). Measuring teacher quality in practice. Measurement Issues and Assessment for Teaching Quality (SAGE Publications), 80–98. 978-1-4129-6144-8.
- Bates, D., Mächler, M., Bolker, B., & Walker, S. (2015). Fitting linear mixed-effects models using lme4. Journal of Statistical Software, 67(1). https://doi.org/10.18637/jss.v067.i01
- Bell, C. A., Dobbelaer, M. J., Klette, K., & Visscher, A. (2019). Qualities of classroom observation systems. School Effectiveness and School Improvement, 0(00), 1–27. https://doi.org/10.1080/09243453.2018.1539014
- Bell, C. A., Gitomer, D. H., McCaffrey, D. F., Hamre, B. K., Pianta, R. C., & Qi, Y. (2012). An argument approach to observation protocol validity. Educational Assessment, 17(2–3), 62–87. https://doi.org/10.1080/10627197.2012.715014
- Bell, C. A., Jones, N., Lewis, J., Qi, Y., Kirui, D., Stickler, L., & Liu, S. (2015). Understanding consequential assessment systems of teaching: Year 2 final report to Los Angeles unified school district. ETS. http://www.ets.org/Media/Research/pdf/RM-15-12.pdf
- Bell, C. A., Jones, N., Lewis, J., Qi, Y., Stickler, L., Liu, S., & McLeod, M. (2016). In Research Memorandum RM-16-12. Princeton, NJ: Educational Testing Service.
- Bell, C. A., Jones, N. D., Qi, Y., & Lewis, J. M. (2018). Strategies for assessing classroom teaching: Examining administrator thinking as validity evidence. Educational Assessment, 23(4), 229–249. https://doi.org/10.1080/10627197.2018.1513788
- Bell, C. A., Qi, Y., Croft, A. J., Leusner, D., McCaffrey, D. F., Gitomer, D. H., & Pianta, R. C. (2014). Improving observational score quality: Challenges in observer thinking. In T. J. Kane, K. A. Kerr, & R. C. Pianta (Eds.), Designing teacher evaluation systems: New guidance from the measures of effective teaching project (pp. 50–97). Jossey-Bass.
- Bieda, K. N., Salloum, S. J., Hu, S., Sweeny, S., Lane, J., & Torphy, K. (2020). Issues with, and insights for, large-scale studies of classroom mathematical instruction. The Journal of Classroom Interaction, 55(1), 41–63.
- Bostic, J., Lesseig, K., Sherman, M., & Boston, M. (2019). Classroom observation and mathematics education research. Journal of Mathematics Teacher Education, 24(1), 5–31. https://doi.org/10.1007/s10857-019-09445-0
- Brennan, R. L. (2001). Generalizability theory. Springer New York. https://doi.org/10.1007/978-1-4757-3456-0.
- Campbell, S. L., & Ronfeldt, M. (2018). Observational evaluation of teachers: Measuring more than we bargained for? American Educational Research Journal, 55(6), 1233–1267. https://doi.org/10.3102/0002831218776216
- Casabianca, J. M., Lockwood, J. R., & McCaffrey, D. F. (2015). Trends in classroom observation scores. Educational and Psychological Measurement, 75(2), 311–337. https://doi.org/10.1177/0013164414539163
- Cash, A. H., Hamre, B. K., Pianta, R. C., & Myers, S. S. (2012). Rater calibration when observational assessment occurs at large scale: Degree of calibration and characteristics of raters associated with calibration. Early Childhood Research Quarterly, 27(3), 529–542. https://doi.org/10.1016/j.ecresq.2011.12.006
- Danielson, C. (2000). Teacher evaluation to enhance professional practice. Association for Supervision & Curriculum Development.
- Engelhard, G. (1996). Evaluating Rater accuracy in performance assessments. Journal of Educational Measurement, 33(1), 56–70. https://doi.org/10.1111/j.1745-3984.1996.tb00479.x
- Engelhard, G., Wang, J., & Wind, S. A. (2018). A tale of two models: Psychometric and cognitive perspectives on rater-mediated assessments using accuracy ratings. Psychological Test and Assessment Modeling, 60(1), 33–52.
- Engelhard, G., & Wind, S. A. (2018). Invariant measurement with raters and rating scales: Rasch models for rater-mediated assessments (First ed.). Routledge.
- Gitomer, D. H., Bell, C. A., Qi, Y., McCaffrey, D. F., Hamre, B. K., & Pianta, R. C. (2014). The instructional challenge in improving teaching quality: Lessons from a classroom observation protocol. Teachers College Record, 116(6), 1–32. https://doi.org/10.1177/016146811411600607
- Gleason, J., Livers, S., & Zelkowski, J. (2017). Mathematics classroom observation protocol for practices (MCOP2): A validation study. Investigations in Mathematics Learning, 9(3), 111–129. https://doi.org/10.1080/19477503.2017.1308697
- Herlihy, C., Karger, E., Pollard, C., Hill, H. C., Kraft, M. A., Williams, M., & Howard, S. (2014). State and local efforts to investigate the validity and reliability of scores from teacher evaluation systems. Teachers College Record, 116(1), 1–28. https://doi.org/10.1177/016146811411600108
- Hill, H. C., Charalambous, C. Y., Blazar, D., McGinn, D., Kraft, M. A., Beisiegel, M., Humez, A., Litke, E., & Lynch, K. (2012). Validating arguments for observational instruments: Attending to multiple sources of variation. Educational Assessment, 17(2–3), 88–106. https://doi.org/10.1080/10627197.2012.715019
- Hill, H. C., & Grossman, P. (2013). Learning from teacher observations: Challenges and opportunities posed by new teacher evaluation systems. Harvard Educational Review, 83(2), 371–384,401. https://doi.org/10.17763/haer.83.2.d11511403715u376
- Hoyt, W. T., & Kerns, M.-D. (1999). Magnitude and moderators of bias in observer ratings: A meta-analysis. Psychological Methods, 4(4), 403–424. https://doi.org/10.1037/1082-989X.4.4.403
- Joe, J., Kosa, J., Tierney, J., & Tocci, C. (2013). Observer calibration. Teachscape.
- Johnson, E. S., Zheng, Y., Crawford, A. R., & Moylan, L. A. (2020). Evaluating an explicit instruction teacher observation protocol through a validity argument approach. The Journal of Experimental Education, 90(2), 1–16. https://doi.org/10.1080/00220973.2020.1811194
- Jones, E., & Bergin, C. (2019). Evaluating teacher effectiveness using classroom observations: A rasch analysis of the rater effects of principals. Educational Assessment, 24(2), 91–118. https://doi.org/10.1080/10627197.2018.1564272
- Kane, M. T. (2006). Validation. In R. L. Brennan (Ed.), Educational measurement (4th ed. pp. 17–64). Praeger Publishers.
- Kane, T. J., Staiger, D. O., McCaffrey, D., Cantrell, S., Archer, J., Buhayar, S., & Parker, D. (2012). Gathering feedback for teaching: Combining high-quality observations with student surveys and achievement gains. Bill & Melinda Gates Foundation, Measures of Effective Teaching Project. http://eric.ed.gov/?id=ED540960
- Kelcey, B. (2015). Measuring teachers’ instruction with multilevel item response theory. International Journal of Research in Educational Methodology, 7(1), 1037–1047. https://doi.org/10.24297/ijrem.v7i1.3857
- Klette, K. (2020). Towards programmatic research when studying classroom teaching and learning. In Ligozat, I. L., Rakhkochkine, A., & J. Almqvist (Eds.), Thinking through didactics in a changing World. European perspectives on learning, teaching and the curriculum (pp. 137–158). Cham: Springer International Publishing.
- Klette, K., & Blikstad Balas, M. (2018). Observation manuals as lenses to classroom teaching: Pitfalls and possibilities. European Educational Research Journal, 17(1), 129–146. https://doi.org/10.1177/1474904117703228
- Learning Mathematics for Teaching Project. (2011). Measuring the mathematical quality of instruction. Journal of Mathematics Teacher Education, 14(1), 25–47. https://doi.org/10.1007/s10857-010-9140-1
- Liu, S., Bell, C. A., Jones, N. D., & McCaffrey, D. F. (2019). Classroom observation systems in context: A case for the validation of observation systems. Educational Assessment, Evaluation and Accountability, 31(1), 61–95. https://doi.org/10.1007/s11092-018-09291-3
- Lortie, D. C. (2002). Schoolteacher: A sociological study (S. E. W. A new Preface, Ed.). University of Chicago Press. https://press.uchicago.edu/ucp/books/book/chicago/S/bo3645184.html
- Lyon, C. J., Oláh, L. N., & Brenneman, M. (2020). A formative assessment observation protocol to measure implementation: Evaluating the scoring inference. Educational Assessment, 25(4), 288–313. https://doi.org/10.1080/10627197.2020.1766957
- McClellan, C., Atkinson, M., & Danielson, C. (2012). Teacher evaluator training & certification: Lessons learned from the measures of effective teaching project. Teachscape.
- Myford, C. M., & Wolfe, E. W. (2003). Detecting and measuring rater effects using many-facet Rasch measurement: Part I. Journal of Applied Measurement, 4(4), 386–422.
- Myford, C. M., & Wolfe, E. W. (2009). Monitoring rater performance over time: A framework for detecting differential accuracy and differential scale category use. Journal of Educational Measurement, 46(4), 371–389. https://doi.org/10.1111/j.1745-3984.2009.00088.x
- OECD. (2002). OECD glossary of statistical terms—Statistical error definition. https://stats.oecd.org/glossary/detail.asp?ID=5058
- OECD. (2020). Global teaching InSights: A video study of teaching. OECD Publishing. https://doi.org/10.1787/20d6f36b-en
- Patrick, H., French, B. F., & Mantzicopoulos, P. (2020). The reliability of framework for teaching scores in kindergarten. Journal of Psychoeducational Assessment, 38(7), 831–845. https://doi.org/10.1177/0734282920910843
- Pianta, R. C., Hamre, B. K., Haynes, N. J., Mintz, S. L., & La Paro, K. M. (2007). CLASS-Secondary manual. Brookes Publishing.
- Praetorius, A.-K., & Charalambous, C. Y. (2018). Classroom observation frameworks for studying instructional quality: Looking back and looking forward. International Journal on Mathematics Education, 50(3), 535–553. https://doi.org/10.1007/s11858-018-0946-0
- Putka, D. J., Le, H., McCloy, R. A., & Diaz, T. (2008). Ill-structured measurement designs in organizational research: Implications for estimating interrater reliability. Journal of Applied Psychology, 93(5), 959–981. https://doi.org/10.1037/0021-9010.93.5.959
- R Core Team. (2018). R: A language and environment for statistical computing. R Foundation for Statistical Computing. https://www.R-project.org/
- Reyes, M. R., Brackett, M. A., Rivers, S. E., White, M., & Salovey, P. (2012). Classroom emotional climate, student engagement, and academic achievement. Journal of Educational Psychology, 104(3), 1–13. https://doi.org/10.1037/a0027268
- Steinberg, M. P., & Donaldson, M. L. (2015). The new educational accountability: Understanding the landscape of teacher evaluation in the Post-NCLB Era. Education Finance and Policy, 1–40. https://doi.org/10.1162/EDFP_a_00186
- Stemler, S. E. (2004). A comparison of consensus, consistency, and measurement approaches to estimating interrater reliability. Practical Assessment, Research & Evaluation, 9(4). https://doi.org/10.7275/96jp-xz07
- Tong, F., Tang, S., Irby, B. J., Lara-Alecio, R., Guerrero, C., & Lopez, T. (2019). A process for establishing and maintaining inter-rater reliability for two observation instruments as a fidelity of implementation measure: A large-scale randomized controlled trial perspective. Studies in Educational Evaluation, 62, 18–29. https://doi.org/10.1016/j.stueduc.2019.04.008
- Walkowiak, T. A., Berry, R. Q., Pinter, H. H., & Jacobson, E. D. (2018). Utilizing the M-Scan to measure standards-based mathematics teaching practices: Affordances and limitations. International Journal on Mathematics Education, 50(3), 461–474. https://doi.org/10.1007/s11858-018-0931-7
- White, M. (2017). Generalizability of scores from classroom observation instruments. Unpublished Doctoral Dissertation. University of Michigan
- White, M. (2018). Rater performance standards for classroom observation instruments. Educational Researcher, 47(8), 492–501. 0013189X18785623. https://doi.org/10.3102/0013189X18785623
- White, M. (2023). August 29. Rater error as a threat to evaluation conclusions: How well do standard methods address this threat? https://doi.org/10.31219/osf.io/r7sza
- White, M., Maher, B., & Rowan, B. (2022). A framework for addressing instrumentation biases when using observation systems as outcome measures in instructional interventions. Journal of Research on Educational Effectiveness, 16(1), 1–27. https://doi.org/10.1080/19345747.2022.2081275
- White, M., Rowan, B., Alter, G., & Greene, C. (2018). User guide to the measures of effective teaching longitudinal database (MET LDB). Inter-University Consortium for Political and Social Research. The University of Michigan.
- White, M., & Stovner, R. B. (2023). Breakdowns in scientific practices: How and why practices can lead to less than rational conclusions (and proposed solutions). https://doi.org/10.31219/osf.io/w7e8q
- Wilhelm, A. G., Rouse, A. G., & Jones, F. (2018). Exploring differences in measurement and reporting of classroom observation inter-rater reliability. Practical Assessment, Research & Evaluation, 23(4), 16.
- Wind, S. A., & Jones, E. (2018). The stabilizing influences of linking set size and model–data fit in sparse rater-mediated assessment networks. Educational and Psychological Measurement, 78(4), 679–707. https://doi.org/10.1177/0013164417703733
- Wind, S. A., & Jones, E. (2019). The effects of incomplete rating designs in combination with rater effects. Journal of Educational Measurement, 56(1), 76–100. https://doi.org/10.1111/jedm.12201
- Wind, S. A., Jones, E., Bergin, C., & Jensen, K. (2019). Exploring patterns of principal judgments in teacher evaluation related to reported gender and years of experience. Studies in Educational Evaluation, 61, 150–158. https://doi.org/10.1016/j.stueduc.2019.03.011