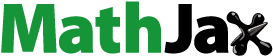
ABSTRACT
Statistical literacy refers to understanding fundamental statistical concepts. Assessment of statistical literacy can take the forms of tasks that require students to identify, translate, compute, read, and interpret data. In addition, statistical instruction can take many forms encompassing course delivery format such as face-to-face, hybrid, online, video capture, and flipped. In this study, we examined statistical literacy of graduate students using a validated assessment tool (the Comprehensive Assessment of Outcomes in Statistics; CAOS) across two increasingly popular delivery formats—hybrid and online. In addition, we examined condensed (six week) semesters to full (16 week) semesters to determine if course length was related to statistical literacy. Our findings suggest that, holding other factors constant, delivery format is not related to statistical literacy for graduate students. This contradicts some existing research that shows hybrid delivery outperforms online only. Our results have important implications for the teaching of statistics as well as for graduate education overall.
1. Introduction
1.1. Statistical Literacy
Constructs such as critical thinking, engagement, motivation, and learning are arguably requisite elements for instruction to be effective and for students to successfully learn (Dziuban Citation2016). Each of these constructs derives from learning theory. The fact that they are essentially abstract, but making them objective in the assessment process can create issues in that very process (Postman Citation2011). Star and Griesemer (Citation1989) described these constructs as boundary objects, “abstract or concrete… [with] different meanings in different social worlds but their structure is common enough to more than one world to make them recognizable … .” Statistical literacy, as well as online and blended learning as types of course modalities, conforms similarly and there is likely no universally accepted definition for and measurement of these. Rather, “we must be cognizant that we are forced to select surrogates to assess the characteristics in which we are interested … . As researchers, our responsibility is to understand that at best these surrogates only approximate the construct with which we are interested” (Dziuban Citation2016, p. 160).
Statistical literacy—more narrowly termed as statistical competency and statistical citizenship—refers to the understanding of fundamental statistical concepts (Rumsey Citation2002). Assessment of statistical literacy can take the forms of tasks that require students to identify, translate, compute, read, and interpret data (https://apps3.cehd.umn.edu/artist/glossary.html). Statistical reasoning involves understanding and being able to explain and interpret statistical information (Garfield Citation2002). Assessment tasks for statistical reasoning include being able to explain why and how (https://apps3.cehd.umn.edu/artist/glossary.html). Statistical thinking refers to understanding why and how investigations that use statistics are conducted (Chance Citation2002) and this concept can be assessed with items that require evaluating, critiquing, generalizing, and/or applying (“Defining and Distinguishing Statistical Literacy, Statistical Reasoning, and Statistical Thinking” n.d.). With some overlap between statistical literacy, reasoning, and thinking, statistical literacy is the foundation for the triad (delMas Citation2002).
The literature on statistical literacy has developed into two broad areas over time. First, statistical literacy has focused on understanding the application of statistics at the student level (Watson and Callingham Citation2003). Second, adult statistical literacy emphasizes people's ability to interpret and critically evaluate statistical information, data-related arguments, or stochastic phenomena and their ability to discuss or communicate their reactions to such statistical information (Gal Citation2002). For this study, we focus on student statistical literacy in the context of graduate education and more specifically the extent to which course modality may relate to statistical literacy.
1.2. Course Modality
With recent technological developments, instruction can now take many forms. Course modality or delivery format is a traditional face-to-face in a classroom, online, or a hybrid of the two. Hybrid, also known as mixed-mode or blended learning, courses allow students to complete some coursework online but still require some face-to-face meetings (Brown Citation2001; Carnevale Citation2002; Oblender Citation2002; Young Citation2002; Ward Citation2004) and have been described as “the best of both worlds” (Young Citation2002, A33). It is now commonplace that blended and online modalities have augmented the traditional face-to-face course, and millions of U.S. college students are enrolled in hybrid courses (e.g., Picciano Citation2015). There are numerous advantages to hybrid courses. For example, students have access to online resources while still having the opportunity for face-to-face guidance from an instructor (Ward Citation2004). Also, students that may not be technologically skilled have the opportunity to hone their technology skills while cushioned with traditional class meetings (Ward Citation2004).
Courses can also be taught completely online. Online courses are those in which all components of the course are delivered via the internet and are becoming much more common in most disciplines (Dutton and Dutton Citation2005). About 25% of postsecondary students were enrolled in at least one online class in fall 2013 (Allen and Seaman Citation2015). Postsecondary institutions continue to adopt online learning as a vehicle to deliver instruction (James, Swan, and Daston Citation2016). Online courses have many advantages including increased accessibility and the ability for students to control when and where they complete the course (Burbules and Callister Citation2000).
There are a number of factors that have driven the increase in variety of course modalities offered in higher education (Kwak, Menezes, and Sherwood Citation2015). These include, for example, decreased student funding (Mortenson Citation2005) and improved technologies that allow online course delivery to be more affordable for institutions to offer and more accessible to students (Twigg Citation2013). Unfortunately, there exists no consensus on the effectiveness of online courses versus face-to-face or hybrid instruction formats despite the many studies conducted (e.g., Watson Citation2011; Kakish et al. Citation2012; Bowen et al. Citation2014; Haughton and Kelly Citation2015). This inconsistency could be the result of a plethora of types of online learning. Another potential source of inconsistency is the methodology used in these studies, which include quasi-experimental studies, a few randomized studies, and many observational studies.
Some research suggests that performance is similar regardless of course modality. Sami (Citation2011) found that there was no difference in student learning outcomes among online, hybrid, and face-to-face formats. Bowen and colleagues (Citation2014) found similar results in their randomized trial of students across six campuses. Students were randomly assigned to take the hybrid mode of instruction or the face-to-face traditional format. The researchers measured the effect of instruction on learning outcomes in a statistics course. Consistent with findings of similar studies (Kakish et al. Citation2012; Haughton and Kelly Citation2015), Bowen et al. (Citation2014) found that learning outcomes are essentially the same for both modes of instruction. Unlike the Kakish and colleagues (Citation2012) study, however, that had the same instructor teaching all sections of the class, Bowen et al. (Citation2014) used as many instructors as there were campuses, with their concomitant institutional policies.
Kakish and colleagues (Citation2012) had a sample drawn from a multicultural student body of an open access undergraduate program. Students in the face-to-face and hybrid format were measured across four sections of an undergraduate statistics class during one semester. Their final examination, which was a common assessment, was used as the performance metric. Although the authors cautioned that other forms of assessment might lead to different results, their results reflected no significant differences between performance of face-to-face and hybrid students. Similarly, Utts (Citation2003) and Ward (Citation2004) found no differences in performance for students enrolled in a hybrid versus a traditional statistics course.
Other research provides less conclusive results, with differences in modality dependent on the outcome (e.g., grades, satisfaction). In a study employing a quasi-experimental design comparing students in face-to-face and flipped hybrid environments (i.e., a hybrid course where the instructor is the facilitator/mediator, assisting students to apply their knowledge, and students learn material through technology [Margulieux et al. Citation2014]), Haughton and Kelly (Citation2015) used four distinct outcome measures including final exams as well as some qualitative assessments. After controlling for observable differences within the two groups, the results of the study indicated that the students in the flipped hybrid environment performed better on the common final exam. There were, however, no significant differences in the final grades or satisfaction with the course for students in different class formats.
Unlike Haughton and Kelly's (Citation2015) study, Scherrer (Citation2015) assessed students' performance as well as instructor evaluation over three modes of instruction—face-to-face, hybrid, and fully online. The results of this study indicated no statistically significant differences in student effort or in student completion of the course. Moreover, the final grade percentage indicated that students in the face-to-face environment performed significantly better than those in the hybrid environment. Other research suggests that the effect of course modality on student performance is a factor of whether learning is cumulative or not, with blended courses leading to no statistical difference when learning is noncumulative but actually leads to a negative effect when the learning is cumulative (Kwak, Menezes, and Sherwood Citation2015).
Still other results suggest that teaching statistics in an online environment has been found to be as or more successful as traditional face-to-face courses (e.g., Dereshiwsky Citation1998; Dutton, Dutton, and Perry Citation2001; Russell Citation2001; Dutton and Dutton Citation2005; Kakish et al. Citation2012). According to a recent meta-analysis published by the U.S. Department of Education (Means et al. Citation2010), performance of graduate students and professionals taking classes with an online component (either fully online or blended) had a statistically higher effect than those in face-to-face classes (mean effect was +0.10). In addition, students in the hybrid format showed better performance in a learning outcome (i.e., direct and objective measure such as standardized test score, exam scores, and similar) compared to those in solely face-to-face or online settings (Means et al. Citation2010). However, they also reported that the effects of course delivery varied depending on the learning content and the characteristics of learners.
Previous research in statistics education does not show consistent results regarding the effectiveness of course delivery format on student learning outcomes or performance. Even though many of these studies indicated no difference in learning outcomes based on course delivery format, some found increased performance in either the face-to-face format, or the hybrid format. Thus, there remains a lack of consensus on the extent to which learning outcomes differ based on course delivery format.
1.3. Semester Length
Course scheduling impacts both the financial considerations of an institution as well as the operational considerations (Shaw et al. Citation2013). Full-length semesters, offered in fall and spring, often include 16 weeks of instruction, and many institutions offer summer terms that are condensed in weeks but include similar instructional hours (Shaw et al. Citation2013). Benefits of condensed courses can be argued. These include, for example, more presence with peers (Bailey and Morais Citation2005) and quicker feedback from faculty (Lee and Horsfall Citation2010). This may, in turn, reduce isolation that can be experienced by students in online settings (Chametzky Citation2013). According to research by Scott (Citation1996), students actually prefer courses offered in condensed time as it increases collegiality, interactions, and discussions as well as fosters a focused learning environment.
A number of researchers have examined the relationship between student learning and course length. Because the same amount of material is covered in a significantly smaller amount of time, it seems logical that summer courses and other courses offered in fewer weeks would lead to poorer student learning (Anastasi Citation2007). Research suggests otherwise, however, with some evidence suggesting that semester length is not statistically related to course performance (Seamon Citation2004). Examining online courses, research suggests no statistical effect of course length on student performance as measured by final course grade and number of assignments completed (Shaw et al. Citation2013).
Other research suggests that students enrolled in summer courses perform at least as well, and in some instances significantly better, than students enrolled in full term courses (Anastasi Citation2007; Daniel Citation2000). Austin and Gustafson (Citation2006) found that grade point average (GPA) is actually higher from courses taken in a condensed semester (3, 4, and 8 weeks relative to 16 weeks) and grades in a condensed course have the same explanatory power for future performance in related courses as compared to 16-week courses, even after controlling for course load, demographics (race, gender, age), and baseline performance (SAT math and verbal, beginning GPA). Mensch (Citation2013) compared the final course grades in online numeric-based courses (algebra, accounting and other courses that involve mathematical calculations) that were offered for 3, 5, and 14 weeks with consistent course material across the three time frames. He found that students were more likely to get an “A” or “B” grade and less likely to withdraw from the 5-week course when compared to 14-week course. Similar results were found by Ferguson and DeFelice (Citation2010) who studied student performance in two online courses where the course content and methods of teaching remained constant but the duration was different: one course was 5 weeks long while the second course was 15 weeks long. Students' average final grade was found to be significantly higher in the five-week course compared to the full semester.
1.4. Masters Versus Doctoral Students
Students in different degree levels, specifically masters versus doctoral, often have different levels of experience with research and statistical methods, and there is evidence to suggest that doctoral students have higher levels of general critical thinking skills (Onwuegbuzie Citation2001). Although developmental differences between educational levels of learners may have implications for instruction (Green and Azevedo Citation2007), there is little research that has examined the relationship between course delivery format and educational level of student (Billings, Skiba, and Connors Citation2005). The research that does exist has largely examined undergraduate and graduate differences. In relation to critical thinking skills specifically in learning online, graduate students have higher perceived skills as compared to undergraduate students (Artino and Stephens Citation2009). Billings, Skiba, and Connors found that undergraduate students perceived greater presence of interactions between students and faculty and more connection to their peers and instructor. In comparison, graduate students spend more time on task (Billings, Skiba, and Connors Citation2005; Artino and Stephens Citation2009) and more time on the course than undergraduates(Billings, Skiba, and Connors Citation2005). There were similar perceptions of satisfaction, adequacy as it related to computer skills, and preference for face-to-face courses for graduate students and undergraduate students (Billings, Skiba, and Connors Citation2005).
1.5. Purpose of the Study
Although extant research has compared student performance and other outcomes based on course delivery format, limited research has examined outcomes of students in introductory statistics courses (e.g., Kakish et al. Citation2012). Additionally, studies have traditionally used end-of-term grades as the measure of student performance which do not always serve as a valid proxy of statistical literacy and often have limited samples—such as one semester of data (e.g., Ward Citation2004; Kakish et al. Citation2012). Using more psychometrically rigorous assessments might allow researchers to better measure student learning outcomes and, thus, better assess the effectiveness of teaching formats. Multiple samples across several semesters might also make such results more reliable. Therefore, one purpose of the current study was to resolve these design issues. First, we used a validated measure of statistical literacy—the Comprehensive Assessment of Outcomes in Statistics (CAOS) (DelMas et al. Citation2007)—to assess students' baseline and end-of-course performance in an introductory statistics class. Second, we collected data from 27 semesters for students enrolled in introductory statistics courses. Given multiple semesters, multilevel modeling provided the vehicle through which we were able to examine the contextual effect of the classroom.
The primary purpose of our study was to investigate how instructional format relates to student learning outcomes (statistical literacy, more specifically) in an introductory masters-level statistics course. Specifically, we investigated the relationship between course delivery approach (hybrid versus online only) and students' statistical literacy, controlling for baseline statistical literacy and graduate level of the student (masters versus doctoral) as well as semester length (6-week versus 16-week courses).Our research question was as follows:
Is there a relationship between students' statistical literacy and course delivery format (hybrid and online) when controlling for student-level characteristics (baseline statistical literacy and graduate level of the student (masters versus doctoral)) and classroom context (6 week summer course versus 16 week fall or spring course)?
The present study attempts to overcome the limitations of past research investigating the multilevel nature (i.e., students within class section) and other methodological design issues of statistical literacy in several different ways. The current study uses a pretest, posttest longitudinal study design (with an attempt to measure student's end-of-semester statistical literacy controlling for statistical literacy with which they begin the semester) as opposed to a cross-sectional design. Baseline statistical literacy was included as a control variable given that examination was based on looking at how students perform at the end of the semester given where they are at baseline. Hence, student's final (measured at end-of-semester) level of statistical literacy can be modeled, while controlling for student's initial or baseline literacy (measured at beginning of semester).
2. Method
2.1. Description of Course
Participants in this study were enrolled in a graduate-level introductory statistics course in a College of Education at a large public research university located in the southeast United States. The course was part of the core curriculum for some graduate degrees in the college, but was also regularly taken as an elective or substitute for a similar course by masters and doctoral students from other colleges on campus as well as within the college. The course was designed to familiarize students with foundational skills in statistical analyses. The course placed emphasis on both understanding the concepts of basic analyses as well as choosing and conducting the appropriate analysis based on the research question and data. The course covered topics such as descriptive statistics, normal distribution, probability, hypothesis testing and simple univariate statistics, and the application of statistical software. There were no mathematics prerequisites for the course.
The course was offered using two different forms of instruction: fully online and mixed mode (hybrid). All students received the same content and materials, including access to the prerecorded lectures and online materials using the university's online class management system. In the hybrid class, students met every few weeks with the instructor, while completing online modules between formal class meetings. Approximately one-half of the content was delivered online while the other half was delivered in the face-to-face classroom. In the online course, there were no physical meetings. For students enrolled in the online section, their only contact with the instructor was via email and office hours.
Although the format varied, all classes had the same instructor, textbook, and coursework (e.g., assignments, projects, exams). The traditional classroom lectures used the same PowerPoint slides and outline as the online videos. Students in all classes had access to the instructor during weekly office hours.
2.2. Participants
Over 27 semesters (fall 2005 through summer 2015), 58% of the students in this study were enrolled in master's degree programs while the remaining 42% were in doctoral programs. Slightly more than one-half were enrolled in the online course (53%) the while the rest were in the hybrid course. Nearly three-fourths (72%) completed the course during a 16-week semester (i.e., fall or spring), while the remaining completed the course in a six-week format (i.e., summer).
2.3. Instrument
In order to measure students' statistical literacy, the Comprehensive Assessment of Outcomes in Statistics (CAOS) (DelMas et al. Citation2007) was administered as a pre- and posttest to all students who were enrolled in the course. The CAOS was developed as part of the Assessment Resource Tools for Improving Statistical Thinking (ARTIST), a project funded by the National Science Foundation (NSF) (DelMas et al. Citation2007).
The CAOS is a 40-item multiple-choice standardized instrument designed to measure students' understanding of statistical concepts appropriate to an introductory statistics course. Each item is related to a specific learning outcome. The instrument was developed over four iterations, the last of which was tested with a national sample of postsecondary students. Items were created and reviewed by postsecondary statistics instructors and leaders in statistics education (DelMas et al. Citation2007). Students complete the CAOS online, it is graded within the automated system, and instructors download a report that includes students' total score on the CAOS and time for completion of the CAOS.
A reliability analysis conducted by DelMas et al. (Citation2007) produced a coefficient alpha of 0.82—above Nunnally and Bernstein's (Citation1994) 0.80 criteria for acceptable reliability. University students made up 39.5% of total examinees for their analysis. In addition, 27.1% of all students were enrolled in a course that did not have a mathematics prerequisite, 41.6% required high school algebra, 28.6% required college algebra, and 2.8% required calculus. Sixty-four percent of the students took the posttest in the classroom. Students in the current study are all graduate-level university students taking a course without a mathematics prerequisite and took the CAOS pre- and posttest outside the classroom.
2.4. Data Collection Procedures
This study was conducted as a nonequivalent quasi-experimental group design as students self-selected the course format in which they enrolled (i.e., we could not randomly assign students to course format). All students were required to take the CAOS test as baseline and as posttest. The pretest was administered during the first week of class, and the posttest was administered during the final week of class. The CAOS is an online assessment, and students in both course formats (hybrid and online) completed the assessment when and where they chose, within the date range parameters for the assignment. Credit for the CAOS was based on completion rather than percentage correct.
In terms of additional control variables with respect to previous experiences as it relates to statistics, there have been attempts to gather these data and some data have been collected. However, that data are neither easily accessible nor complete, with data from many early semesters in the study not available. Thus, including this data in the current analyses was not attempted as it would ultimately result in a large proportion of cases, to the detriment of the study, being excluded.
2.5. Exclusions
Participants included students enrolled in an introductory hybrid or online graduate statistics class during fall 2005 through summer 2015 semesters (n = 1001). During early semesters, consent was required per the university's IRB, and thus this file was further refined to include only students who gave consent (n = 967) (for later semesters, IRB consent was not required as the assessments were required as part of the course). The file was also delimited to include only students who completed the course (n = 878), thus excluding students who withdrew (n = 88) or took an incomplete (n = 6). Students who had completed neither the pre- nor posttest (n = 11) were also excluded (resulting in n = 867).
Following the criteria which were used in the validation study (delMas et al. Citation2006, Citation2007), students who completed the pre- or postassessments that took at least 10 but no more than 60 minutes to complete the assessment were included in the analysis. Students who had missing data on the pre- or postassessment or time to complete the assessment had the score replaced as described in Section 3.1. The time limitation was imposed to eliminate students who may not have taken the test seriously and thus completed the test in less than 10 minutes or students who may have used books or other resources to look up answers and thus took a substantial amount of time to complete the test (>60 min). Although students were not required to answer every item, only in very few cases did students skip questions, and in those instances, it was usually only one or possibly two questions that were not answered. After these additional exclusions were applied, the resulting sample size was 718.
Lastly, there were 12 cases in which it was not possible to identify graduate level, course format, or semester in which the student was enrolled. This may have occurred, for example, in cases where a student audited the course and completed assignments but did not formally enroll in the course. These cases were removed from the datafile, resulting in a sample size of 706. The data were also delimited to include only students enrolled in a degree program (masters, specialists, or doctoral). Thus, 37 nondegree seeking students were excluded from the analyses (resulting in n = 669).
3. Results
3.1. Missing Data
There were no missing data on the categorical variables. Missing data on (i.e., failure to complete) the CAOS pretest were about 2% (n = 16) and CAOS posttest about 16% (n = 107). In some cases, the interpretability of pretest or posttest completion time was not possible. There were some instances where time was coded as a multiple digit value that could not be translated to time (e.g., 587893478). This was unresolvable by the owners of the assessment website, and thus these cases with un-interpretable times were coded as missing relative to time, and time was replaced using missing value replacement techniques described herein. Little's MCAR test was not statistically significant ( = 13.60, df = 14, p = 0.48), allowing us to assume that the data were completely missing at random (i.e., no identifiable pattern to the missing data). The missing values were imputed using the EM algorithm. EM algorithm for missing data replacement is an iterative process that produces maximum likelihood estimates with missing values estimated in an iterative fashion via a regression-based process with predictors including the variables included in the multilevel model (e.g., CAOS pre- and posttest scores, delivery format, course length) as well as additional variables (e.g., pre- and posttime to complete the CAOS) (Graham Citation2009).
3.2. Preliminary Analysis
Due to the nested nature of our data (i.e., students within class sections), we applied two-level multilevel linear modeling (MLM) to test our hypotheses, initially using restricted maximum likelihood estimation in HLM v. 7.01. Prior to computing the models, we tested the assumptions of the multilevel model which include: linearity; independence of the level 2 random effects and level 1 residuals; normality and constant variance of the level 1 residuals; and normality and homoscedasticity of the level 2 random coefficients.
The χ2 statistic indicated violation of homogeneity of the level 1 variance ( = 62.65, df = 26, p < 0.001). Heteroscedasticity is problematic in random slopes models (i.e., significant slope variances may be an artificial artifact of heteroscedasticity) (Snijders and Berkhof Citation2008), and thus was not of concern in this model. We plotted the unstandardized ordinary least squares (OLS) residuals against the level 1 predictors to check for possible nonlinear fixed effects. These results indicated both linearity and homogeneity of variance. We assessed the linearity and homoscedasticity of the level 2 random effects by examining the Empirical Bayes' residuals to the level 2 predictors. These results indicated both linearity and homoscedasticity.
The skewness (0.224) and kurtosis (2.531) were within acceptable ranges to establish normality for the level 1 residuals. The Kolmogorov–Smirnov test of normality, however, suggested a nonnormal distribution (K–S = 0.076, df = 669, p < 0.001). The graphs, including the histogram, Q-Q and boxplot, suggest potential outliers on each end of the scale. We assessed the multivariate normality of the level 2 effects by plotting the Mahalanobis distance and the expected values of the order statistics. The plot shows that the points generally adhere to a diagonal line suggesting evidence of normality.
3.3. Multilevel Results
Multilevel analyses were estimated using robust maximum likelihood estimation (MLR) in Mplus v. 7.4. MLR was selected as the estimation method given some initial evidence of nonnormality. The participants, as level 1 units, consisted of doctoral level students (n = 279) and masters level students (n = 390). The mean CAOS score of masters' students was slightly higher than doctoral students for both the pre- and posttest, and the gain from baseline to posttest is similar to that evidenced in the national sample (DelMas et al. Citation2007). For delivery formats, 18 courses (as level 2 groups) were delivered using a hybrid model, and 9 courses were taught solely online. The majority of courses were full-semester length (i.e., 16 week) delivered in mixed mode format (n = 16, 59%) followed by condensed semester online courses (n = 7, 26%). For both pretest and posttest, the average for students in the hybrid sections was similar to that for students in online sections (pretest, M = 47.51 and M = 48.01; posttest, M = 52.03 and M = 52.22, hybrid and online respectively). In addition, similar means were seen pre and post, regardless of the length of the course (pretest, M = 48.06 and M = 51.22; posttest, M = 51.22 and M = 52.88, 6 week and 16 week, respectively). The means and standard deviations are summarized in .
Table 1. Means and standard deviations of study variables.
3.3.1. Null Model
Prior to hypothesis testing, an unconditional model (i.e., null model with no predictors) was generated to determine the extent to which there was variation between class sections (i.e., an indicator of the need for examination in a multilevel framework). Statistical literacy was the dependent variable. The overall mean CAOS posttest score was 52.13. The intraclass correlation coefficient (ICC) represents the proportion of variance in the outcome between level 2 units (i.e., between class sections). For this study, the results also revealed that approximately 2.0% of the variance in statistical literacy resided between class sections (ICC = 0.020) and 98.0% of the variance was attributable to the individual. Although the variation between class section means did not meet the threshold for statistical significance (= 38.15, df = 26, p = 0.06), indicating there is not statistically significant variation between class sections, this analysis and the nature of our research questions supported using a multilevel model for estimation as data with as little as 1% of the variance residing between groups has been shown to be meaningful (Barcikowski Citation1981).
3.3.2. Contextual Model
The multilevel results are presented in . Because baseline knowledge may affect our results, we first estimated a random intercepts fixed slopes model (i.e., modeling the intercepts as varying randomly across class sections but modeling the slopes as fixed across class section) with the statistical literacy pretest entered as a grand-mean centered level 1 control variable (labeled Model 1 in ). As expected, the statistical literacy pretest is significantly related to the posttest scores ( = 0.66, p < 0.001). Second, we estimated a random intercepts random slopes model (i.e., modeling the intercepts as varying randomly across class sections and modeling the slopes as varying randomly across class sections; with random slopes, we assume the effects of the covariates vary across class section) with the pretest entered as a grand-mean centered level 1 variable. In the new model, the variance between class sections increased indicating that a fixed slopes model fits better and all further analyses include fixed slopes.
Table 2. Multilevel modeling coefficient (standard error) and model fit results for statistical literacy posttest.
In addition to baseline knowledge, graduate level (masters vs. doctoral) may relate to statistical literacy. Thus, we estimated a random intercepts fixed slopes model with a dummy coded degree program classification variable (masters or doctorate) entered as an uncentered level 1 control variable. There was no statistically significant relationship (i.e., insufficient evidence of a relationship) ( = 0.38, p = 0.73) between degree program classification and statistical literacy measured at the end of the course, controlling for the pretest (Model 2 in ). The primary variable of interest, course delivery format (online versus hybrid), was a group-level variable. This was also a binary variable and entered into the random intercepts, fixed slope model as a level 1 uncentered predictor and was not statistically significantly related to posttest statistical literacy (
= −0.21, p = 0.79) (Model 3, ).
Length of the class (6 weeks vs. 16 weeks) was a group-level variable entered uncentered as a control variable at level 2 in the random intercepts, fixed slope model. The model that includes length of course suggests only baseline literacy is a statistically significant predictor of end-of-course statistical literacy (Model 4 in ).
The final model estimated was as follows:
Level 1:where POST TESTij is the statistical literacy posttest score for student i in class j,
is the adjusted mean posttest score in semester j when a student's pretest score is equal to the grand mean pretest score, controlling for degree level and course format,
is the effect of the statistical literacy pretest,
is the effect of the degree level, with masters coded as 1,
is the effect of course format, with online coded as 1, and
is the random variation in the posttest score attributed to student i in class j.
Level 2:where
is the adjusted mean posttest score of students in online, 16-week courses,
is the effect of course length, with 16-week term coded as 1,
is the random variation in the posttest score attributed to semester j,
is the pooled within-group coefficient of the pretest,
is the pooled within-group coefficient of master's level, and
is the pooled within-group coefficient of online course format.
Final, Mixed Model:
Results suggest that students enrolled in a full-term (i.e., 16-week) performed similar to students in the summer term (i.e., 6-week) ( = 1.56, p = 0.31). Additionally, course delivery format (hybrid versus online) is not statistically significantly related to statistical literacy at posttest (
= −0.21, p = 0.79). In addition, there was no statistically significant variation between the semester means (
= 0.88, p = 0.50). This suggests that average statistical literacy was quite similar between the semesters. The model fit statistics (AIC, BIC, SBIC) suggest the more parsimonious models have better fit. This is not surprising given the nonstatistically significant results. The model was defined and built based on theoretical importance rather than model fit criteria (Raftery Citation1995). The results, relative to the previous models tested, are labeled as Model 4 in .
4. Discussion
Our research examined the extent to which course modality relates to students' statistical literacy. Some research has found significant differences in student learning between face-to-face and purely online instruction (Keefe Citation2003) and face-to-face relative to both hybrid and online (Scherrer Citation2011). Our study, however, examined hybrid and online learning. Our results showed that there was no significant difference in statistical literacy of students who are enrolled in hybrid, as compared to online, introductory statistics course when controlling for baseline statistical literacy, graduate level of the student (masters versus doctoral) and length of course (full term versus condensed). This result is consistent with some previous research (Sami Citation2011), which suggests that instructional delivery format (face-to-face, hybrid, online) is not related to student learning in introductory statistics courses.
Although our primary interest was investigating course delivery format, we recognized that there may be other factors that influence student learning. Thus we used theoretically and empirically derived control variables that were potentially related to our results. In terms of course length, students who completed the course during a full-term (16 weeks) scored similar in statistical literacy at the end of the semester as their counterparts who took the course over six weeks. This suggests that there is not a differential effect on ending statistical literacy for students who may have an opportunity to spend more time with the material. Past research has indicated the relationship between graduate student status and enrollment in hybrid classes as influencing student learning outcomes and satisfaction (e.g., Parker Citation2009; Wisker, Robinson, and Shacham Citation2007). Although we are not aware of studies that have measured the impact of course length on statistical literacy specifically, our results are in line with many findings that suggest student performance in abbreviated semesters is at least as good, if not better, than performance from a term of regular length (e.g., Seamon Citation2004; Shaw et al. Citation2013; Austin and Gustafson Citation2006; Anastasi Citation2007; Daniel Citation2000).
5. Limitations and Future Research
This study is not without some limitations. In light of these limitations, we encourage the reader to view these as opportunities for future research. First, the CAOS was a self-administered assessment. We took several steps outlined in the delimitations section to ensure the quality of our data, however, future studies should consider administering the CAOS in a controlled environment. Second, other variables that were not measured in this study might contribute to this result. For instance, practical variables such as instructional media features and computer-mediated communication between students and instructor or between peers may relate to statistical literacy performance. We should note, however, that one significant strength of our study over previous research is that we held constant the instructor and instructional materials, ensuring all students had access to like resources. Indeed, the similarity in statistical literacy between online and hybrid students mimics the meta-analytic findings of smaller effects between online and face-to-face conditions when curriculum and instruction was the same (Means et al. Citation2010). With that said, it is possible that student attributes (e.g., intelligence, personality, self-efficacy) which were not measured in this study may related to learning outcomes and should be considered for inclusion in future research. Finally, our study was limited to graduate-level students enrolled in masters or doctoral programs. These students are likely to be higher achieving and more motivated than the average undergraduate and, therefore, our results may not generalize to students enrolled in undergraduate programs. Future research that expands the level of student is suggested. Additionally, future research on this topic should examine the interaction effect of delivery mode and delivery length and examining different pedagogical strategies for delivering content in compressed or accelerated learning environments. Finally, future research should corroborate and further examine our findings on course length as a primary variable.
6. Conclusion
The purpose of this study was to examine the effects of course delivery format on statistical literacy in graduate education. Specifically, we sought to improve and expand on previous research by designing a rigorous quasi-experiment comparing online to hybrid classes using psychometrically sound measures while controlling as potentially important explanatory variables to isolate the influence of course modality. Our findings complement previous research—which has primarily compared either online or hybrid formats to traditional face-to-face—by suggesting there is no difference between online and hybrid courses on student learning. This is in contrast to some studies which have suggested students in hybrid classes outperform students in online courses (Means et al. Citation2010). These findings are important to both instructors and policy-makers as time and funding continue to become more limited in higher education.
References
- Allen, I. E. and Seaman, J. (2015), Grade Level: Tracking Online Learning in the United States, Wellesley, MA: Babson Survey Research Group and Quahog Research Group, LLC.
- Anastasi, J. S. (2007), “Methods and Techniques: Full-Semester and Abbreviated Summer Courses–An Evaluation of Student Performance,” Teaching of Psychology, 1, 19–22.
- Artino, A. R. and Stephens, J. M. (2009), “Academic Motivation and Self-Regulation: A Comparative Analysis of Undergraduate and Graduate Students Learning Online,” Internet and Higher Education, 12, 146–151.
- Austin, A. M. and Gustafson, L. (2006), “Impact of Course Length on Student Learning,” Journal of Economics and Finance Education, 1, 5, 26–37.
- Bailey, K. D., and Morais, D. B. (2005), “Exploring the Use of Blended Learning in Tourism Education,” Journal of Teaching in Travel and Tourism, 4, 4, 23–36.
- Barcikowski, R. S. (1981), “Statistical Power with Group Mean as the Unit of Analysis,” Journal of Educational Statistics, 3, 6, 267–285.
- Billings, D. M., Skiba, D. J., and Connors, H. R. (2005), “Best Practices in Web-based Courses: Generational Differences Across Undergraduate and Graduate Nursing Students,” Journal of Professional Nursing, 2, 21, 126–133.
- Bowen, W. G., Chingos, M. M., Lack, K. A., and Nygren, T. I. (2014), “Interactive Learning Online at Public Universities: Evidence from a Six-Campus Randomized Trial,” Journal of Policy Analysis and Management, 33, 94–111.
- Brown, D. G. (2001), “Hybrid Courses are Best,” Syllabus Magazine, 15, 22.
- Burbules, N. C. and Callister, T. A. (2000), “Universities in Transition: The Promise and the Challenge of New Technologies,” Teachers College Record, 102, 271–293.
- Carnevale, D. (2002), “Online Students don't Fare as Well as Classroom Counterparts, Study Finds,” The Chronicle of Higher Education, 48, 38.
- Chametzky, B. (2013), Offsetting the Affective Filter: A Classic Grounded Theory Study of Post-secondary Online Foreign Language Learners. Prescott Valley, AZ: Northcentral University.
- Chance, B. (2002), “Components of Statistical Thinking and Implications for Instruction and Assessment,” Journal of Statistics Education, 10.
- Daniel, E. L. (2000), “A Review of Time-Shortened Courses Across Disciplines,” College Student Journal, 34, 298–306.
- delMas, R. C. (2002), “Statistical Literacy, Reasoning, and Learning: A Commentary,” Journal of Statistics Education, 10.
- delMas, R., Garfield, J., Ooms, A., and Chance, B. (2006), “Assessing Students' Conceptual Understanding After a First Course in Statistics,” Paper presented at American Educational Research Association Annual Conference, April 9 at San Francisco, CA.
- DelMas, R., Garfield, J., Ooms, A., and Chance, B. (2007), “Assessing Students' Conceptual Understanding after a First Course in Statistics,” SERJ, 2, 28–58.
- Dereshiwsky, M. I. (1998), “Go Figure: The Surprising Successes of Teaching Statistics Courses via the Internet,” in Proceedings of the Teaching in the Community Colleges Online Conference, Honolulu, HI.
- Dutton, J. and Dutton, M. (2005), “Characteristics and Performance of Students in an Online Section of Business Statistics,” Journal of Statistics Education, 3.
- Dutton, J., Dutton, M., and Perry, J. (2001), “Do Online Students Perform as well as Lecture Students?,” Journal of Engineering Education, 90, 131–136.
- Dziuban, C. D. (2016), “Assessing Outcomes in Online and Blended Learning Research,” in Conducting Research in Online and Blended Learning Environments, eds. C. D. Dziuban, A. G. Picciano, C. R. Graham, and P. D. Moskal, New York, NY: Routledge Taylor and Francis, pp. 158–172.
- Ferguson, J. M. and DeFelice, A. E. (2010), “Length of Online Course and Student Satisfaction, Perceived Learning, and Academic Performance,” International Review of Research in Open and Distance Learning, 2, 73–84.
- Gal, I. (2002), “Adults' Statistical Literacy: Meanings, Components, Responsibilities,” International Statistical Review, 70, 1–25.
- Garfield, J. (2002), “The Challenge of Developing Statistical Reasoning,” Journal of Statistics Education, 10.
- Graham, J. W. (2009), “Missing Data Analysis: Making it Work in the Real World,” Annual Review of Psychology, 60, 549–576.
- Green, J. A. and Azevedo, R. (2007), “A Theoretical Review of Winne and Hadwin's Model of Self-Regulated Learning: New Perspectives and Directions,” Review of Educational Research, 77, 334–372.
- Haughton, J. and Kelly, A. (2015), “Student Performance in an Introductory Business Statistics Course: Does Delivery Mode Matter?,” Journal of Education for Business, 90, 31–43.
- James, S., Swan, K., and Daston, C. (2016), “Retention, Progression and the Taking of Online Classes,” Online Learning, 2, 75–96.
- Kakish, K., Pollacia, L., Heinz, A., Sinclair, J. L., and Thomas, A. (2012), “Analysis of the Effectiveness of Traditional Versus Hybrid Student Performance of an Elementary Statistics Course,” International Journal for the Scholarship of Teaching and Learning, 6, 1–9.
- Keefe, T. J. (2003), “Using Technology to Enhance a Course: The Importance of Interaction,” EDUCAUSE Quarterly, 26, 24–34.
- Kwak, D. W., Menezes, F. M., and Sherwood, C. (2015), “Assessing the Impact of Blended Learning on Student Performance,” Economic Record, 91, 91–106. doi: 10.1111/1475-4932.12155.
- Lee, N., and Horsfall, B. (2010), “Accelerated Learning: A Study of Faculty and Student Experiences,” Innovative Higher Education, 35, 191–202.
- Margulieux, L. E., Bujak, K. R., McCracken, W. M., and Majerich, D. M. (2014), “Flipped, Blended, Flipped, and Inverted: Defining Terms in a Two Dimensional Taxonomy,” Paper presented at 12th Annual Hawaii International Conference on Education, January 5–9, at Honolulu, HI.
- Means, B., Toyama, Y., Murphy, R., Bakia, M., and Jones, K. (2010), Evaluation of Evidence-Based Practices in Online Learning: A Meta-Analysis and Review of Online Learning Studies, Washington, DC: U.S. Department of Education.
- Mensch, S. (2013), “The Impact of Course Length on Online Numeric-Based Course Grades,” Contemporary Issues in Education Research, 6, 4.
- Mortenson, T. G. (2005), State Tax Fund Appropriations for Higher Education: FY1961 to FY2005. Oskaloosa, LA: Postsecondary Education Opportunity.
- Nunnally, J. C. and Berstein, I. H. (1994), Psychometric Theory (3rd ed.), New York: McGraw-Hill.
- Oblender, T. E. (2002), “A Hybrid Course Model: One Solution to the High Online Drop-out Rate,” Learning & Leading with Technology, 6, 42–46.
- Onwuegbuzie, A. J. (2001), “Critical Thinking Skills: A Comparison of Doctoral- and Master's-Level Students,” College Student Journal, 3, 477–481.
- Parker, R. (2009), “A Learning Community Approach to Doctoral Education in the Social Sciences,” Teaching in Higher Education, 1, 14, 43–54.
- Picciano, A. G. (2015), “Research in Online and Blended Learning: New Challenges, New Opportunities,” in Conducting Research in Online and Blended Learning Environments, eds. C. D. Dziuban, A. G. Picciano, C. R. Graham, and P. D. Moskal, New York: Routledge Taylor & Francis, pp. 1–11.
- Postman, N. (2011), Technopoly: The Surrender of Culture to Technology, New York: Random House Digital, Inc.
- Raftery, A. E. (1995), “Bayesian Model Selection in Social Research,” Sociological Methodology, 25, 111–163.
- Rumsey, D. J. (2002), “Statistical Literacy as a Goal for Introductory Statistics Courses,” Journal of Statistics Education, 10.
- Russell, T. L. (2001). The No Significant Difference Phenomenon (5th ed.), Chapel Hill, NC: Office of Instructional Telecommunication, North Carolina State University Press.
- Sami, F. (2011), “Course Format Effects on Learning Outcomes in an Introductory Statistics Course,” MathAMATYC Educator, 2, 48–51.
- Scherrer, C. R. (2011), “Comparison of an Introductory Level Undergraduate Statistics Course Taught with Traditional, Hybrid, and Online Delivery Methods,” INFORMS Transactions on Education, 11, 106–110.
- Scherrer, C. R. (2015), “Comparison of an Introductory Level Undergraduate Statistics Course Taught with Traditional, Hybrid, and Online Delivery Methods,” INFORMS Transactions on Education, 11, 106–110.
- Scott, P. A. (1996), “Attributes of High-Quality Intensive Course Learning Experiences: Student Voices and Experiences,” College Student Journal, 30, 69–77.
- Seamon, M. (2004), “Short- and Long-Term Differences in Instructional Effectiveness Between Intensive and Semester-Length Courses,” Teachers College Record, 4, 106, 852–874. doi: 10.1111/j.1467-9620.2004.00360.x.
- Shaw, M., Chametzky, B., Burrus, S. W., and Walters, K. J. (2013), “An Evaluation of Student Outcomes by Course Duration in Online Higher Education,” Online Journal of Distance Learning Administration[ online]. Available at http://www.westga.edu/∼distance/ojdla/winter164/shaw_chametzky_burrus_walters164.
- Snijders, T. A. B. and Berkhof, J. (2008), “Diagnostic Checks for Multilevel Models,” in Handbook of Multilevel Analysis, eds. J. de Leeuw, and E. Meijer, New York: Springer, pp. 141–175.
- Star, S. L. and Griesemer, J. R. (1989), “Institutional Ecology, Translations and Boundary Objects: Amateurs and Professionals in Berkeley's Museum of Vertegrate Zoology, 1907–39,” Social Studies of Science, 3, 387–420.
- Twigg, C. A. (2013), “Improving Learning and Reducing Costs: Outcomes from Changing the Equation,” Change: The Magazine of Higher Learning, 45, 6014.
- Utts, J., Sommer, B., Acredolo, C., Maher, M. W., and Matthews, H. R. (2003), “A Study Comparing Traditional and Hybrid Internet-Based Instruction in Introductory Statistics Classes,” Journal of Statistics Education, 3.
- Ward, B. (2004), “The Best of Both Worlds: A Hybrid Statistics Course,” Journal of Statistics Education, 3.
- Watson, J. and Callingham, R. (2003), “Statistical Literacy: A Complex Hierarchical Construct,” Statistics Education Research Journal, 2, 3–46.
- Watson, J. M. (2011), “Foundations for Improving Statistical Literacy,” Statistical Journal of the IAOS, 27, 197–204. doi: 10.3233/SJI20110728.
- Wisker, G., Robinson, G., and Shacham, M. (2007), “Postgraduate Research Success: Communities of Practice Involving Cohorts, Guardian Supervisors, and Online Communities,” Innovations in Education and Teaching International, 44, 301–320.
- Young, J. R. (2002), “‘Hybrid’ Teaching Seeks to End the Divide Between Traditional and Online Instruction,” The Chronicle of Higher Education, 48, A33–A34.