Abstract
Popular longitudinal models allow for prediction of growth trajectories in alternative ways. In latent class growth models (LCGMs), person-level covariates predict membership in discrete latent classes that each holistically define an entire trajectory of change (e.g., a high-stable class vs. late-onset class vs. moderate-desisting class). In random coefficient growth models (RCGMs, also known as latent curve models), however, person-level covariates separately predict continuously distributed latent growth factors (e.g., an intercept vs. slope factor). This article first explains how complex and nonlinear interactions between predictors and time are recovered in different ways via LCGM versus RCGM specifications. Then a simulation comparison illustrates that, aside from some modest efficiency differences, such predictor relationships can be recovered approximately equally well by either model—regardless of which model generated the data. Our results also provide an empirical rationale for integrating findings about prediction of individual change across LCGMs and RCGMs in practice.
ACKNOWLEDGMENTS
We are grateful for the feedback of Dr. Patrick Curran,Dr. Robert MacCallum, Dr. Antonio Morgan-Lopez, and Dr. Andrea Hussong on an earlier version of this article.
Notes
1Adding time-varying predictors is also possible but is not the focus of this article.
2In this notation, all Level 1 time scores have random effects; setting elements of to 0 would allow some time scores to only have fixed effects.
3A vector consisting of all K class indicator labels conforms to a multinomial distribution consisting of 1 draw on K categories with probabilities
(McLachlan & Peel, Citation2000).
4Non-classification models that only include linear relationships and do not allow individual differences in change over time have been called variable-oriented methods; we do not use that term for RCGMs because they are not subject to those limitations (see Sterba & Bauer, Citation2010a).
5An alternative strategy to using the per-sample best fitting number of classes would be to use the across-samples average best fitting number of classes in these calculations. Both strategies yielded the same overall pattern of findings regarding hypotheses.
6Because LCGM requires prediction of the entire trajectory as a whole, in this situation LCGM classes could tend to be extracted that have both high, or both low, intercepts and slopes. Then an increment in the predictor could be associated with an increased probability of membership in the high-intercept, high-slope class. Yet, in the population the predictor influences intercepts positively but slopes negatively.
7Another example of factor-specific predictor relationships that are not in concert with growth factor correlations is as follows. A predictor has all-positive or all-negative effects on growth factors, but growth factors are negatively correlated.
8A similar pattern of bias results occurs if each of the other predictors is considered as the moderator (not shown).
FIGURE 6 Study 2 generalizability checks: Predicted trajectories for the LCGM generating condition with implied higher order nonlinear interactions and highly nonmonotonic growth trajectories. Note. LCGM = latent class growth model; RCGM = random coefficient growth model.
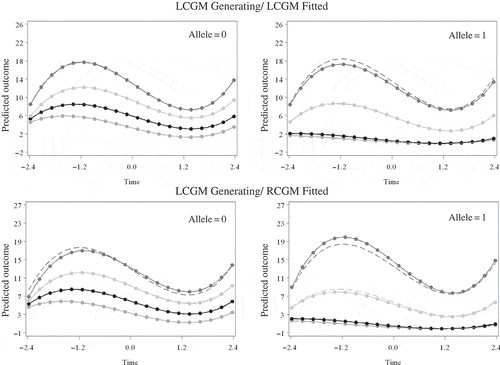
FIGURE 7 Study 2 generalizability checks: Predicted trajectories for the RCGM generating condition where predictors have factor-specific effects not in concert with factor correlations. Note. RCGM = random coefficient growth model; LCGM = latent class growth model. Dashed = true; solid = average model-implied.
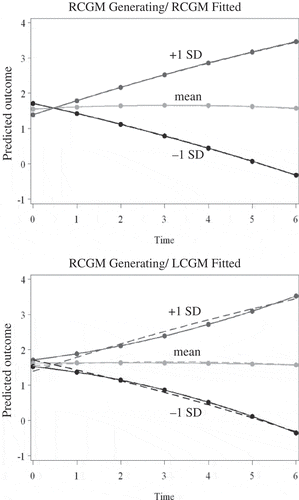