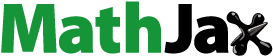
Abstract
An alternative perspective is presented for teaching students the logic and details underlying McNemar's test of the equality of correlated proportions. The new perspective enables straightforward extension to other correlated proportions situations.
1. Introduction
1 It should come as no great revelation to teachers of introductory applied statistics courses that often students do not see the conceptual forest for the computational trees. In a previous article (CitationSerlin and Levin 1996), we described a forest-seeking method that capitalizes on the more-intuitive binomial probability formula to elucidate the less-intuitive hypergeometric probability formula. A trick used for doing that was simply to encourage students to view the conventionally presented 2 × 2 frequency table from a different perspective. In particular, transposing the rows and columns of that table leads directly to a binomial-related approach to understanding the components of the hypergeometric formula. In the present note, we illustrate how the seemingly mysterious test of correlated proportions developed by McNemar (1947) can be viewed from an alternative, more comprehensible, perspective. Contemporary theoretical and empirical efforts in statistics pedagogy highlight the importance of relating new instructional material to students’ existing conceptual structures (e.g., CitationDerry, Levin, and Schauble 1995; CitationLajoie 1998). With the alternative McNemar perspective, generalizations of the procedure to more complex situations are straightforward and similarly more comprehensible.
2. McNemar’s Test of Correlated Proportions
2 In most beginning social-sciences statistics courses, standard hypothesis-testing fare includes the one-, two-, and correlated-sample tests of means, along with the one- and two-sample tests of proportions. Conspicuously absent from this list is McNemar’s test of correlated proportions, often referred to as McNemar’s test of “change.” More properly, however, the procedure represents a test of symmetrical change among those cases in which change actually occurred. Alternatively, and as will be illustrated here, the procedure can be conceptualized as a test of the equality of correlated proportions, even in “nonchange” situations.
3 Of what utility is such a statistical test? Researchers often employ dichotomous dependent variables in a pretest-posttest design to evaluate change or in a matched-pair design to assess the effectiveness of an intervention. Examples of common dichotomous variables of interest include yes-no attitude items and criterion- referenced measures or right-wrong items on a performance test. Of course, from dichotomous variables spring proportions, and just as it is of interest to measure change in mean performance over time, it is frequently of interest to assess changes in proportions (or percentages) over time. For instance, one might wish to document the percentage of prospective voters who change their views of a particular presidential candidate from February to November or the percentage of respondents who become more supportive of a controversial piece of proposed legislation following a statewide media blitz. In a nonrepeated-measures research context, one may wish to compare the performance of specially instructed participants with their matched-pair control counterparts on a dichotomously scored item or on a pass-fail mastery test. In that regard, it should be noted that in situations where matching has been employed, comparing the proportions of “successful” instructed and uninstructed participants via a two-sample chi-square test of homogeneity is not statistically appropriate – just as an independent samples t test would not be appropriate for assessing a difference in means between the two matched samples.
4 With a single sample and a two-period study as an example, the data are typically arrayed in a 2 × 2 table in which one margin represents the two Time 1 categories, the other margin represents the two Time 2 categories, and the test of the hypothesis of equal marginal probabilities is conducted using the McNemar test. Consider , which depicts, say, the number of students who solve a particular problem correctly both toward the beginning (Time 1) and toward the end (Time 2) of a mathematics course. If we let nij denote the frequency of observations falling in the ith Time 1 and jth Time 2 categories (i, j = 1, 2), and with common “dot” notation to represent summation, the total sample size is given by n··. The nij follow a multinomial distribution, and the null hypothesis can be written in terms of the marginal probabilities of interest, pi· and p·i, as:
(1)
Table 1. Table Representing a One-Sample Correlated Proportions Problem
2.1 Conventional Approach
5 With respect to , let the effective sample size be N’ = n12 + n21, the number of observations in the two “change” cells. For N’ ≥ 10, the chi-square distribution with 1 degree of freedom provides a reasonable approximation to the multinomial probability associated with the McNemar test statistic,
(A continuity correction, reflected in the numerator as (|n12 - n21| - 1)2, could be included to improve the approximation.) If this test statistic exceeds the alpha-level critical chi-square value, then it is concluded that the two marginal probabilities, pi· and p·i, are not equal, or in the case of , that the probability of solving the problem correctly is not the same on the two occasions.
6 In introductory social-science statistics texts that cover McNemar’s test – and many do not! – the test is typically described in terms of the just-presented form of the test statistic (see, for example, Ferguson and Takane 1989, pp. 200-202; CitationGlass and Hopkins 1996, pp. 339-340; and CitationHays 1994, pp. 865-866). From a pedagogical standpoint, that form of the test essentially comes “out of nowhere” and without a development that allows students to relate it to previously learned tests, such as the Pearson goodness-of-fit chi-square test.
2.2 Alternative Perspective
7 For the 2 × 2 table under examination, p1· = p11 + p12 and p·1 = p11 + p21, which means that the null hypothesis in (1) is equivalent to the hypothesis that the probabilities in the two change cells are equal,
This restated null hypothesis allows us to shift our focus from the margins of the table to just the two change cells. With attention restricted to those cells and with equal change cell probabilities specified under the null hypothesis, the N’ observations in the change cells are equally likely to fall into one or the other change cell. Accordingly, n12 follows a binomial distribution with sample size N’ and probability parameter p = 0.5. For N’ < 10, the binomial test will provide valid significance probabilities. For N’ ≥ 10, students are reminded that the one-sample test of proportions, H0: p = 0.5, can be conducted either as a normal distribution z test or as its squared equivalent, a one-degree-of-freedom chi-square goodness-of-fit test. (It can readily be shown that a statistical test that focuses on just the “change” cells is identical to a test of the equality of the corresponding multinomial proportions based on the entire table.) For the chi-square case, the test is presented in terms of the familiar “observed” (O) and “expected” (E) cell frequencies as applied to a 2 × 1 table consisting only of the two change cell frequencies (n12 and n21 in ). The goodness-of-fit test yields a value that is identical to that of the conventional McNemar test.
2.3 Example
8 We now illustrate both the conventional and alternative versions of McNemar’s test through actual data that were collected in the first author’s introductory educational statistics course, based on a total of 186 students over five semesters. The example provides an underused hypothesis-testing application of McNemar’s test, namely, assessing the equality of two proportions associated with two different dichotomous variables at the same point in time. For instance, one might wish to determine whether the proportions of respondents who agree with two different questionnaire items are the same, or whether two items on a test are of equal difficulty, as reflected by the proportion of test takers who get each item correct. In terms of the layout, if the focus were on only a single test occasion (e.g., the first test) and the “Time” label were permuted to read “Item,” one might wish to determine whether two different items are of equal difficulty.
9 As adapted to our statistics-course example, on the first day of class students are given a 10-item “arithmetic quiz” (essentially a simple algebra test) to assess their readiness for the course. The test consists of five pairs of items, with the items in each pair tapping the same underlying mathematical operation (i.e., the items comprising each pair are structurally equivalent) but presented in one of two different formats: symbolic or verbal. For instance, for one pair of items, the typical symbolically presented format is “Solve for p: 3/5 = p/250.” The corresponding verbal format for the same problem is “About two people in five across the country read Time magazine. How many people would we expect to read Time in a random sample of 200 people?” Thus, the second format includes exactly the same underlying structure and required solution operations as the first, but it is presented in a verbal context rather than symbolically. Each problem and its paired counterpart are randomly interspersed throughout the test, with separate total scores calculated for the symbolically and verbally presented problems.
10 In addition to the face value of assessing students’ entering mathematical skill as a predictor of their success in the course, the algebra test is used to illustrate a variety of principles related to hypothesis formulation, research design, and statistical inference. For instance, an intuitively understandable hypothesis relates to students’ often-reported states of “statistics anxiety” and “symbol shock”: Although most students possess the prerequisite mathematical problem-solving skills for the course, they will not demonstrate them as well on algebra questions presented in less familiar, more anxiety-producing terms (symbolic) as they will on questions presented in more familiar everyday contexts (verbal). If “mastery” is defined as correctly answering all five problems of a particular format (symbolic or verbal), one could ask: Do beginning statistics students demonstrate equivalent mastery of verbally and symbolically stated algebra problems? The results are summarized in . Applying the conventional McNemar formula yields X2 = (31 - 33)2/(31 + 33) = 0.06, which, based on a Type I error probability of .05, indicates that the difference in marginal proportions is not statistically significant. Thus, contrary to the research hypothesis, it would be concluded that the proportions of students exhibiting mastery of the two different problem formats, verbal (107/186 = .575) and symbolic (105/186 = .564), do not differ significantly. As an aside, the hypothesis was tested nondirectionally in this example because it is not difficult to construct an equally appealing rationale, with ample supporting evidence from the mathematics education literature, that symbolically stated problems are more direct and less confusing than structurally equivalent verbal “story problems.”
Table 2. Number of Students Demonstrating Mastery of a Set of Verbally and Symbolically Presented Algebra Problems
11 In contrast, with the alternative perspective, the data from the present example are recast into the 2 × 1 table in , based on just the N’ = 64 students who exhibited a difference in mastery for the two problem formats. With O1 = n12 = 31, O2 = n21 = 33, and E1 = E2 = (31 + 33)/2 = 32, X2 = [(31 - 32)2/32] + [(33 - 32)2/32] = 0.03 + 0.03 = 0.06, the same value as before. In addition, for the hypothesis H0: p = 0.5, a focus on n12, with expected value N’/2 and variance N’/4, leads to the squared z statistic, X2 = [(n12 - N’/2)2]/(N’/4). This latter formula easily converts to the conventional McNemar formula, because N’ = n12 + n21, n12 - N’/2 = (n12 - n21)/2, and the 22 and 4 cancel.
Table 3. “Change” Cells Extracted From
2.4 Comment
12 So what difference does it make which of these two formulas and associated perspectives is adopted? Pedagogically, we strongly recommend the latter, primarily because it: (1) maps directly onto students’ previously acquired binomial, chi-square contingency table, and goodness-of-fit test concepts; (2) provides consistency and continuity in extending the one-sample correlated proportions test to additional applications (as is demonstrated in Section 3); and (3) can be directly related to other similar statistical-test transformations that students will ultimately encounter (as is noted in Section 4).
3. Comparing Change in Two or More Populations
13 It is not uncommon in repeated-measures studies with dichotomous dependent variables for the researcher to obtain data from two or more samples. For example, one of the samples could be a control group and the other(s) treatment groups. In such experiments, interest focuses on the equality of the individual McNemar change parameters. Let k (k = 1, …, K) denote an individual’s group, and let pi·k and p·ik denote the marginal probabilities of interest in the kth group. If, in the kth group, the difference in corresponding marginal probabilities (the change parameter) is denotedΔik = pi·k - p·ik, then the null hypothesis of interest in the multiple-group design, expressing the equality of the change parameters, is
3.1 The Two-Sample Case
14 In the two-sample case, (1) the number of observations falling into each sample’s change cells is unknown prior to the experiment; and (2) in each of the populations from which the samples came, the extent to which the change parameter differs from zero is given by the relative probability of falling into one of the two change cells. Thus, comparing change parameters involves determining whether the relative probability of falling into one of the change cells is independent of group membership, when neither of the margins is fixed. That is, it involves a test of independence, and the appropriate test is the Fisher-Irwin (or “Fisher exact”) test.
15 To illustrate the various tests for the two-sample case, consider , in which some of the data are re-presented. Students taking the algebra test are also asked to think back to the college entrance examination that they took in high school and to indicate which of their two subtest scores was higher, verbal or quantitative. Another intuitively interesting hypothesis is that students’ differences in mastery of the two problem formats (verbal vs. symbolic) is related to students’ verbal vs. quantitative skill profiles. Of the 186 students taking the algebra test, 105 reported higher verbal than quantitative performance on their college entrance examination, 63 reported higher quantitative than verbal, and 18 either did not take such an examination or could not remember their relative performances. The joint frequency data for the 168 students who could be classified as either “higher verbal” or “higher quantitative” are summarized in .
Table 4. Number of Higher Verbal Students and Higher Quantitative Students Demonstrating Mastery of a Set of Verbally and Symbolically Presented Algebra Problems
16 Consistent with the alternative approach that was discussed for the one-sample case, the change-cell data are extracted and compiled into a separate 2 (verbal/quantitative profiles) × 2 (format combinations) table – see . The key here is to recognize that only the two change cells from each group are material in assessing the hypothesis of comparable change symmetry. Because the margins for the newly constructed 2 × 2 table are not fixed in advance, one can test the comparable change hypothesis using any procedure that is appropriate for an independence chi-square model (or, in the present two-sample case, a z test of the equality of two proportions). As was noted earlier, when that table is associated with relatively small expected cell frequencies, the appropriate statistical test is the Fisher exact test. To perform that test, all possible tables with the observed marginal frequencies must be generated. Then for each table with a change disparity at least as extreme as that in the observed table, one must calculate the corresponding hypergeometric probability. As a measure of change disparity, one can use the difference in the sample proportions, the sample phi coefficient, or the sample Pearson chi-square statistic, all of which are algebraically related in a one-to-one fashion.
Table 5. “Change” Cells Extracted From
17 Alternatively, with large expected “change” cell frequencies, the traditional Pearson chi-square test of independence may be used as a convenient approximation. Additional large-sample approximate procedures include the likelihood ratio X2 test, a test based on multinomial proportions (CitationMarascuilo and Serlin 1979), a test using log-odds ratios (Marascuilo and Serlin 1988, pp. 710-713), and the test of CitationBishop, Fienberg, and Holland (1975). For the data in , with their sufficiently large expected “change” cell frequencies (all are found to be greater than 5), the one-degree-of-freedom Pearson X2 (based on the familiar O - E formula) is equal to 8.18, with an associated significance probability of .004. Based on a Type I error probability of .05 and with reference to the actual data, this indicates that problem-format mastery is related to students’ skill profiles, in the expected direction: for higher verbal scorers, 55/105 (52%) mastered the verbally presented problems, in contrast to 47/105 (45%) who mastered the symbolically presented problems, for a verbal-symbolic difference of 7%; whereas for higher quantitative scorers, the respective values are 43/63 (68%) and 55/63 (87%), for a verbal- symbolic difference of -19%. The difference in these two differences, which is what the two-sample McNemar test is testing, is 26%.
3.2 More Than Two Samples
18 Extension to the K > 2 group situation is straightforward, as we illustrate by extending the present example. It may be recalled that 18 students could not be classified as scoring higher on either the verbal or quantitative portion of their college entrance examination. With these students included as an “unknown” third category, the frequency outcomes are summarized in (complete data) and (“change” cells).
Table 6. Number of Higher Verbal, Higher Quantitative, and Unknown Students Demonstrating Mastery of a Set of Verbally and Symbolically Presented Algebra Problems
Table 7. “Change” Cells Extracted From
19 For the data in , a Pearson chi-square test of homogeneity yields X2= 12.88 based on 2 degrees of freedom, with an associated significance probability of .0016. Because the expected cell frequencies associated with the unknown category are less than 5, an extension of the Fisher exact test was also conducted using the computer program developed by CitationKlotz and Teng (1975) and yielded a significance probability of .001.
20 Familywise α controlled planned post-omnibus between-group statistical comparisons of proportions (e.g., Marascuilo and Serlin 1988) can be conducted in the two-degree-of-freedom situation with the Fisher LSD procedure (CitationLevin, Serlin, and Seaman 1994), as applied to either z or chi-square statistics or to Fisher exact comparisons, depending on sample size. For the present three-group example, Fisher’s LSD procedure combined with Fisher exact comparisons (based on a familywise α of .05) indicate that there is a difference in the problem- format outcomes of higher quantitative students (symbolic format = 55/63 students, or 87%; verbal format =
43/63, or 68%, for a symbolic-verbal difference of 19%) and both higher verbal students, as in the two-sample case (47/105 = 45% and 55/105 = 52%, respectively, difference = -7%) and unknown students (3/18 = 17% and
9/18 = 50%, respectively, difference = -33%).
21 As an interpretive comment, students in the statistics course are alerted to the possibility that numerous demographic and experiential factors in addition to (or other than) the posited mathematics anxiety or relative verbal and quantitative skills could readily contribute to the results, including those related to students’ native language, gender, and the like. For example, foreign students in the class may have had a more difficult time comprehending or interpreting the verbally stated questions than the symbolically stated ones simply because of their English language limitations.
22 For more than two-degree-of-freedom situations, other familywise α controlled multiple-comparison procedures may be employed (CitationLevin et al. 1994). In addition, (1) Klotz and Teng’s (1975) program can be used to perform exact tests of planned or post hoc, simple or complex, contrasts among the change-cell proportions; and (2) when applied to specified contrasts, the approach introduced here also permits straightforward investigation of main effects and interactions in multiple-factor contingency tables.
4. Summary
23 In this note we have introduced a comprehension-enhancing alternative perspective for the McNemar test of change – or, more precisely, the multinomial test of correlated proportions. The present perspective allows for direct extensions to two or more samples in which questions of comparable marginal outcome probabilities are of interest. Of additional note is that the present McNemar simplification maps directly onto analogous ones that students can be taught in the context of one-, two-, and K-sample tests of correlated means based on t and F distributions. Comparing two or more different treatments in terms of pretest-posttest differences, as a simplification of the K × 2 split-plot ANOVA treatment by time interaction, is but one example of such mapping (e.g., CitationLevin and Marascuilo 1977). Indeed, one-sample, two-sample, and three-sample correlated t and F tests applied to students’ actual verbal and symbolic test scores in the present example (rather than dichotomized “mastery” outcomes) produced statistical conclusions consistent with those already discussed.
Acknowledgments
Both authors contributed equally to this note. The constructive feedback provided by three anonymous reviewers of an earlier version of the manuscript was appreciated and incorporated.
References
- Bishop, Y. M. M., Fienberg, S. E., and Holland, P. W. (1975), Discrete Multivariate Analysis: Theory and Practice, Cambridge, MA: MIT Press.
- Derry, S., Levin, J. R., and Schauble, L. (1995), “Stimulating Statistical Thinking Through Situated Simulations,” Teaching of Psychology, 22, 51–57.
- Ferguson, G. A., and Takane, Y.(1989), Statistical Analysis in Psychology and Education (6th ed.), New York: Mc Graw-Hill.
- Glass, G. V., and Hopkins, K. D.(1996), Statistical Methods in Education and Psychology (3rd ed.), Needham Heights, MA: Allynand Bacon.
- Hays, W. L. (1994), Statistics (5th ed.), Orlando: Harcourt Brace.
- Klotz, J. H., and Teng, J. (1975), “One-Way Layout for Counts and the Exact H Test With Ties, “Technical Report 407, University of Wisconsin - Madison, Department of Statistics.
- Lajoie, S. P. (ed.) (1998), Reflections on Statistics: Learning, Teaching, and Assessment in Grades K-12,Mahwah, NJ: Erlbaum.
- Levin, J. R., and Marascuilo, L. A.(1977), “Post Hoc Analysis of Repeated Measures Interactions and GainScores: Whither theInconsistency?,” Psychological Bulletin, 84,247–248.
- Levin, J. R., Serlin, R. C., and Seaman, M. A. (1994), “A Controlled, Powerful Multiple-Comparison Strategy for Several Situations, “Psychological Bulletin, 115, 153–159.
- Marascuilo, L. A., and Serlin, R.C. (1979), “Tests and Contrasts for Comparing Change Parameters for a Multiple Sample Mc Nemar Data Model,” British Journal of Mathematical and Statistical Psychology, 32, 105–112.
- Marascuilo, L. A., and Serlin, R.C.– (1988), Statistical Methods for the Socialand Behavioral Sciences, New York: Freeman.
- Mc Nemar, Q. (1947), “Note on the Sampling Error of the Difference Between Correlated Proportions or Percentages,” Psychometrika,12, 153–157.
- Serlin, R. C., and Levin, J. R. (1996), “Two Alternative Developments of the Hypergeometric Formula: Turningthe Tables,” Journal of Statistics Education, [ Online], 4(2). (http://www.amstat.org/publications/jse/v4n2/serlin.html)