ABSTRACT
Because of their efficiency and ability to keep many other factors constant, twin studies have a special appeal for investigators. Just as with any teaching dataset, a “matched-sets” dataset used to illustrate a statistical model should be compelling, still relevant, and valid. Indeed, such a “model dataset” should meet the same tests for worthiness that news organization editors impose on their journalists: are the data new? Are they true? Do they matter? This article introduces and shares a twin dataset that meets, to a large extent, these criteria. In fact, while more than two decades old, the data are still widely cited today in ongoing related research. This dataset was the basis of a clever study that confirmed an inspired hunch, changed the way pregnancies in HIV-positive mothers are managed, and led to reductions in the rates of maternal-to-child transmission of HIV.
1. Introduction
Because of their efficiency and ability to keep many other factors constant, twin studies have a special appeal for students, teachers, and investigators. Since it is difficult to assemble a sufficient number of twin pairs in a short time, investigators often use a “next best” design, namely, pairs or sets of siblings, neighbors, etc., matched on key potential confounding factors. Variables that cannot be matched on are usually handled using conditional (i.e., within-set) regression adjustments.
The infertility dataset in the R datasets package is often used along with the clogit function in the Survival package to illustrate conditional logistic regression. The dataset contains data from a matched case–control study (Trichopoulos et al. Citation1976) “dating from before the availability” of this type of regression. It is of little surprise therefore, that current students see these data as somewhat outdated. And there is another reason the infertility dataset is a less than ideal teaching example: conclusions made based on these data have since been refuted.
Together with similar case–control data from a tightly-matched study (Panayotou et al. Citation1972), it was suggested that about 45% of the cases of secondary infertility in Greece, and half of ectopic implantations in Athens, “may be attributable to previous induced abortions.” It is important to note that these conclusions relied on self-reported abortion histories. Investigators would eventually become aware of the dangers of relying on self-reported abortion histories and the need to conduct analysis appropriately, see, for example, Melbye et al. (Citation1997) and Rookus and van Leeuwen (Citation1996).
Unfortunately, it was not until the 1990s that, with better data and improved methods, Trichopoulos et al.’s (Citation1976) findings were “considered as an outlier by most investigators, who were inclined to attribute it to chance or to the fact that induced abortions were, at the time, illegal in Greece,” Tzonou et al. (Citation1993), and the relation between induced abortion and ectopic pregnancy was put into question, Atrash et al. (Citation1997). Despite this objective evidence, the infertility dataset continues to be used as a “model” dataset for teaching in textbooks, tutorials, and in the classroom, see, for example, Jewell (Citation2003), Günther and Fritsch (Citation2010), Aragon et al. (Citation2010), and Kerns (Citation2011).
In this article, we provide a teaching dataset containing twin data that are relatively new, that still appear to be true, and that clearly matter. In fact, while more than two decades old, the data continue to be widely cited today in ongoing related research; see, for example, Makunyane, Moodley, and Titus (Citation2016), Lion-Cachet (Citation2016), Milligan and Overbaugh (Citation2014), and Frange and Blanche (Citation2014). We have used these twin data both in introductory courses, for simple comparisons involving paired binary outcomes, and in regression courses that deal with correlated binary outcomes.
2. The International Registry of HIV-Exposed Twins
2.1. Background
What started as a small observational database in late 1990, became an international registry of over 200 pairs of twins and the first evidence suggesting a link between mode of delivery and mother-to-child HIV transmission, Katz (Citation2003). It is no exaggeration to say that as a result of this dataset, standard practices for the care of pregnant women with HIV promptly changed and as a consequence, many lives were saved. In 1991, analysis based on the data suggested that “caesarean delivery may be helpful” in reducing rates of transmission, a conclusion that would be confirmed in a 1999 meta-analysis (International Perinatal HIV Group Citation1999). Besides containing 24 lines of data that themselves make an ideal teaching dataset, Table 3 in the 1999 meta-analysis (International Perinatal HIV Group Citation1999) is a testament to the impact of following up on an anecdote involving just one pair of twins. Only a few years later, following the widespread use of the drug zidovudine in 1994, rates of perinatal HIV in industrialized countries dropped dramatically. In developing countries, attention focused on identifying effective, simple, and affordable interventions, McGowan and Shah (Citation2000). (Challenges remained as not all proposed interventions were found to be successful. Biggar et al. (Citation1996) examined the efficacy of birth canal washing for reducing perinatal transmission in Malawi and found no significant reductions.)
In a March 1993 interview for the “In Their Own Words” project—that documents the work of researchers at the National Institutes of Health (NIH) during the early days of the HIV/AIDS epidemic, Harden and Rodrigues (Citation1993)—Dr. James Goedert, M.D., NIH medical researcher, recalled how the registry originated:
I was invited by the Pediatric AIDS Foundation to go to a meeting in California on risk factors for pediatric AIDS and different kinds of immune response. […] Because of the time difference between California and the East Coast, the first morning I was there at a hotel on the beach I woke up very early. I took a long walk on the beach. I was trying to put together the things I had heard from the day before and make some kind of sense out of them. It was a good time for thinking; the sunrise was not quite over the Pacific, but coming over the mountains in Santa Barbara.
There had been one anecdote of twins being born that someone [Dr. Arye Rubenstein] had mentioned, where one had been infected and the other had not. It seemed to me a possible way to distinguish when the infection occurs; whether it has already occurred before the woman goes into labor, in which case the chance of infection should be random for the first-born and the second-born twin; or whether, as I suspected, again wrongly, the virus was transmitted during separation of the placenta, in which case the second-born should be at higher risk because they are in the womb longer, they are exposed for a longer time.
After assembling a group of collaborators with whom to pursue his idea, invitations to submit data were initially sent to 154 investigators of pediatric AIDS around the world in late 1990. Contributors, including pediatricians, obstetricians, and infectious disease specialists, were asked to provide demographic, clinical, and epidemiological data on sets of HIV-1-infected women and their twin or triplet offspring. Data were abstracted from clinical records and submitted on standardized forms with follow-up forms sent out for any required additional information. All the data obtained was anonymous with no names or identifying information. The definition used for HIV status was based on the Centers for Disease Control classification system; further details in Goedert et al. (Citation1991) and CDC (Citation1987).
Dr. Goedert recalled his excitement when the data first started arriving through the FAX machine: “Every day was like Christmas. I would come in here and there would be a FAX waiting for me, or maybe two, or three, or four, with more data.” Indeed, the FAX machine was indispensible: “It is the one study I have worked on in which 99 percent of it was conducted by FAX machine. […] Without the FAX machine that study would never have been done. We literally faxed out invitation letters with forms, and people faxed the forms back in.”
Results from analysis using the registry data were first published in a poster presented at the VIIth International Conference on AIDS in June 1991 in Florence Italy, Goedert, Duliège and Amos (Citation1991). This first report, based on 82 sets of twins and 1 set of triplets (50 of which had “complete data”) served to welcome new contributors to the registry.
In December 1991, with 101 sets obtained, analysis results were published in the Lancet, Goedert et al. (Citation1991). This milestone article presented strong evidence against the null (p-value = 0.004), suggesting a higher risk of HIV-1 infection for first-born twins. The study was among the first to suggest the link between mode of delivery and perinatal transmission of HIV. As a result, it was highly cited and even reported in the New York Times, Warren (Citation1992).
There was however, some criticism received. In response to the Lancet article, Dr. Marc Bulterys and colleagues at the National University of Rwanda suggested that the results may be due to selection bias, Bulterys et al. (Citation1992): “The much higher risk of HIV-1 infection among first-born twins […] was apparent only among twins identified because of an HIV-1 related illness in at least one twin and not among twins identified because the mother was known to be infected. Selection bias would occur if second-born twins who were HIV-1 infected were more likely to die early and thus would be excluded from the analysis.” In a response Goedert wrote: “We hope that [more] prospectively collected data […] will be contributed to the registry.”
Helpful Hint: Use this criticism as an opportunity to discuss the subtleties of selection bias. Ask students why selection bias could occur with the registry data that was not collected prospectively. Remind students that second-born twins are usually lighter than first-borns and thus their infant mortality is higher.
Data continued to be collected, and in June 1993, an updated analysis was published based on 147 sets of twins and 2 sets of triplets, Goedert et al. (Citation1993). By December 1, 1993, the registry included 203 contributed sets from collaborators in 14 different countries. These included 148 sets with “complete data,” including 115 sets of twins ascertained prospectively. Courtesy of Dr. Goedert and the computer programmer Myhanh Dotrang, who shared it with us in 2011, this is the hivtwins dataset now being made publically available for teaching purposes. Results based on the analysis of the prospectively identified twins were published in the Journal of Pediatrics, Duliège et al. (Citation1995). The analysis confirmed the higher risk of HIV infection among first-born twins. What is more, the research concluded that intrapartum transmission is likely responsible for the majority of pediatric HIV infections, and that reducing exposure to HIV in the birth canal could reduce transmission of the virus between mother and infant: “the protective effect of cesarean delivery is real and clinically meaningful.”
For work on the International Registry of HIV-Exposed Twins, Dr. Goedert received the Public Health Service Outstanding Service Medal and the International AIDS Society 1992 International Life Prize. While today, the original Lancet study has been rightfully overshadowed by the effectiveness of antiretroviral therapy, it remains an important example of the merits of using twin pairs to gain initial insights, an approach used throughout Dr. Goedert's career, see, for example, Cozen et al. (Citation2013).
2.2. The Data
The hivtwins dataset includes 201 sets of twins and 2 sets of triplets. As such, two sets of twins from the registry are missing in the current hivtwins dataset. Thirty-one variables are included, including “in94paper,” which identifies the 115 sets with complete data that were ascertained prospectively (by follow-up of infants born to HIV-infected mothers).
While the particular measures taken by the NIH with respect to confidentiality and informed consent are difficult to ascertain today, students should certainly not be given the impression that these matters can be simply overlooked. Neff (Citation2008) provided a simple and straightforward discussion on these matters with regards to chart review, case reports, and observational studies.
For the current release of the data, we altered two variables to reduce (even further) the risk of anyone identifying any of the mother–infant pairs. The data-dictionary we received from Dr. Goedert contained the codes (001-235) that identified the physicians (and the cities they practiced in) who “contributed” the information. We have left the codes in the dataset, but have removed the names of corresponding physicians and cities. Some students or teachers may want to make a point of checking whether any physician contributed more than 1 mother-twin/triple, and what the statistical implications would be if they did. The original dictionary also identified the country of origin for each observation. We have replaced the 17 countries with the continents. This further anonymized the data while maintaining the possibility for analysts to reproduce published results.
The variables of primary interest in the dataset are: the HIV status of each infant, the delivery method of each infant, and an indicator of whether or not the twins/triplets were identical. Information about the mother is also available and includes: the mother's race, the number of weeks pregnant, the mother's AIDS diagnosis, and her potential HIV risk factors. Finally, the data include variables of birth weight, birth length, and head circumference measurements for the majority of the infants as well as a binary indicator of whether or not the child was breastfed. , described in the next section, provides a summary of the data. , a compass plot, shows the proportion of 1st and 2nd born infants with positive HIV-1 status given delivery method.
Table 1. Replication of the table “Factors for mother-to-infant transmission of HIV-1 infection in 115 prospectively identified twin sets” as published in Duliège et al. (Citation1995), created using the hivtwins dataset.
Figure 1. “Compass plot” shows rates of HIV-1 infection for first born and second born twins given method of delivery; based on data of Duliège et al. (Citation1995).
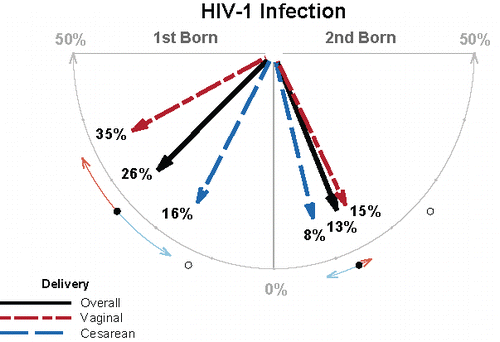
2.3. Replication of the Published Results
With the hivtwins dataset we can easily reproduce the Table “Factors for mother-to-infant transmission of HIV-1 infection in 115 prospectively identified twin sets” as published in Duliège et al. (Citation1995); see . The code to reproduce this table is provided in the Appendix, available in the online supplementary information. Only two differences with the originally published table will be apparent. First, missing are weight measurements for two sets of twins with “same” birth weight. Second, the variable to determine the presence of an HIV infected sibling is missing from hivtwins. Also of note, “Mother's route of infection—Sexual/other” could be calculated differently depending on how one categorizes missing data (i.e., entries of “NA,” “Unknown”).
Potential Pitfall: You may wish to ask students to reproduce the Table. Try not to get distracted by small differences that may occur due to minor choices such as how to calculate birth weight concordance and how to categorize missing entries.
2.4. Simple Analyses, and Discussion of Effect Measures, Suitable for Introductory Courses
The display and analysis of paired binary responses can be challenging. This is particularly so if the sampling is based on outcome, as in case–control studies, but even if it is based on exposure, it is still not that simple. In , we have HIV status for the 115 pairs of twins summarized in a standard two-by-two table. To test if the infection of one twin is independent of the other, we employ the Chi-squared test (Chi-squared = 17.252, df = 1, p-value = 0.000033). Not surprisingly, there is strong evidence in favor of association.
Table 2. The two-by-two table summarizes HIV status for the 115 pairs of twins.
In order to answer the more interesting question—whether or not the infection rate is the same among first and second borns—we can employ McNemar's test. McNemar's test determines if the marginal proportions are significantly different. In this case, is 13% ( = 15/115) significantly different than 26% ( = 30/115)? To answer this, we need only consider the discordant twins (i.e., 4 and 19), the 11 cases in which both the first and second born are infected do not provide additional information. With a continuity correction, we obtain Q = (|4−19| – 1)2/(4+19) = 8.52. Under the null, Q follows a chi-squared distribution with 1 degree of freedom. Therefore, we have a p-value of 0.0035 indicating strong evidence against the null. Fay (Citation2015) provided a good explanation of McNemar's test for both twin data and case–control data with accompanying R code.
We have used the data in in introductory courses to show how to display and compare binary outcomes in paired observations. Whereas many introductory courses merely handwave, and state—without proof or discussion—that the “a” and “d” frequencies in the concordant cells are “uninformative,” teachers may wish to motivate this “McNemar” approach. For example, they can ask what would happen if the paired binary responses were analyzed with a paired t-test. The results may surprise students and prompt some teachers to locate and consult McNemar's original article, McNemar (Citation1947). One can also ask what is the most natural parameter in these situations (i.e., the number that an infinite amount of data would converge to). Is it the absolute difference in two proportions, or their ratio? Why, in this context, do statisticians focus so much on the odds ratio? Is it because the single parameter of the noncentral hypergeometric distribution obtained by conditioning on both margins of a 2 × 2 table is the ratio of the two odds corresponding to the two binomial parameters (i.e., the odds ratio [P1/(1−P1)]/[P2/(1−P2)])? This discussion can lead students toward the simpler P1/P2 comparative parameter, and in nonexperimental contexts, to binary regression models with a log rather than a logistic link. It may also lead one to consider the possibilities available with “marginal” models. What if all infants in the dataset had been (or were to be) delivered by one method?
2.5. More Complex, Regression-Based Analyses
The main analysis results published in Duliège et al. (Citation1995) relied on “quasi-likelihood modeling” to estimate the marginal probability of infection with adjusted odds ratios (and 95% confidence intervals). The authors cite the work of Qaquish and Liang (Citation1992) for their methods. While it may be difficult to use this methodology today within existing software, it is most pleasing that the main result (i.e., “the odds ratio of HIV-1 infection for A twins compared with B twins was 2.4 (CI: 1.4 to 4.0),” Duliège et al. Citation1995) can be reproduced identically by estimating parameters for a marginal model with Generalized Estimating Equations (GEE). Using R's geeglm function within the geepack library, Halekoh, Højsgaard, and Yan (Citation2006), we have:
> library(geepack)
> GEEmodel<-(geeglm(HIV∼ First, id = BATCHID, family = binomial(), data = twins94_long, corstr = “exchangeable”))> round(exp(confint.geeglm(GEEmodel)),1)
lwr95CI Estimate upr95CI(Intercept) 0.1 0.2 0.3First 1.4 2.4 4.0
The risk ratio is another important estimate to consider. In this case, the risk ratio, equal to 2.0, is very intuitive as there are 30 infected first-born twins versus 15 infected second-born twins. It is fit in a binary regression with a log link, where log(P) = log(P2) + Log(P1/P2) x I, and I = 0/1 indicates whether (1) or not (0) the twin is born first. The exponentiated value of the fitted coefficient of I yields the estimate of the “risk ratio” parameter P1/P2.
> GEEmodelRR<-(geeglm(HIV∼ First, id = BATCHID, family = binomial(“log”), data = twins94_long, corstr = “exchangeable”))> round(exp(confint.geeglm(GEEmodelRR)),1)
lwr95CI Estimate upr95CI(Intercept) 0.1 0.2 0.3First 1.4 2.4 4.0
Using GEE, we can also obtain the same coefficient estimates and confidence intervals as those published in Duliège et al. (Citation1995) for mode of delivery. Consider the following R code:
GEEmodel1<-(geeglm(HIV∼ DELIVERY+First,id = BATCHID, family = binomial(), data = na.omit(twins94_long2 [twins94_long2$DELIVERY! = “VAG_CAES”,]), corstr = “exchangeable”)) round(exp(confint.geeglm(GEEmodel1)),1)
lwr95CI Estimate upr95CI (Intercept) 0.0 0.1 0.2DELIVERYVAGINAL 1.1 2.7 6.6 First 1.6 2.8 5.0
Duliège et al. (Citation1995) concluded: “Vaginal delivery was associated with an approximately twofold greater risk of transmission than cesarean delivery […] the adjusted odds ratios of infection were […] 2.8 (CI: 1.6 to 5.0) for first birth, and 2.7 (CI: 1.1 to 6.6) for vaginal delivery.”
The complete R code is provided in the Appendix. Replicating the published odds ratios by quasi-likelihood methods may be an interesting challenge for advanced students, with the understanding that statistical modeling of correlated data has evolved quite substantially since the original publication. Hanley, Negassa, and Forrester (Citation2003) provided an accessible introduction to GEE models, while Sjölander et al. (Citation2012) provided an overview of various analysis methods for correlated binary data with special attention to the analysis of twin studies.
Helpful Hint: Consider using the estimates obtained as an opportunity to discuss the differences between marginal and conditional models, see Gardiner, Luo, and Roman (Citation2009); as well as the choice between Odds Ratio and Risk Ratio metrics, see Schmidt and Kohlmann (Citation2008).
3. Conclusion
As with urban myths, data, data-analyses, and remembered results persist even when they have been subsequently shown to be problematic. This is all the more so in teaching, and when students pursue datasets to illustrate a new statistical method. Consider, for example, the well-known “twin age-at-appendectomy” dataset. Obtained from a questionnaire survey, Duffy, Martin, and Mathews (Citation1990), this is another twin dataset, particularly attractive for illustrating bivariate “survival” models. As joyful as statisticians are when they find such a rare dataset, they should first check with their own experience, and wonder what was it about the data-collection that led to certain “outlying” results. No matter how elegant the matching, a finding that 21% of respondents had undergone appendectomy, or a conclusion that 45% of secondary infertility is caused by induced abortions, or for that matter that winning an Oscar adds 4 years to the longevity of performers (see Han et al. Citation2011), should raise questions as to the data or the data-analysis.
Our pursuit of the data from the registry of HIV-exposed twins began when, in 2011, JH told students in his class about a twin pair where one was HIV+ and the other HIV−, and asked what factors might be responsible for the discordance. One student was so skeptical, even after seeing the 19:4 discordance ratio in the Lancet article, that JH emailed Dr. Goedert and asked about getting the raw data. He immediately responded:
Dear Jim. You bring back very fond memories—the origin of the idea (at a very small pediatric AIDS meeting in California overlooking the Pacific), assembling the collaborators and contributors, monitoring the raw data as they arrived in my Fax machine (very modern then), my amazement at the difference in risk by birth order, going back to contributors to validate birth order (a few changed actually strengthening the difference), and discussions with the Statistician on the analysis. It will take some digging by a programmer or two who are still around, but the odds are good of finding a clean data set. I will let you know.
The digging was successful and JH received the data within a week.
Datasets that underlie substantial public health changes can be an important way to help students not limit themselves to the statistical analyses: the importance of the question, the biological underpinnings, the cleverness or elegance of the design, the quality of the data, and the impact on society, are even more important.
Supplemental Material
Supplementary materials include the Appendix with R Code to reproduce Table 1, Table 2, and the analysis results. Supplemental data for this article can be accessed on the publisher's website.
UJSE_1381055_Supplementary_Files.zip
Download Zip (156.2 KB)Acknowledgments
We thank Dr. James Goedert, Dr. Anne-Marie Duliège, Myhanh Dotrang, and the skeptical student in JH's course BIOS601 in 2011.
References
- Aragon, T. J., Enanoria, W. T., Fay, M., Porco, T., and Samuel, M. (2010), “Applied Epidemiology using R,” UC Berkeley School of Public Health, California: MedEpi Publishing.
- Atrash, H. K., Strauss, L. T., Kendrick, J. S., Skjeldestad, F. E., and Ahn, Y. W. (1997), “The Relation Between Induced Abortion and Ectopic Pregnancy,” Obstetrics & Gynecology, 89(4), 512–518.
- Biggar, R. J., Goedert, J. J., Miotti, P. G., Taha, T. E., Mtimavalye, L., Justesen, A., and Miley, W. (1996), “Perinatal Intervention Trial in Africa: Effect of a Birth Canal Cleansing Intervention to Prevent HIV Transmission,” The Lancet, 347(9016), 1647–1650.
- Bulterys, M., Chao, A., Dushimimana, A., Kageruka, M., Nawrocki, P., Saah, A., and Goedert, J. (1992), “HIV-Exposed Twins,” The Lancet, 339(8793), 628.
- Centers for Disease Control (CDC) (1987), “Classification System for Human Immunodeficiency Virus (HIV) Infection in Children under 13 Years of Age,” Morbidity and Mortality Weekly Report, 36(15), 225–230.
- Cozen, W., Yu, G., Gail, M. H., Ridaura, V. K., Nathwani, B. N., Hwang, A. E., and Goedert, J. J. (2013), “Fecal Microbiota Diversity in Survivors of Adolescent/Young Adult Hodgkin Lymphoma: A Study of Twins,” British Journal of Cancer, 108(5), 1163–1167.
- Duffy, D. L., Martin, N. G., and Mathews, J. D. (1990), “Appendectomy in Australian Twins,” [Letter] American Journal of Human Genetics, 47(3), 590–592. (original citation). Data can be found at https://genepi.qimr.edu.au/staff/davidD/Appendix/
- Duliège, A. M., Amos, C. I., Felton, S., Biggar, R. J., and Goedert, J. J. (1995), “Birth Order, Delivery Route, and Concordance in the Transmission of Human Immunodeficiency Virus Type 1 from Mothers to Twins,” The Journal of Pediatrics, 126(4), 625–632.
- Fay, M. P. (2015), Exact McNemar's Test and Matching Confidence Intervals. Available at https://cran.r-project.org/web/packages/exact2x2/vignettes/exactMcNemar.pdf
- Frange, P., and Blanche, S. (2014), “VIH et Transmission Mère–Enfant,” La Presse Médicale, 43(6), 691–697.
- Gardiner, J. C., Luo, Z., and Roman, L. A. (2009), “Fixed Effects, Random Effects and GEE: What are the Differences?,” Statistics in Medicine, 28(2), 221–239.
- Goedert, J. J., Biggar, R. J., Amos, C. I., Duliège, A. M., and Felton, S. (1991), “High Risk of HIV-1 Infection,” The Lancet, 338(8781), 1471–1475.
- Goedert, J. J., Duliège, A. M., and Amos, C. I. (1991), “The International Registry of HIV Exposed Twins: A First Report,” Poster presented at The VIIth International Conference on AIDS.
- Goedert, J. J., Duliège, A. M., Felton, S., and Biggar, R. J. (1993), “Update On The International Registry Of HIV-Exposed Twins,” Journal of Acquired Immune Deficiency Syndromes, 6(6), 747.
- Günther, F., and Fritsch, S. (2010), “Neuralnet: Training of Neural Networks,” The R Journal, 2(1), 30–38.
- Halekoh, U., Højsgaard, S., and Yan, J. (2006), “The R Package Geepack for Generalized Estimating Equations,” Journal of Statistical Software, 15(2), 1–11.
- Han, X., Small, D. S., Foster, D. P., and Patel, V. (2011), “The Effect of Winning an Oscar Award on Survival: Correcting for Healthy Performer Survivor Bias with a Rank Preserving Structural Accelerated Failure Time Model,” The Annals of Applied Statistics, 5(2A), 746–772.
- Hanley, J. A., Negassa, A., and Forrester, J. E. (2003), “Statistical Analysis of Correlated Data Using Generalized Estimating Equations: An Orientation,” American Journal of Epidemiology, 157(4), 364–375.
- Harden, V. A., and Rodrigues, D. (1993), “Interview with Dr. James J. Goedert,” In Their Own Words, Available at https://history.nih.gov/nihinownwords/docs/page_12_04.html.
- International Perinatal HIV Group (1999), “The Mode of Delivery and the Risk of Vertical Transmission of Human Immunodeficiency Virus type 1–a Meta-Analysis of 15 Prospective Cohort Studies,” New England Journal of Medicine, 340(13), 977–987.
- Jewell, N. P. (2003), Statistics for Epidemiology, Boca Raton, FL: CRC Press.
- Katz, A. (2003), “The Evolving Art of Caring for Pregnant Women with HIV Infection,” Journal of Obstetric, Gynecologic, and Neonatal Nursing, 32(1), 102–108.
- Kerns, G. J. (2011), Introduction to Probability and Statistics Using R, Free Software Foundation.
- Lion-Cachet, C. (2016), “Serodiscordant Twins: A Case Report of Vertical HIV Transmission during PMTCT Option B+ Role Out,” Pediatric Dimensions, 1(6), 1–7, DOI: 10.15761/PD.1000135.
- McGowan, J. P., and Shah, S. S. (2000), “Prevention of Perinatal HIV Transmission during Pregnancy,” Journal of Antimicrobial Chemotherapy, 46(5), 657–668.
- Makunyane, L. L., Moodley, J., and Titus, M. J. (2016), “HIV Transmission in Twin Pregnancy: Maternal and Perinatal Outcomes,” Southern African Journal of Infectious Diseases, 32(2), 1–3.
- McNemar, Q. (1947), “Note on the Sampling Error of the Difference between Correlated Proportions or Percentages,” Psychometrika, 12(2), 153–157.
- Melbye, M., Wohlfahrt, J., Olsen, J. H., Frisch, M., Westergaard, T., Helweg-Larsen, K., and Andersen, P. K. (1997), “Induced Abortion and the Risk of Breast Cancer,” The New England Journal of Medicine, 336, 81–85.
- Milligan, C., and Overbaugh, J. (2014), “The Role of Cell-Associated Virus in Mother-to-Child HIV Transmission,” Journal of Infectious Diseases, 210(suppl 3), S631–S640.
- Neff, M. J. (2008), “Institutional Review Board Consideration of Chart Reviews, Case Reports, and Observational Studies,” Respiratory Care, 53(10), 1350–1353.
- Panayotou, P. P., Kaskarelis, D. B., Miettinen, O. S., Trichopoulos, D. B., and Kalandidi, A. K. (1972), “Induced Abortion and Ectopic Pregnancy,” The American Journal of Obstetrics and Gynecology, 114(4), 507–510.
- Qaquish, B. F., and Liang, K.-Y. (1992), “Marginal Models for Correlated Binary Responses with Multiple Classes and Multiple Levels of Nesting,” Biometrics, 48, 939–950.
- Rookus, M. A., and van Leeuwen, F. E. (1996), “Induced Abortion and Risk for Breast Cancer: Reporting (Recall) Bias in a Dutch Case-Control Study,” Journal of the National Cancer Institute, 88(23), 1759–1764.
- Schmidt, C. O., and Kohlmann, T. (2008), “When to Use the Odds Ratio or the Relative Risk?” International Journal of Public Health, 53(3), 165–167.
- Sjölander, A., Johansson, A. L., Lundholm, C., Altman, D., Almqvist, C., and Pawitan, Y. (2012), “Analysis of 1:1 Matched Cohort Studies and Twin Studies, with Binary Exposures and Binary Outcomes,” Statistical Science, 27(3), 395–411.
- Trichopoulos, D., Handanos, N., Danezis, J., Kalandidi, A., and Kalapothaki, V. (1976), “Induced Abortion and Secondary Infertility,” British Journal of Obstetrics and Gynaecology, 83(8), 645–650.
- Tzonou, A., Hsieh, C. C., Trichopoulos, D., Aravandinos, D., Kalandidi, A., Margaris, D., … and Toupadaki, N. (1993), “Induced Abortions, Miscarriages, and Tobacco Smoking as Risk Factors for Secondary Infertility,” Journal of Epidemiology & Community Health, 47(1), 36–39.
- Warren, L. (1992), “Study of H.I.V. Transmission at Birth,” New York Times, available at http://www.nytimes.com/1992/01/07/science/study-of-hiv-transmission-at-birth.html