Abstract
This article deals with the experimental design and with the solution of an inverse parameter estimation problem, for the identification of the three thermal conductivity components of an orthotropic solid. The experimental setup examined here involves the heating of a specimen in the form of a parallelepiped, through part of one of its surfaces. Different experimental variables are chosen by using the D-optimum criterion, including the number and location of sensors and the size of the heating surface. The unknown parameters are estimated with the Levenberg-Marquardt method of minimization of the least-squares norm.
Introduction
Due to the importance of nonisotropic materials in nowadays engineering, such as composites, a lot of attention has been devoted in the recent past for the identification of their thermal properties through inverse analysis techniques of parameter estimation Citation[1–Citation13].
The choice of various optimal experimental variables, including the number and locations of sensors, as well as the heating and final times, for the model used by Sawaf and Özisik Citation[1] for the estimation of thermal conductivity components of orthotropic solids, was addressed by Mejias et al. Citation[8]. In these two works Citation[1,Citation8], the solid was considered in the form of a cube, with three of its surfaces heated by fluxes of equal magnitude, while the other three surfaces were assumed as insulated. Later, Mejias et al. Citation[10,Citation11] have examined in the experimental design the choice of the boundary conditions for the nonheated surfaces of the cube, as well as the choice of the magnitudes of the applied heat fluxes and of the body dimensions. It was found Citation[10] that the use of constant temperature boundary conditions, instead of insulated boundary conditions, could result in more accurate estimates for the unknown parameters. It was also found Citation[11] that the body dimensions and the magnitudes of the applied heat fluxes should be chosen in accordance with a transformation that maps the orthotropic solid into an isotropic solid Citation[14]. In this case, the three thermal conductivity components could be estimated with identical relative accuracies. Despite such an important feature for the experimental design, the implementation of the experimental setup devised was not simple, because it involved the uniform heating of three surfaces of the solid and the magnitudes of the applied heat fluxes needed to be known for the inverse analysis.
In order to overcome such difficulties, we examine in this article an alternative experimental setup for the estimation of the three thermal conductivity components of orthotropic solids. In such a setup, only one of the surfaces of the solid would be partially heated. It would consist of a heater placed between two identical specimens of the material with unknown thermal conductivity components, thus allowing for better control of the magnitude of the applied heat flux, as well as of its distribution. We note that two-dimensional versions of this setup were examined in references Citation[3,Citation12,Citation13]. The D-optimum criterion Citation[15–Citation18] is used here for the experimental design, involving the choice of different variables, like the number and location of sensors and the size of the heating surface. The Levenberg-Marquardt Method of minimization of the least-squares norm Citation[15,Citation19–Citation21] is applied as the estimation procedure by using simulated measurements with random errors, as described below.
Direct problem
The physical problem considered in this work consists of a parallelepiped with dimensions a*, b* and c*, in the x*, y* and z* directions, respectively, as depicted in . The solid is initially at the uniform temperature (---501----1) . For times t* > 0, the boundary at z* = 0 is partially heated though the surface defined by 0<x* <
(---501----2) and 0<y* <
(---501----3) , while the remaining surface of this boundary is supposed to be insulated. The boundary at z* = c* is kept at a constant and uniform temperature
(---501----4) and the other four boundaries are insulated. The solid is assumed to be orthotropic, with thermal conductivity components
(---501----5) and
(---501----6) in the x*, y* and z* directions, respectively, and the physical properties are assumed constant. The mathematical formulation of such physical problem is given in dimensionless form as
(---501--1-)
(---501--1--)
(---501--1--)
(---501--1-)
(---501--1-)
(---501--1-)
The heat flux supplied at the surface z = 0 is given by
(---501--2)
where
(---501--3)
In EquationEq. (3)
(---501--3) , th is the dimensionless heating time.
The following dimensionless variables were defined in order to write the physical problem in dimensionless form:
(---501--4--)
(---501--4--)
(---501--4--)
(---501--4--)
In EquationEqs. (4a–o)(---501--4--) ,
(---501----7) and l* are characteristic values for thermal conductivity, heat flux and length, respectively. The superscript “*” appearing above denotes dimensional quantities, while ρ and cp are the density and specific heat of the body, respectively.
In the direct problem associated with the physical problem described above, the three thermal conductivity components kx, ky and kz, as well as the solid geometry, initial and boundary conditions, are known. The objective of the direct problem is to determine the transient temperature field T(x, y, z, t) in the body. The solution of the direct problem was obtained analytically by using the Classical Integral Transform Technique, with a domain transformation that maps the heat conduction equation for the orthotropic medium into the equation for an isotropic medium Citation[14]. The analytical solutions for the heating and nonheating periods are given respectively by
Heating Period (0 < t≤ th):
(---501--5-)
Nonheating Period (t > th):
(---501--5-) where
(---501--6--)
(---501--6--) and the eigenvalues are given by:
(---501--7--)
Inverse problem
For the inverse problem considered here, the thermal conductivity components kx, ky and kz are regarded as unknown, while the other quantities appearing in the formulation of the direct problem described above are assumed to be known with a high degree of accuracy.
For the estimation of the vector of unknown parameters PT = [kx, ky, kz], we assume available the transient readings of M temperature sensors. The temperature measurements may contain random errors. Such errors are assumed to be additive, uncorrelated and normally distributed, with zero mean and a known constant standard-deviation σ. Also, for the estimation procedure we consider that no previous information regarding the parameter values is available. Therefore, the solution of the present parameter estimation problem can be obtained through the minimization of the ordinary least-squares norm, which is a minimum variance estimator in this case Citation[15]. The ordinary least-squares norm is given by
(---501--8)
where
(---501--9-)
and each element
(---501----8) is a row vector of length equal to the number of sensors M, that is,
(---501--9-)
We note that Yim and Tim(P) are the measured and estimated temperatures, respectively, for time ti, i = 1, … , I, and for the sensor m, m = 1, … , M. The estimated temperatures are obtained from the solution of the direct problem by using the current available estimate for the vector of unknown parameters PT = [kx, ky, kz].
For the minimization of the least-squares norm Equation(5)(---501--5-) , we apply the Levenberg-Marquardt method Citation[15,Citation19–Citation21]. The iterative procedure of such a method is given by:
(---501--10)
where μk is a positive scalar named as damping parameter and Ωk is a diagonal matrix.
The sensitivity matrix, Jk, is defined by Citation[15]
(---501--11-)
where
(---501--11-)
The purpose of the matrix term μkΩk in EquationEq. (10)(---501--10) is to damp oscillations and instabilities due to the ill-conditioned character of the problem, by making its components large as compared to those of JTJ, if necessary Citation[15]. The damping parameter is made large in the beginning of the iterations. With such an approach, the matrix JTJ is not required to be nonsingular in the beginning of iterations and the Levenberg-Marquardt method tends to the Steepest Descent Method, that is, a very small step is taken in the negative gradient direction. The parameter μk is then gradually reduced as the iteration procedure advances to the solution of the parameter estimation problem and then the Levenberg-Marquardt method tends to the Gauss Method Citation[15]. However, if the errors inherent to the measured data are amplified, generating instabilities on the solution as a result of the ill-conditioned character of the problem, the damping parameter is automatically increased. Such an automatic control of the damping parameter makes the Levenberg-Marquardt method a quite robust and stable estimation procedure, so that it does not require the use of the Discrepancy Principle in the stopping criterion to become stable, like the conjugate gradient method Citation[21].
Statistical analysis
By performing a statistical analysis it is possible to assess the accuracy of (---501----9) , j = 1, 2 and 3, which are the values estimated for the unknown parameters Pj, j = 1, 2 and 3. By taking into account the statistical hypotheses described above, the covariance matrix for the ordinary least-squares estimator is given by Citation[15]:
(---501--12)
where J is the sensitivity matrix and σ is the standard deviation of the measurement errors, which is assumed to be constant. We note that EquationEq. (12)
(---501--12) is exact for linear estimation problems and is approximately used for nonlinear parameter estimation problems.
The standard deviations for the estimated parameters can thus be obtained from the diagonal elements of V as
(---501--13)
where Vjj is the jth element in the diagonal of V.
Confidence intervals at the 99% confidence level for the estimated parameters can be obtained as Citation[15]:
(---501--14)
while the joint confidence region for the estimated parameters is given by Citation[15]:
(---501--15)
where
(---501----10) is the value of the chi-square distribution with 3 degrees of freedom for a given probability.
Design of optimum experiments
Optimum experiments can be designed by minimizing the hypervolume of the confidence region of the estimated parameters, in order to ensure minimum variance for the estimates. The minimization of the confidence region given by EquationEq. (15)(---501--15) can be obtained by maximizing the determinant of V−1, in the so-called D-optimum design Citation[15–Citation18]. Since the covariance matrix V is given by EquationEq. (12)
(---501--12) , we can then design optimum experiments by maximizing the determinant of the Fisher's Information Matrix, JTJ Citation[15–Citation18]. Therefore, optimal experimental variables are chosen based on the criterion.
(---501--16)
For cases involving a single sensor, each element Fr,s, r, s = 1, 2 and 3, of the matrix F ≡ JTJ is given by:
(---501--17)
where I is the number of measurements.
If we take into account constraints, such as a large but fixed number of transient measurements of M sensors and also the maximum temperature in the region, Tmax, we can choose to maximize the determinant of a normalized form of F, here denoted as FI Citation[15], the elements of which are given by:
(---501--18)
where tf is the duration of the experiment.
Results and discussions
For the results presented below we assume the solid with unknown thermal conductivity components in the form of a cube, so that the characteristic length used to obtain the dimensionless problem is taken as l* = a* = b* = c*. Also, the characteristic heat flux is taken as (---501----11) , so that q0 = 1 in EquationEq. (3)
(---501--3) .
We note that for nonlinear estimation problems, such as the one under scrutiny in this work, the analyses of the sensitivity coefficients and of the determinant of FI are not global, because these quantities are functions of the unknown parameters. Therefore, a priori estimated values for the parameters are required for the design of optimum experiments. For the test-cases examined in this article, we used the following dimensionless values for the thermal conductivity components in order to design the experiment: kx = 1, ky = 15 and kz = 15. Such values were chosen based on the work of Dowding et al. Citation[5] for the estimation of the thermal conductivity components of two-dimensional carbon–carbon composites. The dimensionless thermal conductivity kx = 1 implies that (---501----12) . For the design of optimum experiments, involving the maximization of the determinant of (JTJ), we took into account the constraints of a large and fixed number of measurements for each sensor, as well as the maximum temperature in the region, so that the matrix FI with elements given by EquationEq. (18)
(---501--18) was used in the analysis.
Design of optimum experiments
Before proceeding to the analysis of the transient variation of the determinant of the information matrix, let us examine the transient variation of the normalized sensitivity coefficients for different sensor positions. The sensor positions examined in this work are presented in . They include sensors located at the center of the lateral thermally insulated surfaces at y = 1 (sensor 1) and x = 1 (sensor 2), as well as sensors located on the surface in contact with the heater at z = 0 (sensors 3, 4 and 5).
Table I Sensor locations
The normalized sensitivity coefficients were obtained by multiplying the original sensitivity coefficients by the parameters that they are referred to. The normalized sensitivity coefficients with respect to the parameters kx, ky and kz are defined respectively as
(---501--19--)
presents the normalized sensitivity coefficients for sensors 1–5, respectively, by assuming the size of the heating surface as a1 = b1 = 0.75. The heating time was supposed to be th = 1. shows a strong tendency toward linear dependence of the sensitivity coefficients for all sensor positions. Therefore, it is not possible to estimate the three unknown parameters with the readings of a single sensor. On the other hand, it may be possible to estimate the parameters with the readings of more than one sensor, because the constants of proportionality between the sensitivity coefficients are not the same, at the different sensor positions. Note in that the sensitivity coefficients tend to zero after the heating is stopped at t = 1. However, before becoming zero, the sensitivity coefficient with respect to kz undergoes a small increase for sensor 2, as depicted in . It can be noticed in that, generally, the sensitivity coefficient with respect to kz has the largest magnitude among the sensitivity coefficients, for any sensor position. The magnitude of Fz is larger for sensors 3, 4 and 5, located at z = 0. However, note in that the magnitude of Fz decreases when the sensor is located towards the edge of the heating surface. The sensitivity coefficient Fz is negative for sensors 3, 4 and 5, because an increase in the thermal conductivity component kz tends to decrease the temperature over the heating surface. also show that the sensitivity coefficients with respect to kx and ky are negative for sensors 3 and 4, but positive for sensor 5. Such is the case because an increase in the thermal conductivity components in the x and y directions tends to decrease the temperature of sensors 3 and 4 located over the heating surface, but tends to increase the temperature of sensor 5 located at the edge of the heating surface. The largest magnitude of Fx takes place for sensor 2, located at the surface x = 1. Similarly, the largest magnitude of Fy takes place for sensor 1, located at the surface y = 1, although the magnitude of Fy for sensor 3 is practically the same as for sensor 1.
Figure 2 Normalized sensitivity coefficients for: (a) sensor 1; (b) sensor 2; (c) sensor 3; (d) sensor 4; (e) sensor 5.
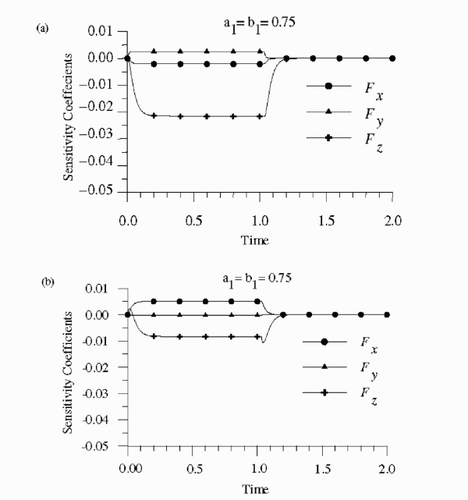
presents the transient variation of the determinant of the information matrix for different heating times, by assuming available the measurements of four different sensor configurations, which are presented in . Configuration 1 consists of the sensors located at the insulated surfaces, sensor 1 at y = 1 and sensor 2 at x = 1, which have the largest magnitudes for Fy and Fx, respectively. Configurations 2–4 were composed by sensors 1, 2 and of one of the sensors located at the boundary z = 0, i.e., sensor 3 for configuration 2, sensor 4 for configuration 3 and sensor 5 for configuration 4. Sensors 3, 4 and 5 have the largest magnitudes for Fz. The results shown in were obtained for a1 = b1 = 0.75.
Figure 3 Determinant of the information matrix for the: (a) sensor configuration 1; (b) sensor configuration 2; (c) sensor configuration 3; (d) sensor configuration 4.
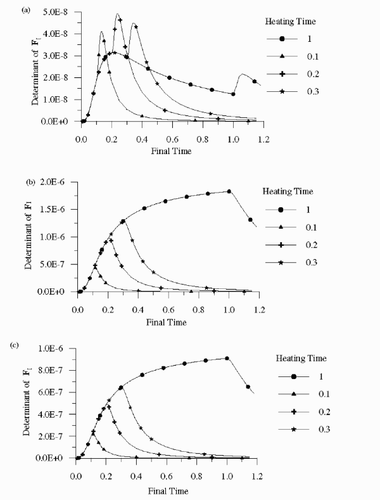
Table II Configurations for the sensors
shows that the determinant of the information matrix undergoes a sudden increase at the moment when the heating is stopped, for configuration 1. This is a result of the change of the shape of the sensitivity coefficient with respect to kz for sensor 2 when the heating is stopped, which undergoes a small increase before tending to zero (see ). For configuration 1, the optimum heating and final times are 0.2 and 0.24, respectively, when the determinant of the information matrix is maximum.
A comparison of shows that the magnitude of the determinant of the information matrix increases when a sensor at z = 0 is used in the analysis, in addition to sensors 1 and 2, with the exception for configuration 4 (see ). The configuration with the maximum determinant of the information matrix is configuration 2, because sensor 3 has the largest magnitude for the sensitivity coefficient with respect to kz (see ). As the sensitivity coefficient with respect to kz decreases, when the sensor at z = 0 is moved towards the edge of heating surface, the maximum determinant of the information matrix decreases and, for configuration 4, the effects of the use of measurements of a sensor at z = 0 are negligible. We note in that, for configurations 2 and 3, the determinant of the information matrix decreases at the moment when the heating is stopped. Such is the case because the sensitivity coefficients tend to zero very fast when the heating is stopped, with the exception of Fz for sensor 2, as depicted in . Therefore, for these configurations the effect of the sudden decrease in the sensitivity coefficients when the heating is stopped is more significant than the change in the shape of Fz for sensor 2. As a result, the heating time shall be chosen identical to the final time for configurations 2 and 3.
Configuration 2 is the best among those examined because of the maximum value obtained for the determinant of the information matrix. Hence, more accurate estimates are expected with configuration 2 than with the other configurations. For the results presented below obtained with such configuration we have used t = th = 1 because the determinant of the information matrix is practically constant for larger times.
Let us now examine the effects of the size of the heating surface on the maximum determinant of the information matrix. shows the maximum values of such determinant, obtained with configuration 2 for tf = th = 1 and for different sizes of the heating surface. shows that the optimum size of the heating surface is a1 = b1 = 0.75. For smaller sizes of such surface, the sensitivity coefficients decrease in magnitude and become more linearly-dependent, resulting in a decrease of the value of the determinant of the information matrix. For larger sizes of the heating surface the physical problem tends toward a one-dimensional problem, independent of the thermal conductivity components in the x and y directions, also resulting in a decrease of the values of the determinant of the information matrix. We note that the best sensor configuration was not changed for the different sizes of the heating surface.
Figure 4 Effects of the size of the heating surface on the determinant of the information matrix for tf = th = 1 and sensor configuration 2.
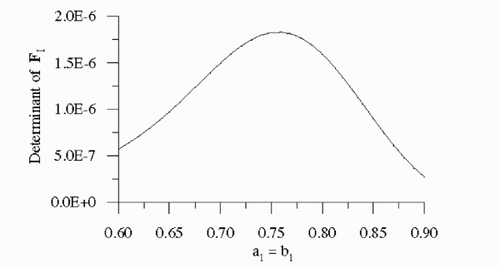
In order to address the sample orientation effects on the design of the experiment, we also examined an alternative arrangement of the thermal conductivity components along the three principal axes involving kx = 15, ky = 15 and kz = 1, instead of kx = 1, ky = 15 and kz = 15 as examined above. For such arrangement, the best configuration consisted of three sensors located at (1, 0.5, 0.5), (0.25, 0.25, 0) and (0.75, 0.75, 0), with a1 = b1 = 0.4 and tf = th = 0.05. However, the maximum determinant of the information matrix was about 10−10 for this alternative arrangement because of smaller magnitudes of the sensitivity coefficients.
Parameter estimation with simulated measurements
We now examine the estimation of the three thermal conductivity components of orthotropic solids, by using the Levenberg-Marquardt method of minimization of the least-squares norm. summarizes the different test-cases examined, as well as the results obtained for the estimated parameters and for the 99% confidence-interval widths. In , test-cases 1–3 correspond to the thermal conductivity arrangement 1, that is, kx = 1, ky = 15 and kz = 15, while test-case 4 correspond to arrangement 2, that is, kx = 15, ky = 15 and kz = 1. Test-cases 1–3 involve different initial-guesses for the unknown parameters for arrangement 1: for test-case 1, the initial guess was P0 = [0.5, 0.5, 0.5], for test-case 2 the initial guess was P0 = [1, 1, 1] and for test-case 3 the initial guess was P0 = [10, 10, 10]. For test-case 4 involving arrangement 2 the initial-guess was P0 = [0.5, 0.5, 0.5]. The results presented in were obtained with 100 transient simulated measurements per sensor, of three sensors arranged in accordance with the best configuration specified in the section above. The duration of the experiment and the heating time were also specified in accordance with experimental design performed above, that is, for test-cases 1–3 we used tf = th = 1 and for test-case 4 we used tf = th = 0.05. The results presented in were obtained with measurements containing random errors with standard-deviation σ = 0.01Ymax (where Ymax is the maximum measured temperature). In order to avoid biased results because of the random number generator used to compute the simulated measurements, the estimated parameters and the 99% confidence intervals presented in were averaged over 50 runs of the inverse problem solver, obtained with different sets of simulated measurements.
Table III Test-cases and estimated parameters
An analysis of the results obtained for test-cases 1–3, involving different initial-guesses for arrangement 1, shows that the estimation procedure is not sensitive to the initial-guesses used here. Also, the results for these test-cases show that more accurate estimates are obtained for kz than for ky. This is caused by the larger sensitivity coefficients with respect to kz than with respect to ky for this arrangement (kx = 1, ky = 15 and kz = 15), as illustrated by . The 99% joint rectangular confidence region, obtained by the multiplication of the 99% confidence interval widths of the three parameters, for arrangement 1 (test-cases 1–3) is 0.0006. The 99% joint rectangular confidence region for arrangement 2 (test-case 4) is 0.46. The larger confidence region for arrangement 2 is a result of the smaller determinant of the information matrix when compared to arrangement 1, as discussed above.
Conclusions
In this work we examined the estimation of the three thermal conductivity components of an orthotropic solid, by using one single heated surface. The D-optimum criterion was used for the design of the experiment. The optimum size of the heating surface, as well as the heating and final times, was obtained for different sensor configurations and for two different thermal conductivity arrangements. The inverse problem of parameter estimation was solved with the Levenberg-Marquardt method of minimization of the least-squares norm. The confidence regions of the estimated parameters were significantly reduced through the proper design of the experiment, so that accurate results were obtained by using in the inverse analysis simulated transient measurements containing random errors.
Nomenclature
Greeks
Superscripts
Subscripts
Acknowledgments
This work was partially funded by the CNPq grant 522.469/94-9 and by the FAPERJ grant E-26/170.344/2000.
Additional information
Notes on contributors
M.M. Mejias ‡
E-mail: [email protected]
E-mail: [email protected]
Notes
E-mail: [email protected]
E-mail: [email protected]
E-mail: [email protected]
- Sawaf, BW, and Özisik, MN, 1995. Determining the constant thermal conductivities of orthotropic materials, Int. Comm. Heat Mass Transfer 22 (1995), pp. 201–211.
- Sawaf, B, Özisik, MN, and Jarny, Y, 1995. An inverse analysis to estimate linearly temperature dependent thermal conductivity components and heat capacity of an orthotropic medium, Int. J. Heat Mass Transfer 38 (16) (1995), pp. 3005–3010.
- Taktak, R, 1992. Design and Validation of Optimal Experiments for Estimating Thermal Properties of Composite Materials. 1992, Ph.D. dissertation. Michigan State University.
- Taktak, R, Beck, JV, and Scott, EP, 1993. Optimal experimental design for estimating thermal properties of composite materials, Int. J. Heat Mass Transfer 36 (12) (1993), pp. 2977–2986.
- Dowding, KJ, Beck, JV, and Blackwell, B, 1996. Estimation of directional-dependent thermal properties in a carbon-carbon composite, Int. J. Heat Mass Transfer 39 (15) (1996), pp. 3157–3164.
- Dowding, KJ, and Blackwell, B, 1999. "Design of experiments to estimate temperature dependent thermal properties". In: 3rd Int. Conf. on Inverse Problems in Engineering: Theory and Practice. 1999. p. pp. 509–518, Port Ludlow, WA.
- Dowding, K, Beck, JV, Ulbrich, A, Blackwell, B, and Hayes, J, 1995. Estimation of thermal properties and surfaces heat flux in carbon-carbon composite, J. of Termophysics and Heat Transfer 9 (2) (1995), pp. 345–351.
- Mejias, MM, Orlande, HRB, and Özisik, MN, 1999. Design of optimum experiments for the estimation of the thermal conductivity components of orthotropic solids, Hybrid Methods in Engineering 1 (1) (1999), pp. 37–53.
- Mejias, MM, Orlande, HRB, and Özisik, MN, June 13–18 1999. "A Comparison of different parameter estimation techniques for the identification of the thermal conductivity components of orthotropic solids". In: 3rd Int. Conf. on Inverse Problems in Engineering: Theory and Practice. June 13–18 1999. p. pp. 325–332, Port Ludlow, WA.
- Mejias, MM, Orlande, HRB, and Özisik, MN, August 20–22, 2000. "On the choice of boundary conditions for the estimation of the thermal conductivity components of orthotropic solids". In: ASME National Heat Transfer Conference, Paper NHTC 2000 – 12062. August 20–22, 2000, Pittsburgh.
- Mejias, MM, Orlande, HRB, and Özisik, MN, 2003. Effects of the heating process and body dimensions on the estimation of the thermal conductivity components of orthotropic solids, Inv. Problems Engineering 11 (2003), pp. 75–89.
- Aville-Ramos, C, and Haji-Sheikh, A, 2001. Estimation of thermophysical properties of composites using multi-parameter estimation and Zeroth-order regularization, Inv. Problems Engineering 9 (2001), pp. 507–536.
- Kim, S, Jung, B, Kim, H, and Lee, W, 2003. Inverse estimation of thermophysical properties for anisotropic composite, Experimental Thermal and Fluid Science 27 (2003), pp. 697–704.
- Özisik, MN, 1993. Heat Conduction. New York. 1993, 2nd Edn..
- Beck, JV, and Arnold, KJ, 1977. Parameter Estimation in Engineering and Science, Wiley. New York. 1977.
- Artyukhin, E, 1985. Experimental design of measurement of the solution of coefficient-type inverse heat conduction problem, J. Eng. Phys. 48 (1985), pp. 372–376.
- Fadale, TD, Nenarokomov, AV, and Emery, AF, 1995. Two approaches to optimal sensor locations, ASME J. Heat Transfer 117 (1995), pp. 373–379.
- Emery, AF, and Fadale, TD, 1997. The effects of imprecisions in thermal sensor location and boundary conditions on optimal sensor location and experimental accuracy, ASME J. Heat Transfer 119 (1997), pp. 661–665.
- Levenberg, K, 1944. A Method for the solution of certain non-linear problems in least squares, Quart. Appl. Math. 2 (1944), pp. 164–168.
- Marquardt, DW, 1963. An algorithm for least squares estimation of nonlinear parameters, J. Soc. Ind. Appl. Math. 11 (1963), pp. 431–441.
- Özisik, MN, and Orlande, HRB, 2000. Inverse Heat Transfer: Fundamentals and Applications, Taylor & Francis. New York. 2000.