ABSTRACT
A public health approach to countering the threat from extremism aims to manage vulnerability before behaviour escalates to require involvement from the criminal justice system. Fundamental to applying a public health approach is understanding how risk (and protective) factors can be modified, in other words, the functional roles of these factors. To unpack the functional roles of risk factors, a more dynamic approach to modelling the complex relationships between factors is needed. In the present study we surveyed a representative sample of the UK general population (n = 1500) where participants self-reported risk factors and indicators for vulnerability to radicalisation. Operationalising analytical guidance from a Risk Analysis Framework (RAF), we applied psychometric network modelling to visualise the relationships among risk factors relating to individual-level propensities, situational influences, and exposure to extremism-enabling environments. We present our results as a series of network graphs and discuss (a) how risk factors ‘cluster’ or ‘co-occur’, (b) the most influential risk factors which may be important for intervention and prevention, and (c) ‘risk pathways’ which suggest potential putative risk and/or protective factors. We present our findings as evidence for a public health approach to countering the threat from extremism.
Introduction
Countering the threat from grievance-fuelled violence continues to evolve towards a public health approach. Examples include the UK’s PREVENT arm of the CONTEST strategy (Home Office, Citation2018), and the recent establishment of the US’ Centre for Prevention Programs and Partnerships (CP3) (Department of Homeland Security, Citation2021). Both are explicit in their aims to treat and manage vulnerability before behaviour escalates to the point where involvement from the criminal justice system is required. Vulnerability to radicalisation can be understood as susceptible people – individuals who demonstrate characteristics which make them more susceptible to radicalisation – who are at risk of exposure to extremism-enabling environments (Bouhana, Citation2019). Vulnerable people seen by up-stream services such as PREVENT or CP3 have not, and likely will not, commit a terrorist offence. In fact, many have not expressed a clear ideology (Baldet, Citation2020). Therefore, the goal is not to predict who will progress on to engage in violence, but rather to treat and manage underlying vulnerabilities, to primarily mitigate risk, but also to facilitate care and improve quality of life (Barry-Walsh et al., Citation2020) – this is the aim of a public health approach.
Bhui et al. (Citation2012) advocate for a public health approach to countering radicalisation, arguing that criminal enforcement alone has proven ineffective and interventions that target the most vulnerable are more effective. Bhui et al. (Citation2012) propose four steps: (1) comprehensively define the problem (2) establish risk and protective factors which can be modified through interventions, (3) establish ‘what works’ through designing, implementing, and evaluating interventions, and (4) implement effective interventions in a range of settings and evaluate their impact. Understanding how risk and protective factors drive vulnerability to radicalisation is therefore key to successfully implementing a public health approach.
Previous research provides evidence for a number of risk and protective factors which, to varying degrees, are validated empirically through studies either (a) describing prevalence rates (e.g. Gill et al., Citation2014a) (b) drawing comparisons between violent extremists and non-violent extremists (e.g. LaFree et al., Citation2018), (c) comparing factors across different types of violent extremists (e.g. Gruenewald et al., Citation2013) or (d) comparing violent extremists to the general population (e.g. Clemmow, Schumann et al., Citation2020). Several robust meta-analyses and systematic reviews synthesised the evidence finding empirical support for a lengthy list of risk and protective factors (see Desmarais et al., Citation2017; Gill et al., Citation2021; Hassan et al., Citation2018; Lösel et al., Citation2020; Wolfowicz et al., Citation2020; Trimbur et al., Citation2021).
This is a marked improvement from the field’s data sparsity only a decade ago (Monahan, Citation2012). However, to successfully implement a public health approach, practitioners require more than an understanding of a factor’s presence. They require an understanding of how a factor can be functionally relevant to vulnerability to radicalisation, as this provides evidence towards identifying risk and protective factors that can be modified. Recent empirical research designs moved some way toward establishing relevance by articulating the temporal relationships between risk factors using sequence analyses (Corner & Gill, Citation2019), generating configurations of risk factors and tying these to the theorised causal mechanisms using cluster analysis (Clemmow, Gill, et al., Citation2020; Clemmow, Bouhana, et al., Citation2019), and identifying mediating and moderating influencers (e.g. Rottweiler & Gill, Citation2020).
In the present study we build upon the growing body of empirical work and introduce psychometric network modelling to research on extremism, and crime more generally, as an analytical strategy capable of modelling complex problems. We employ the network framework to model vulnerability to radicalisation, as theorised by a previously designed Risk Analysis Framework (RAF; Bouhana, Citation2019), to visualise the mutually reinforcing interactions among risk factors as network graphs. In the following section we first introduce our theoretical framework which informs the study conceptualisation, before introducing our analytical strategy.
Analytical rationale
To guide analysis, we operationalise the previously designed RAF (see Bouhana, Citation2019). The RAF draws on Situational Action Theory (SAT) (Wikström, Citation2010) and opportunity theories to hypothesise multilevel mechanisms that underlie causal processes in pathways to radicalisation. The RAF conceives radicalisation as the outcome of the interaction between action-relevant individual propensities and terrorism-supportive criminogenic settings, whose features support a person’s perception of their own capability to offend (successfully), leading to the emergence of situations that trigger and sustain their motivation. Individuals differ in their susceptibility to environmental influences capable of inducing moral change (of which radicalisation is a special case). As a general, interactionist framework, the RAF is organised around these key mechanisms and processes, as opposed to discrete indicators, which are theorised to be subject to change and therefore unstable grounds for informing practice on their own (Clemmow, Gill, Bouhana et al., Citation2020; Corner & Gill, Citation2019).
The RAF articulates relations between causal factors and processes at multiple levels of analysis (individual, situational, social ecological, systemic), across each phase of an extremist event (radicalisation, attack planning and preparation, attack). In the present study, we limit the scope to consider interactions among the individual and situational levels of analysis, only, for two main reasons. First, modelling the relations among factors across each of the levels of the RAF would require a far larger sample size and a far longer survey than was feasible here. Future work will seek to model interactions across the social ecological and systemic levels to visualise the framework in its entirety. Second, practitioners are unlikely to have the capacity to exert much influence at a social ecological or systemic level. Hence, we apply the network framework to model vulnerability to radicalisation as conceptualised by the RAF as emerging from the interaction between individual-level propensities (propensity), situational influences (situation), and exposure to terrorism supportive people, places, and settings (exposure).
Analytical strategy
Psychometric network approaches are increasingly popular in the psychological sciences, specifically in psychopathology, and emerged as an alternative to the latent variable model. Rather than conceptualising mental disorders, such as depression, as the root cause of passive symptoms, disorders are considered systems of mutually reinforcing interactions among symptoms (Borsboom, Citation2008). We argue that vulnerability to radicalisation can be conceptualised in the same way; where there is no underlying root cause, but rather vulnerability emerges as the outcome of mutually reinforcing causal interactions among risk factors.
The psychometric network approach has been employed extensively to model complex, multidimensional constructs in research on psychopathology (Robinaugh et al., Citation2020), attitudes (Dalege et al., Citation2017), personality (Costantini et al., Citation2015), behaviour (De Beurs, Citation2017; Heino et al., Citation2019), and more. However, the use of psychometric network modelling to understand crime and violence has thus far been limited, with notable exceptions that demonstrate the potential of the approach (Bogaerts et al., Citation2020; McCuish et al., Citation2021). Of particular relevance to the present study, Bogaerts et al. (Citation2020) used longitudinal network modelling to understand changes in clinical risk and protective factors among 317 male forensic patients, across two time points. The results, visualised as network graphs, elicit novel insights about which risk and protective factors may be most influential, and therefore the most appropriate targets for intervention. This provides further evidence to suggest the network framework as an appropriate analytical strategy for the understanding how risk factors relate to vulnerability to radicalisation.
Some readers may be more aware of social network analysis (SNA). It has been employed extensively in research on extremists and criminals (for reviews see Gill et al., Citation2014b; Perliger & Pedahzur, Citation2011; Bichler & Malm, Citation2018). Although they share a common language, it is important to point out that psychometric network modelling departs from the more commonly used SNA in a number of ways. In SNA graphs, nodes represent people and edges represent their interconnections. In psychometric network graphs, nodes represent variables and edges define the nature of the statistical relationship among these. Most importantly, with SNA, edges between nodes are observed. An edge between node A and node B would indicate a real-world connection between person A and person B. In psychometric network modelling however, the edges are parameters estimated from data.
Metrics from network graph theory such as centrality measures, provide further insight into the structure of psychometric network graphs. For instance, in the present study we estimate node centrality to identify the most influential nodes (in this case risk factors) in the network. Identifying influential risk factors may highlight important mediators as well as potential targets for interventions to diminish their influence (or in other words suggest how to ‘dismantle the network’). It is also possible to detect sub-communities within a larger network which provide an insight into clusters of risk factors and how these relate to the larger structure. Another feature allows for the identifications of shortest paths, or pathways. For instance, in the present study, we describe pathways between the different communities of nodes, suggesting how propensity, situation, and exposure relate to one another.
Method
Sample
We surveyed a nationally representative UK sample. The sample was representative in terms of age, gender, and ethnicity. Participants were recruited via an online access panel, Prolific. Research has increasingly made use of online access panels such as Amazon’s Mechanical Turk (MTurk). These panels are online platforms where users receive payment for their participation in research. Recently, several alternatives have emerged, one being Prolific. Prolific differs from MTurk in that it was created for researchers to facilitate academic research. Therefore, it is explained to users that they will be participating in academic research upon registration (see Palan & Schitter, Citation2018 for a more detailed overview). Prolific maintains a pool of approved participants (approximately 150,000 persons) who register online to participate in academic studies in exchange for payment. Participants are vetted and quality controlled via a scoring and reporting feature. For example, a participant who clicks through a survey without reading the questions or fails several attention checks can be reported to Prolific or have their submission rejected. This will also affect their Prolific ‘score’ which is available to researchers upon review. In the present study, a user score did not factor into our decision to accept or reject their submission, rather this decision-making was informed by a series of attention-checks, as described below.
Prolific achieves a representative sample based on the most recent census information available. Invitations to participate in your study are distributed to registered users who satisfy the necessary quotas. If after 48 h, you remain waiting for a participant to satisfy a certain quota, Prolific may relax the sampling criteria. For instance, after 48 h, if you still require an Asian, female, aged 18–27 to participate in your study, Prolific will relax the constraints to invite any Asian female to complete your study. Prolific reports that the result is typically a sample that is approximately 98% representative.
To participate in the study participants were required to give informed consent. Participants were able to withdraw their consent at any point during the survey. In these instances, participant’s data were marked as ‘returned’ and they were excluded from data collection. Their place in the study was reallocated to another potential participant until the study quota was met. Seventy-three participants returned their submission and so their data were not collected. A further 40 participants failed to complete the study and their data were not released by Prolific.
Given the nature of the participant pool and to control for possible inattention, nine attention checks were included (Oppenheimer et al., Citation2009). Some evidence suggests that excluding participants solely based on a single attention check failure may result in bias (Berinsky et al., Citation2014). Hence, participants who failed an attention check were escalated to a manual review of their data. In review, we examined the length of time a participant spent completing the questionnaire, the pattern of their responses (i.e. for scale items, was the same answer selected for every question?) and whether they failed any other attention checks. Upon consideration of all these factors, a decision was made about whether to reject or accept a submission. Upon rejection, participants received a message detailing why their response was rejected and were invited to query it should they feel the rejection unfounded. Their place in the study was automatically reassigned to another suitable participant from the pool until the study quota had been met. The final sample size was 1500. Participants ranged from 18 to 87 years of age, with a mean age of 47.76 years (SD = 15.70). The sample included 773 (51.5%) females and 727 (48.5%) males. Table S1 in the supplementary materials details the sociodemographic descriptive statistics of the sample.
It is important here to consider the relevance of employing a representative general population sample. The general population is not comparable to violent extremists, and we make no claims that our results could inform how to predict who may or may not engage in actual violence. Instead, the focus of our paper is on vulnerability to radicalisation and informing an upstream preventative approach to mitigating risk by managing vulnerability. Therefore, employing an offender sample would not be appropriate given that those referred to preventative service are not offenders and much of the focus of a public health approach is diverting the vulnerable away from the criminal justice system. Vulnerability is present to varying degrees among the general population and employing a representative sample affords us greater generalisability. However, there are important limitations to consider regarding our sample, which we elaborate upon further in the discussion.
Measures
The full survey is hosted on the Open Science Framework (OSF) and is available here.Footnote1 Survey items were designed to measure the prevalence of risk factors and indicators for vulnerability to radicalisation among a general population. The development of the survey is described in further detail elsewhere (Clemmow et al., Citation2020). Measures were generated from a codebook used to collate a dataset of lone-actor terrorists (Gill et al., 2014) The codebook was drawn from an extensive review of the wider literature and included over 200 risk factors and indicators associated with radicalisation (see for a full list of all measures). The codebook displays substantial overlap with the factors identified in the systematic reviews and meta-analyses cited in the introduction.
Attitudinal items and psychological constructs were not self-reported here, given the inherent bias of doing so. Instead, these items were measured with pre-existing scale items. Thrill-seeking was measured with three items (‘I sometimes find it exciting to do things that could be dangerous’, ‘I often do things without thinking of the consequences’, ‘Sometimes I will take a risk just for the fun of it’). Impulsivity was measured with six items (‘I always say what I think, even if it is not nice or smart’, ‘If I want something, I do it immediately’, ‘I lose my temper easily’, ‘When I am really angry, other people better stay away from me’, ‘I often act on the spur of the moment without stopping to think’, ‘I like to test myself every now and then by doing something a little risky’). Both were measured along a 7-point Likert scale (Strongly disagree to Strongly agree). To create dichotomous variables (necessary for the present analytical strategy), Cronbach’s alpha was calculated for each of the scales (thrill-seeking = 0.806, impulsivity = 0.726) as well as an average score for each participant. Scores of >4 (somewhat agree, agree, strongly agree) were present and all other values were absent. We chose to be more lenient here (>4 versus >3) given the exploratory nature of the research. The results of our analysis will thus be best considered as hypothesis-generating, rather than confirmatory, and hence should be replicated.
Risk factors were mapped onto propensity, situation, and exposure based on analytical guidance from the RAF, as has been done previously (Clemmow, Gill, et al., Citation2020; Clemmow et al., Citation2019; Corner & Gill, Citation2019). Propensity was operationalised with 23 factors. Many of these risk factors are also associated with general crime/deviance/negative life outcomes in general. However, previous research finds considerable overlap between propensity and indeed situational factors among a range of different types of offenders including mass murderers (Clemmow, Gill et al., Citation2020; Gill et al., Citation2021; Horgan et al., Citation2016) , the pathologically fixated (Clemmow et al., Citation2021), lone-actor terrorists (Gill et al., 2014), and many more. When and for whom these propensities drive vulnerability to radicalisation specifically, is theorised by the RAF to be due to exposure, via selection effects. In other words, one can be highly susceptible to radicalisation, but to be vulnerable to radicalisation is to be susceptible to exposure. Differential susceptibility is theorised whereby someone highly susceptible may need very little exposure to become radicalised, whereas someone more resilient may require prolonged and sustained exposure to become radicalised. Hence many of the propensity factors operationalised here relate to a range of adverse outcomes. A key aspect to understanding the relevance of risk factors to vulnerability to radicalisation is how they relate to exposure.
Situation was operationalised with 19 factors. To measure situational indicators, rather than prevalence over a life course, respondents were asked to indicate which of these they had experienced ‘within the last year’. Exposure was disaggregated as active exposure and passive exposure based on previous research which conceptualised exposure to people, places, or materials supportive of extremism as active (e.g. self-initiated) or passive (e.g. inadvertent/accidental) (Pauwels et al., Citation2014; Pauwels & Schils, Citation2016). The purpose of doing so is to allow the model to consider the interactions among active and passive exposure to extremism-supportive people, places, and settings, independently. This is because inadvertent exposure to extremism is likely not driven by the same processes as self-motivated exposure to extremism (Pauwels et al., Citation2014), although the two are presumed highly associated. Data are available upon reasonable request for replication purposes.
Statistical analysis
To construct psychometric network graphs, parameters are estimated from the data and visualised as a weighted network between variables. The network structure is analysed using measures from network graph theory. Researchers familiar with social network analysis will be familiar with these. In the present study, we compute centrality measures (strength) to estimate node importance, implement a clustering algorithm (Walktrap) to identify communities of nodes, and compute shortest paths to identify pathways between the communities detected.
Network accuracy and centrality measures are more accurate and reliable as sample size increases. However, sample sizes afforded to researchers, particularly in criminology, are often relatively small (compared to analogous fields). Therefore, it is important to consider the accuracy and reliability of any network. To do so, we (a) estimate confidence intervals on the edge weights, (b) assess the stability of centrality indices, and (c) test for significant differences between edge-weights and centrality indices. The results of these tests provide important information about the reliability and replicability of our findings.
Network estimation
We used the R package IsingFit (Van Borkulo et al., Citation2015) to estimate the network graph (R code is available in the supplementary materials). The package computes an undirected network graph and applies a LASSO (Least Absolute Shrinkage and Selection Operator) regularisation. The LASSO penalty shrinks regression coefficients and sets very small coefficients to zero. The result is a network which is both parsimonious and optimises goodness of fit. This is one way that psychometric network modelling can handle many variables in relatively small sample sizes. In addition, the regularisation employs a tuning parameter. Values range from 0 to 1. If set to zero, the optimal tuning parameter is selected with ordinary BIC, otherwise it is selected with EBIC. In the present study the hyperparameter, gamma, was set to 0.25. A low gamma value may result in more spurious edges whereas a high gamma value will remove more edges. 0.25 is a reasonable value for an exploratory analysis. Further details can be found here (Hevey, Citation2018). Lastly, we implement the AND-rule. The AND-rule requires regression coefficients between two nodes to both be non-zero for a connecting edge to be drawn. The alternative, the OR-rule, is more lenient, where only one non-zero regression coefficient between two nodes is required for a connecting edge to be drawn. The edge weights are the mean of the coefficients between two nodes. An edge connecting two nodes can therefore be interpreted as a significant association, controlling for all other nodes in the network. Tutorial papers for implementing a psychometric network approach in R are widely available (Costantini et al., Citation2015; Epskamp et al., Citation2018).
The Walktrap clustering algorithm (Pons & Latapy, Citation2005) in the igraph package (Csardi & Nepusz, Citation2006) was used to detect communities, or clusters, within the network. The algorithm is a hierarchical clustering algorithm which constructs communities based on random walks. Short distance random walks are assigned to the same community. To compute random walks, start at a node, pick a random neighbour, and move to it, then repeat. The nodes you visit most often will be assigned to the same community. Communities were identified with igraph and plotted as network graphs using qgraph. There are a range of community detection algorithms available to researchers. Some are more effective than others dependent on things like the number of nodes or the sample size. The Walktrap algorithm was chosen here as it is largely effective at detecting communities among a small sample size (n = 1500). A full discussion of various community detection algorithms is available, here (Yang et al., Citation2016).
A series of further graphs illustrating the shortest paths to exposure were computed. The resultant network graphs differ from the overall graph in that they illustrate possible ‘routes’ to exposure, highlighting potential mediators between propensity and situation risk factors. Shortest paths are the minimum number of steps needed to go from one node to another.
All graphs were visualised with the qgraph package (Epskamp et al., Citation2012). We implemented the Fruchterman and Reingold (Citation1991) algorithm which places strongly connected nodes at the centre of the graph, closer together, and less connected nodes towards the periphery of the graph.
Centrality measures
Centrality measures are one way to compute node importance in a network. Three centrality measures from network graph theory are often computed in psychometric network graphs; strength, closeness, and betweenness. Strength quantifies how well a node is connected to other nodes in a network. It is the sum of the standardised weights of all significant edges in the network. Those more familiar with SNA my recognise strength as akin to degree centrality. Closeness quantifies a node’s proximity to all other nodes in the network. It is the sum of a node’s shortest paths. Betweenness measures the number of times a node is on the shortest path between other nodes. The reliability, and to some extent, interpretability, of closeness and betweenness in psychometric networks is somewhat debatable (Bringmann et al., Citation2019; Epskamp et al., Citation2018). However, node strength is considered a reasonably stable estimate of node importance. Therefore, the bootnet package (Epskamp et al., Citation2018) was used to estimate node strength, only.
Network stability
It is important to calculate and report the stability of networks and all centrality indices. These metrics can inform judgements about the reliability and accuracy of network graphs. We used the R package bootnet to investigate the stability of the networks. We bootstrapped 95% confidence intervals around the edge weights, estimated the correlation-stability coefficient for centrality metrics (ranging from 0 to 1; values above 0.25 imply moderate stability, above 0.5 strong stability), and computed the edge-weights difference test and the centrality difference test. These methods are described in detail elsewhere (Epskamp et al., Citation2018). Briefly, edge weight accuracy relates to the confidence with which you can interpret the order of the edge weights. Low accuracy, indicated by wide confidence intervals, would mean the order of the edge weights should be interpreted cautiously. Centrality stability also relates to the degree of confidence with which you can interpret the order of the centrality estimates. Low centrality stability means that interpreting the order of centrality measures should proceed with caution.
Results
Descriptive statistics
All item descriptive statistics are presented in .
Table 1. Descriptive statistics for all items.
Network graph
is the network graph for the full sample (n = 1500). Details of network accuracy and stability are provided in the supplementary material. All bootstrap tests were performed with 2000 samples. Figure S1 displays the bootstrapped difference test between non-zero edges. Results suggest that the order of the edge weights, or the ‘thicknesses’ of the edges connecting two nodes, can be interpreted reasonably reliably. Figure S2 displays the bootstrapped confidence intervals of estimate edge-weights for the estimated network. Similarly, results suggest the edges are reasonably accurate. displays node strength. Disrespected, psychological distress, and non-violent offending had the highest node strength, suggesting these nodes exert greater influence over the whole network. Figure S3 in the supplementary materials displays the average correlations between strength of networks sampled with persons dropped and the original sample. The correlation-stability coefficient was 0.439, suggesting that node strength can be interpreted with reasonable confidence.
Figure 1. Network analysis of risk factors and indicators associated with vulnerability to radicalisation. Communities identified with the Walktrap clustering algorithm.
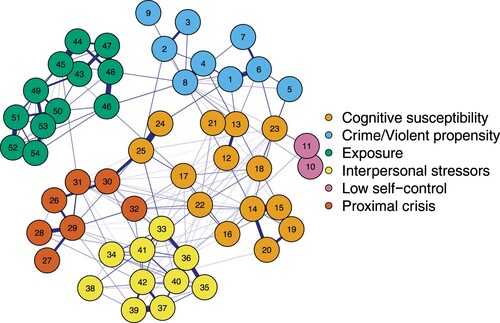
Figure 2. Node strength. Values displayed as raw z-scores. Nodes ordered from highest to lowest strength. Higher strength indicates greater overall importance to the network.
Crime/violent propensity [blue]: 1 – expelled from school, 2 – problematic drug use, 3 – problematic alcohol use, 4 – arrested as an adolescent, 5 – expressed a desire to hurt others, 6 – violent as a child/adolescent, 7 – committed a violent offence, 8 – committed a non-violent offense, 9 – perpetrator of domestic abuse.
Low self-control [pink]: 10 – thrill-seeking, 11 – impulsivity.
Cognitive susceptibility [orange]: 12 – victim of bullying as a child/adolescent, 13 – victim of violence as a child/adolescent, 14 – psychological distress, 15 – diagnosed mental disorder, 16 – difficulties coping with change, 17 – isolated, 18 – obsessive thinking, 19 – previous suicide attempt, 20 – history of self-harm, 21 – grew up in an abusive home, 22 – chronic stress, 23 – anger management problems, 24 – dropped out of school/university, 25 – worsening performance at work/school.
Proximal crisis (all indicators measured as ‘within the last year’) [red]: 26 – recent unemployment, 27 – death in the family, 28 – proximate life change, 29 – proximate crisis, 30 – work stress, 31 – interrupted in pursuing a life goal, 32 – financial problems.
Interpersonal stressors (all indicators measured as ‘within the last year’) [yellow]: 33 – experienced being degraded, 34 – experienced prejudice, 35 – had a promise broken, 36 – experienced being disrespected, 37 – ignored by someone important to them, 38 – harmed by the negligence of someone else, 39 – not cared for by someone important to them, 40 – victim of physical/verbal assault, 41 – felt like a helpless victim, 42 – problematic personal relationships.
Exposure: [green] 43 – known someone who has extremist views, 44 – witnessed a direct threat of extremist violence, 45 – witnessed verbal statements in support of violent extremism, 46 – received extremist propaganda online, 47 – known of extremism in their community, 48 – received propaganda offline, 49 – face-to-face interactions with extremists, 50 – has extremist friends, 51 – engaged with extremist propaganda, 52 – searched for extremist content online, 53 – chosen to spend time in places where there is extremism, 54 – interacted virtually with extremists.
![Figure 2. Node strength. Values displayed as raw z-scores. Nodes ordered from highest to lowest strength. Higher strength indicates greater overall importance to the network.Crime/violent propensity [blue]: 1 – expelled from school, 2 – problematic drug use, 3 – problematic alcohol use, 4 – arrested as an adolescent, 5 – expressed a desire to hurt others, 6 – violent as a child/adolescent, 7 – committed a violent offence, 8 – committed a non-violent offense, 9 – perpetrator of domestic abuse.Low self-control [pink]: 10 – thrill-seeking, 11 – impulsivity.Cognitive susceptibility [orange]: 12 – victim of bullying as a child/adolescent, 13 – victim of violence as a child/adolescent, 14 – psychological distress, 15 – diagnosed mental disorder, 16 – difficulties coping with change, 17 – isolated, 18 – obsessive thinking, 19 – previous suicide attempt, 20 – history of self-harm, 21 – grew up in an abusive home, 22 – chronic stress, 23 – anger management problems, 24 – dropped out of school/university, 25 – worsening performance at work/school.Proximal crisis (all indicators measured as ‘within the last year’) [red]: 26 – recent unemployment, 27 – death in the family, 28 – proximate life change, 29 – proximate crisis, 30 – work stress, 31 – interrupted in pursuing a life goal, 32 – financial problems.Interpersonal stressors (all indicators measured as ‘within the last year’) [yellow]: 33 – experienced being degraded, 34 – experienced prejudice, 35 – had a promise broken, 36 – experienced being disrespected, 37 – ignored by someone important to them, 38 – harmed by the negligence of someone else, 39 – not cared for by someone important to them, 40 – victim of physical/verbal assault, 41 – felt like a helpless victim, 42 – problematic personal relationships.Exposure: [green] 43 – known someone who has extremist views, 44 – witnessed a direct threat of extremist violence, 45 – witnessed verbal statements in support of violent extremism, 46 – received extremist propaganda online, 47 – known of extremism in their community, 48 – received propaganda offline, 49 – face-to-face interactions with extremists, 50 – has extremist friends, 51 – engaged with extremist propaganda, 52 – searched for extremist content online, 53 – chosen to spend time in places where there is extremism, 54 – interacted virtually with extremists.](/cms/asset/3998a5a0-6bfa-43aa-b160-be918eddbfeb/gpcl_a_2027944_f0002_ob.jpg)
The Walktrap clustering algorithm identified 6 communities, as can be seen in . Communities were labelled by researchers based on the presenting pattern of risk factors and indicators, and analytical guidance from the RAF. Specifically, we relate the identified communities to underlying causal mechanisms theorised by the RAF.
Community 1: cognitive susceptibility
Nodes relating to propensity disaggregated into two communities, labelled as cognitive susceptibility, and crime/violent propensity. Cognitive susceptibility includes nodes indicative of a pervasive, individual-level susceptibility including diagnosed mental disorder, psychological distress, adverse childhood experiences, chronic stress, and self-isolation. Two nodes theorised as situational factors are included here; dropped out of school/university and worsening performance at work or school.
Community 2: crime/violent propensity
Crime/violent propensity includes nodes related to criminality and/or violence. For example, perpetrating domestic abuse, a desire to hurt others, drug and alcohol problems, exclusion from school, and both violent and non-violent offending. Except for two situation nodes, all nodes previously theorised by research operationalising the RAF as relating to propensity were classified as such.
Community 3: interpersonal stressors
Interpersonal stressors is a community characterised by experiences of personal (being ignored, not cared for, problematic personal relationships) and social (prejudice, degraded, victimised) stressors.
Community 4: proximal crisis
Proximal crisis is characterised by significant, and to some extent, goal-oriented, interruptions or crises. For instance, losing employment, significant change in life circumstances, financial problems, and death in the family. This is similar to patterns of situational stressors disaggregated from a dataset of US lone-actor grievance-fuelled violence offenders (Clemmow, Schumann et al., Citation2020).
Community 5: low self-control
Low self-control includes thrill-seeking and impulsivity. We disaggregated low self-control to model two facets of low self-control independently, as previous research suggests that thrill-seeking and impulsivity can have independent effects on behaviour (Lynam & Miller, Citation2004). suggests that this may be the case here. Specifically, thrill-seeking appears associated with a crime/violent propensity, via non-violent offending, and impulsivity appears associated with situational stressors, via being disrespected. The RAF conceives of susceptibility as an interaction among pre-existing morality, executive functions, and capacity for self-control (Bouhana, Citation2019). This interaction can be seen in where self-control may be a potential mediator among differential propensities and situational influences.
Community 6: exposure
Active and passive exposure nodes were classified as a single community, labelled exposure. Whilst the drivers of ‘self-initiating’ versus ‘inadvertent’ exposure to extremism may theoretically differ, the conceptualisation of these items as a single community illustrates the high degree of association between unintended exposure, and self-motivated exposure to terrorism (and vice versa). It is of note that it may be theoretically important to consider the drivers of passive and active exposure separately. Here we simply present the results of a deductive analysis which identifies natural groupings from within the data.
Pathways to exposure
A psychometric network approach is unlike more traditional statistical analyses as there is not necessarily a dependent variable, as conceptualised in regression models for example. However, relations among or between certain communities of nodes may be of particular interest to researchers. In the present instance, we focus on exposure as the RAF theorises vulnerability to radicalisation as emerging from the interaction between susceptible people and extremism-supportive people, places, or settings. Previous research highlights the equifinality of pathways to radicalisation. A visual inspection of the numerous ‘routes’ between propensity, situation, and exposure () reiterates the need to conceptualise vulnerability to radicalisation as emerging from a dynamic, interactional system. Computing shortest paths detects the ‘quickest’ routes to exposure and can help identify potential mediators. The resultant graphs can be seen in .
Figure 3. Network analysis of risk factors and indicators associated with vulnerability to radicalisation. Shortest path from cognitive susceptibility to exposure highlighted.
Crime/violent propensity [blue]: 1 – expelled from school, 2 – problematic drug use, 3 – problematic alcohol use, 4 – arrested as an adolescent, 5 – expressed a desire to hurt others, 6 – violent as a child/adolescent, 7 – committed a violent offence, 8 – committed a non-violent offense, 9 – perpetrator of domestic abuse.
![Figure 3. Network analysis of risk factors and indicators associated with vulnerability to radicalisation. Shortest path from cognitive susceptibility to exposure highlighted.Crime/violent propensity [blue]: 1 – expelled from school, 2 – problematic drug use, 3 – problematic alcohol use, 4 – arrested as an adolescent, 5 – expressed a desire to hurt others, 6 – violent as a child/adolescent, 7 – committed a violent offence, 8 – committed a non-violent offense, 9 – perpetrator of domestic abuse.](/cms/asset/fd32ceb6-8036-4b34-9dc6-5eacdb8e1bfe/gpcl_a_2027944_f0003_oc.jpg)
Figure 4. Network analysis of risk factors and indicators associated with vulnerability to radicalisation. Shortest path from crime and/or violence supportive morality to exposure highlighted.
Crime/violent propensity [blue]: 1 – expelled from school, 2 – problematic drug use, 3 – problematic alcohol use, 4 – arrested as an adolescent, 5 – expressed a desire to hurt others, 6 – violent as a child/adolescent, 7 – committed a violent offence, 8 – committed a non-violent offense, 9 – perpetrator of domestic abuse.
Low self-control [pink]: 10 – thrill-seeking, 11 – impulsivity.
Cognitive susceptibility [orange]: 12 – victim of bullying as a child/adolescent, 13 – victim of violence as a child/adolescent, 14 – psychological distress, 15 – diagnosed mental disorder, 16 – difficulties coping with change, 17 – isolated, 18 – obsessive thinking, 19 – previous suicide attempt, 20 – history of self-harm, 21 – grew up in an abusive home, 22 – chronic stress, 23 – anger management problems, 24 – dropped out of school/university, 25 – worsening performance at work/school.
Proximal crisis (all indicators measured as ‘within the last year’) [red]: 26 – recent unemployment, 27 – death in the family, 28 – proximate life change, 29 – proximate crisis, 30 – work stress, 31 – interrupted in pursuing a life goal, 32 – financial problems.
Interpersonal stressors (all indicators measured as ‘within the last year’) [yellow]: 33 – experienced being degraded, 34 – experienced prejudice, 35 – had a promise broken, 36 – experienced being disrespected, 37 – ignored by someone important to them, 38 – harmed by the negligence of someone else, 39 – not cared for by someone important to them, 40 – victim of physical/verbal assault, 41 – felt like a helpless victim, 42 – problematic personal relationships.
Exposure[green] : 43 – known someone who has extremist views, 44 – witnessed a direct threat of extremist violence, 45 – witnessed verbal statements in support of violent extremism, 46 – received extremist propaganda online, 47 – known of extremism in their community, 48 – received propaganda offline, 49 – face-to-face interactions with extremists, 50 – has extremist friends, 51 – engaged with extremist propaganda, 52 – searched for extremist content online, 53 – chosen to spend time in places where there is extremism, 54 – interacted virtually with extremists.
![Figure 4. Network analysis of risk factors and indicators associated with vulnerability to radicalisation. Shortest path from crime and/or violence supportive morality to exposure highlighted.Crime/violent propensity [blue]: 1 – expelled from school, 2 – problematic drug use, 3 – problematic alcohol use, 4 – arrested as an adolescent, 5 – expressed a desire to hurt others, 6 – violent as a child/adolescent, 7 – committed a violent offence, 8 – committed a non-violent offense, 9 – perpetrator of domestic abuse.Low self-control [pink]: 10 – thrill-seeking, 11 – impulsivity.Cognitive susceptibility [orange]: 12 – victim of bullying as a child/adolescent, 13 – victim of violence as a child/adolescent, 14 – psychological distress, 15 – diagnosed mental disorder, 16 – difficulties coping with change, 17 – isolated, 18 – obsessive thinking, 19 – previous suicide attempt, 20 – history of self-harm, 21 – grew up in an abusive home, 22 – chronic stress, 23 – anger management problems, 24 – dropped out of school/university, 25 – worsening performance at work/school.Proximal crisis (all indicators measured as ‘within the last year’) [red]: 26 – recent unemployment, 27 – death in the family, 28 – proximate life change, 29 – proximate crisis, 30 – work stress, 31 – interrupted in pursuing a life goal, 32 – financial problems.Interpersonal stressors (all indicators measured as ‘within the last year’) [yellow]: 33 – experienced being degraded, 34 – experienced prejudice, 35 – had a promise broken, 36 – experienced being disrespected, 37 – ignored by someone important to them, 38 – harmed by the negligence of someone else, 39 – not cared for by someone important to them, 40 – victim of physical/verbal assault, 41 – felt like a helpless victim, 42 – problematic personal relationships.Exposure[green] : 43 – known someone who has extremist views, 44 – witnessed a direct threat of extremist violence, 45 – witnessed verbal statements in support of violent extremism, 46 – received extremist propaganda online, 47 – known of extremism in their community, 48 – received propaganda offline, 49 – face-to-face interactions with extremists, 50 – has extremist friends, 51 – engaged with extremist propaganda, 52 – searched for extremist content online, 53 – chosen to spend time in places where there is extremism, 54 – interacted virtually with extremists.](/cms/asset/b9f8fbf8-491f-4c8e-b8e3-3d452da43046/gpcl_a_2027944_f0004_oc.jpg)
Figure 5. Network analysis of risk factors and indicators associated with vulnerability to radicalisation. Shortest path from goal interrupted to exposure highlighted.
Crime/violent propensity [blue]: 1 – expelled from school, 2 – problematic drug use, 3 – problematic alcohol use, 4 – arrested as an adolescent, 5 – expressed a desire to hurt others, 6 – violent as a child/adolescent, 7 – committed a violent offence, 8 – committed a non-violent offense, 9 – perpetrator of domestic abuse.
Low self-control [pink]: 10 – thrill-seeking, 11 – impulsivity.
Cognitive susceptibility [orange]: 12 – victim of bullying as a child/adolescent, 13 – victim of violence as a child/adolescent, 14 – psychological distress, 15 – diagnosed mental disorder, 16 – difficulties coping with change, 17 – isolated, 18 – obsessive thinking, 19 – previous suicide attempt, 20 – history of self-harm, 21 – grew up in an abusive home, 22 – chronic stress, 23 – anger management problems, 24 – dropped out of school/university, 25 – worsening performance at work/school.
Proximal crisis (all indicators measured as ‘within the last year’) [red]: 26 – recent unemployment, 27 – death in the family, 28 – proximate life change, 29 – proximate crisis, 30 – work stress, 31 – interrupted in pursuing a life goal, 32 – financial problems.
Interpersonal stressors (all indicators measured as ‘within the last year’) [yellow]: 33 – experienced being degraded, 34 – experienced prejudice, 35 – had a promise broken, 36 – experienced being disrespected, 37 – ignored by someone important to them, 38 – harmed by the negligence of someone else, 39 – not cared for by someone important to them, 40 – victim of physical/verbal assault, 41 – felt like a helpless victim, 42 – problematic personal relationships.
Exposure [green]: 43 – known someone who has extremist views, 44 – witnessed a direct threat of extremist violence, 45 – witnessed verbal statements in support of violent extremism, 46 – received extremist propaganda online, 47 – known of extremism in their community, 48 – received propaganda offline, 49 – face-to-face interactions with extremists, 50 – has extremist friends, 51 – engaged with extremist propaganda, 52 – searched for extremist content online, 53 – chosen to spend time in places where there is extremism, 54 – interacted virtually with extremists.
![Figure 5. Network analysis of risk factors and indicators associated with vulnerability to radicalisation. Shortest path from goal interrupted to exposure highlighted.Crime/violent propensity [blue]: 1 – expelled from school, 2 – problematic drug use, 3 – problematic alcohol use, 4 – arrested as an adolescent, 5 – expressed a desire to hurt others, 6 – violent as a child/adolescent, 7 – committed a violent offence, 8 – committed a non-violent offense, 9 – perpetrator of domestic abuse.Low self-control [pink]: 10 – thrill-seeking, 11 – impulsivity.Cognitive susceptibility [orange]: 12 – victim of bullying as a child/adolescent, 13 – victim of violence as a child/adolescent, 14 – psychological distress, 15 – diagnosed mental disorder, 16 – difficulties coping with change, 17 – isolated, 18 – obsessive thinking, 19 – previous suicide attempt, 20 – history of self-harm, 21 – grew up in an abusive home, 22 – chronic stress, 23 – anger management problems, 24 – dropped out of school/university, 25 – worsening performance at work/school.Proximal crisis (all indicators measured as ‘within the last year’) [red]: 26 – recent unemployment, 27 – death in the family, 28 – proximate life change, 29 – proximate crisis, 30 – work stress, 31 – interrupted in pursuing a life goal, 32 – financial problems.Interpersonal stressors (all indicators measured as ‘within the last year’) [yellow]: 33 – experienced being degraded, 34 – experienced prejudice, 35 – had a promise broken, 36 – experienced being disrespected, 37 – ignored by someone important to them, 38 – harmed by the negligence of someone else, 39 – not cared for by someone important to them, 40 – victim of physical/verbal assault, 41 – felt like a helpless victim, 42 – problematic personal relationships.Exposure [green]: 43 – known someone who has extremist views, 44 – witnessed a direct threat of extremist violence, 45 – witnessed verbal statements in support of violent extremism, 46 – received extremist propaganda online, 47 – known of extremism in their community, 48 – received propaganda offline, 49 – face-to-face interactions with extremists, 50 – has extremist friends, 51 – engaged with extremist propaganda, 52 – searched for extremist content online, 53 – chosen to spend time in places where there is extremism, 54 – interacted virtually with extremists.](/cms/asset/011a61a5-c6df-4680-a3b7-565727be6398/gpcl_a_2027944_f0005_oc.jpg)
Figure 6. Network analysis of risk factors and indicators associated with vulnerability to radicalisation. Shortest path from interpersonal problems to exposure highlighted.
Crime/violent propensity [blue]: 1 – expelled from school, 2 – problematic drug use, 3 – problematic alcohol use, 4 – arrested as an adolescent, 5 – expressed a desire to hurt others, 6 – violent as a child/adolescent, 7 – committed a violent offence, 8 – committed a non-violent offense, 9 – perpetrator of domestic abuse.
Low self-control [pink]: 10 – thrill-seeking, 11 – impulsivity.
Cognitive susceptibility [orange]: 12 – victim of bullying as a child/adolescent, 13 – victim of violence as a child/adolescent, 14 – psychological distress, 15 – diagnosed mental disorder, 16 – difficulties coping with change, 17 – isolated, 18 – obsessive thinking, 19 – previous suicide attempt, 20 – history of self-harm, 21 – grew up in an abusive home, 22 – chronic stress, 23 – anger management problems, 24 – dropped out of school/university, 25 – worsening performance at work/school.
Proximal crisis (all indicators measured as ‘within the last year’) [red]: 26 – recent unemployment, 27 – death in the family, 28 – proximate life change, 29 – proximate crisis, 30 – work stress, 31 – interrupted in pursuing a life goal, 32 – financial problems.
Interpersonal stressors (all indicators measured as ‘within the last year’) [yellow]: 33 – experienced being degraded, 34 – experienced prejudice, 35 – had a promise broken, 36 – experienced being disrespected, 37 – ignored by someone important to them, 38 – harmed by the negligence of someone else, 39 – not cared for by someone important to them, 40 – victim of physical/verbal assault, 41 – felt like a helpless victim, 42 – problematic personal relationships.
Exposure [green]: 43 – known someone who has extremist views, 44 – witnessed a direct threat of extremist violence, 45 – witnessed verbal statements in support of violent extremism, 46 – received extremist propaganda online, 47 – known of extremism in their community, 48 – received propaganda offline, 49 – face-to-face interactions with extremists, 50 – has extremist friends, 51 – engaged with extremist propaganda, 52 – searched for extremist content online, 53 – chosen to spend time in places where there is extremism, 54 – interacted virtually with extremists.
![Figure 6. Network analysis of risk factors and indicators associated with vulnerability to radicalisation. Shortest path from interpersonal problems to exposure highlighted.Crime/violent propensity [blue]: 1 – expelled from school, 2 – problematic drug use, 3 – problematic alcohol use, 4 – arrested as an adolescent, 5 – expressed a desire to hurt others, 6 – violent as a child/adolescent, 7 – committed a violent offence, 8 – committed a non-violent offense, 9 – perpetrator of domestic abuse.Low self-control [pink]: 10 – thrill-seeking, 11 – impulsivity.Cognitive susceptibility [orange]: 12 – victim of bullying as a child/adolescent, 13 – victim of violence as a child/adolescent, 14 – psychological distress, 15 – diagnosed mental disorder, 16 – difficulties coping with change, 17 – isolated, 18 – obsessive thinking, 19 – previous suicide attempt, 20 – history of self-harm, 21 – grew up in an abusive home, 22 – chronic stress, 23 – anger management problems, 24 – dropped out of school/university, 25 – worsening performance at work/school.Proximal crisis (all indicators measured as ‘within the last year’) [red]: 26 – recent unemployment, 27 – death in the family, 28 – proximate life change, 29 – proximate crisis, 30 – work stress, 31 – interrupted in pursuing a life goal, 32 – financial problems.Interpersonal stressors (all indicators measured as ‘within the last year’) [yellow]: 33 – experienced being degraded, 34 – experienced prejudice, 35 – had a promise broken, 36 – experienced being disrespected, 37 – ignored by someone important to them, 38 – harmed by the negligence of someone else, 39 – not cared for by someone important to them, 40 – victim of physical/verbal assault, 41 – felt like a helpless victim, 42 – problematic personal relationships.Exposure [green]: 43 – known someone who has extremist views, 44 – witnessed a direct threat of extremist violence, 45 – witnessed verbal statements in support of violent extremism, 46 – received extremist propaganda online, 47 – known of extremism in their community, 48 – received propaganda offline, 49 – face-to-face interactions with extremists, 50 – has extremist friends, 51 – engaged with extremist propaganda, 52 – searched for extremist content online, 53 – chosen to spend time in places where there is extremism, 54 – interacted virtually with extremists.](/cms/asset/fca78200-097e-423f-a8ca-3c5177cdb575/gpcl_a_2027944_f0006_oc.jpg)
Figure 7. Network analysis of risk factors and indicators associated with vulnerability to radicalisation. Shortest path from proximal crisis to exposure highlighted.
Crime/violent propensity [blue]: 1 – expelled from school, 2 – problematic drug use, 3 – problematic alcohol use, 4 – arrested as an adolescent, 5 – expressed a desire to hurt others, 6 – violent as a child/adolescent, 7 – committed a violent offence, 8 – committed a non-violent offense, 9 – perpetrator of domestic abuse.
Low self-control [pink]: 10 – thrill-seeking, 11 – impulsivity.
Cognitive susceptibility [orange]: 12 – victim of bullying as a child/adolescent, 13 – victim of violence as a child/adolescent, 14 – psychological distress, 15 – diagnosed mental disorder, 16 – difficulties coping with change, 17 – isolated, 18 – obsessive thinking, 19 – previous suicide attempt, 20 – history of self-harm, 21 – grew up in an abusive home, 22 – chronic stress, 23 – anger management problems, 24 – dropped out of school/university, 25 – worsening performance at work/school.
Proximal crisis (all indicators measured as ‘within the last year’) [red]: 26 – recent unemployment, 27 – death in the family, 28 – proximate life change, 29 – proximate crisis, 30 – work stress, 31 – interrupted in pursuing a life goal, 32 – financial problems.
Interpersonal stressors (all indicators measured as ‘within the last year’) [yellow]: 33 – experienced being degraded, 34 – experienced prejudice, 35 – had a promise broken, 36 – experienced being disrespected, 37 – ignored by someone important to them, 38 – harmed by the negligence of someone else, 39 – not cared for by someone important to them, 40 – victim of physical/verbal assault, 41 – felt like a helpless victim, 42 – problematic personal relationships.
Exposure [green]: 43 – known someone who has extremist views, 44 – witnessed a direct threat of extremist violence, 45 – witnessed verbal statements in support of violent extremism, 46 – received extremist propaganda online, 47 – known of extremism in their community, 48 – received propaganda offline, 49 – face-to-face interactions with extremists, 50 – has extremist friends, 51 – engaged with extremist propaganda, 52 – searched for extremist content online, 53 – chosen to spend time in places where there is extremism, 54 – interacted virtually with extremists.
![Figure 7. Network analysis of risk factors and indicators associated with vulnerability to radicalisation. Shortest path from proximal crisis to exposure highlighted.Crime/violent propensity [blue]: 1 – expelled from school, 2 – problematic drug use, 3 – problematic alcohol use, 4 – arrested as an adolescent, 5 – expressed a desire to hurt others, 6 – violent as a child/adolescent, 7 – committed a violent offence, 8 – committed a non-violent offense, 9 – perpetrator of domestic abuse.Low self-control [pink]: 10 – thrill-seeking, 11 – impulsivity.Cognitive susceptibility [orange]: 12 – victim of bullying as a child/adolescent, 13 – victim of violence as a child/adolescent, 14 – psychological distress, 15 – diagnosed mental disorder, 16 – difficulties coping with change, 17 – isolated, 18 – obsessive thinking, 19 – previous suicide attempt, 20 – history of self-harm, 21 – grew up in an abusive home, 22 – chronic stress, 23 – anger management problems, 24 – dropped out of school/university, 25 – worsening performance at work/school.Proximal crisis (all indicators measured as ‘within the last year’) [red]: 26 – recent unemployment, 27 – death in the family, 28 – proximate life change, 29 – proximate crisis, 30 – work stress, 31 – interrupted in pursuing a life goal, 32 – financial problems.Interpersonal stressors (all indicators measured as ‘within the last year’) [yellow]: 33 – experienced being degraded, 34 – experienced prejudice, 35 – had a promise broken, 36 – experienced being disrespected, 37 – ignored by someone important to them, 38 – harmed by the negligence of someone else, 39 – not cared for by someone important to them, 40 – victim of physical/verbal assault, 41 – felt like a helpless victim, 42 – problematic personal relationships.Exposure [green]: 43 – known someone who has extremist views, 44 – witnessed a direct threat of extremist violence, 45 – witnessed verbal statements in support of violent extremism, 46 – received extremist propaganda online, 47 – known of extremism in their community, 48 – received propaganda offline, 49 – face-to-face interactions with extremists, 50 – has extremist friends, 51 – engaged with extremist propaganda, 52 – searched for extremist content online, 53 – chosen to spend time in places where there is extremism, 54 – interacted virtually with extremists.](/cms/asset/b58beab4-1725-4142-923b-26d278ed937c/gpcl_a_2027944_f0007_oc.jpg)
highlights pathways from cognitive susceptibility to exposure. Activations between crime/violent and situational nodes are evident. For instance, cognitive susceptibility nodes related to poor mental health connect to exposure nodes via crime/violent propensity nodes. This is true also of nodes related to adverse childhood experiences. Interactions among nodes obsessive thinking, chronic stress, and isolation demonstrate connections with both proximal crisis nodes (goal interrupted) as well as a direct pathway to exposure, via receiving propaganda online.
highlights pathways from crime/violent propensity nodes to exposure. These shortest paths suggest that a violent propensity may be a direct driver of exposure. Interestingly, all pathways to exposure activate via passive exposure nodes. Passive exposure is highly associated with active exposure and the present findings suggest perhaps a ‘gateway’ to self-initiated exposure to radicalising influences.
highlights pathways between low self-control and exposure. Disaggregating thrill-seeking and impulsivity from low self-control allows for the modelling of potentially differential effects. Here, thrill-seeking is a risk factor for exposure via non-violent offending.
and display shortest paths between interpersonal stressors, proximal crisis, and exposure nodes, respectively. The pathways reiterate the importance of goal interruption and isolation.
Discussion
The present study applied the psychometric network framework to understand vulnerability to radicalisation. A general aim was to demonstrate the utility of a psychometric network approach to terrorism studies, and more broadly, crime. Specifically, the findings provide data-driven evidence for the conceptualisation of vulnerability to radicalisation as the outcome of a dynamic, interactional system, as theorised by the RAF. In this section, we first discuss the results from the overall network graph. Second, we discuss the identified pathways to exposure. Third, we consider the practical implications of the present findings, particularly in terms of a public health approach.
A psychometric network approach to vulnerability to radicalisation
The present study characterises risk factors and indicators for vulnerability to radicalisation as a complex system, where factors interact and cause each other in a network structure. Psychometric network analysis is model free and driven by the data. In this way, it may be a useful tool to model theoretical constructs and how they may relate to each other, without introducing researcher inference, as we have done here with the RAF. Such an approach could provide useful insights across the study of crime in general.
More specifically, the results disaggregate communities of nodes which map onto mechanisms theorised by the RAF. Previous work operationalised these mechanisms with existing behavioural indicators collated by Gill et al. (2014), Clemmow, Gill, et al. (Citation2020), Clemmow et al. (Citation2019), and Corner and Gill (Citation2019). This was based on guidance from the RAF as well as inference. Hence it is promising to see these constructs emerge naturally. This provides support for previous operationalisations of the RAF and further empirical evidence for the framework as a tool for understanding radicalisation.
In terms of node importance, being disrespected, psychological distress, and non-violent offending were identified as the most central nodes/risk factors. This is not unexpected as being disrespected relates more generally to forming a grievance; a known driver of the motivation to engage in terrorism (Piazza, Citation2017; Ravndal, Citation2018). The role of psychological distress too, has been widely researched and implicated in pathways to engagement in extremism (Corner & Gill, Citation2019; Corner et al., Citation2018). Past offending similarly is both a common behavioural correlate of extremism (Jensen et al., Citation2020; LaFree et al., Citation2018), as well as an indicator of a crime and/or violent propensity (Bouhana, Citation2019). Whilst these results may appear unsurprising, their utility is in providing a guide for targeted interventions. Important nodes in a network are useful targets for intervention (Borsboom & Cramer, Citation2013). Changes in their presence may result in the most change across the entire network of risk factors. Supressing the occurrence or mitigating the impact of these risk factors, in other words, may ‘dismantle the network’. We discuss this in more detail in terms of the practical implications of these findings.
Community detection identified 6 communities. Communities relating to propensity (cognitive susceptibility and crime/violent propensity) and situation (interpersonal stressors and proximal crisis) were highly interconnected. This accords with previous calls for research to consider the compounding effect of multiple risk factors (Gill, Citation2015), or a pathways approach to understanding radicalisation (Horgan, Citation2008). Simply, there is likely no single driver of radicalisation, rather it is the crystallisation of personal and situational characteristics, converging in time and space, which results in radicalisation, for some (Bouhana, Citation2019; Gill, Citation2015; Horgan, Citation2008). The overall network graph visualises this.
In contrast, propensity and situation communities demonstrated less connectivity with the exposure community. Given that actively engaging with extremism-supportive people, places, and settings, is low base rate, this makes sense (see Clemmow, Schumann et al., Citation2020). Indicators relating to propensity and situation have been shown to be reasonably prevalent among the general population (Clemmow, Schumann et al., Citation2020). However, many do not go on to interact with people, places, or settings who support extremism, develop extremist beliefs, engage with extremist movements, or commit violent extremist acts. Hence the present findings suggest how configurations of personal and situational risk factors may drive active or passive exposure (and vice versa); perhaps some way to understanding ‘when and for whom’ exposure may be relevant to becoming vulnerable to radicalisation. We elaborate upon this next as we discuss in more detail the detected pathways to exposure.
Pathways to exposure
The role of exposure in understanding radicalisation is key (Taylor & Horgan, Citation2006; Wiktorowicz, Citation2004). Exposure likely impacts upon pathways to radicalisaiton at multiple points, during belief formation, engagement, and onto violent action (Pauwels et al., Citation2014). Measuring constructs such as radicalisation, or the emergence of motivation, from a practical perspective, is challenging. However, exposure is often objectively observable, and so could be useful to attend to for the purposes of identifying those vulnerable to radicalisaiton. Hence understanding the drivers of active exposure may help signal those most vulnerable to belief formation, but also those who may go on to be vulnerable to engagement, and committing violent action.
The results suggest that a criminal and/or violent propensity may be a driver of exposure. Previous criminality, including past violence is often a correlate of radicalisation. For instance, Coid et al. (Citation2016) conducted a general population survey of 3679 18–34-year-old men in the UK. They found significant positive associations with drug misuse, previous criminal behaviour, previous violence, and extreme pro-British views. Previous violence and past imprisonment were associated with extreme anti-British views. More recently, Jensen et al. (Citation2020) explored the criminal histories of 1, 900 US extremists. They found that past criminal behaviour (violent or non-violent), was the strongest predictor of radicalised individuals committing ideologically motivated violence.
Research on violence in general consistently reports past criminal behaviour, including violence, as a reliable predictor of future violence. For example, in a prospective longitudinal study of 411 South London young males, Farrington (Citation2001) found that past convictions for non-violent crimes predicted adult violence better than past convictions for future violence. Equally, past antisocial behaviour indicated risk for future violence. In the present study, non-violent offending was identified as an important node. Particularly noteworthy are the node’s connections to exposure.
Selection is described as a mechanism for exposure by the RAF (Bouhana, Citation2019). In terms of self-selection, offenders with a proclivity for crime and/or violence may choose to spend time with people, or in places, both online and offline, that promote extremist moral norms. This may be one explanation for the interaction between non-violent offending, witnessing verbal statements in support of violent action, and receiving propaganda online. In terms of social selection, person-specific characteristics may dictate the types of spaces (online and offline), where individuals spend time. In this way, previous criminality may be a social selection factor. Equally, an individual’s history of offending may be related to the same social selection factors that drive exposure, i.e. ethnicity, socioeconomic status, religion, and so on.
Shortest path analysis from cognitive susceptibility to exposure highlighted connections to exposure via situational and crime and/or violent propensity nodes; visualising the equifinality of pathways to exposure. Interestingly, in terms of mental illness and psychological distress, there is no direct ‘route’ to exposure. This is concordant with Corner and Gill’s (Citation2019) state transition diagrams which suggest that mental disorder likely impacts upon trajectories differently, at different points during the offence commission process. Corner et al. (Citation2018) summarise the evidence base for mental disorders, personality traits, and grievance-fuelled targeted violence. They summarise that ‘(E)xamination of different disorders, situations, demographics, along with unique experiences provide more rounded answers regarding attribution of mental disorder to criminal and violent behaviour’ (Corner et al., Citation2018, p. 460).
Nuanced research such as Monahan et al. (Citation2001) too moves beyond simplistic explanations and analyses how multiple factors such as past violence, previous criminality, adverse childhood experiences, and situational influences, interact with diagnosed mental disorder and personality traits to result in violent behaviour. In other words, how mental disorder may drive violence for some people, in some circumstances. In addition to the cognitive susceptibility-exposure pathway highlighted, findings similar to Monahan et al. (Citation2001) can be seen in the overall graph. How mental disorder may drive exposure in different people, under different circumstances, is illustrated by the numerous routes from nodes related to poor mental health, to exposure. In general, the results echo calls from Corner and colleagues for a more nuanced approach to understanding the role of mental disorder in vulnerability to radicalisation. Specifically, the present findings highlight several potential mediators to consider with confirmatory research designs and possibly when designing preventative programming.
Lastly in terms of cognitive susceptibility, isolation appears an important node. The inverse, social support, is often a protective factor in extremist research. Milla and Hudiyana (Citation2019) conducted structured interviews with 241 prisoners serving sentences for terrorism offences in Indonesia. They found that the higher the breadth of relationships with people outside the terrorist group, the lower the commitment to the radical group. Similarly, Rousseau et al.’s (Citation2021) Canadian survey found those high in perceived social support scored lower in violent extremist sympathies. High social support also managed to buffer the impact of other risk factors positively associated with violent extremist sympathies such as perceived discrimination. Cardeli et al.’s (Citation2020) analysis of 542 Somali Refugees in the United States also demonstrates the mediating role of social bonds upon adversity and radicalisation. The present results suggest again that isolation may be an important mediator between cognitive susceptibility risk factors and exposure.
The shortest path from low self-control to exposure highlights thrill-seeking as a potential driver of exposure. Previous research reports thrill-seeking is associated with aggression and crime/delinquency (Wilson & Scarpa, Citation2011). The association among thrill-seeking, non-violent offending, and exposure, reiterates the findings of previous research. Similarly, research on extremism widely acknowledges the role of thrill-seeking in pathways to radicalisation. Some theorise the role of stable personality factors (Horgan, Citation2003; Kruglanski et al., Citation2018; Victoroff, Citation2005). For instance, Borum (Citation2003) summarises several predispositions which may impact upon the development of a terrorist propensity. One of which, thrill-seeking, may be a significant ‘pull’ factor, via perceived excitement, that attracts some to an extremist narrative. Hence it follows that thrill-seeking may be an important risk factor for exposure.
Schumpe et al. (Citation2020) conducted a series of studies to empirically test the role thrill-seeking in political violence, in the context of Significance Quest Theory. Over seven studies, the authors found a positive effect of thrill-seeking on support for political violence. Furthermore, Nussio (Citation2020) compared voluntary and nonvoluntary joiners of Colombian insurgent and paramilitary groups, arguing that the nonvoluntary joiners would have similar characteristics to non-joiners. Despite similarities in demographic characteristics, the nonvoluntary joiners scored higher on three measures of sensation seeking (boredom susceptibility, disinhibition, and thrill and adventure-seeking).
Many extremist risk assessment tools also consider the role of thrill-seeking. For instance, the Vulnerability Assessment Framework (VAF) (Home Office, Citation2012), the ERG 22+ and the VERA-2R (Lloyd & Dean, Citation2015). Articulating the role of thrill-seeking in active exposure may help specify how some come to seek out engagement with extremism.
Pathways from nodes conceptualised as relating to situational influences present similarly to previous research. Clemmow et al. (Citation2019) disaggregated four person-exposure patterns (PEPs) from a cohort of 125 lone-actor terrorists. The results demonstrated how different individual-level susceptibilities, interact with situational and exposure indicators along trajectories to violent extremism. The situational PEP suggested acute stress and wider situational influences may be key to the emergence of the motivation to act; seemingly in the absence of a cognitive susceptibility or crime/violent propensity. The two situational pathways to exposure presented here conclude similarly. Routes to exposure absent of interactions with more distal risk factors are evident. Hence it may be important to consider the influence of situational factors in the absence of more distal, compounding risk factors as drivers of exposure. Next, we consider the practical implications of the present findings.
Practical implications
A psychometric network approach to violent extremism presents further evidence for conceptualising risk factors and indicators for vulnerability to radicalisation as a dynamic system. This has important practical implications and provides support for both a structured professional judgement (SPJ) approach to risk assessment (Logan & Lloyd, Citation2019; Monahan, Citation2012, Citation2017), and a multi-agency and/or public-health approach to preventing extremism.
Douglas and Kropp (Citation2002, p. 619) describe how ‘a prominent reconceptualization within the risk assessment field has been increased attention to risk management where the primary task at hand is not the accurate specification of who will or will not be violent per se but rather the proper selection of strategies to reduce violence and mitigate risk’. Taking a preventative approach, our findings have relevance to the risk management of the potentially vulnerable. In particular, our findings have relevance to an SPJ approach. An SPJ approach to risk assessment and management draws together knowledge on evidence-based risk factors, with experience and theory-informed judgements. Given the multifinality of risk factors and the equifinality of pathways to vulnerability to radicalisation, such an approach may be more stable grounds for risk management than actuarial-based tools. The network graphs visualise the difficulty of relying on actuarial tools which band levels of risk based on the presence of a risk factor. The results instead align with SPJ principles of conceptualising risk as the outcome of multifactorial interactions, and that the relevance of risk factors and their associations with other risk and protective factors, matter more.
More generally, the results provide further support for a multi-agency and/or public health approach to tackling extremism. For example, highlights the pathway between poor mental health, adverse childhood experiences, other stressors, and exposure. It is these antecedent risk factors which many prevention efforts in practice try to detect, manage, and mitigate. For instance, multi-agency hubs across the UK look beyond the presence or absence of single risk factors and formulate multi-agency responses to complex problems (Home Office, Citation2018). FTAC and QFTAC take a similar approach (albeit specifically focussed on mental health) among the pathologically fixated and grievance-fuelled violence offenders in general (Pathé et al., Citation2018). Pathé et al. (Citation2018, p. 48) describe how ‘(T)traditional counter-terrorist policing methods cannot ameliorate this multi-factorial problem, but inter-agency cooperation and skill sharing can enhance current efforts’. The results demonstrated here are amongst the first to empirically verify the conceptual approaches embedded in such upstream preventative effots.
suggests that effective interventions aimed at reducing criminal propensities and aggression may reduce the drivers of susceptibility to exposures, thus reducing the numbers of people who are exposed to extremists, their materials, and settings. The same is true for in relation to interventions focused upon either improving self-control or offering alternative thrill-inducing initiatives into someone’s routine activities. Such early prevention efforts may have no need to be labelled as ‘counter-terrorism’. At the core of a public health approach is managing vulnerability to mitigate risk and improve quality of life – this has far wider-reaching implications than counterterrorism.
For instance, a public health approach to violent crime in Scotland has largely been viewed as successful, with calls for London to implement a similar strategy to tackle a growing knife crime problem (Torjesen, Citation2018). Advocates suggest implementing the ‘Scottish model’ to identify those most vulnerable and improve their immediate situation, whilst inoculating the general population through education and changing social norms. In terms of extremism, taking a public health approach and identifying those most vulnerable, early on, and implementing evidenced-based interventions, may ‘deactivate’ some of the drivers of radicalisation, thus ‘dismantling the network’.
Limitations and future research
It is important to consider the limitations of the present study. First, Prolific users are not a naïve survey population, where naivety here is taken to mean non-professional survey respondents who are not familiar with many research materials and protocols. However, research comparing MTurk, Prolific, and CrowdFlower (CF) finds the users of the latter two more naïve and honest than MTurk users, a higher response rate yet higher rate of attention check failure in CF users, and that Prolific users produced data of comparable quality to MTurk’s, and better than CF’s (Peer et al., Citation2017). Despite such findings, itis still necessary to consider the implications of employing a potentially non-naïve sample However, Prolific users may be more naïve survey respondents than samples typically afforded to researchers, such as undergraduate student samples, who are often experienced survey participants, and equally not representative of the general population. Moreover, Prolific provides researchers with new opportunities to engage quickly, with global populations, at a relatively low cost, and is potentially a promising tool for a field which often succumbs to such issues with data availability.
A sample gathered via Prolific may also be subject to selection bias, where sampling is limited to those who have access to the internet. The generalisability of the sample may therefore be somewhat limited. However, again, research is often limited by the nature of the sample afforded to researchers; for instance, undergraduate students are typical participants in psychology research. In this respect, Prolific may in fact provide researchers wider access to more diverse samples than has previously been afforded to them.
Second, the data were self-reported. Dependent on the research design, directly self-reported information can be participant to a number of biases and errors, including underreporting socially undesirable items, overreporting socially desirable items, interviewer effects, bystander effects, and more (Krumpal, Citation2013; Tourangeau & Yan, Citation2007). However, as Gomes et al. (Citation2019) summarise, no research design will be absent of these. It is therefore up to researchers to attempt to manage the extent of any biases, and more importantly, to report these transparently. It is likely the present study succumbed to underreporting of sensitive attitudes and behaviours.
One way to address such underreporting may be to employ an indirect questioning design (future research may wish to consider such an approach if replicating the present study). We chose to employ a direct questioning design based on the findings of previous research gathering self-reports of sensitive attitudes and behaviours with Prolific users (Clemmow, Schumann et al., Citation2020). Clemmow, Schumann et al. (Citation2020) compared an indirect questioning design (the Unmatched Count Technique) to a direct questioning design, and found the former elicited more truthful (and usable) data than indirect questioning. However, we suggest that further work consider alternative questioning designs to further explore the extent and effect of any potential biases, particularly with Prolific users.
Considering network analysis, the present study was exploratory. One way to consider the results of network analysis is as hypothesis generating. We suggest replicating these findings in novel samples, perhaps by conducting confirmatory hypothesis testing utilising the potential mediators identified here. The reliability and accuracy of network analysis is also important to attend to. Whilst the metrics presented here suggest moderate reliability and accuracy, without replication, any findings and their subsequent interpretation should remain tentative.
Lastly, the present study employs a representative general population sample. Our findings are likely generalisable to those susceptible to radicalisation, i.e. potentially vulnerable populations who have not engaged in extremism or extremist violence. The applicability of our findings to violent extremists is limited, however previous research has demonstrated similar clusters of risk factors emerging from a cohort of lone-actor terrorists (Clemmow et al., Citation2019). To address this limitation, we recommend conducting further research among populations presenting different gradations of risk in order to identify risk factors which may differentiate between those who are vulnerable to radicalisation, those who are radicalised, and those who actually commit extremist violence.
Further, whilst a representative sample affords researchers greater generalisability, in the present instance it is important to consider the implications of sampling respondents who may typically be considered ‘less risky’. In particular, our sample includes respondents aged up to 80 years old. Previous research suggests proclivity for crime decreases with age (Shover & Thompson, Citation1992), and the same is likely true of those vulnerable to radicalisation. As a first step, we felt it justified to consider how vulnerability to radicalisation appeared in a sample representative of the general population. In the future, we will consider replicating the present study amongst a sample more characteristic of the typical offender, i.e. male or under 50 years old.
Conclusion
The network framework has gained popularity in the psychological sciences. There are opportunities for researchers studying crime and violence to apply this novel approach to how we understand criminal behaviour. One interesting application to consider is how person-specific individual networks may help practitioners formulate treatment plans. For instance, in terms of psychopathology, a single patient’s symptoms may be modelled as a network to help a clinician make decisions about appropriate treatment and intervention opportunities. This may equally be relevant to consider for practitioners working in forensic settings who need to articulate a case formulation for guiding treatment and intervention, tailored to the individual.
Time series networks could also prove an interesting avenue for future research. Analysis of time series data in terrorism studies is limited, given the obvious challenges. However, utilising general population samples may suggest a route forward. Collecting time series, or panel data, among a general population sample, is feasible. Such data can then be modelled as time series networks. These graphs are directed and may provide further insight into how risk factors cause and relate to each other, over time. Such work among offending samples could also be an important research endeavour.
Our results provide support for calls to conceptualise vulnerability to radicalisation as emerging from a dynamic system. As the field continues to progress beyond a profiling approach, novel research designs demonstrate the complexity of the problem at hand. Equally, the evolution of preventative programs towards a public health approach alludes to a practical need to model such complexity. The present findings demonstrate how a network approach may be one way to do so; affording researchers a more nuanced insight into complex and heterogenous populations. This has wider implications for criminology in general. The applications of the network approach are vast and could provide researchers with a further analytical tool to explore crime and violence problems.
Supplementary_material.docx
Download MS Word (1.5 MB)Disclosure statement
No potential conflict of interest was reported by the author(s).
Additional information
Funding
Notes
1 The data employed in the present study were collected as part of a wider survey, hence there are some measures that appear in the full survey on the OSF that are not utilised here.
References
- Baldet, W. (2020). Prevent and the terrorism act: We need to talk about Kieron. https://www.radicalrightanalysis.com/2020/02/27/prevent-and-the-terrorism-act-we-need-to-talk-about-kieron/
- Barry-Walsh, J., James, D. V., & Mullen, P. E. (2020). Fixated threat assessment centres: Preventing harm and facilitating care in public figure threat cases and those thought to be at risk of lone-actor grievancefuelled violence. In K. Warburton & S. Stahl (Eds.), Decriminalizing mental illness (pp. 328). Cambridge University Press.
- Berinsky, A. J., Margolis, M. F., & Sances, M. W. (2014). Separating the shirkers from the workers? Making sure respondents pay attention on self-administered surveys. American Journal of Political Science, 58(3), 739–753. https://doi.org/10.1111/ajps.12081
- Bhui, K. S., Hicks, M. H., Lashley, M., & Jones, E. (2012). A public health approach to understanding and preventing violent radicalization. BMC Medicine, 10(1), 16. https://doi.org/10.1186/1741-7015-10-16
- Bichler, G., & Malm, A. (2018). Social network analysis. In Routledge Handbook of Crime Science (pp. 207–222). Routledge.
- Bogaerts, S., Spreen, M., Masthoff, E., & Jankovic, M. (2020). Longitudinal network structure and changes of clinical risk and protective factors in a nationwide sample of forensic psychiatric patients. International Journal of Offender Therapy and Comparative Criminology, 64(15), 1533–1550.
- Borsboom, D. (2008). Psychometric perspectives on diagnostic systems. Journal of Clinical Psychology, 64(9), 1089–1108. https://doi.org/10.1002/jclp.20503
- Borsboom, D., & Cramer, A. O. J. (2013). Network analysis: An integrative approach to the structure of psychopathology. Annual Review of Clinical Psychology, 9(1), 91–121. https://doi.org/10.1146/annurev-clinpsy-050212-185608
- Borum, R. (2003). Understanding the terrorist mind-set. FBI L. Enforcement Bull, 72, 7.
- Bouhana, N. (2019). The moral ecology of extremism a systemic perspective. https://assets.publishing.service.gov.uk/government/uploads/system/uploads/attachment_data/file/834354/Bouhana-The-moral-ecology-of-extremism.pdf
- Bringmann, L. F., Elmer, T., Epskamp, S., Krause, R. W., Schoch, D., Wichers, M., Wigman, J. T. W., & Snippe, E. (2019). What do centrality measures measure in psychological networks? Journal of Abnormal Psychology, 128(8), 892–903. https://doi.org/10.1037/abn0000446
- Cardeli, E., Sideridis, G., Lincoln, A. K., Abdi, S. M., & Ellis, B. H. (2020). Social bonds in the diaspora: The application of social control theory to Somali refugee young adults in resettlement. Psychology of Violence, 10(1), 18–29. https://doi.org/10.1037/vio0000259
- Clemmow, C., Bouhana, N., & Gill, P. (2019). Analyzing person-exposure patterns in lone-actor terrorism. Criminology & Public Policy, 19(2), 451–482. https://doi.org/10.1111/capp.v19.2
- Clemmow, C., Gill, P., Bouhana, N., Silver, J., & Horgan, J. (2020). Disaggregating lone-actor grievance-fuelled violence: Comparing lone-actor terrorists and mass murderers. Terrorism and Political Violence, 1–26. https://doi.org/10.1080/09546553.2020.1718661
- Clemmow, C., Gill, P., Corner, E., Farnham, F., Taylor, R., Wilson, S., Taylor, A., & James, D. (2021). A data-driven classification of outcome behaviors in those who cause concern to British public figures. Psychology, Public Policy, and Law, 27(4), 509–521. https://doi.org/10.1037/law0000269
- Clemmow, C., Schumann, S., Salman, N. L., & Gill, P. (2020). The base rate study: Developing base rates for risk factors and indicators for engagement in violent extremism. Journal of Forensic Sciences, 65(3), 865–881. https://doi.org/10.1111/1556-4029.14282
- Coid, J. W., Bhui, K., MacManus, D., Kallis, C., Bebbington, P., & Ullrich, S. (2016). Extremism, religion and psychiatric morbidity in a population-based sample of young men. The British Journal of Psychiatry, 209(6), 491–497. https://doi.org/10.1192/bjp.bp.116.186510
- Corner, E., & Gill, P. (2019). Psychological distress, terrorist involvement and disengagement from terrorism: A sequence analysis approach. Journal of Quantitative Criminology, 36(3), 499–526. https://doi.org/10.1007/s10940-019-09420-1
- Corner, E., Gill, P., Schouten, R., & Farnham, F. (2018). Mental disorders, personality traits, and grievance-fueled targeted violence: The evidence base and implications for research and practice. Journal of Personality Assessment, 100(5), 459–470. https://doi.org/10.1080/00223891.2018.1475392
- Costantini, G., Epskamp, S., Borsboom, D., Perugini, M., Mõttus, R., Waldorp, L. J., & Cramer, A. O. J. (2015). State of the aRt personality research: A tutorial on network analysis of personality data in R. Journal of Research in Personality, 54, 13–29. https://doi.org/10.1016/j.jrp.2014.07.003
- Csardi, G., & Nepusz, T. (2006). The igraph software package for complex network research. InterJournal, Complex Systems, 1695(5), 1–9.
- Dalege, J., Borsboom, D., van Harreveld, F., Waldorp, L. J., & van der Maas, H. L. J. (2017). Network structure explains the impact of attitudes on voting decisions. Scientific Reports, 7(1), 1–11. https://doi.org/10.1038/s41598-017-05048-y
- De Beurs, D. (2017). Network analysis: A novel approach to understand suicidal behaviour. International Journal of Environmental Research and Public Health, 14(3), 219. https://doi.org/10.3390/ijerph14030219
- Department of Homeland Security. (2021). Center for the preventio programs and pertnerships. Retrieved May 16, 2021, from https://www.dhs.gov/CP3
- Desmarais, S. L., Simons-Rudolph, J., Brugh, C. S., Schilling, E., & Hoggan, C. (2017). The state of scientific knowledge regarding factors associated with terrorism. Journal of Threat Assessment and Management, 4(4), 180. https://doi.org/10.1037/tam0000090
- Douglas, K. S., & Kropp, P. R. (2002). A prevention-based paradigm for violence risk assessment: Clinical and research applications. Criminal Justice and Behavior, 29(5), 617–658.
- Epskamp, S., Borsboom, D., & Fried, E. I. (2018). Estimating psychological networks and their accuracy: A tutorial paper. Behavior Research Methods, 50(1), 195–212. https://doi.org/10.3758/s13428-017-0862-1
- Epskamp, S., Cramer, A. O. J., Waldorp, L. J., Schmittmann, V. D., & Borsboom, D. (2012). Qgraph: Network visualizations of relationships in psychometric data. Journal of Statistical Software, 48(4), 1–18. https://hdl.handle.net/11245/1.380173, https://doi.org/10.18637/jss.v048.i04
- Farrington, D. P. (2001). Predicting adult official and self-reported violence. In G.-F. Pinard & L. Pagani (Eds.), Clinical assessment of dangerousness: Empirical contributions (pp. 66–88). Cambridge University Press. https://doi.org/10.1017/CBO9780511500015.005
- Fruchterman, T. M. J., & Reingold, E. M. (1991). Graph drawing by force-directed placement. Software: Practice and Experience, 21(11), 1129–1164. https://doi.org/10.1002/spe.4380211102
- Gill, P. (2015). Lone-actor terrorists: A behavioural analysis. Routledge.
- Gill, P., Clemmow, C., Hetzel, F., Rottweiler, B., Salman, N., Van Der Vegt, I., Marchment, Z., Schumann, S., Zolghadriha, S., Schulten, N., Taylor, H., & Corner, E. (2021). Systematic review of mental health problems and violent extremism. The Journal of Forensic Psychiatry & Psychology, 32(1), 51–78. https://doi.org/10.1080/14789949.2020.1820067
- Gill, P., Horgan, J., & Deckert, P. (2014a). Bombing alone: Tracing the motivations and antecedent behaviors of lone-actor terrorists. Journal of Forensic Sciences, 59(2), 425–435. https://doi.org/10.1111/1556-4029.12312
- Gill, P., Lee, J., Rethemeyer, K. R., Horgan, J., & Asal, V. (2014b). Lethal connections: The determinants of network connections in the provisional Irish republican army, 1970–1998. International Interactions, 40(1), 52–78. https://doi.org/10.1080/03050629.2013.863190
- Gill, P., Silver, J., Horgan, J., Corner, E., & Bouhana, N. (2021). Similar crimes, similar behaviors? Comparing lone-actor terrorists and public mass murderers. Journal of Forensic Sciences, 66(5), 1797–1804.
- Gomes, H. S., Farrington, D. P., Maia, Â, & Krohn, M. D. (2019). Measurement bias in self-reports of offending: A systematic review of experiments. Journal of Experimental Criminology, 15(3), 313–339. https://doi.org/10.1007/s11292-019-09379-w
- Gruenewald, J., Chermak, S., & Freilich, J. D. (2013). Distinguishing “loner” attacks from other domestic extremist violence: A comparison of far-right homicide incident and offender characteristics. Criminology and Public Policy, 12(1), 65–91.
- Hassan, G., Brouillette-Alarie, S., Alava, S., Frau-Meigs, D., Lavoie, L., Fetiu, A., Varela, W., Borokhovski, E., Venkatesh, V., Rousseau, C., & Sieckelinck, S. (2018). Exposure to extremist online content could lead to violent radicalization: A systematic review of empirical evidence. International Journal of Developmental Science, 12(1-2), 71–88.
- Heino, M. T. J., Knittle, K., Fried, E., Sund, R., Haukkala, A., Borodulin, K., Uutela, A., Araujo-Soares, V., Vasankari, T., & Hankonen, N. (2019). Visualisation and network analysis of physical activity and its determinants: Demonstrating opportunities in analysing baseline associations in the let’s move It trial. Health Psychology and Behavioral Medicine, 7(1), 269–289. https://doi.org/10.1080/21642850.2019.1646136
- Hevey, D. (2018). Network analysis: A brief overview and tutorial. Health Psychology and Behavioral Medicine, 6(1), 301–328.
- Home Office. (2012). Channel: Vulnerability assessment framework. https://www.gov.uk/government/publications/channel-vulnerability-assessment
- Home Office. (2018). Counter-terrorism strategy (CONTEST) 2018. https://assets.publishing.service.gov.uk/government/uploads/system/uploads/attachment_data/file/97995/strategy-contest.pdf
- Horgan, J. (2003). The search for the terrorist personality. In A. Silke (Ed.), Terrorists, victims and society (pp. 3–27). Wiley & Sons Ltd.
- Horgan, J. (2008). From profiles to pathways and roots to routes: Perspectives from psychology on radicalization into terrorism. The ANNALS of the American Academy of Political and Social Science, 618(1), 80–94. https://doi.org/10.1177/0002716208317539
- Horgan, J. G., Gill, P., Bouhana, N., Silver, J., & Corner, E. (2016). Across the universe? A comparative analysis of violent radicalization across three offender types with implications for criminal justice training and education. National Criminal Justice Reference System.
- Jensen, M. A., Safer-Lichtenstein, A., James, P. A., & LaFree, G. (2020). The link between prior criminal record and violent political extremism in the united. In D. Weisburd, E. Savona, B. Hasisi, & F. Calderoni (Eds.), Understanding recruitment to organized crime and terrorism (pp. 121–146). Springer. https://doi.org/10.1007/978-3-030-36639-1_6
- Kruglanski, A. W., Jasko, K., Chernikova, M., Dugas, M., & Webber, D.. (2018). To the fringe and back: Violent extremism and the psychology of deviance. American Psychologist, 72(3), 217–230. https://doi.org/10.1037/amp0000091
- Krumpal, I. (2013). Determinants of social desirability bias in sensitive surveys: A literature review. Quality and Quantity, 47(4), 2025–2047.
- LaFree, G., Jensen, M. A., James, P. A., & Safer-Lichtenstein, A. (2018). Correlates of violent political extremism in the United States. Criminology; An interdisciplinary Journal, 56(2), 233–268. https://doi.org/10.1111/1745-9125.12169
- Lloyd, M., & Dean, C. (2015). The development of structured guidelines for assessing risk in extremist offenders. Journal of Threat Assessment and Management, 2(1), 40–52. https://doi.org/10.1037/tam0000035
- Logan, C., & Lloyd, M. (2019). Violent extremism: A comparison of approaches to assessing and managing risk. Legal and Criminological Psychology, 24(1), 141–161. https://doi.org/10.1111/lcrp.12140
- Lösel, F., Bender, D., Jugl, I., & King, S. (2020). Resilience against political and religious extremism, radicalization, and related violence: A systematic review of studies on protective factors. In D. Weisburd, E. Savona, B. Hasisi, & F. Calderoni (Eds.), Understanding recruitment to organized crime and terrorism (pp. 55–84). Springer.
- Lynam, D. R., & Miller, J. D. (2004). Personality pathways to impulsive behavior and their relations to deviance: Results from three samples. Journal of Quantitative Criminology, 20(4), 319–341. https://doi.org/10.1007/s10940-004-5867-0
- McCuish, E., Bouchard, M., & Beauregard, E. (2021). A network-based examination of the longitudinal association between psychopathy and offending versatility. Jounral of Quantitative Criminology, 37(3), 693–714. https://doi.org/10.1007/s10940-020-09462-w
- Milla, M. N., & Hudiyana, J. (2019). The protective role of friendship: Cross-group friendship mediates the effect of ideological quest for significance on commitment to a radical group. Differences, 76, 87. https://doi.org/10.7454/proust.v2i2.42
- Monahan, J. (2012). The individual risk assessment of terrorism. Psychology, Public Policy, and Law, 18(2), 167. https://doi.org/10.1037/a0025792
- Monahan, J. (2017). The individual risk assessment of terrorism: Recent developments. The handbook of the criminology of terrorism (pp. 520–534). Wiley.
- Monahan, J., Steadman, H. J., Silver, E., Appelbaum, P. S., Robbins, P. C., Mulvey, E. P., Roth, L. H., Grisso, T., & Banks, S. M. (2001). Rethinking risk assessment: The MacArthur study of mental disorder and violence. Oxford University Press.
- Nussio, E. (2020). The role of sensation seeking in violent armed group participation. Terrorism and Political Violence, 32(1), 1–19. https://doi.org/10.1080/09546553.2017.1342633
- Oppenheimer, D. M., Meyvis, T., & Davidenko, N. (2009). Instructional manipulation checks: Detecting satisficing to increase statistical power. Journal of Experimental Social Psychology, 45(4), 867–872. https://doi.org/10.1016/j.jesp.2009.03.009
- Palan, S., & Schitter, C. (2018). Prolific. ac-A subject pool for online experiments. Journal of Behavioral and Experimental Finance, 17, 22–27.
- Pathé, M. T., Haworth, D. J., Goodwin, T., Holman, A. G., Amos, S. J., Winterbourne, P., & Day, L. (2018). Establishing a joint agency response to the threat of lone-actor grievance-fuelled violence. The Journal of Forensic Psychiatry & Psychology, 29(1), 37–52. https://doi.org/10.1080/14789949.2017.1335762
- Pauwels, L., Brion, F., & De Ruyver, B. (2014). Explaining and understanding the role of exposure to new social media on violent extremism. An integrative quantitative and qualitative approach (RADIMED): Summary. https://orfeo.kbr.be/bitstream/handle/internal/4197/synTA043_en.pdf?sequence=1
- Pauwels, L., & Schils, N. (2016). Differential online exposure to extremist content and political violence: Testing the relative strength of social learning and competing perspectives. Terrorism and Political Violence, 28(1), 1–29.
- Peer, E., Brandimarte, L., Samat, S., & Acquisti, A. (2017). Beyond the Turk: Alternative platforms for crowdsourcing behavioral research. Journal of Experimental Social Psychology, 70, 153–163.
- Perliger, A., & Pedahzur, A. (2011). Social network analysis in the study of terrorism and political violence. PS: Political Science & Politics, 44(1), 45–50. https://doi.org/10.1093/isq/sqv010
- Piazza, J. A. (2017). The determinants of domestic right-wing terrorism in the USA: Economic grievance, societal change and political resentment. Conflict Management and Peace Science, 34(1), 52–80. https://doi.org/10.1177/0738894215570429
- Pons, P., & Latapy, M.. (2005). Computing communities in large networks using random walks. In P. Yolum, T. Güngör, F. Gürgen, & C. Özturan (Eds.), Computer and Information Sciences - ISCIS 2005. ISCIS 2005. Lecture Notes in Computer Science (Vol. 3733, pp. 284–293). Springer. https://doi.org/10.1007/11569596_31
- Ravndal, J. A. (2018). Explaining right-wing terrorism and violence in Western Europe: Grievances, opportunities and polarisation. European Journal of Political Research, 57(4), 845–866. https://doi.org/10.1111/1475-6765.12254
- Robinaugh, D. J., Hoekstra, R. H. A., Toner, E. R., & Borsboom, D. (2020). The network approach to psychopathology: A review of the literature 2008–2018 and an agenda for future research. Psychological Medicine, 50(3), 353–366. https://doi.org/10.1017/S0033291719003404
- Rottweiler, B., & Gill, P. (2020). Conspiracy beliefs and violent extremist intentions: The contingent effects of self-efficacy, self-control and law-related morality. Terrorism and Political Violence, 1–20. https://doi.org/10.1080/09546553.2020.1803288
- Rousseau, C., Oulhote, Y., Lecompte, V., Mekki-Berrada, A., Hassan, G., & El Hage, H. (2021). Collective identity, social adversity and college student sympathy for violent radicalization. Transcultural Psychiatry, 58(5), 654–668. https://doi.org/10.1177/1363461519853653
- Schumpe, B. M., Bélanger, J. J., Moyano, M., & Nisa, C. F. (2020). The role of sensation seeking in political violence: An extension of the Significance Quest theory. Journal of Personality and Social Psychology, 118(4), 743–761. https://doi.org/10.1037/pspp0000223
- Shover, N., & Thompson, C. Y. (1992). Age, differential expectations, and crime desistance. Criminology, 30(1), 89–104.
- Taylor, M., & Horgan, J. (2006). A conceptual framework for addressing psychological process in the development of the terrorist. Terrorism and Political Violence, 18(4), 585–601.
- Torjesen, I. (2018). Can public health strategies tackle London’s rise in fatal violence? British Medical Journal Publishing Group, 361, https://doi.org/10.1136/bmj.k1578
- Tourangeau, R., & Yan, T. (2007). Sensitive questions in surveys. Psychological Bulletin, 133(5), 859.
- Trimbur, M., Amad, A., Horn, M., Thomas, P., & Fovet, T. (2021). Are radicalization and terrorism associated with psychiatric disorders? A systematic review. Journal of Psychiatric Research, 141, 214–222.
- van Borkulo, C. D., Borsboom, D., Epskamp, S., Blanken, T. F., Boschloo, L., Schoevers, R. A, & Waldorp, L. J.. (2015). A new method for constructing networks from binary data. Scientific Reports, 4(1), 14. https://doi.org/10.1038/srep05918
- Victoroff, J. (2005). The mind of the terrorist: A review and critique of psychological approaches. Journal of Conflict Resolution, 49(1), 3–42. https://doi.org/10.1177/0022002704272040
- Wikström, P. O. (2010). Situational action theory. In V. F. Cullen & P. Wilcoux (Eds.), Encyclopedia of criminological theory (pp. 1001–1008). Sage.
- Wiktorowicz, Q. (2004). Joining the cause: Al-Muhajiroun and radical Islam. The Roots of Radical Islam.
- Wilson, L. C., & Scarpa, A. (2011). The link between sensation seeking and aggression: A meta-analytic review. Aggressive Behavior, 37(1), 81–90. https://doi.org/10.1002/ab.20369
- Wolfowicz, M., Litmanovitz, Y., Weisburd, D., & Hasisi, B. (2020). A field-wide systematic review and meta-analysis of putative risk and protective factors for radicalization outcomes. Journal of Quantitative Criminology, 36(3), 407–447. https://doi.org/10.1007/s10940-019-09439-4
- Yang, Z., Algesheimer, R., & Tessone, C. J. (2016). A comparative analysis of community detection algorithms on artificial networks. Scientific Reports, 6(1), 1–18.