Abstract
Stage-sequential (or multiphase) growth mixture models are useful for delineating potentially different growth processes across multiple phases over time and for determining whether latent subgroups exist within a population. These models are increasingly important as social behavioral scientists are interested in better understanding change processes across distinctively different phases, such as before and after an intervention. One of the less understood issues related to the use of growth mixture models is how to decide on the optimal number of latent classes. The performance of several traditionally used information criteria for determining the number of classes is examined through a Monte Carlo simulation study in single- and multiphase growth mixture models. For thorough examination, the simulation was carried out in 2 perspectives: the models and the factors. The simulation in terms of the models was carried out to see the overall performance of the information criteria within and across the models, whereas the simulation in terms of the factors was carried out to see the effect of each simulation factor on the performance of the information criteria holding the other factors constant. The findings not only support that sample size adjusted Bayesian Information Criterion would be a good choice under more realistic conditions, such as low class separation, smaller sample size, or missing data, but also increase understanding of the performance of information criteria in single- and multiphase growth mixture models.
FUNDING
This study was supported, in part, by grants from the National Institute on Alcohol Abuse and Alcoholism (R01 AA019511 and R01 AA019511-02S1).
Notes
1EMA is a sampling method to assess subjects’ current behaviors and experiences in real time to avoid retrospective recall. In recent years, EMA data collection has been very prosperous due to advances in collecting methods using electrical devices, such as Palm Pilots or cellular phones.
2There are two important, unresolved issues in utilizing those stage-sequential growth mixture models: One is determining the required sample size for accurate estimation of parameters and the other is deciding on the optimal number of latent classes. For the former, Kim (Citation2012) carried out a thorough Monte Carlo study to get tangible sample size requirements for accurate estimation across various simulation conditions, and especially found the close relationship between the sample size required and the number of time points collected.
3To use log likelihood difference test, the degrees of freedom for the difference test should equal the difference in the number of parameters of the two models; that is, the regularity conditions should be met. Thus, the log likelihood difference test is not applicable for nested latent class analysis or mixture models that differ in the number of classes (Everitt, Citation1981; McLachlan & Peel, Citation2000; Nylund et al., Citation2007; Tofighi & Enders, Citation2008).
4A similar overview also appears in Kim (Citation2012), which examined the sample size requirement for accurate estimation of parameters in single- and multiphase GMM.
5In Nagin’s (1999) group-based approach, systematic individual differences from the mean trajectory within classes are not allowed, which is also referred to as latent class growth analysis (LCGA). Nagin’s LCGA models are not considered in this study.
6The three path diagrams in are reproduced from Kim (Citation2012).
FIGURE 2 Three stage-sequential growth mixture models. (a) A traditional piecewise GMM. (b) A discontinuous piecewise GMM. (c) A sequential process GMM. y1 to y4 are the first phase measures and y5 to y8 are the second phase measures; int = intercept; slp = slope; c = latent class variable; X = covariates; and U = distal/proximal outcome variables.
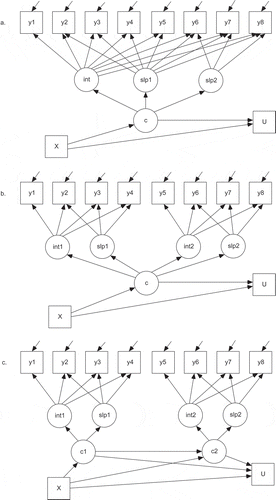
7Kim (Citation2012) investigated the issue of the sample size requirement for accurate estimation of parameters in single- and multiphase growth mixture models, and this article tried to examine the issue of determining the latent classes in the same models. Therefore, factor specifications for the two Monte Carlo studies were quite similar, although the purposes, the procedures, and the results were very different.
8Through a review of several single- and multiphase growth mixture models, the number of extracted latent classes was between two and seven (Duncan, Duncan, Strycker, Okut, & Li, Citation2002; Hix-Small, Duncan, Duncan, & Okut, Citation2004; Kim & Kim, Citation2012; Li et al., Citation2001; Muthén, Citation2001b, Citation2001c; Muthén & Muthén, Citation2000), and four was the average.
9Covariates are important in correctly specifying the model, in finding the proper number of classes, and in correctly estimating class proportions and class membership (Muthén, Citation2004). The performance of all ICs was worse with covariates than without covariates in Tofighi and Enders (Citation2008).
10High class separation with even class probabilities was defined as a simple structure, which is similar to a factor analysis model in which there are unique items that identify each of the factors (i.e., no or low cross-loadings). On the other hand, low class separation with possibly uneven class probabilities was defined as a complex structure (Kim, Citation2012; Nylund et al., Citation2007).
11Estimation time with missing data were about 100 times longer than without missing data especially when the number of indicators were seven and seven in sequential process GMM. The actual time was more than 10 days per model with a modern personal computer, and a single condition required five models to be estimated with sequential process GMM.
12The effects of all the factors were examined at the initial stage. To save space, and to be more realistic, only the results of the three controllable factors are provided in this study.
13For reference, the default value of the random start option in Mplus is 10 random sets of initial starting values and two final stage optimizations.