Current State of Introductory Statistics
AR: Thanks very much, Danny, for agreeing to this interview for the Journal of Statistics Education. I always begin these interviews by asking what you were doing and planning at age 18, and then I proceed to ask a series of biographical questions. But this time I'm going to postpone asking about your background, so we can jump right into some of your current thoughts about statistics education. Let's start with “Stat 101,” an algebra-based introductory course taught at almost all two- and four-year colleges and universities across the U.S., as well as at the high school level with AP Statistics. What do you think of the standard version of Stat 101?
DK: There are both good and bad things about Stat 101. I think its main benefit has been to provide contact with statistics for mathematics instructors. We rely on people with math training to teach statistics, but there is little or nothing in the math curriculum to prepare them for this. Identifying Stat 101 with algebra ties it in to the traditional math curriculum. That is been a hugely important factor in getting math departments to accept statistics as a legitimate subject for students to take. And, like it or not, math departments have been the gatekeeper for quantitative subjects for a long time.
Unfortunately, Stat 101 does not prepare students to address many of the ways that data and statistics are needed today to deal with complexity. For instance, just about every news report of statistical work contains the phrase “after adjusting for ….” Nothing in Stat 101 helps a student make sense of this sort of statement. The course is way too heavily oriented toward p-values. The analysis methods are a 100 years old, or more. Much of the curricular innovation is about ways to overcome the severe difficulties students have with the presentation of methods with algebraic notation; that is pretty ironic for a course described as “algebra-based.” Everyone talks about how data can be used to inform decision making, but Stat 101 does not provide any meaningful framework for thinking about decisions.
Danny Kaplan is DeWitt Wallace Professor of Mathematics and Computer Science at Macalester College. He received Macalester's Excellence in teaching Award in 2006 and the CAUSE/USCOTS Lifetime Achievement Award in 2017. This interview took place via email on March 4–June 17, 2017.
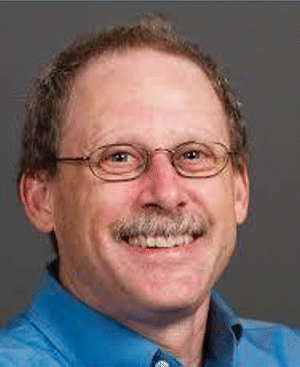
Perhaps, the biggest cost to Stat 101 is the way it displaces more meaningful data- and statistics-related topics from the curriculum: modeling, decision making, causal reasoning, and modern computing.
AR: I'm intrigued by your list of displaced topics (and I can't get the Island of Misfit Toys from Rudolph-the-Red-Nosed Reindeer out of my mind). You've written a textbook on statistical modeling. Is it intended as an alternative to a typical Stat 101 course? Have you used it yourself for that purpose? If so, please describe this course.
DK: Yes, the book we use for our intro stat course at Macalester College is Statistical Modeling: A Fresh Approach (Kaplan Citation2011). We have been teaching that course for more than 10 years. It has no pre-requisites, so any student can take it. More than half of all students at Macalester do take it.
The basic operation in the course is linear modeling: constructing a model of a response variable from one or more explanatory variables. The phrase “or more” is critical here. Students are introduced to covariates from the very beginning. This is not just warning them about so-called “lurking” variables, but giving them a way to express statistically how two or more different inputs can shape an output. This has some substantial benefits. First, the kinds of problems that are open to analysis are much more interesting and realistic. Statistics becomes a way of dealing with complexity. Second, students have an opportunity to make creative choices. Rather than worrying whether a t-test or z-test or chi-squared test is the “right” test, students use linear modeling for everything: the creativity comes in deciding what explanatory variables are relevant. Third, relationships are described as effect sizes: quantities with physical meaning. This helps, for example, when trying to distinguish between a “substantial” relationship and a “significant” relationship. Students can see that the depiction of a system depends very much on how the modeler chooses to describe it. If you want to model causation, you need to make sure that your model reflects the real-world mechanisms at work.
I do not think you can do a course like this if you rely on algebra. But you can do it if you use computers appropriately. With the right notation, it is no more difficult to build a model than it is to calculate a grand mean or groupwise means. With the help of our colleagues Randy Pruim and Nicholas Horton, that notation has been simplified and streamlined and is available via the mosaic package for R.
Another big benefit to using computer notation rather than algebraic notation is that you can represent ideas like sampling. There is no algebraic notation for “take a random sample” or “repeat this many times.” But computer languages–particularly in R with the mosaic package–have a very straightforward way of expressing those concepts and directly examining their consequences.
AR: Can you provide an example from that course in which students learn to understand and apply the phrase “after adjusting for” that you emphasized earlier?
DK: I want my students to know something about the world, so I give them data on country-by-country death rates (measured as deaths per thousand people in a year). Their job is to figure out which countries are safe and healthy, and which are dangerous and unhealthy. Here are the data from a few countries: Afghanistan 14.12; Somalia 13.91; North Korea 9.18; South Sudan 8.42; United States 8.15; Haiti 7.91. That gives you a sense of how dangerous it is to live in the United States. Other places that are surprisingly dangerous: Japan 9.38; Denmark 10.23; Canada 10.40; Belgium 10.76; Germany 11.29. And, shockingly safe: Israel 5.54; Egypt 4.77; Gaza 3.09.
It is true that safety and health varies tremendously from country to country. But the big killer is … aging. Old people die at a high rate, young people do not. European countries and Japan have a relatively elderly population. The median age of people in Japan is 46.9 years. Gaza and Egypt have a very young population, with median ages of 16.9 and 23.8, respectively. If you want to draw a meaningful conclusion about safety and health, you need to adjust the death rates to take age into account.
There are some simple ways to do this, for instance, by stratifying cases according to the value of the covariate. For the death-rate data, this might mean comparing 30-year olds in one country to 30-year olds in another.
Two examples from my book are described in the GAISE College Report (American Statistical Association Citation2016). One is about how state spending on schools relates to standardized test outcomes. (Spoiler alert: Higher spending is associated with lower test scores. But that pattern goes away when you take into account that in low-spending states, only the most elite fraction of students take the tests.) Another example is about mortality and smoking in a survey from the 1970s. You see the ill effect of smoking only when you adjust for age.
AR: How about if I also ask for an example that you use for students to model and explore ideas of causation?
DK: The need to take other variables into account is most pressing when you want to make causal statements: unsafe and unhealthy conditions cause death; smoking increases mortality. The message of Stat 101 is that the only legitimate way to infer a causal relationship is by doing a controlled experiment with random assignment. But there are many important matters where it is not possible to do an experiment and yet responsible people need to be able to say something meaningful about how things are connected in the real world.
Statistics actually has a lot to say about how to draw responsible causal inferences in nonexperimental settings. We ought to be showing our students statistics is not helpless and data are not meaningless when it comes to causality. Epidemiologists are taught how to diagram hypothesized causal paths so that they can select covariates appropriately.
In teaching about causality, I have found a few helpful approaches. One is to emphasize that data analysis is inevitably tied to assumptions about how the world works. Students are obliged to bring to the table their notions of what causes what. Those notions may be incomplete or incorrect, but data are analyzed conditioned on those assumptions. Another approach is to construct simulations of real-world systems from which to generate data. That way, it is clear what the connections are between variables. The simulation may not accord very well with the real world, but it provides a setting to try out techniques that can identify the relationships that generated the data.
One example I use is a set of hypothesized relationships between sickness and a drug, say aspirin. In the simulation, aspirin reduces sickness. But also, people are more likely to take aspirin when they are sick. So aspirin influences sickness and sickness influences aspirin consumption. Can we untangle those relationships?
Imagine that we can carry out a controlled experiment, but let us make it realistic and include patients who do not follow the experimental protocol: they might take aspirin on the side regardless of whether they are in the treatment or control groups, or they might fail to take the assigned drug or placebo because it is inconvenient or they are feeling particularly healthy or ill. In short, a mess.
Students have no trouble translating the description into a diagram of causal connections. And they will suggest ways of dealing with the mess. For instance, they might use blood tests to measure the actual amount of aspirin taken. Or they might interview the subjects carefully with an eye to throwing out the data from subjects who did not comply with the protocol. It turns out that neither of these is a good approach, since both fail to break the causal connection between how a patient feels and consequently taking or avoiding aspirin. You can confirm this from the simulation. Instead, a completely counter-intuitive approach called “intent to treat” can be a good way to carry out the analysis. You can verify that intent to treat works in the simulation and you can see why it works by examining a diagram of causal connections.
AR: We've covered modeling and causal reasoning from your list of displaced topics. Can you provide an example about decision-making from your introductory course?
DK: Decision making is an important topic, but it is not exclusively a statistics topic. For instance, economics has a lot of useful things to say. And it is helpful to introduce decision making in a context that is both familiar and important to students. Making space for all this–stat, econ, context–was not something I could manage in the intro stats course. So at Macalester, I developed a separate, no pre-requisite course for this.
There are many contexts which would be appropriate for teaching decision making in a compelling way–international development, criminal justice, personal finances, and so on. The one that made sense at Macalester was medicine and public health. So I developed an epidemiology course. Part of the course is about nuts-and-bolts epidemiology like contagion, case-control, and so on. And I think for any of those other contexts I mentioned there would need to be some nuts-and-bolts taught to make clear the difficulties of decision making: What is parole? How important are roads? What is a mortgage? But ultimately, any decision making course will be about making choices about X with crummy data. For epidemiology, X equals health and illness.
Should we recommend mammography for women or PSA testing for men? What heroic treatments should be available by an insurance plan? What would you need to know to make such decisions in a way that reasonably reflects public mores but also respects limits to resources?
Some facets of decision making are clearly statistical: Do we have enough information to say something useful about an issue? What are the sensitivity and specificity of tests? Is it worthwhile to collect more data? How do you choose the correct conditional probability to describe risk, and how do you estimate that conditional probability from the available information?
Decision making is also about resource allocation and trade-offs. This is where economics comes into play. In economics, you have to deal with multiple conflicting goals. The techniques developed for this–constrained optimization, shadow prices (a.k.a. Lagrange multipliers)–provide tremendous insight.
Data Computing
AR: The fourth displaced topic in your list is modern computing. I know that you are very interested, and have done considerable work with, the role of scientific and statistical computing in the undergraduate curriculum. Please tell us about your scientific computing course at Macalester.
DK: When I arrived at Macalester in the mid-1990s, having spent 15 years in a research environment, I knew a lot about computing and nothing about statistics. My project from day one was to bring scientific computing to the undergraduate curriculum. Computing is an important component of just about every quantitative scientist's skill set, yet graduate students were still expected to learn it on their own, to pick it up on the proverbial street.
I developed an intro course, Scientific Programming, which used a mainstream language in science, MATLAB, and focused on a set of examples closely tied to typical scientific computing tasks, for example, signal and image processing, databases, numerical modeling, and solution. At the time, that course had an enrollment larger than all the other computer sciences courses combined. The book I wrote for that course has just been translated into Python (Kaplan, Levy, and Lambert Citation2016). Interest in the intro course led to another course, now called Numerical Linear Algebra, which is about the theory and application of the classic algorithms in scientific computing, things like singular value decomposition, splines, and so on.
In parallel with this, I was assigned to teach Stat 101. But, motivated by Brad Efron's work and drawing on my involvement in the very early days of machine learning, I had a very computational approach to the inferential side of Stat 101. This spilled over into the descriptive statistics part of that course: models can inform much more than means, and it is no harder to type “lm()” than “mean().” The challenge I faced was making the commands simple and expressive enough that students could master them with little difficulty. I had taught programming, so I knew that it was not feasible to teach regular programming as part of Stat 101. But there are two traits to a computer language that can make it much easier to use without having to write programs. R, which had just come on the scene, had both of these: vectorization and a “functional” paradigm. I made a “three-command” rule for myself. Every statistical calculation in the course had to be do-able in three lines: one to read in the data, one to do the statistics, one to display the results graphically or in tabular form. Over many years, this became the germ of the “mosaic” package. Gratifyingly, the reactions from my fellow faculty went from “You can't do that with students,” to “How do you do that?” to “How do I do that?”
I have used a similar approach in the new introductory data science course, Data Computing. Systems like “dplyr” draw on just a small number of basic operations and have a very clear notation. So students can learn to do data wrangling and visualization in just 10 hours of class time, with enough left over for them to learn some statistics as well! Even better if that statistics can be taught in a machine-learning style, giving a pretty broad data science curriculum in just one course.
AR: Please tell us more about this Data Computing course. What kinds of students (majors) take it? Are there any pre-requisites? What topics are included?
DK: Like data science generally, the point is to extract actionable information from data. There are not any pre-requisites, so the emphasis is on designing, making, and interpreting graphical displays; even people without previous technical training can deal with graphics.
There are two theoretical frameworks that form the backbone of the course. One is the “grammar of graphics,” a formal scheme invented by Wilkinson in the late 1990s (Wilkinson Citation1999). For us, the purpose of the grammar is to provide a common way to describe a large variety of graphics. Using that, designing graphics becomes a matter of providing answers to a handful of questions: what variables will define the graphics frame, what glyphs will be used to represent individual cases or statistics on groups of cases, what variables will be mapped to the graphical features of the glyphs–color, size, shape, and so on. This works well as a vehicle for exploring what makes some graphics successful in telling a story and others not. The students can change the roles of the various different variables being depicted and judge for themselves whether that has improved the graphic or not. It helps tremendously that there is a software system, ggplot2 in R, which has a notation that parallels the graphic, so expressing a design in software is pretty much the same thing as describing the design as ideas.
The second theoretical framework is relational database operators. These are what make it possible to “wrangle” data from their given form–which may involve multiple sources–into a form that is “glyph ready,” that is, ready to be depicted as graphics. There is a huge advantage to using relational operators instead of general programming constructs. Learning loops, indexing, conditionals, etc. is hard; the relational operators are much easier: building blocks from which bigger operations can be built. And again, because we are using R rather than, say, SQL, there is an expressive and concise notation.
We get students from every division of the college in this course. And, each semester, there are a few local alumni taking the course who need to work with data in their job but who never got the chance to learn a systematic approach. The course closely follows the text Data Computing (Kaplan Citation2015), which was written for the course.
My most important goal for the course is that students learn to read. Writing computer notation is almost always a matter of finding a close example and modifying it to suit the purpose in hand. But to find and modify an example, you need to be able to read what other people have done. And by reading, you can see what sorts of things are possible. This applies to graphics as well as code. By learning the grammar of graphics, students are learning not just to gain a visual impression from graphics but how to read them: to see exactly how the graphic relates to data and also to imagine how the graphic might have been done differently and perhaps more effectively.
Beginnings
AR: We started this interview in the present, but now I'd like to go to the past. The ideas and experiences that you've described about teaching and curriculum suggest that you have a background that transcends narrow training in statistics. What did you study as an undergraduate, and where, and what were your career aspirations at that point?
DK: My undergraduate field was physics. Physics is all about modeling, which fits very nicely with statistics. (On the other hand, I, like most physicists, was taught the maxim: “If your experiment needs statistics, you ought to have done a better experiment.” Not helpful!) I did not see myself becoming a research physicist–I had always been primarily interested in policy issues: energy, pollution, arms control. I decided to switch fields and work on policy and economics. And, since I graduated into a recession, graduate school looked like a good choice. I got a master's degree in “engineering-economic systems” and worked for a few years as an energy economist, building models of the adoption of energy efficient technologies by industry. “Engineering-economics” is a funny but apt name. Engineers like to design, build, and refine things. Economists try to deal with how society sets things up. You cannot steer the economy, but you can figure out which little pushes will help you move toward where you want to be.
I missed doing science, and I liked building things, so I left economics for biomedical engineering. I gravitated toward cardiology–a physics-like system. And the engineering was really machine learning: the attempt to extract physiologically useful information from heart rate, blood pressure, and respiratory signals. After getting my doctorate, I worked for a small medical electronics company and then moved to the department of physiology at McGill medical school.
In retrospect, I can see statistics throughout these wandering years. Machine learning. Design and evaluation of diagnostics. The econometrician and engineer's primary concern with causal relationships. But at the time, I thought of myself as building mathematical models of biology. I did not even know what a t-test is.
By 1995, the French–English factionalism and economic disintegration of Quebec was such that it was uncertain that we could have a future there. Macalester College was looking for someone to develop a biomathematics major, so we moved to Minnesota.
AR: What were your first few years at Macalester like? What did you teach, and what was your teaching style at that point? Did the biomathematics major come to be?
DK: Although I was not hired to focus on statistics, from the beginning I taught “elementary statistics” just about every semester. This was difficult, and not just because I did not know anything about what was in the book. I had never taught any introductory-level course before, and I did not have any grounding in what statistics is used for and the good and bad reasons why things are set up the way they are. I figured out a few tricks that worked for me: I never used class notes because, if I did, I would be looking at the notes and not at the students; I tried as much as possible to make the class a guided discussion because, if I did not, I would be spending all the time talking. I would much rather be asking questions than professing answers.
The first semester, I had been given diskettes with special statistics software. It was a widely used mouse-driven system, but I will not use the name. What the software did was quite simple, but explaining the sequence of menu pull-downs and checkboxes to get a result was very complex and had no unifying logic. So by the second semester, I dumped the “official” software and started writing demos and labs in R. Students complained: “Why can't I use Excel?” I was not sympathetic. I told them that they were there to broaden their horizons, and if there is one thing I know much more about than they, it is software and computing. I was happy to work with them to use R, but unwilling to abandon them to whatever they had picked up on the street about Excel. And besides, although Excel is an excellent tool for making things happen on the screen, the paradigm makes debugging very hard and provides no support for verifying that what you think you are computing is actually what you are computing.
I made mistakes. I wrote labs about statistical procedures that had 20+ steps–following the path I had taken trying to figure this statistical stuff out for myself. I talked too much. I used too much algebra. I hated making such mistakes because it was robbing the students of their one chance to learn this stuff and to discover that they could do it. We have this bizarre system in higher education of throwing teachers into the classroom without any supervision or support for development. So there was no choice but to make mistakes and learn from them.
The statistics was very much a sideline. My main themes were mathematical modeling, dynamics, and differential equations, and what was then called “computational science.” I discovered within a year that there was no audience for biomathematics: the mathematics majors were not interested in biology, and the biology majors did not have time to take mathematics. Not that the biologists were scared of math. The biology major required both a semester of calculus and of statistics.
In scoping out the possibilities of a biomath major, I learned something that became very important. The calculus that was being taught was irrelevant to biology. It also did not help with statistics. I took calculus in 1975 and never used most of it despite being a physics major. Nobody deals with convergence of series or limits. And even in the old days, if you need to integrate something, you used a table of integrals. The hard part, modeling, representing the world in mathematical terms, was not even touched on in the calculus curriculum.
Biologists very much benefit from understanding functions of several variables and interpreting them with partial differences and derivatives. Differential equations provide a lot of insight. And statistics is closely tied to linear algebra, not calculus. These topics are not reached until the third, fourth, or fifth semester of a calculus sequence. A typical biology student does not get anywhere near those courses.
Why not teach the biology- and statistics-related topics in a first-semester course? That is where it is needed. So that is what I developed: an entry-level, modeling-based course about the topics that scientists can actually use in their work – modeling, functions of two (or more) variables, numerical calculations, the phase plane, units and dimensions and the consequences of doing arithmetic on dimensional quantities (as opposed to pure numbers), constrained optimization, fitting linear combinations. That modeling approach was extended to statistics. And everything was embedded in computing.
Not unexpectedly, what worked for the biologists worked for the economists and others. Eventually, this led to the foundation of Macalester's major in Applied Math and Statistics (AMS), now one of the most popular at the college.
Statistics at Macalester
AR: Please tell us about this major. What courses comprise it? How hard was it to establish? What is especially good about it? What challenges does it face? (Feel free to take your pick from these questions, or answer your own!)
DK: There is a common core to AMS: three semesters of calculus, linear algebra, the introductory course for computer science majors, and our statistical modeling course (which is also our introductory course taken by students in other majors). A typical path for a statistics-oriented student continues on with the second statistics course: machine learning. Then probability, Bayesian statistics, and survival analysis. There is a lot of variation in student selections for the final required course: computational linear algebra, mathematical modeling, artificial intelligence, a pure-math course, databases, network science. There is also a senior-level mathematical statistics course, but that is not a common choice. The applied math path is very similar: Bayesian statistics and survival analysis would be replaced by differential equations and the student would take both mathematical modeling and computational linear algebra. Everyone must take at least two computing courses and three statistics courses.
This will sound corny, but the key element in establishing the major is the mutual respect that the mathematics, CS, and statistics faculty in my department have toward each other. When the statistical modeling course was developed, the mathematicians, without any prodding, decided to require the course for the math major. The applied calculus course was important too. It turns out that there are a lot of students turned off by high-school calculus but who are strongly motivated by seeing genuine uses for mathematics. So the number of math majors increased substantially, and those new majors brought their interest in applications to the math courses they took.
I particularly like that the program takes a “big tent” approach: if a student is interested in what we have to offer, we want to bring that student into the tent. In the early years, we tried to make it as easy as possible to have an AMS major, for example, allowing the econometrics course to count toward the major. Now, demand is so heavy that we risk starting to restrict whom we will accept into the tent.
AR: Let me ask for more details about two of these courses. Machine learning is not typically a second course in statistics; how do you make that work? And I can't help myself from asking about the Bayesian course: what do you teach in that course?
DK: Machine learning makes a very natural early course in statistics. The things that might be off-putting to statistics educators are in fact very helpful at getting students to see a big picture. For example, machine learning has inherited some of the culture of software development. One aspect of this is the idea of “abstraction,” that it is a good thing if the user of a function does not need to know how the function works internally. For instance, linear models and smoothers are both classes of functions. The abstraction for both involves (1) training (finding a good function to match the data) and (2) evaluation (finding the output for a given input). It does not matter that the implementation of these is simple or difficult.
A reasonable Day 1 for a machine-learning class is to display a scatterplot with some clear ski-jump shaped relationship between y and x. Now display the function that comes from simple regression. On top of that, put a function generated by a smoother. Ask which function is better. This is a simple recipe for a rewarding class discussion. When students get to the point where they say the smoother is better because it is closer to the data, generate a more flexible smoother that is even closer than that.
It is kind of fun that machine learning includes so many different kinds of model architectures: recursive partitioning, support vector machine, discriminant analysis, random forest, and so on. But that is not what is important. The fundamental statistical questions are how to measure a function's performance as a predictor, how to compare competing functions, and how to incorporate existing knowledge into the functions you build from data.
The Bayesian course was developed by my colleague, Alicia Johnson. It covers about what you would expect: the operation and interpretation of Bayes rule, settings where there are particular advantages to a Bayesian approach, and computer techniques up through MCMC. I guess there are some people who claim that Bayesian philosophy, computing, and applications are not accessible at the undergraduate level, but Alicia has certainly made it work for our students.
Is there anyone in the world of statistics who thinks Bayes will be less important in the coming years? We know where things are heading. Alicia is moving in that direction and taking our students with her.
Pop Quiz
AR: Now let's begin the “pop quiz” portion of the interview, where I'll ask a series of questions and request that you keep your responses to just a few sentences or less. First, please tell us about your family.
DK: My wife and I have three daughters, and recently become empty nesters. Two little dogs co-inhabit the nest with us.
AR: Please name some (non-statistics) books that you've read recently.
DK: Caught in the Revolution, a history of the 1917 Russian revolution in St. Petersburg, The Diaries of Adam and Eve by Mark Twain, and a history of the battle of Waterloo by Bernard Cornwell.
AR: What are some of your favorite travel destinations? Perhaps you could mention one place you've been for professional reasons and one strictly for pleasure.
DK: I used to teach a summer-school class in Barcelona–a city of lovely architecture between the mountains and the Mediterranean. For pleasure, I like quiet. I try each summer to go kayak-camping in the boundary waters, on the border between Minnesota and Canada.
AR: What are some of your hobbies outside of statistics and education?
DK: Over the last two years, I have been taking up woodworking in a slow but steady way, mostly using hand tools. Last year was the “summer of dovetails.” They started out shaky, but became neater over the months. I am still learning how to saw straight.
AR: Next, please tell us something about yourself that is likely to surprise JSE readers.
DK: My first publication was in the Bulletin of the Atomic Scientists, a short feasibility study (and therefore a critique) of space-based ballistic missile defense. It was reprinted in the Pentagon's daily news summary.
AR: Now I'll ask a fanciful question: You can have dinner anywhere in the world with three companions, but the dinner conversation must focus on statistics education. Who would you invite, and where would you dine?
DK: John Tukey, Florence Nightingale, William Playfair. All three were highly inventive people who emphasized the use of data to communicate understanding. I would go to Din Tai Fung, a 1-star Michelin restaurant in Taipei. (I have been to a branch in Los Angeles.)
AR: Let's get even more fanciful. If you could travel to any point in time, past or future, what would you choose, and why?
DK: I'd go to Florence in the second half of the fifteenth century: great art, food, politics.
AR: And now back to reality, what has been your favorite course to teach in your career?
DK: I have really liked teaching the “Data Computing” course. The students are so enthusiastic and so satisfied when they get things working.
AR: The last question in the pop quiz consists of four questions with which I collect data from students. The binary question is: Do you consider yourself an early bird or a night owl? The nonbinary categorical question: On what day of the week were you born? (You might consult www.timeanddate.com.) A discrete variable comes from asking: How many of the seven Harry Potter books have you read? And finally a question about a continuous variable: How many miles from where you were born do you live now? (You might consult www.distancefromto.net.)
DK: Early bird. Wednesday, All seven (aloud, to my girls). 924 miles.
Parting Thoughts
AR: Congratulations on recently receiving the CAUSE/USCOTS Award Lifetime Achievement Award in Statistics Education. During the presentation of this award, I was especially struck by comments from two of your nominators. Your Macalester colleague Victor Addona compared your success in teaching and curriculum development to the famous quote about hockey great Wayne Gretzky, who said that his key to success was skating to where the puck was going to be rather than where the puck was. Nick Horton then commented that boxes were invented to give you something to think outside of. I want to ask a better question than simply: How do you do this? How about: What advice can you offer to someone who wants to break out of conventional thinking about the teaching of statistics?
DK: It is not always a good idea to break out of conventional thinking, but we in statistics work in an environment of very rapid change: computing, the ubiquity of data from remote sensing to genetics to text messages, the shift to an information and medicalized economy, the huge increase in demand and need for postsecondary education. It would be amazing if the statistical techniques developed a century ago were still the best way to go. I encourage people to think about the contemporary reasons why the field of statistics is so important today and what techniques are needed to deal with today's world. Then engineer the curriculum around those techniques. Drop the educational metaphor about “starting on a life-long journey.” Focus, particularly at the college level, at getting students to the place they need to be. Students cannot afford to be dropped off in 1910 and expected to bushwhack their own way to the present.
AR: I understand that you have retired from Macalester as of a few weeks ago. Do you plan to stay active in statistics education?
DK: Absolutely. My “retirement” from Macalester is a way to provide more time to work on education projects by stepping away from the demands of day-to-day teaching. I want to participate in the inevitable transition to online and interactive modes of teaching. And, after 20 years being privileged to work at an elite undergraduate institution, I want to see if anything I have learned in that setting can be of use to improve educational opportunities for a broader range of students.
AR: Which among your many professional accomplishments are you most proud of?
DK: Getting traction on the idea that statistical modeling is an accessible and effective entry point for learning about reasoning with data.
AR: Before I ask my final question, let me ask whether there's anything that you wish I had asked that I haven't. In other words, is there anything you'd like to say for which I have not yet provided an opportunity?
DK: I would like to mention what most surprised me about mathematics education. Keep in mind that up until my 30s, I had no plan or calling to become a teacher. The limited amount of math I studied was for the purposes of being able to do things as a scientist and engineer. Of course, I knew that there are people who find math easy and many others who find it terribly difficult. I naturally assumed that the people who find math easy had the option of studying subjects in which central concepts are presented in a mathematical fashion–physics, engineering, and such–and that people who find math hard did other subjects. I saw no evidence at all for a correlation between mathematical inclination and professional ability.
So I was shocked to discover the world of remedial math for college students. People trying to start careers in fields such as nursing for which they are perfectly well suited being obliged to take courses about medieval mathematics taught by faculty who have absolutely no contact with the fields their students are studying. Imagine, a class of 200 nursing students studying “completing the square” taught by a highly regarded mathematician who is not even aware of the sorts of units of measurement used in nursing. I do not have to imagine it; I have seen it. And heard that upwards of half the students will not pass the course. This is like the days of blood-letting in medicine.
Hundreds of thousands of students are hit by this every year. There are sincere and thoughtful efforts at reform, but nowhere near enough investment and support for the reform efforts.
AR: Thanks very much for taking the time to answer my questions so thoughtfully, Danny. My final question is the same that I have asked of others in this interview series: What advice do you have for JSE readers who are fairly new to statistics education?
DK: First, think about statistics as science. Science progresses as it invents new ways of extending human perception: telescopes, microscopes, photography, radio waves, magnetic resonance, gene sequences, and so on. Similarly, statistics progresses as it provides new ways of observing and organizing: collating data about a state or a population, comparing theory to data as with chi-squared, quantifying correlation, constructing models from data. Those statistical ways of observing continue to advance as with machine learning, modern graphics. Teaching a statistics course about chi-squared and correlation is like teaching a biology course up through the invention of the microscope; it is a start, but it does not prepare you very well for the issues of today and tomorrow.
Second, in much the same way that people cannot see radio waves, people are bad at perceiving and communicating uncertainty and risk. We invented the radio so that we can “see” radio waves. This inventiveness did not stop with Marconi. Similarly, we have invented ways of “seeing” and communicating uncertainty. This inventiveness did not stop with Bernoulli. Think about the needs people have today for dealing with uncertainty and teach methods and concepts for meeting those needs. Does the central limit theorem help with communicating about uncertainty? No. Are p-values an effective way to convey uncertainty? After 80 years of using p-values, people consistently make fundamental errors and fail to understand the very limited context in which p-values can make any sense at all. It is time to move on.
References
- American Statistical Association. (2016), “Guidelines for Assessment and Instruction in Statistics Education College Report 2016.” Available at http://www.amstat.org/education/gaise
- Kaplan, D. (2011), Statistical Modeling: A Fresh Approach (2nd ed.), Project Mosaic Books, St. Paul, MN.
- ——— (2015), Data Computing: An Introduction to Wrangling and Visualization in R, Project Mosaic Books, Saint Paul, MN.
- Kaplan, D., Levy, S., and Lambert, S. (2016), Introduction to Scientific Computation and Programming in Python, Project Mosaic Books, St. Paul, MN.
- Wilkinson, L. (1999), The Grammar of Graphics, Spring-Verlag, New York, NY.