Dennis Pearl is Professor of Statistics at Pennsylvania State University and Director of the Consortium for the Advancement of Undergraduate Statistics Education (CAUSE). He is a Fellow of the American Statistical Association. This interview took place via email on November 18–29, 2016.
Beginnings
AR: Thanks very much, Dennis, for agreeing to this interview for the Journal of Statistics Education. Let's start with the 18-year-old version of you. Where were you then, and what were your thoughts about education and career goals at that point?
DP: That would be 1969. I was a sophomore at Berkeley, protesting the Vietnam War and discovering that I was no longer the smartest guy in the class like I was used to be in high school. I had a terrific AP calculus teacher in high school and figured I'd be a math major, go on to graduate school, and become a math professor. Unfortunately, I had a pretty boring instructor in my linear algebra class at Berkeley and the same guy was going to teach the next course in the series, so I decided to shop for something else. My brother was a senior there and convinced me to take an introductory statistics class with him taught by Erich Lehmann. By the second week I was hooked! My math classes were about understanding elegance while statistics was about understanding evidence and it just seemed that the world needed more people that could do the latter.
At that point, I didn't think much about education except noting which qualities I liked in an instructor and which I didn't. I liked organized professors like Lehmann, witty professors like Elizabeth Scott, professors that kept their class involved in discussions like David Freeman, professors that had the class construct their own knowledge like Jerzy Neyman, and friendly professors like Kjell Doksum. Then, there was David Blackwell, who was in a class by himself, as the quintessential explainer of complex things and who also had all of the traits mentioned above. The Berkeley Statistics Department had a lot of great teachers, and all of them gave me a feel for the relevance of the subject. I had the opposite feelings about the math instructors I encountered and so I became a Stat major.
AR: My, that's quite a list of professors that you had as an undergraduate. What did you do after you earned your bachelor's degree, and how did you make that decision?
DP: Oops—I only had Neyman as a grad student—but his teaching style was unique. He just stayed seated and asked random students to go to the board and work things out. If you were lost, he called on someone else—but after a few sessions every student worked like crazy to be prepared!
At the end of spring quarter in 1971, I had the units to graduate and I had no doubt that I was going to grad school in statistics—but felt like I needed to slow down and possibly take some time off (I had only just turned 20 years old). So, I postponed my graduation until after fall quarter and luckily was selected to take part in an NSF-supported research program organized by an engineering professor that turned out to be a life-changing experience. It involved eight senior undergrads: two from math, two from statistics, two from engineering, and two from ecology. Our task was to model fire and its effects. We had access to four farmers in California who were doing controlled burns on their property. The math students worked out differential equations on how the fire might spread; my teammate and I in statistics designed the experiments to collect data and then worked to analyze it; the engineering students devised the instrumentation to measure stuff; and the ecology students made predictions about the effects on the non-plant organisms in the burn area. At the end of the summer, I was picked to go to the national AAAS meetings in Philadelphia to present our findings, where I heard talks by Carl Sagan and met Margaret Mead. That summer I stopped thinking about statistics as a branch of mathematics and realized that what I wanted in life was a career as a Statistical Scientist, with the emphasis on science.
In my final quarter as an undergrad (Fall 1971), I asked David Blackwell if he would supervise me in a reading course to attempt to model the spread of fire using ideas from game theory, so I was able to continue that experience with my teaching idol. From January until grad school started for me in September 1972, I spent a little time working to have enough money to travel around Europe, and I met my wife in Jerusalem (so my second life-changing experience in one year).
AR: That was quite a year! I hope you haven't averaged two life-changing experiences per year since then, because that would probably be too much change. You stayed at Berkeley for graduate school, right? Did you consider going elsewhere?
DP: Yes—I stayed in Berkeley for another 10 years and never considered anyplace else for grad school. I was a T.A. for Freeman, Pisani, and Purves (2007) as they wrote their classic introductory text. I became involved in studies of molecular evolution when the field was just new. I worked on a congressionally mandated study of the effects of ozone depletion on human health (my dissertation was about a stochastic model of skin cancer), on studies of law school admission policies toward women and minorities, and on studies of the workings of the subconscious brain. ResearchGate.net tells me I'm at about 7500 citations now, and probably a good quarter of them came from my work as a grad student. So things were pretty interesting in my teaching, research, and collaborative work, while during those years our three children were born and my wife and I were reasonably active politically. I guess I slowed down to averaging perhaps a little under one life-changing event per year!
AR: Was teaching a strong interest of yours at that point, or just something you had to do in addition to your research? Did you have opinions about the FPP text/course then; did you have input into its development?
DP: Well, I loved teaching and wanted to be better at it, but I wasn't really thinking systematically about it. We would have weekly TA meetings and Freeman, Pisani, and Purves—but especially Freeman—would talk about the big ideas of the week and tell us how to remove the mathematics and just relay the core concepts of the discipline in English. I participated in the discussions, but I was just learning from them and not really contributing. After I had taught the class once, I started speaking up more, about the details of the logistics of running the recitation sessions, to let the new TAs know what seemed to be working for me. It wasn't really until I got to Ohio State, and was coordinating TAs of my own, that I started to take a more scholarly approach to teaching.
AR: Did you go to Ohio State directly from Berkeley? Did you also consider nonacademic opportunities?
DP: Yep—straight to Ohio State. OSU Statistics had been given five new positions and the chair of the department, Jagdish Rustagi, was visiting Berkeley and Stanford. He offered me a position based only on the recommendation of my advisor (Elizabeth Scott) and a ten-minute conversation with me. I never thought about nonacademic opportunities, and the OSU position sounded great, so we moved to Columbus without so much as an on-site interview. Things worked differently then.
AR: I assume that research was the top priority for new faculty, but what were your teaching assignments in the first few years, and how did you approach them?
DP: For my first couple of years at OSU my teaching centered on graduate courses in discrete data, probability courses for engineers, and coordinating the large FPP-based intro course. For the first two, I worked hardest at developing examples that would engage the students and at trying to make classroom discussions go beyond the same four or five top students. For the intro course, I worked hardest at coordinating the TAs, holding regular meetings with them, developing activities for them to do in recitation, and being sure they had what they needed to do a good job. By 1984, I started to work on changing from a lecture/recitation format to a lecture/lab format. I created an “honors” section of the course and wrote a lab activity manual for it. I wrote a grant to start the first computer lab for teaching in the Arts & Sciences at OSU and won that, so I was doing computer labs starting in 1985. Paul Velleman had just created DataDesk and I wanted to use it a bit for exploratory analyses, but mostly for doing simulations to illustrate concepts.
Statistical Buffet
AR: Two of your education projects at Ohio State for which you received national recognition and acclaim were the Electronic Encyclopedia of Statistics Examples and Exercises and the Statistics Buffet. Which came first, and please tell us about that project.
DP: The Encyclopedia project (EESEE—pronounced “easy”) came first by about a decade. I worked with Elizabeth Stasny and Bill Notz at OSU and with Paul Velleman at Cornell on a series of NSF projects to build technology resources for instructors. At OSU, we created EESEE that included examples with background information, the protocol, the dataset, student exercises, and instructors notes. The collection grew to several thousands of pages of materials as we continued to work on it for about 20 years. David Moore had W.H Freeman include it as an electronic supplement to his textbooks, so we were able to sustain the project a good deal after the NSF funding ended. (There's a Flash version from midway in the project that is still freely accessible at www.macmillanlearning.com/catalog/static/whf/eesee/eesee.html.) Meanwhile, Paul had a great vision for where things were going technologically and translated our content into a web product he called DASL (Data And Story Library).
AR: What was the genesis of the Statistics Buffet project?
DP: I guess the genesis of that project would be in the successes and failures of the 1990s.
Jeff Witmer was using some of my labs at Oberlin in the late 80s and showed them to Dick Scheaffer, so I joined the advisory board for Dick's Activity-Based Statistics NSF project. There I met Joan Garfield, George Cobb, Bob Stephenson, Judith Singer, Jim Landwehr, Ann Watkins, and Don Bentley, and a couple other stellar people I apologize to for not remembering at the moment. I became plugged into the broader Stat Ed community and I learned a great deal about what works and what doesn't from that outstanding group.
Back at OSU, Mark Berliner and I wrote the university's General Education Data Analysis requirement, and we included the idea that acceptable courses had to have a statistical modeling component and also feature the use of technology in analyzing data or illustrating principles. Those went into effect in 1991, and our annual enrollment swelled from 1000 to 3000 students per year in the course I coordinated. I also joined the committee that designed a new teaching building for the mathematical sciences and was able to create a large computer lab complex to meet our course needs.
So, by the mid-90s I had an infrastructure in place with facilities, systematic T.A. training, and systematic data collection about the course; we had hands-on, simulation, and data analysis labs; a nice repository of examples; and a bunch of neat ideas that I saw coming out of the growing Stat Ed community. The good news was that students as a whole were enjoying the class more and seemed to be learning more, with the success rate having risen from about 70% in 1991 to 80% in 1995. The bad news was that, no matter what I tried, there always seemed to be that 20% of the class that did not succeed (grade C- or lower), give or take 5% from section to section. I continued to tweak things for the remainder of the decade and had some small successes and failures, but the overall picture stayed the same. Then around 2000, Joe Verducci was running our summer T.A. training course and pointed me to Richard Felder's work on learning styles. After reading a couple of his papers, I began to feel that optimizing for the group average could only take us so far and that we needed to be thinking more about optimizing for the individual, so I came up with the broad outline of an individualized education plan that I called the Statistics Buffet and pitched it to the Provost's office in a local grant competition.
AR: Before I ask how the Statistics Buffet worked, I'm curious about the GE requirement you mentioned. Most colleges and universities that I know of have a quantitative GE requirement for which either a mathematics or a statistics course will suffice. But you instituted a GE requirement specifically for data analysis at OSU? Did that take a political battle, and how did you win? Did many departments offer courses that would satisfy the requirement?
DP: I think in many places such fights are between Mathematics and Statistics interests, who are both vying for credit hours. In our case, Bostick Wyman in the Mathematics Department was either chair or vice-chair of the university-wide committee and was an ally of Statistics. He approached Mark and me, saying that he was arguing for a Gen Ed Data Analysis requirement and asked us to write up what that should look like. To our surprise, they just took our paragraph and plugged it in without any pushback. For about a decade very few other departments offered competing courses, so we had more than 90% of the students (Psychology taught a course for their majors, and Chemistry and Physics were exempted provided they included data analysis as a component of their lab courses). There's been lots of leakage since then, as data analysis became a more and more important component of many areas, and the Gen Ed was also substantially revised when OSU changed to the semester system five years ago.
AR: Very interesting, thanks. Now back to the Statistics Buffet—how did that work?
DP: In one lab section, students would be generating the data for their regression lab by doing a quick hands-on experiment (e.g., timing how long it takes, Y, to complete a connect-the-dots puzzle of different lengths, X), while in the room next door a dataset from the EPA might be used for the lab. Or in a sampling distribution lab, one room has students generating their data by hand and comparing results from student-to-student, while the room next door has students using simulated data from an applet. For many students, generating data by hand helps them form a better connection to statistical concepts, while other students find such activities to be disconnected “busy work.” In the Buffet model, students had a choice as to whether they'd like their labs to include more hands-on data generation activities versus more simulation-based activities. In lecture, some students would be doing some kind of group activity each session like coming to a solution with a few others in adjacent seats, while in the lecture hall next door students would face individual contemplation of questions (e.g., a clicker question). Our Buffet model gave students a choice as to whether they preferred more group activity in lecture. Similarly, students could choose whether they preferred a weekly on-line problem-solving format or an instructor-facilitated problem-solving format. Logistically, students signed up for a lecture and lab time as usual. Then, in the first week of the term they took Felder's learning styles inventory and Tuckman's school strategies inventory and were given advice by software as to what choices we thought would be best for them—but they could ignore that advice and choose whichever learning path they wanted amongst the 23 = 8 choices. Since we had two lectures scheduled simultaneously (and three labs scheduled simultaneously), we could just assign students to the appropriate rooms.
This structure was wrapped in the Savion and Middendorf (Citation1994) EEGP learning model designed to enhance comprehension and retention by providing, for each of our explicit course learning objectives
an Example in the students' own life,
an Example outside that sphere to show that the concept extends to many circumstances,
the Generalization or concept at hand, and
Practice in applying the concept.
Meanwhile, course assessments were the same for all students to keep things “fair.” For example, even though students did different labs and had a different lecture experience, we assigned a weekly report that was the same for all students—it simply asked “How was learning objective xyz illustrated in your lab, how was it illustrated in your lecture, and how was it illustrated in the homework?” That also helped to show students how different components of the course tied together.
AR: How did you assess whether the Buffet was effective, and what did you learn from that?
DP: We primarily used a variety of within-course measurements. The Buffet was piloted in spring 2002 in half of the course sections and then became the standard for all sections in fall 2002. The same final exam was used for the Spring, Fall and Winter quarters before the pilot, during the pilot, and for the year following. Scores (total and by various subsets of learning objectives) were examined in conjunction with data on student preparedness drawn from OSU's data warehouse like High School GPA, OSU GPA, ACT/SAT percentiles in Math, along with other student characteristics such as gender, age, and race. Overall, students did about 5% better under the buffet model than under the previous model (half a letter grade). We were happy to find that grades on tests and class assignments were independent of Buffet choices and that the measured benefit of the Buffet model was independent of preparedness, gender, age, and race. Further, the 5% overall improvement was only about 3% for learning objectives taught the same way in all sections compared with a little over 7% for the learning objectives that were taught differently in different sections. The improved test scores translated into a rate of C- or worse (D, F, or Withdrawal) that went from 20% down to 12% and then continued to go down linearly until it reached around 6% in 2010. That's important because that meant several hundreds of students didn't have to repeat the course and pay the extra associated tuition or suffer an extension in time to graduation. Also, section-to-section variability in the success rate was reduced from about 5% pre-Buffet to 1–2% afterward.
Beginning in the 2005–2006 school year until I left OSU in 2014, the course was also assessed using a more structured set of nationally normed inventories like the Statistical Thinking And Reasoning Test (START) from Joan Garfield's group at University of Minnesota, Candace Schau's Student Attitudes Towards Statistics (SATS) inventory, and Morgan Earp's Statistics Anxiety Measure (SAM). But those were used more to inform other projects.
AR: Does the Buffet approach continue to this day at OSU? And do you know of other institutions that have adopted it?
DP: When I left OSU in 2014, the department hired Michelle Everson, so the course was turned over to very capable hands. I think it is important to completely redesign every course about once a decade, and I 'm sure she's doing a great job in building on my efforts. The Buffet redesign was supported by a grant from the Pew Program in Redesign run by the National Center for Academic Transformation (NCAT). NCAT is a very effective organization in helping programs, institutions, and systems use technology to simultaneously increase learning and reduce costs (see www.thencat.org). Anyways, the Buffet model is one of the several redesign strategies they advocate and, as a result, it has been adopted at some other institutions (e.g., in Chemistry at Missouri University of Science and Technology; in Psychology at Chattanooga State; and in Nutrition at the University of Southern Mississippi).
Education Research
AR: I want to come back to ask about your leaving OSU later, but for now let me shift gears a bit. You were a member of the group that produced the report “Using Statistics Effectively in Mathematics Education Research.” Can you tell us about your involvement in that project?
DP: That was another Dick Scheaffer project (the final report is at www.amstat.org/asa/files/pdfs/EDU-UsingStatisticsEffectivelyinMathEdResearch.pdf). He brought together an interesting group of math educators, assessment and measurement people, educational statistics folks, and statistics educators to discuss the use of statistics in math education research. I contributed to several sections and to the follow-up meetings we had with NSF and others, but I think Ingram Olkin contributed the most interesting idea. He had worked on a very influential report back in the 1960s that succeeded in getting statistical issues more systematically addressed in medical studies. Because of him, that report (and ours) was written as guidelines on how to report studies rather than trying to dictate so-called best practices. Our report was also careful to lay out the different stages of an educational research program and how statistical issues should be aligned with the purpose(s) of each stage.
AR: Am I right that you have extensive experience working with clinical trials? Do you think the statistical/scientific principles of clinical trials are applicable to mathematics and statistics education research? Do you see any particular challenges or difficulties with applying ideas of clinical trial ideas to education research?
DP: Yes—I have done a lot of collaborative work in the medical area, especially in cancer studies, ranging from basic research to translational to clinical investigations. Good statistical practice applies everywhere, but obviously different applications have different traditions and different challenges arising from the subset of study designs and infrastructure available for use. With respect to medical versus education research traditions, I think both can learn from each other. Medical research always has its eye on the main target—improving human health—even when the research at hand is at the very basic level trying to understand how stuff works. I think sometimes education research “fails to see the forest for the trees” and ignores how things fit into an overall program that builds generalizable knowledge. On the other hand, good basic research in education will carefully define the concepts, the constructs used to approach them, and the measurement properties of the variables examined. Medical research sometimes “fails to see the trees for the forest” and often creates proxy variables from lab assays that they mistakenly examine for years before realizing they aren't really studying what they think they're studying.
Now our country spends about the same amount per person per year for education and medicine—but expenditures on medical research are about a hundred-fold higher than expenditures on education research—so that's one big challenge. Medical research has then created an amazing infrastructure to tap into (e.g., well-stocked labs, continuing databases, ongoing clinical and basic research collaboratives, common baseline measurements, and an army of postdocs and lab technicians) that helps them move quickly to build on successes when they get them. Education needs that, and I've been advocating for focusing on research infrastructure for decades. Luckily, the advent of online learning and automated data collection have dramatically expanded the types of study designs that can be used in education research and has reduced the cost of doing that research, so I see a really bright future for figuring out what works to improve student learning.
AR: It seems to me that one big difference between medical studies and education ones is that randomized experiments are much more common in medical studies. And when education studies use random assignment, that's often at the level of groups of students rather than individual students. Do you agree with this, and do you think randomized experiments will become (or perhaps already have become) more common in education studies?
DP: That's a little bit of a misconception. Randomized studies in clinical medicine dominate for phase 3 studies, but remember that there are dozens of basic science experiments (lab assay or animal experiments replicated but not commonly randomized) for every phase 1 human trial (safety and feasibility studies not commonly randomized), and five of those for every phase 2 experiment (often using historical controls), and five of those for every phase 3 trial. We hear about the randomized trials because those represent a focal point of a successful research program. Anyway, education research now has many more design options because of the learning that takes place online. Nowadays, we can readily randomize at the student level or at the learning objective level and eliminate instructor effects in education studies that examine online “interventions.” There are also better data analytic resources for handling large observational datasets that are having a big effect on medicine and hopefully will play a bigger role in education as we build the databases necessary for such efforts to be fruitful.
AR: Boy, your first sentence there is a very nice way to say that I was wrong with the premise to my question! Turning from education research in general to statistics education in particular, what do you see as the most pressing priorities for statistics education research in the next decade?
DP: As far as topic priorities for research, I think the report that I wrote with Joan, Bob, Randall, Jennifer, Herle, and Hollylynne about five years ago still holds up pretty well (Pearl et al. Citation2012), as long as you add in more ties to online teaching and using statistical approaches like simulation-based inference and Bayesian modeling. Anyway, there are many worthy topics, but I would still advocate that the top priority for statistics education research should be in building infrastructure. We need to have a couple of hundred institutions keeping a common set of baseline data on their students and as many as possible of those participating in ongoing administration of various nationally/globally normed instruments of student learning, attitudes, anxiety, motivation, etc. We should also be conducting probability samples of statistics instructors every year to keep track of the true state of things, and we should be giving an army of undergraduate and graduate students experience in doing research on the collected data. By investing in a culture of assessment, we can do quality studies of all types in half the time without constantly re-inventing every wheel.
AR: This sounds terrific, and I'd be delighted to see things transpire as you describe. I see that this report came from a research retreat held at ASA headquarters. Do you know if ASA or any other group has any initiatives currently underway along these lines?
DP: Yes—Joan and I received an ASA Member Initiative to have a couple of meetings that got things started on that report, and while she was ASA President Jessica Utts asked a group of us to revise the report in order to modernize it and extend it beyond undergraduate education. The group has only met virtually so far, and I hope we do complete Jessica's task this upcoming year. Regarding the research infrastructure ideas—I received a couple of small NSF grants back in 2007 and 2008 with Kathy Harper, a colleague in Physics Education, to pursue demonstration projects in defining the database areas across all STEM disciplines (the WISER and INQUERI projects). People in different disciplines were excited about the ideas of the project, and we came to a general agreement about the administrative structure—but we had trouble finding the sustainable funding to pull it off. I also received a couple of grants in 2008 and 2010 with Joan Garfield to demonstrate the feasibility of the idea of doing a random survey of statistics instructors (the e-ATLAS projects). For the random survey, Joan's group developed an instrument to study how statistics is taught at the college level, while my group developed the probability-based sampling plan and drew a random sample of about a hundred instructors. We also drew a non-probability sample of about 300 participants so we could gauge the differences between a typical volunteer sample and a true probability-based sample of instructors. Much of this is becoming cheaper to do over time and so I do want to build on these efforts. I agree with you that working with the ASA is the appropriate route to make it sustainable.
Consortium for the Advancement of Undergraduate Statistics Education (CAUSE)
AR: That's great that progress is being made on this front. Now I'd like to ask directly about a topic that we've skirted thus far: your serving as Director of the Consortium for the Advancement of Undergraduate Statistics Education (CAUSE). How did you come to take on this role, and what appealed to you about it? (I suspect that readers are expecting to see “this was another Dick Scheaffer project” in your response.)
DP: Actually, it was Joan Garfield and Deb Rumsey who received funding from ASA (but that was when Dick Scheaffer was ASA President, so the theme continues!) to hold a series of meetings about starting some kind of national undergraduate statistics education effort. I'm including a picture from the July 2001 meeting. We decided on a basic structure with a Director overseeing the organization and three sub-components (Research, Professional Development, and Resources) each supervised by separate Associate Directors. Deb was going to be the Director, Joan would be the A.D. for Research, and Beth Chance and you would share the role as A.D. for Professional Development. Also, we hadn't settled on an AD for Resources though Jackie Dietz (who had just finished her successful stint as Founding Editor of JSE) was considering taking on the job. As things turned out, Deb had a new baby and Jackie declined, so new team members were needed for those responsibilities. Joan called and asked if I would take on the role of Director since I had been active at the meetings. I agreed to try and work out a plan to do that and make it feasible to move the organization forward. I asked Rog Woodard, who was then at OSU and working closely with me on the Buffet project, to be the A.D. for Resources, and asked Doug Wolfe as Chair of OSU Statistics if the Department could provide a course relief for Rog and I to take on those jobs. Everyone agreed and we began to work.
Back Row: George Cobb, Allan Rossman, Roger Hoerl; Middle Row: Beth Chance, Mary Parker, Deb Rumsey, Jeff Witmer, Bill Notz, Gale Rex Bryce; First Row: Joan Garfield, Paul Stephenson, Dennis Pearl, Rog Woodard.
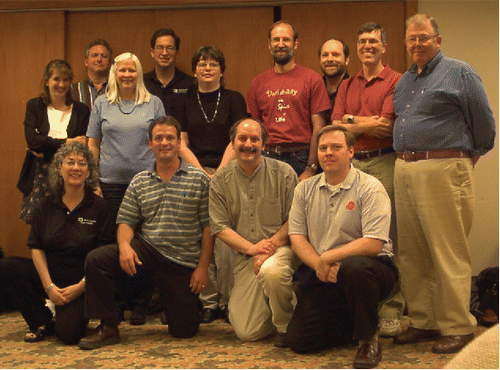
AR: What were some of the early challenges, and also some of the early success, for CAUSE?
DP: The challenge to me was to put together the financial and staff resources to get things done. I was confident I could do that—but my biggest fear was that I wouldn't be able to get people interested in working on projects. Happily, there was lots of good will toward CAUSE in the statistics education community and I had an ace in the hole—Joan Garfield. Joan knew everyone and was respected by everyone. She gave me the names of folks to ask for help and, for the research oriented things, she asked them herself—to my amazement almost everyone said yes. Now, at that point Lee Zia at NSF had created a wonderful vision for a National Science Digital Library (NSDL) that would be a repository of resources for teaching and learning in the STEM disciplines. So, I worked with Rog Woodard in putting together a proposal for CAUSE to operate the statistics education NSDL and CAUSEweb was born with Rog as the editor and Ginger Rowell as the Associate Editor and with Justin Slauson hired as our web programmer. The NSF reviewers said that the editorial board that we assembled from Joan's recommendations was a “Who's Who of Statistics Education.” I agreed.
We also had a couple of other big successes in our first five years that helped propel things to the next level. Working with Tom Short, we received a major workshop grant (the CAUSEway program) enabling us to provide a series of about 30 multi-day workshops that were free to the participants. Then, working with Deb Rumsey, we launched the NSF-funded CAUSEmos program that supported three USCOTS conferences, provided funds for eight faculty-learning communities, and allowed us to hire Jean Scott as our program coordinator to handle the logistics of it all. Jean really became the face of CAUSE over the next eight years.
AR: I suspect that this will be the most softball question of the interview: For someone who has never been to the CAUSE website or participated in a CAUSE-sponsored activity, how would you recommend that they get involved, and why should they want to get involved? But let me make this question a bit harder by asking you to address three different groups: those for whom statistics teaching is their primary professional activity, undergraduate instructors in other disciplines such as mathematics or social sciences who occasionally teach statistics, and education researchers interested in statistics education.
DP: If you are a nonstatistician teaching undergraduate classes that involve statistical concepts then go to www.CAUSEweb.org to find a unique collection of songs, cartoons, well-researched quotes, and other fun items for teaching elementary statistical concepts; or find ways to connect your teaching to real-world applications though Chance News hosted on CAUSEweb.
If you are an education researcher interested in getting involved in statistics education research, go to CAUSEweb to find a searchable annotated index to the literature; national reports providing guidelines for programs, research topics, and methods; and links to appropriate assessment items and inventories in statistics education research.
If your career is in teaching statistics, then come to CAUSEweb to hear recordings of nearly 200 webinars and 100 hours of archived virtual conference sessions on the teaching and learning of statistics; come to share your own teaching tips and browse links to collections of on-line and hands-on activities and technological resources for teaching; find out how your students can take part in national undergraduate research competitions (USPROC) or in monthly cartoon caption contests; come to add your name to the map of people in statistics education and find others in your area; come to find out about how to implement new ideas in pedagogy like teaching statistical inference using simulation.
If you are in any of these groups, then come to CAUSEweb to sign up for our eNEWS that will keep you informed about professional development opportunities like webinars, workshops, and conferences on teaching statistics and to hear about the activities of the latest statistics education projects funded by NSF. And this is just a sampling. CAUSEweb is meant to be both a portal for anyone interested in the teaching and learning of statistics at the undergraduate level and a link to a great community of like-minded people.
AR: You mentioned “fun” items such as cartoons in this response. You've done a good bit of work (although that seems like an odd word choice here) on incorporating “fun” into teaching statistics. Can you summarize what you've done and learned on this topic?
DP: Yes—I've “worked” with Larry Lesser from University of Texas at El Paso (UTEP) since the start of CAUSEweb in 2003 on the development of our collection of fun resources for teachers. Then, as part of a CAUSE faculty learning community on teaching with fun, we met John Weber from Perimeter College of Georgia State (GPC) and started to do more systematic research in the area. We received an NSF grant in 2012 (project UPLIFT) to do randomized experiments of the effect of fun teaching on learning and student anxiety and also to remove some barriers that instructors had in using the resources in the CAUSEweb collection (e.g., providing high-quality recordings of songs). Results from our randomized trial were recently published in JSE (Lesser, Pearl, and Weber, Citation2016). It showed that students who were asked to listen and sing along with songs illustrating specific learning objectives got the correct answer on test items about those objectives an average of 7.7% more often than students who learned without the songs. Also, just last year, we received another NSF grant (project SMILES) that we are quite excited about. We are creating a large group of interactive songs that we are testing in new experiments. The interactive songs work kind of like MadLibs, where students are asked questions and then the words they use in their answers become part of the song via a synthetic voice. We hope that the increased student engagement required will boost the learning gains provided by songs.
Moving to Penn State
AR: Let's get back to your biographical story. A few years ago you left Ohio State and moved to Penn State. What was your motivation behind that move?
DP: I retired from OSU in 2013 just before a series of very negative changes in the Ohio retirement system were to take place. As might be expected, I wasn't very good at being retired and, because I was now an Emeritus Professor, the OSU Statistics Department could no longer provide any support for my efforts with CAUSE. On the other hand, Penn State was looking to build a stronger presence in statistics education and had the resources to do that. They were hiring Kari Lock Morgan as an Assistant Professor, and as part of hiring me, they were able to provide partial ongoing support for USCOTS and for Lorey Burghard, Kathy Smith, and Bob Carey, our new CAUSE staff. Leaving friends and collaborators at Ohio State was a tough decision—but this new opportunity was too good to pass up.
AR: Are you teaching classes at Penn State, as well as directing CAUSE and continuing your research program? If so, what kinds of courses are you teaching?
DP: I do teach a regular load. I teach an introductory statistical concepts course completely online as well as face-to-face. Those courses give me a laboratory for my fun teaching research. I'm also really enjoying teaching fully online and hope to share some of my work in that setting in an USCOTS poster next year. Next, I teach upper division applied probability, which has been another interest of mine over the years—I've collaborated with Ivo Dinov at University of Michigan and Kyle Siegrist at University of Alabama on developing resources for that course as part of the NSF funded Distributome project. Finally, every-other-year I facilitate a graduate course on Statistics Education (first offering was last spring). That course is part of the graduate program at University of Minnesota and I worked with Bob delMas and Liz Fry on providing a Penn State version where we discuss the articles of the week at PSU for an hour and then hook up virtually with their class when they have invited visitors. Bob and Liz did a great job in setting up an exciting class and our ability to piggy-back off of their hard work allowed us to create a quality experience for PSU students and faculty.
AR: I'm tempted to ask about what's different in your teaching now as compared to the beginning of your career. I reserve the right to ask that later, but I'm more interested in hearing what you think has not changed in your teaching over the years.
DP: Wow! That really is the tougher question since I started teaching in the pre-computing, pre-internet era. I guess one constant is that I've always taught through applied examples. Even when I've taught Master's and PhD statistics courses, I have never introduced a statistical idea to a class without putting it in the context of an application. If there's no application—I don't see the point and I wouldn't expect my students to either.
AR: Speaking of examples, what are some of your favorites, for teaching whatever statistical idea you care to mention here?
DP: Most of my favorite examples vary from term to term since I like examples that come straight from the news—especially those with interesting confounders. One regression example that I've used for decades involves an experiment that one of my introductory statistics students did in 1986 to satisfy a class project requirement I had. She put the numbers one to nine on tickets in a hat and had each of 16 volunteers from her dorm select a ticket at random (with replacement) and then drink that many beers (e.g., pick the number 5 and you drink 5 beers). Thirty minutes after consuming the alcohol, a police officer administered a breathalyzer test to determine the blood alcohol content of the volunteer. Finally, she also included the weight and sex of each student in the dataset and had the officer take everyone's BAC at baseline to confirm they were at zero at that point. This was back when the drinking age was 18 in Ohio and it was easier to get approval on the human subjects issues—so please don't try this at home! Anyways, the resulting data is wonderful to use for regression (How does the number of beers X affect BAC Y?), for multiple regression (How does the weight of the subject affect the prediction of Y based on X?), and for inference (Is the affect of drinking on BAC greater for women than for men, even after accounting for weight?). It also makes for good discussions of assumptions since the plot of BAC vs. beers is very linear within the x = 1 to 9 range but would clearly not be linear as you move in either direction and also the fact that students drew the numbers at random means we can assume that X is independent of Gender/Weight. We put the data set into EESEE so it's publicly available.
Pop Quiz
AR: Now let's begin the “pop quiz” portion of the interview, where I'll ask a series of questions and request that you keep your responses to just a few sentences or less. First, please tell us about your family.
DP: I'm lucky to have both of my parents still alive at ages 94 and 95 and still living in their own home in the Seattle area. Barbara and I have been married for 44 years and have three great kids, two great son-in-laws, and five wonderful grandchildren (so far). Our youngest daughter's family in Philadelphia is just a three-and-a-half hour drive or train ride away, but we have to go out to California to see everyone else. So, if anyone out there wants to invite me to give a talk in the Sacramento area, I'm yours!
AR: Please name some (nonstatistics) books that you've read recently.
DP: I think I'm going to fail the pop quiz since I rarely read much in the way of fiction, except when I read to my grandchildren, which is about every time I see them. A few days ago I read Shel Silverstein's The Giving Tree to my three-year old grandson. He loves trees and gets mad when the boy in the story grows up and chops down the tree to build a house and then a boat. Every kid I know, including me, loves Goodnight Moon by Margaret Wise Brown so I've probably read that a hundred times over the years.
AR: Relax, this pop quiz is ungraded. Think of it as a formative rather than a summative assessment. Next, what are some of your favorite travel destinations? Perhaps you could mention one place you've been for professional reasons and one strictly for pleasure.
DP: I'll give you two each. On the professional side it would be a tossup between Cape Town, South Africa, where ICOTS was held in 2002, and Kristineberg, Sweden, where I gave a short course in 2008 on statistical phylogenetics hosted by University of Gothenburg. For pleasure—I love the Yellowstone area for its diversity of scenery and wildlife. I'd also like to get back to Greece where we had a great family reunion some years back: mile-for-mile one of the prettiest countries on the planet.
AR: What are some of your hobbies outside of statistics and education?
DP: I collect progressive political buttons from the 20th century like those from the civil rights, anti-war, feminist, LGBT rights, and environmental movements. I have about 10,000 buttons altogether. My wife and I collect kaleidoscopes—but in that case having 40 is considered a collection.
AR: Wow, 10,000 buttons is a lot! Perhaps you could wear a few hundred of them at the next USCOTS. Next, please tell us something about yourself that is likely to surprise JSE readers.
DP: I grew up riding a unicycle instead of a bike and I was pretty good at it as a teenager—for example, being able to ride backward while pedaling with one foot and going off of a curb. Now, I just go forward and backward and try to avoid breaking any bones. I do still do my unicycle challenge in every undergraduate class I teach. If at least 5% of the class gets a perfect score on a midterm, I will give a lecture while riding my unicycle. I've only had to do that once, right after my 60th birthday in 2011 at OSU—but the offer is still good here at Penn State.
AR: Please excuse me while I search youtube for a video of this… Okay, I'm back now, and disappointed that my search was not fruitful. Now I'll ask a fanciful question: You can have dinner anywhere in the world with three companions, but the dinner conversation must focus on statistics education. Who would you invite and where would you dine?
DP: Not on youtube, but you can find a picture in the OSU Stat Department newsletter at http://www.stat.osu.edu/sites/default/files/news/2011StatisticsNewsletter.pdf.
For the dinner companions, I guess I would start with Hans Rosling, whose work is always filled with optimism and shows the power of statistics interwoven with interesting stories better than anyone I've seen. Next, I would invite Joan Garfield since she loves fine dining, always helps me focus on the big picture, and we can talk about our grandkids after dinner. Finally, I would add the head of the MacArthur Foundation, so I could pitch my idea for their $100 million grant program for solving an important world problem (Why not give the whole world the tools to evaluate evidence?). I would dine at Chibchas restaurant in Catheys Valley, California. It's a Columbian food place that opened in 1968 and where I ate only once—as part of that research experience on modeling fire back in 1971. I hope your question is fanciful enough that we can go to a restaurant that closed five years ago!
AR: Hmm, I guess I'd better make that dinner reservation soon. First let's get even more fanciful. If you could travel to any point in time, past or future, what would you choose, and why?
DP: Yikes! Time travel makes this an impossible question. Should I go back in time to have dinner with Abe Lincoln and Mahatma Ghandi and Yitzhak Rabin to tell them to stay out of Ford's Theater, the Birla House, and Kings of Israel Square? It's probably not a good idea to mess with the timeline, so I'd like to take my wife out to dinner in 56 years for our 100th anniversary to that restaurant in Paris that scans your taste buds as you enter and then provides the perfect meal for your individual palate. I'll go with the Nobel Peace Prize winners for the previous two years as guests. I guess I'm just more curious about the future than the past.
AR: Getting back to reality, what has been your favorite course to teach in your career?
DP: Instead of a typical qualifying exam, the PhD Students in the College of Medicine at OSU were charged with writing a small grant proposal and that proposal was to include a statistical methods section. I developed a course in biostatistical collaboration where our students worked with the Medical College students in writing those methods sections and helped to make sure that statistical issues were well handled in every aspect of the proposals (about one stat student per five students in their program). I taught our students about various biomedical assays and their statistical properties, how to collaborate with medical scientists in writing successful NIH grants, and worked with them in providing solutions to some of the more difficult statistical issues that arose in the 30 grants we helped write per year. It was a lot of fun.
AR: The last question in the pop quiz consists of four questions with which I collect data from students. The binary question is: Do you consider yourself an early bird or a night owl? The non-binary categorical question: On what day of the week were you born? (You might consult www.timeanddate.com.) A discrete variable comes from asking: How many of the seven Harry Potter books have you read? And finally a question about a continuous variable: How many miles from where you were born do you live now? (You might consult www.distancefromto.net.)
DP: I am an early bird who was born on a Saturday but never read any Harry Potter books and now lives 2262 miles from where I was born.
Concluding Thoughts
AR: Some of our colleagues are very optimistic about statistics at this point in time, because data abound more than ever and so the ability to draw insights from data is more important than ever. Others are somewhat pessimistic about the prospects for statistics, in light of the emergence of the field of data science in which many non-statisticians are generating data analyses. What's your view of the health of our discipline in the year 2017?
DP: I'm squarely in the optimists camp. Today many statisticians are developing new software and new computational algorithms. Does that imply the end of computer science? No—it enhances computer science, shows them new areas of application, and gives them new folks to collaborate with. Today many computer scientists are generating data analyses. Is that the end of Statistics? No—I see that as enhancing our field, giving us new areas of application, and new folks to collaborate with.
AR: What do you see as the most pressing need in statistics education for the next 5–10 years?
DP: I'll stick with the basics: Infrastructure and Community. Infrastructure for both instructors and researchers as I mentioned earlier. We also need to be better at connecting people to the community of statistics instructors on a more global scale and do a better job at professional development for teachers with little/no statistical training. The advent of successful virtual meetings like eCOTS shows the possibilities with its simultaneous face-to-face regional components to match its global electronic component. I'd like to see many more virtual meetings on a variety of topics and at a variety of organizational levels (i.e., not just prepared conference style sessions—but also brain storming and help-oriented sessions). I also love the virtual poster sessions we have at eCOTS—I can see doing those on an almost continuous basis.
AR: Of all of your activities and accomplishments in statistics education, which one are you most proud of?
DP: I guess that would have to go to building up CAUSE, since that has had the most effect.
AR: Thanks very much for providing me with this interview, Dennis. I think JSE readers will learn a lot from reading about your career and your ideas for statistics education. What advice do you have for JSE readers who are fairly new to statistics education?
DP: Go to www.CAUSEweb.org. Participate in a webinar. Go to USCOTS in May 2017 and attend a workshop as well as the whole conference. Arrange a DataFest at your school or take your students to one nearby. Have your students participate in the caption contest or in USPROC. Share your favorite resource with others. Give us feedback on what you think are the most important projects we should be doing. Sign up for the CAUSE eNEWS list. Attend some of the education sessions at JSM. Join the ASA Stat Ed Section and/or the Teaching Statistics in the Health Sciences Section. And keep reading JSE!
And thanks to you Allan—I hope you get a chance to go to some of those fanciful dinners you arrange with each JSE issue!
References
- Freedman, D., Pisani, R., and Purves, R. (2007), Statistics (4th ed.), New York: W. W. Norton & Co.
- Lesser, L. M., Pearl, D. K., and Weber, J. J. (2016), “Assessing Fun Items' Effectiveness in Increasing Learning of College Introductory Statistics Students: Results of a Randomized Experiment,” Journal of Statistics Education, 24, 54–62.
- Pearl, D. K., Garfield, J. B., delMas, R., Groth, R. E., Kaplan, J. J., McGowan, H., and Lee, H. S. (2012), Connecting Research to Practice in a Culture of Assessment for Introductory College-level Statistics. Available at: www.causeweb.org/research/guidelines/ResearchReport_Dec_2012.pdf.
- Savion, L. and Middendorf, J. (1994), “Enhancing Concept Comprehension and Retention,” The National Teaching & Learning Forum, 3, 6–8.