ABSTRACT
The BRPF1 protein is encoded by the BRPF1 gene. In addition, the BRPF1 gene is known to be upregulated in leukaemia. Recent studies have shown that it is also overexpressed in hepatocellular carcinoma (HCC) as well. Therefore, BRPF1 is a significant target for anti-cancer drug development studies, especially on HCC. 40 terpenoids and flavonoids were chosen because of their anticancer properties given in the literature. In this study, the biological activity of molecules was also investigated with in silico structure-activity relationship analysis. In addition, interactions between a series of terpenoids and flavonoids and the BRPF1 protein were investigated by molecular docking and molecular dynamics simulations. The energy change caused by the interactions of BRPF1 with different compounds was also evaluated by MM/PBSA calculations. It has been revealed that compound 5 (−9.2 kcal/mol), a kind of secoclerodane type diterpenoid, has a higher affinity both compared to other flavonoids and terpenoids, and 9F9 (−7.9 kcal/mol), a selective BRPF1 inhibitor. The study presented in this article demonstrates that compound 5, as a natural product, could form a chemical scaffold for the development of selective BRPF1 bromodomain inhibitors.
Introduction
Cancer, which occurs due to poor living conditions and genetic factors, increases every day and remains among the leading causes of death, despite the new anticancer therapies developed. Among the many types of cancers, the most common type of cancer in children is leukaemia (28%) [Citation1]. There are many mutations determined to cause this disease. Bromodomain and plant homeodomain finger containing 1 (BRPF1) protein has been experimentally proven to be effective in leukaemia in recent studies [Citation2].
Bromodomains are protein interaction sites that provide acetylation to be read. The plant homeodomains (PHD fingers) also recognize and bind acetylation sites [Citation3,Citation4]. BRPF1 provides increased acetylation activity because it contains both regions (Bromodomain and PHD finger). Therefore, a significant phenotypic response has been obtained in leukaemia cells with BRPF1 inhibition [Citation5]. In addition, it has been found that the BRPF1 gene, which encodes the BRPF1 protein, is up-regulated not only in leukaemia but also in hepatocellular carcinoma, and it has even been shown that this upregulation may be associated with the high mortality rate of liver cancer patients [Citation6].
It has been clinically proven that the use of high doses of a single chemotherapy agent in cancer treatment can cause many side effects. For this reason, a treatment protocol in the form of a combination of different chemotherapeutics at low doses is generally applied today. The use of natural compounds with chemotherapy agents has been investigated, and many compounds have been reported to increase the chemotherapeutic effect [Citation7–9], help to overcome cancer multidrug resistance [Citation10] and protect cells against the side effects of chemotherapeutics [Citation11–13].
Terpenoids are composed of isoprene units and are natural products of the mevalonate pathway [Citation14]. Bicyclic diterpenoids of the clerodane type are called seco-diterpenoids if they have opened rings [Citation15]. Flavonoids, on the other hand, are a very large family of secondary metabolites containing hydroxylated forms of polyphenol structures [Citation16]. Although there are many in silico studies about drug-receptor interactions with bromodomains in the literature [Citation17–21], there are few studies on the interactions between flavonoids, terpenoids, and bromodomain proteins [Citation22–25].
In this study, the ability of a group of natural compounds to inhibit BRPF1 protein was investigated computationally, and it was aimed to determine natural products that could be drug precursors for leukaemia and HCC. For this purpose, living cell conditions were mimicked biophysically, and molecular docking and molecular dynamics (MD) simulations were supported by energetic calculations.
Materials and methods
In silico biological activity prediction
PASS (Prediction of Activity Spectra for Substances) online web tool [Citation26] was used to determine in silico the biological activity properties of molecules downloaded from PubChem [Citation27] in ‘sdf’ format and recorded in ‘.mol’ format with the DSV program [Citation28]. PASS online web tool makes a prediction based on the analysis of structure-activity relationships (SAR) of molecules. In this way, the drug-like nature of ligands is examined with the PASS online database. Pa denotes the probability of molecules being a member of the active compound’s family for that properties (antimetastatic, antineoplastic and anticarcinogenic), while Pi denotes the probability of being a member of the inactive compound’s family. The web tool suggests filtering methods such as Pa >0.7, Pa > Pi, and Pa > 0.3 after estimation. If the compound with the highest Pa values is selected in the estimation, the possibility of the chosen compound matching with a known pharmacological agent arises. Suppose the Pa value is chosen between 0.5 and 0.7. In that case, we have a lower chance of accurately determining the experimental activity, but our probability of detecting a molecule analogous to a known drug molecule also decreases. If the Pa value is chosen below 0.5, the chance of detecting experimental activity will be considerably reduced. In this study, since natural flavonoids and terpenoids that are not clinically active substances or analogues were selected, compounds with high Pa values were chosen as the target, and Pa > 0.8 values were emphasized.
Molecular docking
Rigid docking was performed employing the AutoDock Vina program [Citation29]. Autodock Vina optimization algorithm is an algorithm that includes local optimization procedures, and stochastic global optimization approaches such as genetic algorithms, particle swarm optimization, and simulated annealing. Using this algorithm, the global minimum of the receptor-ligand complex and the low-score conformation of the ligands are found [Citation29,Citation30].
Receptor preparation
A comprehensive screening was made in the RCSB protein databank [Citation31] for the BRPF1 protein, and 75 structures were found. These structures were especially examined according to their resolution and apo/halo conformation properties and 3 different X-ray crystallographic 3D structures (PDB ID: 4QYL [Citation32], 4UYE [Citation5], 5O55 [Citation2]) were selected considering the modelling studies carried out with BRPF1 in the literature. Since it is more accurate to work on active conformation in inhibitor drug development studies, 3 halo structures have been emphasized. The properties of all BRPF1 structures in the RCSB protein databank, as well as the 3 selected 3D structures, are given in Table S1.
First, the structures have been simplified to include a single chain. All water and heteroatoms were then removed except the binding package. These two steps were carried out through the DSV program. Finally, structures recorded in PDB format in DSV were opened with the ADT program [Citation33], hydrogens and Gasteiger partial charges were added, and they were recorded in PDBQT format with ADT’s Grid/Macromolecules module.
Ligand preparation
19 terpenoids and 21 flavonoids (Table S2) extracted from plants growing in Turkey, New Zealand, Taiwan, Southeast Asia, and China were identified from the literature according to experimental data for molecular docking [Citation34–40]. Molecules were downloaded from the PubChem database, and those that could not be found in PubChem were drawn with ChemSketch [Citation41] and their hydrogens were added to the DSV program. All ligands were recorded in PDB format with the DSV program. Finally, 40 ligands were prepared by ADT’s ‘Ligand’ module automatically, Gasteiger charges were added, non-polar hydrogens were merged, the number of rotatable bonds was determined, and TORSDOF values were adjusted accordingly, and ligands were recorded in PDBQT format.
Docking protocol
A grid box was created via the ‘Grid/Grid Box’ module of the ADT to determine the area to be scanned for docking. This box should contain the binding package of protein. In our study, box properties were determined by taking the ligands crystallized together with the protein in a 3D structure to the centre ().
Table 1. Grid box features for molecular docking.
Molecular dynamics simulations
Energy values and binding profiles of molecular docking were examined. In addition, molecules with higher docking scores than the reference molecule (9F9) were also considered in the ligand selection. For this reason, MD simulations were applied to 10 terpenoids, 9 flavonoids, and reference molecule (9F9) ().
Table 2. Selected 19 compounds for MD simulations and reference molecule.
For MD simulations, the 3D structure of BRPF1 with PDB ID: 4UYE code was chosen. AMBER v20 [Citation42] molecular dynamics package was used for MD simulations. The ANTECHAMBER module in the AMBER v20 package was used to create the topology files required for molecules. In this way, atomic equivalence, and missing force fields were examined, relevant regulations were completed, and the AM1-BCC charge model was used to calculate atomic point charges. The AM1-BCC model is a model that produces high-quality atomic charges that mimic the HF/6-31 G* electrostatic potential for organic molecules. Parameterization using the GAFF and AMBER14SB force fields [Citation43], ionization, and solvation of the protein-ligand complexes were performed with the LEAP module of AMBER v.20. Two sodium ions (Na+) were added to all complexes to neutralize the system. Around the complex, 10 Å TIP3P water buffer was placed in all directions and the protein-ligand system was covered with explicit solvent in a rectangular box.
Minimization and MD protocol
SANDER modules of AMBER v.20 were used for the minimization phase. Minimization was carried out in two steps, in the first step, the positions of TIP3P molecules and ions were minimized by keeping the protein-ligand complex constant. 500 steps of steepest descent minimization were applied, followed by 500 steps of conjugate gradient minimization. In the second step, 25,000 steps of minimization were implemented to the whole system without any restraints.
PMEMD. CUDA module of AMBER v.20 was used for the heating and MD equilibration stages. In the heating stage, the system was heated to 300 K by applying weak restraint to the protein-ligand complex in a constant volume periodic boundary. In this process, the temperature was controlled by using Langevin dynamics at a collision frequency of 1.0 ps−1. Completed at 50 ps using 50,000 molecular dynamics steps with a time step of 2 fs per step. In the MD equilibration stage, the SHAKE algorithm was used, which inhibits the effect of hydrogen on large-scale dynamics by restricting all hydrogen-containing bonds. In the simulation, isotropic position scaling with a relaxation time of 2 ps and constant pressure periodic boundary conditions were employed to keep the system pressure at 1 atm. This calculation was carried out for 150 ns with 150,000,000 steps of 1 fs for each system and results were recorded every 10 ps.
Post-trajectory analysis
MD simulation results were saved in trajectory files and using these files throughout the whole simulation, RMSD, Hydrogen bond analyses were performed with the CPTRAJ [Citation44] module of AMBER v.20.
Binding free energy calculations were performed by MM/PBSA, MM/GBSA modules [Citation45] of AMBER v.20. While the salt concentration was determined as 0.150 M in the calculations, the ionic strength, the fill ratio, and the interior dielectric constant values were determined as 0.15, 4.0 and 1.0, respectively. The energy components of 10 ns trajectories between 140–150 ns for 19 different complexes were investigated. The equations that form the basis of MM/PB(GB)SA calculations were given in our previous study [Citation46].
Results and discussion
In silico biological activity prediction
After the literature review, the bioactivity scores of 40 molecules with known anticancer properties were calculated for their antimetastatic, antineoplastic and anticarcinogenic properties. shows that the Pa value of 34 compounds was higher than the Pi value for anticancer properties, and compounds 30, 33 and 40 give a very high probability of activity in terms of anticancer, antineoplastic and antimetastatic properties.
Table 3. Selected 19 compounds for MD simulations and reference molecule.
Molecular docking
Comparison of 3D structures of BRPF1 protein
Docking was implemented for 3 different structures of the BRPF1 protein (PDB ID: 4QYL, 4UYE, 5O55) and all ligands given in Table S2. The results are given in Table S3 for comparison. Examining the energy values and the binding profiles obtained with the 3 structures, it was determined that the highest scores were obtained with the 4QYL structure.
The resolution values of the first (4QYL), the second (4UYE), and the third (5O55) structures are 1.8 Å, 1.64 Å, and 1.45 Å, respectively. The highest goodness of fit with experimental data was reached in the second structure (4UYE) with a rate of 95%, the lowest was reached in the third structure (5O55) with a rate of 75%. Considering these features of the structures and docking results, it was decided to continue the MD simulations with the first structure (4QYL).
Energetic results of docking
Molecular docking results with the binding affinities of the 19 selected molecules are shown in . The lowest binding affinity value of −9.2 kcal/mol was obtained in molecule 5, a seco-clerodane-type diterpenoid. In addition, the second-lowest binding affinity value of −9.1 kcal/mol was obtained in molecule 8, a newly discovered clerodane diterpenoid, and molecule 13, a known clerodane structure. Molecular docking results for the 3 highest-scoring binding modes of 40 molecules are also given in Table S4.
Table 4. Binding affinities of docked compounds.
The binding profiles of the reference molecule (9F9) and other molecules were also examined, and it was observed that there were significant interactions within the binding pocket of molecule 5. It is recognized that the reference molecule only makes 2 carbon-hydrogen bonds with ILE 24, while compound 5 makes 3 conventional hydrogen bonds with ASN 23, SER 26, and GLU 27. It has been revealed that the reference molecule is tightly packed by π-interactions. In addition, it is given in that molecule 5 is surrounded by van der Waals interactions and unfavourable bumps.
MD simulations
MD simulations were conducted for 20 molecules given in and RMSD values were calculated during the 150 ns simulation (Figure S2). The average RMSD value for all molecules is given in . When both RMSD plots and average RMSD values are compared with the simulation carried out with the reference molecule, it was observed that the complex systems were largely stable (Average RMSD values ~ 2). Small fluctuations observed in RMSD graphs were mostly detected before 100 ns (Figure S2). The protein was found to be stabilized after 100 ns.
Table 5. Average RMSD values during the 150 ns MD simulation of all compounds and reference.
To show the residues interacting with the ligands, the RMSF values of the Cα atoms were analysed from the time-averaged positions for the BRPF1 protein structure. Fluctuations in residues occur due to interactions with ligands. Changes in protein structure due to the binding of 20 compounds were expressed with RMSF graphs. In , the RMSF curves of molecule 5 and the reference compound are given on the same graph. It has been shown that molecule 5 causes the highest atomic fluctuations between residues 20–25 (orange), 30–35 (pink), and 79–85 (blue), and the positions of the relevant residues on the protein are given in .
Figure 2. RMSF values were observed during 150 ns MD simulation on residues in the BRPF1 protein. The black line plots compound 5 and the red line plots the reference compound.
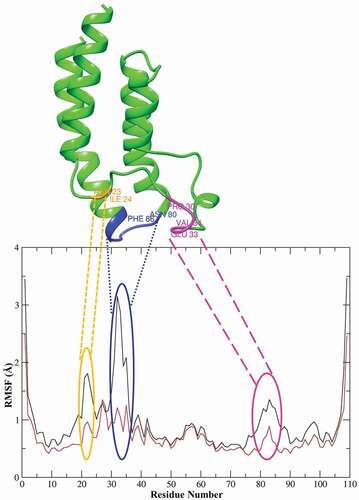
Trajectory analysis was performed for the 150 ns MD to understand the interaction of the compounds with BRPF1 in detail. Although all frames within 150 ns were scanned, more emphasis was placed on simulation beyond 100 ns due to high stability. As a result, although all compounds remained in the binding pocket throughout the simulation, they had little interaction with the protein (Figure S1). However, molecule 5 comes to the forefront by trajectory analysis, and it is revealed that it is packed in the active site with hydrogen bonds (). While the binding profile of molecule 5 with BRPF1 was examined, both the docking result and the MD result showed a great interaction between molecule 5 and the acyl group. While 2 hydrogen bonds were detected between the -O atom of the acyl group and the residues of GLU 27, and SER 26 in the docking results (), it was determined that the acyl group made 2 bonds with ASN 23 and GLU 27 in the MD results. In addition, the ‘linker -O atom’ that connects the acyl group to the molecular skeleton makes 1 hydrogen bond with PHE 47 residue. 3 hydrogen bonds were also detected between the -O atom in the furan ring and the residue GLU 33, and between the -O atom in the γ-lactone ring and ASN 80, ILE 24, and ILE 85 ().
Figure 3. Position and hydrogen bonds of 5 in the binding pocket after 150 ns of MD. The black dashed lines represent hydrogen bonds.
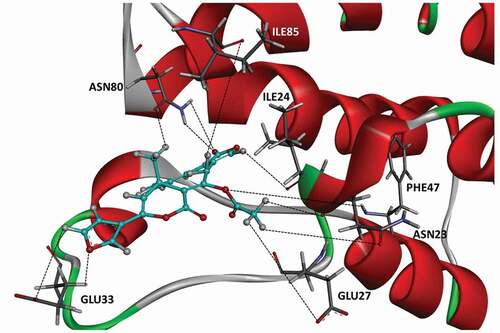
According to the trajectory analysis, the molecule that gives the best result in flavonoids is molecule 30. The binding profile between molecule 30 and BRPF1 is given in . The compound 30 contains 2 methyloxane rings and 5 hydrogen bonds were detected between the hydroxyl groups attached to these rings and residues ASN 23, GLU 27, and PRO 30. Despite the existent 5 hydrogen bonds, 2 new hydrogen bonds were detected between the hydroxyl groups attached to the dihydrochromene regions and residues GLU 33 and ASN 80.
Figure 4. Position and hydrogen bonds of compound 30 in the binding pocket after 150 ns of MD. The black dashed lines represent hydrogen bonds.
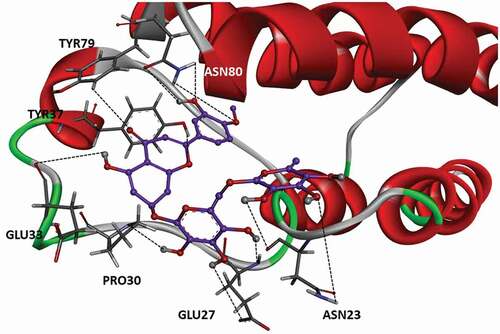
Furthermore, there are 2 hydrogen bonds between the -O atom in the dihydrochromene group and the TYR 37, and TYR 79 residues. Finally, 3 hydrogen bonds were detected between the ASN 80 residue and the hydroxyl, -OCH3 groups bound to the benzene ring ().
Hydrogen bond analysis was applied for 15,000 frames during the 150 ns simulation performed with all ligands. In the literature, bonds with an average bond distance of less than 2.5 Å and an average bond angle between 90–180° have been reported as acceptable [Citation47]. In the hydrogen bond analysis, the acceptable bonds were observed in 20 frames, and more were given in the filtered Table S5. All the hydrogen bonds in Table S5 were examined and it was determined that the majority of the compounds bonded with GLU 27, GLU 33, and ASN 80 at different frame numbers. The properties of hydrogen bonds developed during the simulation between molecule 5 and BRPF1 are given in . According to the results presented in , it is observed that molecule 5 forms hydrogen bonds with ASN 23 in the 9144 frames and with ASN 80 in the 4580 frames. In addition, hydrogen bonds with an average bond distance of 2.16 Å with the GLY 27 residue were observed only in 542 frames, although they had the lowest bond length.
Table 6. H bond analysis results for compound 5.
Determining the effectiveness of different functional groups in the formation of non-covalent interactions is a very important issue for drug development studies. In this study carried out with natural products, when the functional groups in the compounds are examined according to their hydrogen bond acceptor and donor properties, it is revealed that phenol, furan, methyloxane rings which have -O atoms in their structures are of key importance (, and S1). In addition, the functional groups that trigger interactions the most are the hydroxyl groups attached to these cyclic structures as -dihydroxy and -trihydroxy (Figure S1).
As in the molecule 18 (Euphoroylean B), molecule 6 (12 β-hydroxydolabella-(3E,7E)-diene) and molecule 1 (schistochilic acid D) it has been observed that the large crown structures of the compounds are not very effective in the ligand-BRPF1 interaction but lead to many unfavourable bumps ( and S1). Unfavourable bumps are also known to lower affinity as they indicate thrusts that destabilize the complex [Citation48]. Therefore, compounds are expected to bind in vitro with a higher affinity than is obtained in both docking and MM/PBSA results.
Another important functional group in diterpenoid structures is γ-lactone. Compound 5, which gives the highest scores in energetic, and trajectory analyses in our study, is a very important functional group for ligand-BRPF1 interaction ().
The most striking functional group in flavonoids is the benzopyrane (chromene) structure in the flavone skeleton. Another notable compound in this study is the molecule 30. When the hydrogen bonds of molecule 30 are examined, it is observed that methyloxane groups play a major role in the ligand-protein interaction as well as the dihydrochromene group ().
MM/PB(GB)SA calculations
The interactions of the compounds with BRPF1 cause changes in the energetic values. To determine this change and compare it with the change created by the reference molecule, MM/PB(GB)SA calculations were performed and given in . For the Poisson Boltzmann and Generalized Born solvation models, polar and non-polar energies were calculated separately for 20 different complexes. In addition, the van der Waals, electrostatic, and dispersion energies along with their standard errors of them are given in Table S6.
When the MM/PBSA ∆G values were sorted from the lowest to largest value, the compounds 5, 30, 11, 13, and 5 got the values of −19.92 ± 0.1 kcal/mol, −16.67 ± 1.4 kcal/mol, 12, −15.39 ± 1.0 kcal/mol, and −14.76 ± 0.1 kcal/mol, respectively. If similarly sorting for MM/GBSA ∆G results, −59.90 ± 1.0 kcal/mol for compound 30, −52.54 ± 0.7 kcal/mol for compound 5, −48.19 ± 0.8 kcal/mol for compound 11 and −46.84 for compound 40 ± 0.5 kcal/mol values were obtained. However, considering the reference value, only molecule 5 had a better score than the reference molecule (−16.87 ± 0.9 kcal/mol) in terms of MM/PBSA ∆G value, but only composite 30 had a better score than the reference (−52.69 ± 0.7 kcal/mol) in terms of MM/GBSA ∆G value.
The effect of different energy components on total energy was also analysed. The energy composition in the MM/PB(GB)SA results is given in Table S6. Also, demonstrates the energy composition of compounds 5, 30, and reference compounds. Accordingly, it has been determined that the highest effect on energy is provided by van der Waals interactions, while the lowest effect is achieved by the electrostatic effect. On the other hand, Generalized Born solvation has been observed to make the energy more negative, but this has led to quite different energetic results from the docking results. Tables S6 and S7 show that the MM/PBSA results are closer to the docking results.
Table 7. Binding free energies for compounds 5, 30 and reference.
Although docking and MM/PBSA results were found to be relatively close to each other in our study, MM/GBSA results were found to be quite different. Although it has been reported in the literature that MM/PBSA analyses give results very close to the experimental results [Citation47], different opinions have also been encountered [Citation45,Citation49,Citation50].
Conclusions
Diterpenoids and flavonoids are natural products whose anticancer properties have been extensively demonstrated in the literature [Citation8,Citation12,Citation15,Citation16,Citation37,Citation51–53]. In this study, the biological activities of 40 natural compounds were investigated by in silico SAR analysis. It was observed that the Pa value of 34 compounds for anticancer properties was higher than the Pi value. In the second step, 19 diterpenoids and flavonoids showing inhibitory properties specific to BRPF1 protein were examined by in silico methods. Both docking results and MD simulation results with energetic analyzes showed that molecule 5 gave higher scores than the reference compound (9F9). In the RMSF analysis, fluctuations triggered by hydrogen bonds and hydrophobic interactions in the residues were investigated. All compounds were shown in Figure S3 to interact with the active site identified in the literature for the BRPF1 protein [Citation2,Citation54,Citation55].
In conclusion, it is computationally shown that compounds 5 and 30 are potential novel BRPF1 inhibitors due to their natural anticancer agent properties against leukaemia and HCC. According to the MD simulation performed with the explicit solvent model revealed that the system was stabilized by the binding of compounds 5 and 30. When all diterpenoids and flavonoids used in the study are examined, it is revealed that different functional groups with the -O atom they contain trigger non-covalent interactions. Based on these results, although in vitro and in vivo analyzes are recommended to understand the true inhibitory potential of compounds 5 and 30, the molecules show promise as anticancer agents.
Supplemental Material
Download PDF (1.2 MB)Acknowledgements
The author thanks the Max Planck Institute for Dynamics of Complex Technical Systems for providing computing resources.
This article was performed within the framework of COST (European Cooperation in Science and Technology) Action CA17104 (STRATAGEM) – “New diagnostic and therapeutic tools against multidrug-resistant tumors”.
Disclosure statement
No potential conflict of interest was reported by the author(s).
Supplementary material
Supplemental data for this article can be accessed at: https://doi.org/10.1080/1062936X.2022.2096113
Additional information
Funding
References
- R.L. Siegel, K.D. Miller, H.E. Fuchs, and A. Jemal, Cancer statistics, 2021, CA, Cancer J. Clin. 71 (2021), pp. 7–33. doi:10.3322/caac.21654.
- J. Zhu, C. Zhou, and A. Caflisch, Structure-based discovery of selective BRPF1 bromodomain inhibitors, Eur. J. Med. Chem. 155 (2018), pp. 337–352. doi:10.1016/j.ejmech.2018.05.037.
- S.P. Boyson, C. Gao, K. Quinn, J. Boyd, H. Paculova, S. Frietze, and K.C. Glass, Functional roles of bromodomain proteins in cancer, Cancers (Basel) 13 (2021), pp. 3606. doi:10.3390/cancers13143606.
- S. Gokani and L.K. Bhatt, Bromodomains: A novel target for the anticancer therapy, Eur. J. Pharmacol. 911 (2021), pp. 174523. doi:10.1016/j.ejphar.2021.174523.
- E.H. Demont, P. Bamborough, C. Chung, P.D. Craggs, D. Fallon, L.J. Gordon, P. Grandi, C.I. Hobbs, J. Hussain, E.J. Jones, A. Le Gall, A.-M. Michon, D.J. Mitchell, R.K. Prinjha, A.D. Roberts, R.J. Sheppard, and R.J. Watson, 1,3-Dimethyl benzimidazolones are potent, selective inhibitors of the BRPF1 bromodomain, ACS Med. Chem. Lett. 5 (2014), pp. 1190–1195. doi:10.1021/ml5002932.
- C.L.H. Cheng, F.H.C. Tsang, L. Wei, M. Chen, D.W.C. Chin, J. Shen, C.T. Law, D. Lee, C.C.L. Wong, I.O.L. Ng, and C.M. Wong, Bromodomain-containing protein BRPF1 is a therapeutic target for liver cancer, Commun. Biol. 4 (2021), pp. 1–14. doi:10.1038/s42003-021-02405-6.
- J. Cheung, E.N. Gary, K. Shiomi, and T.L. Rosenberry, Structures of human acetylcholinesterase bound to dihydrotanshinone I and territrem B show peripheral site flexibility, ACS Med. Chem. Lett. 4 (2013), pp. 1091–1096. doi:10.1021/ml400304w.
- S.R. Lin, C.H. Chang, C.F. Hsu, M.J. Tsai, H. Cheng, M.K. Leong, P.J. Sung, J.C. Chen, and C.F. Weng, Natural compounds as potential adjuvants to cancer therapy: Preclinical evidence, Br. J. Pharmacol. 177 (2020), pp. 1409–1423. doi:10.1111/bph.14816.
- X. Montané, O. Kowalczyk, B. Reig-Vano, A. Bajek, K. Roszkowski, R. Tomczyk, W. Pawliszak, M. Giamberini, A. Mocek-Płóciniak, and B. Tylkowski, Current perspectives of the applications of polyphenols and flavonoids in cancer therapy, Molecules. 25 (2020), pp. 3342. doi:10.3390/molecules25153342.
- B.M.F. Gonçalves, D.S.P. Cardoso, and M.J.U. Ferreira, Overcoming multidrug resistance: Flavonoid and terpenoid nitrogen-containing derivatives as ABC transporter modulators, Molecules. 25 (2020), pp. 3364. doi:10.3390/molecules25153364.
- F. Fontana, M. Raimondi, M. Marzagalli, A. Di Domizio, and P. Limonta, Natural compounds in prostate cancer prevention and treatment: Mechanisms of action and molecular targets, Cells. 9 (2020), pp. 460. doi:10.3390/cells9020460.
- E.R. Sauter, Cancer prevention and treatment using combination therapy with natural compounds, Expert Rev. Clin. Pharmacol. 13 (2020), pp. 265–285. doi:10.1080/17512433.2020.1738218.
- W.K. Yu, Z.Y. Xu, L. Yuan, S. Mo, B. Xu, X.D. Cheng, and J.J. Qin, Targeting β-catenin signaling by natural products for cancer prevention and therapy, Front. Pharmacol. 11 (2020), pp. 1–12. doi:10.3389/fphar.2020.00984.
- D. Jones, G.O. Ormondroyd, S.F. Curling, C.-M. Popescu, and M.-C. Popescu. 2 - Chemical Compositions of Natural Fibres, M. Fan and F. Fu, eds., Woodhead Publishing, Sawston, United Kingdom, 2017, pp. 23–58.
- R. Li, S.L. Morris-Natschke, and K.-H. Lee, Clerodane diterpenes: Sources, structures, and biological activities, Nat. Prod. Rep. 33 (2016), pp. 1166–1226. doi:10.1039/c5np00137d.
- A.N. Panche, A.D. Diwan, and S.R. Chandra, Flavonoids: An overview, J. Nutr. Sci. 5 (2016), pp. e47. doi:10.1017/jns.2016.41.
- D. Luo, J.B. Tong, X.C. Xiao, S. Bian, X. Zhang, J. Wang, and H.Y. Xu, Theoretically exploring selective-binding mechanisms of BRD4 through integrative computational approaches, SAR QSAR Environ. Res. 32 (2021), pp. 985–1011. doi:10.1080/1062936X.2021.1999317.
- S.L. Wu, J. Zhao, H.B. Sun, H.Y. Li, Y.Y. Yin, and L.L. Zhang, Insights into interaction mechanism of inhibitors E3T, E3H and E3B with CREB binding protein by using molecular dynamics simulations and MM-GBSA calculations, SAR QSAR Environ. Res. 32 (2021), pp. 221–246. doi:10.1080/1062936X.2021.1887351.
- S.L. Wu, L.F. Wang, H.B. Sun, W. Wang, and Y.X. Yu, Probing molecular mechanism of inhibitor bindings to bromodomain-containing protein 4 based on molecular dynamics simulations and principal component analysis, SAR QSAR Environ. Res. 31 (2020), pp. 547–570. doi:10.1080/1062936X.2020.1777584.
- L.F. Wang, Y. Wang, Z.Y. Yang, J. Zhao, H.B. Sun, and S.L. Wu, Revealing binding selectivity of inhibitors toward bromodomain-containing proteins 2 and 4 using multiple short molecular dynamics simulations and free energy analyses, SAR QSAR Environ. Res. 31 (2020), pp. 373–398. doi:10.1080/1062936X.2020.1748107.
- Y. Wang, L.F. Wang, L.L. Zhang, H.B. Sun, and J. Zhao, Molecular mechanism of inhibitor bindings to bromodomain-containing protein 9 explored based on molecular dynamics simulations and calculations of binding free energies, SAR QSAR Environ. Res. 31 (2020), pp. 149–170. doi:10.1080/1062936X.2019.1701075.
- Y.H. Kim, M. Kim, M. Yoo, J.E. Kim, H.K. Lee, J.N. Heo, C.O. Lee, M. Yoo, K.Y. Jung, C.S. Yun, S.W. Moon, H.K. Chang, C.W. Chung, S. Pyo, S.U. Choi, and C.H. Park, A natural compound, aristoyagonine, is identified as a potent bromodomain inhibitor by mid-throughput screening, Biochem. Biophys. Res. Commun. 503 (2018), pp. 882–887. doi:10.1016/j.bbrc.2018.06.091.
- F.D. Prieto-Martinez and J.L. Medina-Franco, Charting the bromodomain BRD4: Towards the identification of novel inhibitors with molecular similarity and receptor mapping, Lett. Drug Des. Discov. 15 (2018), pp. 1002–1011. doi:10.2174/1570180814666171121145731.
- J. Li, W. Zou, K. Yu, B. Liu, W. Liang, L. Wang, Y. Lu, Z. Jiang, A. Wang, and J. Zhu, Discovery of the natural product 3’,4’,7,8-tetrahydroxyflavone as a novel and potent selective BRD4 bromodomain 2 inhibitor, J. Enzyme Inhib. Med. Chem. 36 (2021), pp. 903–913. doi:10.1080/14756366.2021.1906663.
- I.A. Ramallo, V.L. Alonso, F. Rua, E. Serra, and R.L.E. Furlan, A bioactive Trypanosoma cruzi bromodomain inhibitor from chemically engineered extracts, ACS Comb. Sci. 20 (2018), pp. 220–228. doi:10.1021/acscombsci.7b00172.
- D.A. Filimonov, A.A. Lagunin, T.A. Gloriozova, A.V. Rudik, D.S. Druzhilovskii, P.V. Pogodin, and V.V. Poroikov, Prediction of the biological activity spectra of organic compounds using the pass online web resource, Chem. Heterocycl. Compd. 50 (2014), pp. 444–457. doi:10.1007/s10593-014-1496-1.
- S. Kim, P.A. Thiessen, E.E. Bolton, J. Chen, G. Fu, A. Gindulyte, L. Han, J. He, S. He, B.A. Shoemaker, J. Wang, B. Yu, J. Zhang, and S.H. Bryant, PubChem substance and compound databases, Nucl. Acids Res. 44 (2016), pp. D1202–D1213. doi:10.1093/nar/gkv951.
- Discovery studio visualizer. Dassault Systèmes, San Diego, USA, 2019; software available at https://www.3dsbiovia.com/products/collaborative-science/biovia-discovery-studio/visualization-download.php.
- O. Trott and A. Olson, AutoDock Vina: Improving the speed and accuracy of docking with a new scoring function, efficient optimization, and multithreading, J. Comput. Chem. 31 (2010), pp. 455–461. doi:10.1002/jcc.21334.
- T. Gaillard, Evaluation of AutoDock and AutoDock Vina on the CASF-2013 benchmark, J. Chem. Inf. Model. 58 (2018), pp. 1697–1706. doi:10.1021/acs.jcim.8b00312.
- S.K. Burley, C. Bhikadiya, C. Bi, S. Bittrich, L. Chen, G.V. Crichlow, C.H. Christie, K. Dalenberg, L. Di Costanzo, J.M. Duarte, S. Dutta, Z. Feng, S. Ganesan, D.S. Goodsell, S. Ghosh, R.K. Green, V. Guranović, D. Guzenko, B.P. Hudson, C.L. Lawson, Y. Liang, R. Lowe, H. Namkoong, E. Peisach, I. Persikova, C. Randle, A. Rose, Y. Rose, A. Sali, J. Segura, M. Sekharan, C. Shao, Y.-P. Tao, M. Voigt, J.D. Westbrook, J.Y. Young, C. Zardecki, and M. Zhuravleva, RCSB protein data bank: Powerful new tools for exploring 3D structures of biological macromolecules for basic and applied research and education in fundamental biology, biomedicine, biotechnology, bioengineering and energy sciences, Nucl. Acids Res. 49 (2021), pp. D437–D451. doi:10.1093/nar/gkaa1038.
- M.Y. Lubula, B.E. Eckenroth, S. Carlson, A. Poplawski, M. Chruszcz, and K.C. Glass, Structural insights into recognition of acetylated histone ligands by the BRPF1 bromodomain, FEBS Lett. 588 (2014), pp. 3844–3854. doi:10.1016/j.febslet.2014.09.028.
- G.M. Morris, H. Ruth, W. Lindstrom, M.F. Sanner, R.K. Belew, D.S. Goodsell, and A.J. Olson, AutoDock4 and AutoDockTools4: Automated docking with selective receptor flexibility, J. Comput. Chem. 30 (2009), pp. 2785–2791. doi:10.1002/jcc.21256.
- E. Bautista, R.A. Toscano, and A. Ortega, 5,10- seco - neo -clerodanes and neo -clerodanes from Salvia microphylla, J. Nat. Prod. 77 (2014), pp. 1088–1092. doi:10.1021/np4009893.
- S.Y. Ng, T. Kamada, M. Suleiman, and C.S. Vairappan, A new seco-clerodane-type diterpenoid from Bornean liverwort Schistochila acuminata, Nat. Prod. Commun. 11 (2016), pp. 1071–1072.
- A. Moulishankar and K. Lakshmanan, Data on molecular docking of naturally occurring flavonoids with biologically important targets, Data Br. 29 (2020), pp. 105243. doi:10.1016/j.dib.2020.105243.
- J. Rivera-Chávez, C. Bustos-Brito, E. Aguilar-Ramírez, D. Martínez-Otero, L.D. Rosales-Vázquez, A. Dorazco-González, and P. Cano-Sánchez, Hydroxy- neo-clerodanes and 5,10-seco-neo-clerodanes from Salvia decora, J. Nat. Prod. 83 (2020), pp. 2212–2220. doi:10.1021/acs.jnatprod.0c00313.
- S. Shaker, J. Sang, X.L. Yan, R.Z. Fan, G.H. Tang, Y.K. Xu, and S. Yin, Diterpenoids from Euphorbia royleana reverse P-glycoprotein-mediated multidrug resistance in cancer cells, Phytochemistry. 176 (2020), pp. 112395. doi:10.1016/j.phytochem.2020.112395.
- F. Aydoğan, E.H. Anouar, M. Aygün, H. Yusufoglu, C. Karaalp, and E. Bedir, An unprecedented diterpene with three new neoclerodanes from Teucrium sandrasicum O. Schwarz, J. Mol. Struct. 1231 (2021), pp. 1–12.
- C. Bustos-Brito, D. Pérez-Juanchi, J. Rivera-Chávez, A.D. Hernández-Herrera, B.Y. Bedolla-García, S. Zamudio, T. Ramírez-Apan, L. Quijano, and B. Esquivel, Clerodane and 5 10-seco-clerodane-type diterpenoids from Salvia involucrata, J. Mol. Struct. 1237 (2021), pp. 130367. doi:10.1016/j.molstruc.2021.130367.
- ACD/ChemSketch, Advanced Chemistry Development Inc., Toronto, Canada, 2001; software available at www.acdlabs.com
- D.A. Case, H.M. Aktulga, K. Belfon, I.Y. Ben-Shalom, S.R. Brozell, D.S. Cerutti, T.E. Cheatham, V.W.D. Cruzeiro, T.A. Darden, R.E. Duke, G. Giambasu, M.K. Gilson, H. Gohlke, A.W. Goetz, R. Harris, S. Izadi, S.A. Izmailov, C. Jin, K. Kasavajhala, and P.A. Kollman, Amber 2021, 2021; software available at https://ambermd.org/AmberMD.php
- J. Wang, R.M. Wolf, J.W. Caldwell, P.A. Kollman, and D.A. Case, Development and testing of a general amber force field, J. Comput. Chem. 25 (2004), pp. 1157–1174. doi:10.1002/jcc.20035.
- D.R. Roe and T.E. Cheatham, PTRAJ and CPPTRAJ: Software for processing and analysis of molecular dynamics trajectory data, J. Chem. Theory Comput. 9 (2013), pp. 3084–3095. doi:10.1021/ct400341p.
- S. Genheden and U. Ryde, The MM/PBSA and MM/GBSA methods to estimate ligand-binding affinities, Expert Opin. Drug Discov. 10 (2015), pp. 449–461. doi:10.1517/17460441.2015.1032936.
- S.S. Timur, G. Yalçın, Ö. Çevik, C. Andaç, and R.N. Gürsoy, Molecular dynamics, thermodynamic, and mutational binding studies for tumor-specific LyP-1 in complex with p32, J. Biomol. Struct. Dyn. 1102 (2017), pp. 1–11.
- S. Burmaoglu, E.A. Kazancioglu, M.Z. Kazancioglu, R. Sağlamtaş, G. Yalcin, I. Gulcin, and O. Algul, Synthesis, molecular docking and some metabolic enzyme inhibition properties of biphenyl-substituted chalcone derivatives, J. Mol. Struct. 1254 (2022), pp. 132358. doi:10.1016/j.molstruc.2022.132358.
- A.S.A. Manap, A.C.W. Tan, W.H. Leong, A.Y.Y. Chia, S. Vijayabalan, A. Arya, E.H. Wong, F. Rizwan, U. Bindal, S. Koshy, and P. Madhavan, Synergistic effects of curcumin and piperine as potent acetylcholine and amyloidogenic inhibitors with significant neuroprotective activity in sh-sy5y cells via computational molecular modeling and in vitro assay, Front. Aging Neurosci. 10 (2019), pp. 1–17.
- M.C. Lee, R. Yang, and Y. Duan, Comparison between generalized-Born and Poisson-Boltzmann methods in physics-based scoring functions for protein structure prediction, J. Mol. Model. 12 (2005), pp. 101–110. doi:10.1007/s00894-005-0013-y.
- G. Poli, G. Carlotta, F. Rizzolio, and T. Tuccinardi, Application of MM-PBSA methods in virtual screening, Molecules. 25 (2020), pp. 459–494. doi:10.3390/molecules25081971.
- Y.C. Huang, P.C. Lee, J.J. Wang, and Y.C. Hsu, Anticancer effect and mechanism of hydroxygenkwanin in oral squamous cell carcinoma, Front. Oncol. 9 (2019), pp. 1–10.
- V. Singamaneni, B. Lone, J. Singh, P. Kumar, S. Gairola, S. Singh, and P. Gupta, Coronarin K and L: Two novel labdane diterpenes from Roscoea purpurea: An ayurvedic crude drug, Front. Chem. 9 (2021), pp. 1–7.
- Y. Xia, R. Chen, G. Lu, C. Li, S. Lian, T.W. Kang, and Y. Do Jung, Natural phytochemicals in bladder cancer prevention and therapy, Front. Oncol. 11 (2021), pp. 1–22. doi:10.3389/fonc.2021.652033.
- S.S. Çınaroǧlu and E. Timuçin, Comprehensive evaluation of the MM-GBSA method on bromodomain-inhibitor sets, Brief. Bioinform. 21 (2020), pp. 2112–2125. doi:10.1093/bib/bbz143.
- W.S. Palmer, Development of small molecule inhibitors of BRPF1 and TRIM24 bromodomains, Drug Discov. Today Technol. 19 (2016), pp. 65–71. doi:10.1016/j.ddtec.2016.06.005.