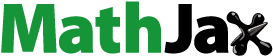
ABSTRACT
Phytochemicals are now increasingly exploited as remedial agents for the management of diabetes due to side effects attributable to commercial antidiabetic agents. This study investigated the structural and molecular mechanisms by which betulinic acid exhibits its antidiabetic effect via in vitro and computational techniques. In vitro antidiabetic potential was analysed via on α-amylase, α-glucosidase, pancreatic lipase and α-chymotrypsin inhibitory assays. Its structural and molecular inhibitory mechanisms were investigated using Density Functional Theory (DFT) analysis, molecular docking and molecular dynamics (MD) simulation. Betulinic acid significantly (p < 0.05) inhibited α-amylase, α-glucosidase, pancreatic lipase and α-chymotrypsin enzymes with IC50 of 70.02 μg/mL, 0.27 μg/mL, 1.70 μg/mL and 8.44 μg/mL, respectively. According to DFT studies, betulinic acid possesses similar reaction in gaseous phase and water due to close values observed for highest occupied molecular orbital (HOMO) and lowest occupied molecular orbital (LUMO) and the chemical descriptors. The dipole moment indicates that betulinic acid has high polarity. Molecular electrostatic potential surface revealed the electrophilic and nucleophilic attack-prone atoms of the molecule. Molecular dynamic studies revealed a stable complex between betulinic acid and α-amylase, α-glucosidase, pancreatic lipase and α-chymotrypsin. The study elucidated the potent antidiabetic properties of betulinic acid by revealing its conformational inhibitory mode of action on enzymes involved in the onset of diabetes.
Introduction
Diabetes has remained one of the fastest growing global health issues affecting the total health and well-being of individuals, with about 537 million cases recorded in 2021, causing a high rate of global morbidity and mortality [Citation1]. Diabetes is a metabolic disease characterized by impairments in the metabolism of carbohydrates, fats and proteins, leading to excessive glucose and lipid levels in circulation [Citation1]. High blood glucose (hyperglycaemia) is the key culprit in the pathophysiology of diabetes. It results from alterations in insulin secretion and insulin action due to impairment in β-cell function and insulin resistance, respectively [Citation2,Citation3]. Obesity is a major risk factor for several metabolic diseases especially type 2 diabetes, with insulin resistance emerging as the major link [Citation4]. Excessive consumption of carbohydrates and decreased physical exercise have been associated with insulin resistance, and high production of hepatic glucose and release of fatty acids (lipolysis) are its known major characteristics [Citation5,Citation6]. Additionally, increased activity of plasma protease has been attributed to obesity-induced insulin resistance in type 2 diabetes [Citation7]. Thus, effective regulation of glucose homoeostasis as well as lipid and protein metabolisms are vital for the prevention of diabetes [Citation8].
With the basic knowledge of diabetes pathophysiology, one of the key treatment approaches is targeted towards inhibition of carbohydrate (such as α-glucosidase) and lipid (pancreatic lipase) digestive enzyme activities as well as serine proteases (such as chymotrypsin). Inhibition of nutrient digestion and absorption help to reduce postprandial blood glucose levels, maintain glucose homoeostasis and improve insulin sensitivity [Citation7,Citation9,Citation10]. Common commercial α-glucosidase, lipase and chymotrypsin enzyme inhibitors include acarbose, orlistat and chymostatin, respectively. However, due to the side effects reported on the use of several types of glucose-lowering drugs and the cost of treatment of diabetes [Citation11,Citation12], there has been a paradigm shift to the use of natural, safer and inexpensive alternatives. Phytochemicals have increasingly been exploited as therapeutic targets towards the management of hyperglycaemia and diabetes complications owing to their glucose lowering properties [Citation13,Citation14]. Among these phytochemicals is betulinic acid.
Betulinic acid (3β-Hydroxy-lup-20(29)-en-28-oic acid), a pentacyclic lupane-type triterpene (), is among the plant phytochemicals that have been proven to have a wide range of pharmacological activities [Citation15,Citation16]. These include anti-inflammatory, anti-cancer, hepato-protective, antimalarial, anti-bacterial and anti-HIV activities [Citation16–18]. The anti-diabetic properties of betulinic acid have been demonstrated in streptozotocin and nicotinamide induced diabetic mice by reducing blood glucose and amylase concentrations as well as improving insulin sensitivity and pancreatic histology [Citation19]. Chen et al. [Citation20] reported the potent inhibitory effect of betulinic acid on α-glucosidase and its ameliorative effect on postprandial hyperglycaemia in type 2 diabetes condition. Betulinic acid was also reported by Ajala-Lawal et al. [Citation15] to attenuate hyperglycaemia, oxidative stress and inflammation and improve insulin insensitivity in high-fructose induced diabetic rats. Additionally, the anti-obesity effect of the triterpene was demonstrated via its ability to inhibit pancreatic lipase [Citation21]. Despite the numerous reported antidiabetic studies on betulinic acid, there is a paucity of information on the detailed molecular mechanisms by which the triterpene exerts its inhibitory effect on the major digestive enzymes involved in the development of diabetes.
Computational modelling approaches have now become essential for drug discovery process and development. They help to reveal 3D structures and ligand binding orientations of most clinically important targets, in a molecular state critical to its biofunction [Citation22]. These computational techniques including molecular docking, molecular dynamic simulations, free energy calculations and post-analysis profiling predictions help to predict inhibitors of proteins responsible for a particular disease. Molecular modelling and simulations provide deeper understanding and useful information on drug–target interaction at electronic and atomic level, which serve as a guide for executing more specific in vitro and in vivo experimental procedures [Citation23,Citation24]. Thus, the present study is aimed at elucidating the structural and molecular mechanisms by which betulinic acid exerts it inhibitory action on a-glucosidase, a-amylase, pancreatic lipase and chymotrypsin enzymes to gain deeper knowledge on it the antidiabetic properties, using in vitro and in silico models.
Materials and methods
Betulinic acid
Betulinic acid (≥98% HPLC grade; Mw: 456.70 g/mol; Batch No: MKCQ5563) was procured from Sigma-Aldrich, Johannesburg, South Africa. A stock solution of 10 mg/mL was prepared for further analysis.
In vitro biological activities
α-Glucosidase inhibitory activity
The inhibitory potential of betulinic acid on α-glucosidase enzyme was determined using a previously described protocol (Ademiluyi and Oboh, 2013). Briefly, 50 µL each of the sample or standard (acarbose) concentrations (15–240 µg/mL) was mixed with 100 µL solution of 1.0 U/mL α-glucosidase in phosphate buffer (100 mM, pH 6.8) and incubated for 15 min at 37°C. About 50 μL of 5 mM p-nitrophenyl-α-D-glucopyranoside (pNPG) in phosphate buffer was then added to the reaction mixture and further incubated for 20 min at 37°C. Absorbance of the liberated p-nitrophenol from pNPG was measured at 405 nm. The inhibitory activity was calculated as expressed below:
α-Amylase inhibitory activity
α-amylase inhibitory activity of betulinic acid was determined with slight modification of a previously described method [Citation2]. Briefly, 50 µL each of the sample or standard (acarbose) concentrations (15–240 µg/mL) was incubated with 100 µL solution of 2.0 U/mL porcine pancreatic amylase in 100 mM phosphate buffer (pH 6.8) for 20 min at 37°C. About 50 µL of 1% starch in phosphate buffer was then added to the mixture and further incubated for 10 min at 37°C. Two hundred microlitres of dinitrosalicylate (DNS) reagent was added to the mixture and boiled for 10 min. Absorbance was read at 540 nm. The inhibitory activity was calculated according to the expression below:
Pancreatic lipase inhibitory activity
The pancreatic lipase enzyme inhibitory activity of betulinic acid was determined with slight modification of a previously described method [Citation25]. One hundred microlitres of each sample or standard (orlistat) concentrations was mixed with 169 μL of Tris buffer (100 mM Tris – HC1 and 5 mM CaCl2, pH 7.0). Forty microlitres of porcine pancreatic lipase solution (2.5 mg/mL) in 10 mM MOPS (morpholine propane sulphonic acid) and 1 mM ethylenediaminetetraacetic acid (EDTA; pH 6.8) was then added and incubated for 15 min at 37°C. Five microlitres of the substrate (10 mM p-NPB (p-nitrophenyl butyrate in dimethyl formamide)) was added to the reaction mixture and further incubated for 30 min at 37°C. Absorbance was read at 405 nm. The inhibitory activity was calculated using the formula below:
α-Chymotrypsin inhibitory activity
The inhibitory activity of chymotrypsin was carried out according to the method described by Saleem et al. [Citation26]. A mixture containing 60 μl of 50 mM Tris-HCl buffer (pH 7.6), 15 μl (0.9 units) of chymotrypsin (Sigma, USA) and varying concentrations (15–240 µg/mL) of betulinic acid or the standard protease inhibitor, chymostatin was incubated at 37°C for 20 min. After incubation, 15 μL of N-succinyl phenyl-alanine-p-nitroanilide (1.3 mM) was added to the mixture and incubated for another 30 min (37°C). The absorbance was read at 410 nm, and the percentage inhibition was calculated as
Computational studies
Density functional theory (DFT)
The three-dimensional (3D) spatial data file (SDF) format of betulinic acid was retrieved from PubChem structure library Betulinic Acid | C30H48O3 – PubChem (nih.gov). The molecule visualization platform, Gauss View [Citation27] was used to visualize, clean, and set up the retrieved structure for calculations. DFT calculations were performed using Gaussian ’16 computational chemistry software package available on the centre for high-performance computing (CHPC) cluster in Cape Town. For all calculations, m06-2× functional; a hybrid meta exchange-correlation functional recommended for main group chemistry was used [Citation28]. In addition, a no-diffuse 6–311 g(d,p) basis set suitable for neutral organic molecules was chosen. All calculations were performed, first in the vacuum, and then in water to check for solvent effect on the chemical activity of betulinic acid.
Molecular dynamics
System preparation
X-ray crystal structures of Pancreatic-lipase, α-Amylase, α-Chymotrypsin and α-Glucosidase enzymes (PDB code: 1LPB, 1HOE, 2CHA and 3L4Y) [Citation29–32] was obtained from RSCB Protein Data Bank (RSCB PDB) [Citation33]. In order to prepare these structures for molecular dynamic simulation, we utilized the Molegro Molecular Viewer (MMV) [Citation34,Citation35] and UCSF Chimera software package. All missing residues from two enzymes were added by a structural refinement tool known as Modeller [Citation36].
Molecular docking and molecular dynamic simulations
Preceding docking, UCSF Chimera was used to eliminate all co-factors and non-standard residues such as Cl−, Na+, etc. as well as water molecules surrounding the protein structure [Citation36]. Afterwards, betulinic acid was charged and hydrogenated. AutoDock Tools GUI was used to estimate the ligand docking and adding of a partial Gasteiger charge [Citation36,Citation37] as the docking score results for indicated inhibitor bonded to pancreatic-lipase, α-amylase, α-chymotrypsin and α-glucosidase enzymes are −5.5, −4.3, −6.4 and −5–2, respectively. Overall, systems consisting of all enzymes bound to betulinic acid underwent a 200 ns MD simulation using the AMBER20 CPU and GPU packages [Citation38,Citation39], PMEMD engine. An atomic partial charge was generated for betulinic acid inhibitor by means of the AMBER FF14SB of the ANTECHAMBER programme [Citation40,Citation41]. The Leap module of Amber 20 enabled the addition of hydrogen atoms, sodium (Na+) or chloride (Cl−) counter ions to the systems for neutralization. The systems were initially minimized for 2500 steps with 500 kcal/mol Å2 restraint potential, and a whole minimization step of 5000 steps was further run without restraint using the conjugate algorithm. Thereafter, the system underwent gradual heating from 0 to 300 K for 50 ps by means of a Langevin thermostat in a canonical assemblage (NVT) [Citation42,Citation43]. Following heating, equilibration was undertaken at a temperature of 300 K, excluding all restraints, and a maintained atmospheric pressure of 1 bar by utilizing the Berendsen barostat until all systems had reached equilibration [Citation44–46]. The complex then underwent a 200 ns MD simulation with the SHAKE algorithm being employed to constrict all hydrogen atoms [Citation47]. Co-ordinates of the four enzymes bonded to betulinic acid complexes were saved after the 200 ns production period at every 1 picosecond (1 ps) intervals. The generated trajectories of each system were analysed using the PTRAJ and CPPTRAJ modules [Citation48]. The generated data and subsequent complexes were visualized using Microcal Origin analytical software [Citation49,Citation50], UCSF Chimera [Citation36].
Post-molecular dynamic simulation analysis
The complexes formed were saved at intervals of 1 ps, and subsequent trajectories were analysed using the integrated CPPTRAJ module within AMBER 20. Post-simulation analysis for the systems included assessments of protein stability (RMSD), flexibility (RMSF) and solvent accessible surface area (SASA). The computation of binding free energy was accomplished through the utilization of the Molecular Mechanics/Generalized Born Surface Area (MM/GBSA) technique.
Dynamic cross-correlation matrix (DCCM) analysis
We used dynamic cross-correlation analysis to investigate the fluctuations and movements in the backbone of the α-carbon atoms. Cross-correlation components for i and j Cα atoms are shown in EquationEquation 1(1)
(1) :
where ri = Cαi = standard time throughout the MD trajectory. Significantly correlated movements are symbolized by Cij = 1, while Cij = −1 symbolized highly anticorrelated movements in the trajectory. The divergence of motion from 1 to − 1 indicates that i and j movements are anticorrelated. The DCCM matrix was carried out using the CPPTRAJ package in Amber 20, and the matrices were plotted and evaluated using Origin software (www.originlab.com).
Thermodynamic (free binding energy) computations
The Molecular Mechanics/Generalized Born Surface Area (MM/GBSA) approach [Citation51–57] was utilized to determine an estimate of the binding free energy between betulinic acid inhibitor and the enzymes pancreatic-lipase, α-amylase, α-chymotrypsin, and α-glucosidase. The binding free energy, denoted as ΔGbind, serves as a dependable tool widely employed to quantify the energies involved in the formation of protein-ligand complexes. It is calculated using the following equation:
The ΔGbind is the sum of the gas and solvent energy minus entropy (TS).
where Egas is representative of the addition of the internal energy terms of the AMBER force fields which includes Eint which represents angles, torsions and bonds, Evdw which represents covalent van der Waals energy and Eelec which represents the electrostatic energy components that are non-bonded.
The following equation is representative of the solvent energy calculation:
GGB is representative of the polar solvation impact and GSA is representative of the non-polar solvation impact which is calculated using the solvent accessible surface area (SASA). This is attained by using a water probe radius of 1.4 Å. A surface tension constant (c) was represented by a measure of 0.0072 kcal/mol and ‘b’ to 0 kcal/mol [Citation37,Citation58].
Statistical analysis
Results are presented as mean ± SD of triplicate (n = 3) experiments. Data analysis was achieved by using SPSS (Windows V25) and statistically significant differences (p < 0.05) between the test groups were determined using a one-way analysis of variance and followed by the comparison of mean values with Tukey’s HSD multiple range test.
Results and discussion
Chronic hyperglycaemia, a typical culprit of diabetes pathology, is characterized by disturbances in the metabolism of carbohydrates, fats and proteins, which further progresses and complicates the disease. As a multifaceted chronic disease, several therapeutic agents have been exploited in the management of diabetes as evident by clinically used drug varieties [Citation59]. Due to the numerous presenting adverse effects of the drugs, coupled with issues of affordability, plants and their phytochemicals have become a subject of interest for managing the disease. The onus is on researchers to continually explore these natural products to understand their mechanisms of action with the aim of identifying a drug candidate. In the present study, the structural and molecular mechanisms by which betulinic acid, a plant triterpene, demonstrates its antidiabetic action were elucidated via in vitro and computational techniques.
In vitro study
Routine or excessive ingestion of carbohydrates can lead to postprandial hyperglycaemia. One remedial approach for diabetes is to curb postprandial blood glucose levels, by inhibiting the enzymatic activities of α-amylases and α-glucosidases. This suppresses carbohydrate digestion, thus delaying the absorption of glucose [Citation60,Citation61]. As represented in , betulinic acid inhibited α-amylase activity at increasing concentrations with an IC50 of 150.12 ± 2.46 μM as compared to acarbose which inhibited at a lower IC50 of 49.53 ± 0.23 μM. α-Glucosidase activity was also significantly (p < 0.05) inhibited by betulinic acid in a dose-dependent trend when compared to acarbose () with IC50 values of 19.45 ± 0.74 μM and 12.32 ± 0.67 μM, respectively (). This portrays the potential of betulinic acid to suppress elevated blood glucose, thus portraying its antidiabetic properties. This is consistent with inhibitory effect of betulinic acid on α-amylase and α-glucosidase enzymes recently reported by De Silva et al. [Citation62] as inhibition of these enzymes is recognized as one of the mechanisms by which the terpene elicits its anti-diabetic activities.
Figure 2. (a) α-amylase and (b) α-glucosidase inhibitory activities of betulinic acid. Data = mean ± SD; n = 3. abValues with different letters above the bars for a given concentration are significantly (p < 0.05) different from each other.
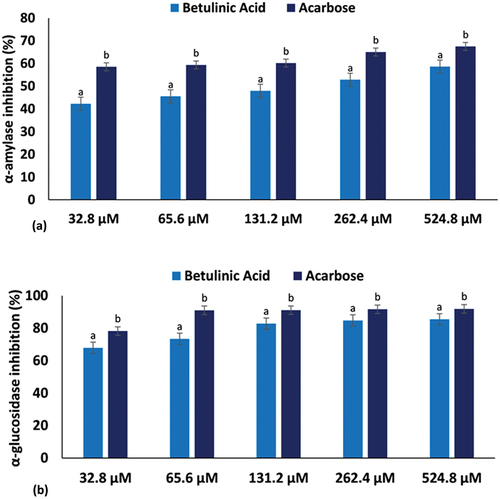
Table 1. IC50 values of biological activities.
Abnormalities in the metabolism of lipids and proteins are typical of type 2 diabetes. Insulin resistance has been attributed to excessive lipolysis and increased plasma protease activity [Citation5,Citation7]. Inhibition of pancreatic lipase activity, an enzyme responsible for the hydrolysis of triacylglycerols to free fatty acids, is another therapeutic approach for T2D treatment [Citation61]. The use of antilipolytic and antiprotease agents have been reported to improve insulin sensitivity and glucose monitoring [Citation5,Citation7]. In the present study, lipase activity was inhibited significantly (p < 0.05) by betulinic acid and the standard drug, orlistat, as shown in with low IC50 values of 24.05 ± 0.68 μM and 12.38 ± 0.14 μM (), respectively. As well, the triterpene significantly (p < 0.05) inhibited α-chymotrypsin (34.98 ± 1.82 μM) and compared favourably with the activity of the standard protease, chymostatin (24.10 ± 0.91 μM) as indicated in . This further indicates the potential of betulinic acid to maintain glucose homoeostasis and improve diabetes.
DFT study
The optimized structure of betulinic acid obtained from geometry optimization is presented in , and the optimization energy is −1397.23 Hartree.
Electronic and chemical reactivity descriptors
The chemical properties of a molecule have been linked to the energies of the frontier molecular orbitals (FMOs) and other associated parameters [Citation63]. FMOs are the lowest unoccupied molecular orbital (LUMO) and the highest occupied molecular orbital (HOMO), while the energies associated with them are designated ELUMO and EHOMO, respectively. The FMOs are important electronic properties for interpreting the bioassay results of a molecule. In order words, they describe the manner and pattern in which the molecule interacts with other species in certain environment [Citation64,Citation65]. The ELUMO and EHOMO obtained from the time-dependent DFT (TD-DFT) calculation are presented in . Also, the parameters for chemical and electronic properties are presented, as obtained from the following equation previously reported [Citation66,Citation67].
Table 2. Electronic and reactivity descriptors.
From the denotations, ΔE is the band gap energy, a measure of the energy difference between LUMO and HOMO. I is the ionization energy, while A is the electron affinity. Meanwhile, LUMO and HOMO energies, respectively, represent the capacity of a molecule to accept and donate electrons, thus corresponding to the electron affinity and ionization potential, respectively [Citation52,Citation68].
Furthermore, the band gap measures electron conductivity, i.e. the flow of electron across the molecule from the HOMO to LUMO orbitals. The band gap determines the chemical stability, hardness (η) and softness (δ), and electronegativity (χ) of the molecule [Citation69]. The chemical potential (Cp) describes the escaping tendency of electrons and measures the chemical reactivity of the molecule in terms of the amount of energy required for a change to occur in the molecule [Citation70,Citation71].
The electrophilicity index, denoted as ω, describes the molecule’s stabilization energy when saturated by electrons approaching from the surroundings [Citation72]. In general, among the chemical descriptors, a juxtapose of the band gaps in gas phase and water gives an insignificant difference of 0.04 eV. This accounts for about the very close values obtained for the parameters of other descriptors as shown in , reflecting that the molecule will react the same way in both media. A similar contribution is obtained for the LUMO and HOMO maps (). Thus, this warrants further studies for its chemical and biological activity in other solvent media.
A significant difference was observed in the dipole moments, µD, with 2.97 D and 4.48 D in gas phase and water, respectively. This property describes the polarity of the molecules [Citation73], and as obtained here, shows that betulinic acid is polar and readily interacts with an aqueous solution probably by H-bonding and related intermolecular forces.
Molecular electronic potential surface
The molecular electrostatic potential (MEP) surface describes the charge distribution across the molecule [Citation73]. Furthermore, it helps in understanding the drug–receptor interactions and biological recognition processes in a molecule [Citation74]. The blue region indicates positive electrostatic potential, and it has electrophilic reactivity while the red region is for negative potential and it is prone to nucleophilic attack. As indicated in , the hydroxyl H atom of the carboxylic acid moiety is prone to electrophilic attack, while the O atoms of hydroxyl group joined to terminal cyclohexane are prone to nucleophilic attack.
Molecular dynamics
Structural stability, flexibility and solvent accessible surface area analysis of betulinic acid against pancreatic-lipase, α-amylase, α-chymotrypsin and α-glucosidase
This study used molecular dynamics to explore the inhibitory activity and interactions of betulinic acid with its enzyme targets, pancreatic-lipase, α-amylase, α-chymotrypsin and α-glucosidase. The stability and flexibility of the bound enzyme complexes were computed from the root mean square deviation (RMSD), root mean square fluctuation (RMSF) and solvent accessible surface area analysis (SASA). The tracing disrupted movements and preventing artefacts that could appear during the simulation need the stability of a system to be validated. Therefore, we evaluated the stability of betulinic acid inside the active sites of the targeted enzymes, because this might affect the interaction dynamics course of betulinic acid inhibitor [Citation75,Citation76]. The orientation that the ligand displays within a specific binding site may have an impact on ligand stability [Citation77–79]. The therapeutic impact of a small molecule depends on its stability in a target protein’s binding region [Citation80]. The root mean square deviation measures the difference between a protein’s backbones from its initial structural conformation to its final position. However, the residual conformational analysis is a measure of the nature of fluctuation exhibited by individual residue corresponding to the effect of ligand induction on the protein cumulatively granting its therapeutic efficacy. For four systems, RMSF was calculated for each amino acid residue during MD simulation of 200 ns. Usually, an elevated RMSF value is associated with high fluctuations in the backbone structure, resulting in a more flexible structure [Citation37]. Conversely, a reduced RMSF value indicates a less flexible structure. The displacement and re-orientation of the surface protein residues in the four systems were explored by estimating the solvent-accessible surface area (SASA) further to obtain the differential conformational changes of the investigated systems. Protein folding is critical in the functional characteristic of the biomolecule and is driven by the core hydrophobic amino acids at the pocket site. The degree of interaction of amino acids with the solvent and interior of the pocket is proportional to the surface area exposed to the surrounding. According to this analysis, the average betulinic acid inhibitors RMSD, RMSF and SASA within pancreatic-lipase, α-Amylase, α-Chymotrypsin and α-Glucosidase enzymes are presented in .
Table 3. RMSD, RMSF and SASA profile of betulinic acid bound to lipase, α-amylase, α-chymotrypsin and α-glucosidase.
Additionally, a structural visualization using simulation RMSD, RMSF and SASA post-analyses betulinic acid complexes inside the binding site of the selected enzymes is shown in , respectively.
Figure 7. Structural representation of alterations occurring during the binding of betulinic acid with lipase (black), amylase (red), chymotrypsin (green) and glucosidase (purple), showing the conformational stability, C-α atoms RMSD for targeted enzymes to betulinic acid bound.
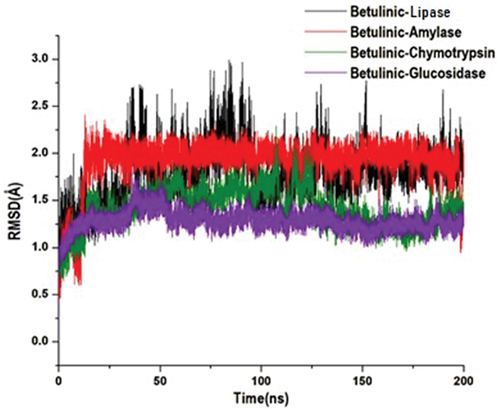
Free binding energy calculations of betulinic acid inhibitor with lipase, α-amylase, α-chymotrypsin and α-glucosidase enzymes
The therapeutic effects elicited by a drug strongly rely on a protein’s binding site and the activities that occur when binding ensues. To determine the inhibitory potential of betulinic acid inhibitor against the lipase, α-amylase, α-chymotrypsin and α-glucosidase enzymes, the binding free energy was evaluated by employing the Molecular Mechanics/Generalized Born Surface Area (MM/GBSA) method. From the results obtained, it was found that betulinic acid had bound to α-chymotrypsin enzyme favourably, with ∆G values measuring −28.40 kcal/mol, −23.67 kcal/mol, −23.33 kcal/mol and −15.88 kcal/mol for α-Glucosidase, α-Amylase and lipase, respectively (). These highest binding energies were calculated after 200 ns simulation time. Moreover, the promising ∆G values could indicate analogous modes of binding that possibly underlie the ability of betulinic acid to bind concurrently to these enzymes.
Table 4. MM/GBSA-based binding free energy profile of betulinic acid bound to lipase, α-amylase, α-chymotrypsin and α-glucosidase.
Perresidue interaction analyses
Decompositions of the binding-free energy have been shown to present a useful insight and significant annotation of the trajectories produced by MD simulations of protein-ligand complexes. We decomposed the overall binding energy into per-residue energy contributions of individual amino acid residues in the active site to give further insight into the differences between pancreatic lipase, α-amylase, α-chymotrypsin and α-glucosidase enzyme inhibition by the betulinic acid enzyme inhibition modes (). Interactions between electropositive and electronegative residues within the active site play a crucial role in enhancing ligand binding and stabilization at the target site. These interactions give rise to strong intermolecular forces, thereby increasing the binding affinity of the ligand for the active site. Additionally, the interactions between ligands and specific residues are essential for identifying key binding site residues and understanding their combined interactions with the drugs.
Figure 10. Per-residue decomposition plots showing individual energy contributions to the binding and stabilization of betulinic acid inhibitor at the binding sites of lipase (a), α-amylase (b), α-chymotrypsin (c) and α-glucosidase (d) enzymes. This reveals that energy contributions were highest in binding sites amino acid.
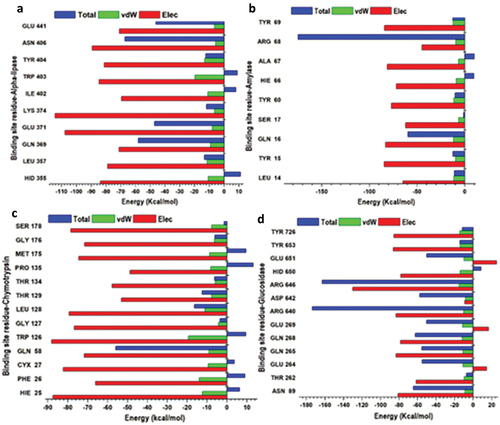
These analyses provided insights into the energy contributions of individual residues that interacted with the betulinic acid inhibitor at the active site pockets over the 200 ns MD simulation period, as depicted in .
DCCM analysis
Different enzyme dynamics between the three conditions were examined and plotted using a dynamic cross-correlation matrix for observing the correlated movements of all residues. DCM plots are represented by different colours, highly positive regions range from green to red (strongly correlated) and highly negative range from light blue to black (strongly anticorrelated) movement for specific residues, respectively. As evident in (), betulinic acid bound to lipase enzyme complex displayed higher correlated motions compared to the α-amylase, α-chymotrypsin and α-glucosidase enzyme complexes. These results are correlated with RMSF and RMSD, which justified that the region of the binding site exhibits a highly correlated motion during the simulation per time. Taken together, these results suggested that the conformation flexibility and stability of the entire enzyme were improved by the binding of betulinic acid.
Figure 11. Dynamic cross-correlation matrix analyses for Betulinic acid where (a), (b), (c) and (d) represent inhibitor binding mode at the binding site of lipase, α-amylase, α-chymotrypsin and α-glucosidase enzymes. Numbers closer to 1 indicate high correlation, while those closer to − 1 indicate anticorrelation between pairs of residues.
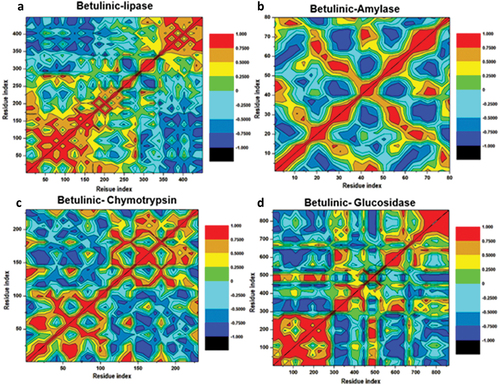
Conclusion
Results from the present study elucidate the potent antidiabetic properties of betulinic acid by exploring its conformational inhibitory mode of action on the digestive enzymes linked to diabetes including carbohydrate, protein and lipid hydrolysing enzymes. This is evident by its significant inhibitory effect on α-glucosidase, α-amylase, α-chymotrypsin and pancreatic lipase in vitro which was corroborated by the high binding energy and stable complexes with these enzymes as revealed by RMSD, RMSF, SASA and MM/GBA at 200 ns MD simulation. Further, in vivo experiments are recommended to bolster the results from this study.
Disclosure statement
No potential conflict of interest was reported by the author(s).
Additional information
Funding
References
- IDF, Diabetes Atlas, 10th ed., International Diabetes Federation. IDF, Brussels, Belgium, 2021.
- A.O. Ademiluyi and G. Oboh, Soybean phenolic-rich extracts inhibit key-enzymes linked to type 2 diabetes (α-amylase and α-glucosidase) and hypertension (angiotensin I converting enzyme) in vitro, Exp. Toxicol. Pathol. 65 (2013), pp. 305–309. doi:10.1016/j.etp.2011.09.005.
- Y. Wu, Y. Ding, Y. Tanaka, and W. Zhang, Risk factors contributing to type 2 diabetes and recent advances in the treatment and prevention, Int. J. Med. Sci. 11 (2014), pp. 1185. doi:10.7150/ijms.10001.
- V.F. Salau, O.L. Erukainure, and M.S. Islam, Phenolics: Therapeutic applications against oxidative injury in obesity and type 2 diabetes pathology, in Pathology, Victor R. Preedy ed., Academic Press (Elsevier Inc), 2020, pp. 297–307. doi:10.1016/B978-0-12-815972-9.00029-9.
- A.S. Al-Goblan, M.A. Al-Alfi, and M.Z. Khan, Mechanism linking diabetes mellitus and obesity, Diabetes Metab. Syndr. Obes. Targets Ther. 7 (2014), pp. 587–591. doi:10.2147/DMSO.S67400.
- D.R. Leitner, G. Frühbeck, V. Yumuk, K. Schindler, D. Micic, E. Woodward, and H. Toplak, Obesity and type 2 diabetes: Two diseases with a need for combined treatment strategies-EASO can lead the way, Obes. Facts 10 (2017), pp. 483–492. doi:10.1159/000480525.
- C.-S. Kuo, J.-S. Chen, L.-Y. Lin, G.W. Schmid-Schönbein, S. Chien, P.-H. Huang, J.-W. Chen, and S.-J. Lin, Inhibition of serine protease activity protects against high fat diet-induced inflammation and insulin resistance, Sci. Rep. 10 (2020), pp. 1725. doi:10.1038/s41598-020-58361-4.
- V.F. Salau, O.L. Erukainure, O.M. Ijomone, and M.S. Islam, Caffeic acid regulates glucose homeostasis and inhibits purinergic and cholinergic activities while abating oxidative stress and dyslipidaemia in fructose-streptozotocin-induced diabetic rats, J. Pharm. Pharm. 74 (2022), pp. 973–984. doi:10.1093/jpp/rgac021.
- S.M.I. Elbashir, H.P. Devkota, M. Wada, N. Kishimoto, M. Moriuchi, T. Shuto, S. Misumi, H. Kai, and T. Watanabe, Free radical scavenging, α-glucosidase inhibitory and lipase inhibitory activities of eighteen Sudanese medicinal plants, BMC Complement Altern Med. 18 (2018), pp. 1–12. doi:10.1186/s12906-018-2346-y.
- N. Ikarashi, R. Takeda, K. Ito, W. Ochiai, and K. Sugiyama, The inhibition of lipase and glucosidase activities by acacia polyphenol, Evid. Based Complement. Altern. Med. 2011 (2011), pp. 272075. doi:10.1093/ecam/neq043.
- Y. Cao and X.-M. Liu, Should we still be concerned about the potential side effects of glucagon-like peptide-1 receptor agonists on thyroid C cells?, Endocrine 48 (2015), pp. 47–52. doi:10.1007/s12020-014-0354-3.
- O.L. Erukainure, K.P. Otukile, K.R. Harejane, V.F. Salau, A. Aljoundi, C.I. Chukwuma, and M.G. Matsabisa, Computational insights into the antioxidant and antidiabetic mechanisms of cannabidiol: An in vitro and in silico study, Arab. J. Chem. 16 (2023), pp. 104842. doi:10.1016/j.arabjc.2023.104842.
- V.F. Salau, O.L. Erukainure, K.A. Olofinsan, N.Z. Msomi, O.K. Ijomone, and M.S. Islam, Ferulic acid mitigates diabetic cardiomyopathy via modulation of metabolic abnormalities in cardiac tissues of diabetic rats, Fund. Clin. Pharm. 37 (2023), pp. 44–59. doi:10.1111/fcp.12819.
- D.-W. Huang, S.-C. Shen, and J.S.-B. Wu, Effects of caffeic acid and cinnamic acid on glucose uptake in insulin-resistant mouse hepatocytes, J. Agric. Food Chem. 57 (2009), pp. 7687–7692. doi:10.1021/jf901376x.
- R. Ajala-Lawal, N. Aliyu, and T. Ajiboye, Betulinic acid improves insulin sensitivity, hyperglycemia, inflammation and oxidative stress in metabolic syndrome rats via PI3K/Akt pathways, Arch. Physiol. Biochem. 126 (2020), pp. 107–115. doi:10.1080/13813455.2018.1498901.
- P. Yogeeswari and D. Sriram, Betulinic acid and its derivatives: A review on their biological properties, Curr. Med. Chem. 12 (2005), pp. 657–666. doi:10.2174/0929867053202214.
- A. Hordyjewska, A. Ostapiuk, A. Horecka, and J. Kurzepa, Betulin and betulinic acid: Triterpenoids derivatives with a powerful biological potential, Phytochem. Rev. 18 (2019), pp. 929–951. doi:10.1007/s11101-019-09623-1.
- G. Bringmann, W. Saeb, L.A. Assi, G. Francois, A.S. Narayanan, K. Peters, and E.-M. Peters, Betulinic acid: Isolation from Triphyophyllum peltatum and Ancistrocladus heyneanus, antimalarial activity, and crystal structure of the benzyl ester, Planta Med. 63 (1997), pp. 255–257. doi:10.1055/s-2006-957666.
- G.A. Birgani, A. Ahangarpour, L. Khorsandi, and H.F. Moghaddam, Anti-diabetic effect of betulinic acid on streptozotocin-nicotinamide induced diabetic male mouse model, Braz. J. Pharm. Sci. 54 (2018), pp. e17171. doi:10.1590/s2175-97902018000217171.
- S. Chen, B. Lin, J. Gu, T. Yong, X. Gao, Y. Xie, C. Xiao, J.Y. Zhan, and Q. Wu, Binding interaction of betulinic acid to α-glucosidase and its alleviation on postprandial hyperglycemia, Molecules 27 (2022), pp. 2517. doi:10.3390/molecules27082517.
- J. Kim, Y.S. Lee, C.S. Kim, and J.S. Kim, Betulinic acid has an inhibitory effect on pancreatic lipase and induces adipocyte lipolysis, Phytother. Res. 26 (2012), pp. 1103–1106. doi:10.1002/ptr.3672.
- A.V. Sadybekov and V. Katritch, Computational approaches streamlining drug discovery, Nature 616 (2023), pp. 673–685. doi:10.1038/s41586-023-05905-z.
- F. Olawale, K. Olofinsan, O.M. Ogunyemi, K.O. Karigidi, G.A. Gyebi, I.M. Ibrahim, and O. Iwaloye, Deciphering the therapeutic role of Kigelia africana fruit in erectile dysfunction through metabolite profiling and molecular modelling, Inform. Med. Unlocked 37 (2023), pp. 101190. doi:10.1016/j.imu.2023.101190.
- J. Weddell and P. Imoukhuede, Computational systems biology for the VEGF family in angiogenesis, in Encyclopedia of Cardiovascular Research and Medicine, Sawyer, D. B., ed., Elsevier, Oxford, 2017, pp. 659–676. doi:10.1016/B978-0-12-809657-4.99548-6.
- Y.S. Kim, Y.M. Lee, H. Kim, J. Kim, D.S. Jang, J.H. Kim, and J.S. Kim, Anti-obesity effect of Morus bombycis root extract: Anti-lipase activity and lipolytic effect, J. Ethnopharmacol. 130 (2010), pp. 621–624. doi:10.1016/j.jep.2010.05.053.
- H. Saleem, I. Ahmad, M. Ashraf, M.S.A. Gill, M.F. Nadeem, M.N. Shahid, and K. Barkat, In vitro studies on anti-diabetic and anti-ulcer potentials of Jatropha gossypifolia (Euphorbiaceae), Trop. J. Pharm. Res. 15 (2016), pp. 121–125. doi:10.4314/tjpr.v15i1.17.
- R. Dennington, T.A. Keith, and J. Millam, GaussView, 6.0. 16, Semichem Inc, Shawnee Mission, KS, USA, 2016.
- Y. Zhao and D.G. Truhlar, The M06 suite of density functionals for main group thermochemistry, thermochemical kinetics, noncovalent interactions, excited states, and transition elements: Two new functionals and systematic testing of four M06-class functionals and 12 other functionals, Theor Chem. Acc. 120 (2008), pp. 215–241.
- M.-P. Egloff, F. Marguet, G. Buono, R. Verger, C. Cambillau, and H. van Tilbeurgh, The 2.46. ANG. resolution structure of the pancreatic lipase-colipase complex inhibited by a C11 alkyl phosphonate, Biochemistry 34 (1995), pp. 2751–2762. doi:10.1021/bi00009a003.
- L. Sim, K. Jayakanthan, S. Mohan, R. Nasi, B.D. Johnston, B.M. Pinto, and D.R. Rose, New glucosidase inhibitors from an ayurvedic herbal treatment for type 2 diabetes: Structures and inhibition of human intestinal maltase-glucoamylase with compounds from Salacia reticulata, Biochemistry 49 (2010), pp. 443–451. doi:10.1021/bi9016457.
- J.J. Birktoft and D. Blow, Structure of crystalline α-chymotrypsin: V. The atomic structure of tosyl-α-chymotrypsin at 2 Å resolution, J. Mol. Biol. 68 (1972), pp. 187–240. doi:10.1016/0022-2836(72)90210-0.
- J.W. Pflugrath, G. Wiegand, R. Huber, and L. Vértesy, Crystal structure determination, refinement and the molecular model of the α-amylase inhibitor hoe-467A, J. Mol. Biol. 189 (1986), pp. 383–386. doi:10.1016/0022-2836(86)90520-6.
- H.M. Berman, T. Battistuz, T.N. Bhat, W.F. Bluhm, P.E. Bourne, K. Burkhardt, Z. Feng, G.L. Gilliland, L. Iype, and S. Jain, The protein data bank, Acta Crystallogr. D 58 (2002), pp. 899–907. doi:10.1107/S0907444902003451.
- S. Kusumaningrum, E. Budianto, S. Kosela, W. Sumaryono, and F. Juniarti, The molecular docking of 1, 4-naphthoquinone derivatives as inhibitors of Polo-like kinase 1 using molegro virtual docker, J. Appl. Pharm. Sci. 4 (2014), pp. 047–053.
- R. Thomsen and M.H.M. Christensen, MolDock: A new technique for high-accuracy molecular docking, J. Med. Chem. 49 (2006), pp. 3315–3321. doi:10.1021/jm051197e.
- E.F. Pettersen, T.D. Goddard, C.C. Huang, G.S. Couch, D.M. Greenblatt, E.C. Meng, and T.E. Ferrin, UCSF chimera—a visualization system for exploratory research and analysis, J. Comput. Chem. 25 (2004), pp. 1605–1612. doi:10.1002/jcc.20084.
- E.Y. Salifu, A.R. Issahaku, C. Agoni, M.A. Ibrahim, N. Manimbulu, and M.E. Soliman, Prioritizing the catalytic gatekeepers through pan-inhibitory mechanism of entrectinib against ALK, ROS1 and TRKA tyrosine kinases, Cell Biochem. Biophys. 80 (2022), pp. 11–21. doi:10.1007/s12013-021-01052-2.
- J. Eberhardt, D. Santos-Martins, A.F. Tillack, and S. Forli, AutoDock Vina 1.2.0: New docking methods, expanded force field, and Python bindings, J. Chem. Inf. Model 61 (2021), pp. 3891–3898. doi:10.1021/acs.jcim.1c00203.
- O. Trott and A.J. Olson, AutoDock Vina: Improving the speed and accuracy of docking with a new scoring function, efficient optimization, and multithreading, J. Comput. Chem. 31 (2010), pp. 455–461. doi:10.1002/jcc.21334.
- B.-S.I. Case DA, S.R. Brozell, D.S. Cerutti, T.E. Cheatham III, V.W.D. Cruzeiro, T.A. Darden, R.E. Duke, D. Ghoreishi, M.K. Gilson, H. Gohlke, A.W. Goetz, D. Greene, R. Harris, N. Homeyer, S. Izadi, A. Kovalenko, T. Kurtzman, T.S. Lee, S. LeGrand, P. Li, C. Lin, J. Liu, T. Luchko, R. Luo, D.J. Mermelstein, K.M. Merz, Y. Miao, G. Monard, C. Nguyen, H. Nguyen, I. Omelyan, A. Onufriev, F. Pan, R. Qi, D.R. Roe, A. Roitberg, C. Sagui, S. Schott-Verdugo, J. Shen, C.L. Simmerling, J. Smith, R. Salomon-Ferrer, J. Swails, R.C. Walker, J. Wang, H. Wei, R.M. Wolf, X. Wu, L. Xiao, D.M. York, and P.A. Kollman, Amber 2018, University of Carlifornia, San Francisco 1, 2018.
- R. Salomon‐Ferrer, D.A. Case, and R.C. Walker, An overview of the amber biomolecular simulation package, Wiley Interdiscip. Rev. Comput. Mol. Sci. 3 (2013), pp. 198–210. doi:10.1002/wcms.1121.
- J.W. Ponder and D.A. Case, Force fields for protein simulations, Adv. Protein Chem. 66 (2003), pp. 27–85.
- J. Wang, R.M. Wolf, J.W. Caldwell, P.A. Kollman, and D.A. Case, Development and testing of a general amber force field, J. Comput. Chem. 25 (2004), pp. 1157–1174. doi:10.1002/jcc.20035.
- H.J. Berendsen, J.V. Postma, W.F. Van Gunsteren, A. DiNola, and J.R. Haak, Molecular dynamics with coupling to an external bath, J. Chem. Phys. 81 (1984), pp. 3684–3690. doi:10.1063/1.448118.
- M.D. Hanwell, D.E. Curtis, D.C. Lonie, T. Vandermeersch, E. Zurek, and G.R. Hutchison, Avogadro: An advanced semantic chemical editor, visualization, and analysis platform, J. Cheminf. 4 (2012), pp. 1–17. doi:10.1186/1758-2946-4-17.
- G.S. Grest and K. Kremer, Molecular dynamics simulation for polymers in the presence of a heat bath, Phys. Rev. A 33 (1986), pp. 3628. doi:10.1103/PhysRevA.33.3628.
- K.F. Omolabi, C. Agoni, F.A. Olotu, and M.E. Soliman, ‘Finding the needle in the haystack’-will natural products fit for purpose in the treatment of cryptosporidiosis?–A theoretical perspective, Mol. Simul. 47 (2021), pp. 636–649. doi:10.1080/08927022.2021.1895435.
- D.R. Roe and T.E. Cheatham III, PTRAJ and CPPTRAJ: Software for processing and analysis of molecular dynamics trajectory data, J. Chem. Theory Comput. 9 (2013), pp. 3084–3095. doi:10.1021/ct400341p.
- E. Seifert, OriginPro 9.1: Scientific data analysis and graphing software—Software review, J. Chem. Inf. Model. 54 (2014), pp. 1552. doi:10.1021/ci500161d.
- B. Hess, H. Bekker, H.J. Berendsen, and J.G. Fraaije, LINCS: A linear constraint solver for molecular simulations, J. Comput. Chem. 18 (1997), pp. 1463–1472. doi:10.1002/(SICI)1096-987X(199709)18:12<1463:AID-JCC4>3.0.CO;2-H.
- P.A. Kollman, I. Massova, C. Reyes, B. Kuhn, S. Huo, L. Chong, M. Lee, T. Lee, Y. Duan, and W. Wang, Calculating structures and free energies of complex molecules: Combining molecular mechanics and continuum models, Acc. Chem. Res. 33 (2000), pp. 889–897. doi:10.1021/ar000033j.
- S. Gunasekaran, R.A. Balaji, S. Kumeresan, G. Anand, and S. Srinivasan, Experimental and theoretical investigations of spectroscopic properties of N-acetyl-5-methoxytryptamine, Can. J. Anal. Sci. Spectrosc. 53 (2008), pp. 149–162.
- T. Hou, J. Wang, Y. Li, and W. Wang, Assessing the performance of the MM/PBSA and MM/GBSA methods. 1. The accuracy of binding free energy calculations based on molecular dynamics simulations, J. Chem. Inf. Model. 51 (2011), pp. 69–82. doi:10.1021/ci100275a.
- M. Ylilauri and O.T. Pentikäinen, MMGBSA as a tool to understand the binding affinities of filamin–peptide interactions, J. Chem. Inf. Model. 53 (2013), pp. 2626–2633. doi:10.1021/ci4002475.
- A. Onufriev, D. Bashford, and D.A. Case, Modification of the generalized born model suitable for macromolecules, J. Phys. Chem. B 104 (2000), pp. 3712–3720. doi:10.1021/jp994072s.
- N. Homeyer and H. Gohlke, Free energy calculations by the molecular mechanics Poisson− Boltzmann surface area method, Mol. Inf. 31 (2012), pp. 114–122. doi:10.1002/minf.201100135.
- I. Massova and P.A. Kollman, Combined molecular mechanical and continuum solvent approach (MM-PBSA/GBSA) to predict ligand binding, Perspect. Drug Disc. Design 18 (2000), pp. 113–135. doi:10.1023/A:1008763014207.
- D. Sitkoff, K.A. Sharp, and B. Honig, Accurate calculation of hydration free energies using macroscopic solvent models, J. Phys. Chem. 98 (1994), pp. 1978–1988. doi:10.1021/j100058a043.
- S. Oyedemi, T. Koekemoer, G. Bradley, M. van de Venter, and A. Afolayan, In vitro anti-hyperglycemia properties of the aqueous stem bark extract from Strychnos henningsii (Gilg), Int. J. Diabetes Develop. Ctries 33 (2013), pp. 120–127. doi:10.1007/s13410-013-0120-8.
- J. Nazaruk and M. Borzym-Kluczyk, The role of triterpenes in the management of diabetes mellitus and its complications, Phytochem. Rev. 14 (2015), pp. 675–690. doi:10.1007/s11101-014-9369-x.
- P. Inthongkaew, N. Chatsumpun, C. Supasuteekul, T. Kitisripanya, W. Putalun, K. Likhitwitayawuid, and B. Sritularak, α-glucosidase and pancreatic lipase inhibitory activities and glucose uptake stimulatory effect of phenolic compounds from, Dendrobium formosum, Rev. Bras. Farmacogn. 27 (2017), pp. 480–487. doi:10.1016/j.bjp.2017.05.005.
- D. De Silva, U. Senarath, and P.K. Perera, Molecular mechanisms of antidiabetic effect of betulinic acid in lotus rhizome, Bratisl. Lek. Listy 124 (2023), pp. 707–717. doi:10.4149/BLL_2023_108.
- B.Y.G. Mountessou, A.S.W. Mbobda, H.-G. Stammler, E.O. Akintemi, M.B. Mbah, G.M. Happi, S.F. Kouam, B.N. Lenta, N. Sewald, and T. Singh, Crystal structure, spectroscopic analysis, electronic properties and molecular docking study of costunolide for inhibitor capacity against Onchocerca volvulus main protease, J. Mol Struct. 1282 (2023), pp. 135185. doi:10.1016/j.molstruc.2023.135185.
- Y. Kaddouri, F. Abrigach, E.B. Yousfi, M. El Kodadi, and R. Touzani, New thiazole, pyridine and pyrazole derivatives as antioxidant candidates: Synthesis, DFT calculations and molecular docking study, Heliyon 6 (2020), pp. e03185. doi:10.1016/j.heliyon.2020.e03185.
- S. Sebastian, N. Sundaraganesan, B. Karthikeiyan, and V. Srinivasan, Quantum mechanical study of the structure and spectroscopic (FT-IR, FT-Raman, 13C, 1H and UV), first order hyperpolarizabilities, NBO and TD-DFT analysis of the 4-methyl-2-cyanobiphenyl, Spectrochim. Acta A Mol. Biomol. Spectrosc. 78 (2011), pp. 590–600. doi:10.1016/j.saa.2010.11.028.
- T.E. Olalekan, E.O. Akintemi, B. Van Brecht, and G.M. Watkins, Synthesis, characterization and DFT studies of Schiff bases of p-methoxysalicylaldehyde, Bull. Chem. Soc. Ethiop. 37 (2023), pp. 675–688. doi:10.4314/bcse.v37i3.11.
- M.D. Olawale, E.O. Akintemi, B.E. Agbaffa, and J.A. Obaleye, Synthesis, characterization, adsorption study, quantum mechanics, Monte carlo and molecular dynamics of lead based polymeric compound towards mopping of aqueous methyl red dye, Results Chem. 4 (2022), pp. 100499. doi:10.1016/j.rechem.2022.100499.
- C.S. Abraham, J.C. Prasana, and S. Muthu, Quantum mechanical, spectroscopic and docking studies of 2-amino-3-bromo-5-nitropyridine by density functional method, Spectrochim. Acta part A: Molec. Biomolec. Spectr. 181 (2017), pp. 153–163. doi:10.1016/j.saa.2017.03.045.
- B. Kosar and C. Albayrak, Spectroscopic investigations and quantum chemical computational study of (E)-4-methoxy-2-[(p-tolylimino) methyl] phenol, Spectrochim. Acta part A: Molec. Biomolec. Spectr. 78 (2011), pp. 160–167. doi:10.1016/j.saa.2010.09.016.
- E.O. Akintemi, K.K. Govender, and T. Singh, A DFT study of the chemical reactivity properties, spectroscopy and bioactivity scores of bioactive flavonols, Comput. Theor. Chem. 1210 (2022), pp. 113658. doi:10.1016/j.comptc.2022.113658.
- T.E. Bakare, E.O. Akintemi, S.A. Ogundare, and W.E. van Zyl, The Co3O4–Co3 (PO4) 2 nanocomposite supercapacitor system: Synthesis, electrochemistry, temperature effects, and computational studies, Solid State Sci. 131 (2022), pp. 106951. doi:10.1016/j.solidstatesciences.2022.106951.
- S.K. Nasiri, A. Reisi-Vanani, and M. Hamadanian, Molecular structure, spectroscopic, local and global reactivity descriptors and NBO analysis of C32H12: A new buckybowl and sub-fullerene structure, Polycycl. Aromat. Compd. 40 (2018), pp. 693–704. doi:10.1080/10406638.2018.1472108.
- T.L. Yusuf, E.O. Akintemi, S. Olagboye, and G.F. Tolufashe, Investigating the biological actions of some Schiff bases using density functional theory study, Phys. Sci. Rev. 8 (2021), pp. 535–547. doi:10.1515/psr-2019-0131.
- E. Huo, S. Shahab, S. Al Saud, W. Cheng, P. Lu, M. Sheikhi, R. Alnajjar, and S. Kaviani, Quantum chemical modeling, synthesis, spectroscopic (FT-IR, excited states, UV–vis) studies, FMO, QTAIM, NBO and NLO analyses of two new azo derivatives, J. Mol. Struct. 1243 (2021), pp. 130810. doi:10.1016/j.molstruc.2021.130810.
- J. Mukherjee and M.N. Gupta, Increasing importance of protein flexibility in designing biocatalytic processes, Biotechnol. Rep, 6 (2015), pp. 119–123. doi:10.1016/j.btre.2015.04.001.
- Y. Xie, J. An, G. Yang, G. Wu, Y. Zhang, L. Cui, and Y. Feng, Enhanced enzyme kinetic stability by increasing rigidity within the active site, J. Biol. Chem. 289 (2014), pp. 7994–8006. doi:10.1074/jbc.M113.536045.
- M.S. Celej, G.G. Montich, and G.D. Fidelio, Protein stability induced by ligand binding correlates with changes in protein flexibility, Protein Sci. 12 (2003), pp. 1496–1506. doi:10.1110/ps.0240003.
- K. Liu, E. Watanabe, and H. Kokubo, Exploring the stability of ligand binding modes to proteins by molecular dynamics simulations, J. Comput. Aided Mol. Des. 31 (2017), pp. 201–211. doi:10.1007/s10822-016-0005-2.
- C. Agoni, E.Y. Salifu, G. Munsamy, F.A. Olotu, and M. Soliman, CF 3 -pyridinyl substitution on antimalarial therapeutics: Probing differential ligand binding and dynamical inhibitory effects of a novel triazolopyrimidine-based inhibitor on Plasmodium falciparum dihydroorotate dehydrogenase, Chem. Biodivers. 16 (2019), pp. e1900365. doi:10.1002/cbdv.201900365.
- I. Luque and E. Freire, Structural stability of binding sites: Consequences for binding affinity and allosteric effects, Proteins Struct. Funct. Bioinf. 41 (2000), pp. 63–71.