Abstract
To find new biomarkers and establish histopathology protein fingerprint models for early detection of oral squamous cell carcinoma (OSCC), laser capture microdissection (LCM) technology was utilized in 21 OSCC tissues and 7 oral leukoplaque (OLK) tissues as well as their adjacent normal tissues. Each sample was then detected by SELDI-TOF-MS technology and CM10 protein chip as well as bioinformatics tools. Three proteomic biomarker patterns were identified. Pattern 1 distinguishes patients with OLK from healthy individuals. Pattern 2 distinguishes patients with OSCC from healthy individuals. Pattern 3 distinguishes patients with OSCC from patients with OLK. The analysis yielded both a specificity and a sensitivity of 90.48% for pattern 1, a specificity of 100.00% and a sensitivity of 85.71% for pattern 2, and a specificity of 100.00% and a sensitivity of 85.71% for pattern 3. Proteome mass/charge 3714, 3515, and 4944 built the distinguished protein peaks between the OSCC tumor and adjacent normal tissues. The accuracy of the blind prediction was 90.48% (38/42). M/Z 15122 and 7569 built the distinguished protein peaks between the OLK and adjacent normal tissues. M/Z 3738 and 11366 built the distinguished protein peaks between the OSCC and the OLK. By employing LCM technology combined with SELDI-TOF-MS technology and bioinformatics approaches, histopathology would not only facilitate the discovery of better biomarkers for OSCC and OLK, but also provide a useful tool for molecular diagnosis by potential biomarker.
Oral squamous cell carcinoma (OSCC) is a disease usually marching to recurrence and metastasis, and its 5-year survival rate is only 50%, so early detection of OSCC is critical to avoid deformity and malfunction of swallowing, speech, head and neck activity, which may result from the radical treatment for a later diagnosis, while the unique local resection for early stage of OSCC will survive 90% of these patients Citation[1–3].
Rather than the genome, it is the proteome that controls and participates directly in the physiologic and pathologic life procedures Citation[4]. In proteomics, the technology of micro-array and bio-informatics carved out a new way to effectively seek tumor markers. Surface-enhanced laser desorption/ionization-time of flight-mass spectrometry (SELDI-TOF-MS) is such a new path to provide high-throughout protein profiling Citation[5]. In this study, the differential proteomics patterns in tissues among OSCC, OLK tissues, and adjacent normal tissues, respectively, was detected by SELDI-TOF-MS technology following application of laser-capture microdissection (LCM) Citation[6–9] to iced and modified Mayer's hematoxylin stained tissue sections. The proteomics biomarker patterns of OSCC patients were obtained to build the OSCC diagnosis models.
METHODS
Tissue Samples and Protein Extraction
Histologically squamous mucosa specimens were collected from primary resected 21 OSCC and 7 OLK patients, who provided written informed consent for planned studies and were approved by Institutional Review Board, and were immediately frozen. Six-micrometer cyrosections were cut onto special PEN membrane slides for LCM use, except for the first and last sections, which were cut onto conventional glass slides. Sections were immediately fixed with 100% ethanol. Conventional glass slides tissue sections were stained with hematoxylin and eosin to be confirmed as being either dysplasia OLK, malignant invasive OSCC, or normal epithelium (nonmalignant). Special PEN membrane slides tissue sections were stained with modified Mayer's hematoxylin for 5 minutes and subsequently rinsed 2 times with 1 ml PBS (pH 7.4). Section specimens were stored in liquid nitrogen and used for downstream applications. First, they were dehydrated with 70-95-100% ethanol, and then thoroughly air dried. For LCM, stained uncovered slides were viewed and after locating the cells of interest, a CapSure LCM Cap (Arcturus, Mountain View, CA, USA) was placed over the target area and pulsed with laser to adhere cells () to the cap, which after sufficient capture (5000 cells) was transferred to a 0.5mL sterile microfuge tube for immediate processing. For each sample, approximately 10,000–15,000 cells were captured on multiple caps, which were subsequently processed for protein extraction. The procedure of protein extraction from CapSure caps was essentially as described Citation[6], Citation[10]. Briefly, 3 times U7SU2 Lysis volume of NaAC protein extraction buffer was placed in each tube and with the enclosed caps for 20 minutes in 4°C. The extraction solution was then recovered by centrifugation (10,000rpm) for 5min, pooled and stored at −80°C until ready to be processed for analysis.
Figure 1. Laser-capture microdissection of OSCC; tumor cryosections were fixed, stained with hematoxylin and eosin (H&E), dehydrated and analyzed under the microscope. Once tumor cells of interest were identified, microdissection was performed, and the cell purity confirmed by inspecting the area of tissue that underwent procurement and the captured cells.
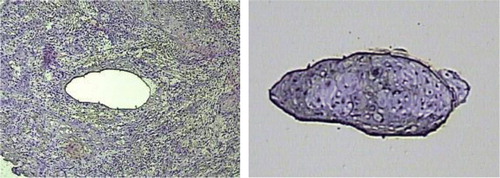
The used reagents included sequence grade trypsin (Promega, Madison, WI), dithiothreitol (DDT), iodacetamide, ammonium bicarbonate (NH4HCO3), and formic acid (ICN Biomedical Inc., Aurora, OH). HPLC grade solvents (methanol, chloroform and acetonitrile) were purchased from Fisher Scientific (Hanover Park, IL). Briefly, the frozen protein extracts were thawed to room temperature, followed by denaturation (25mM DDT) and alkylation (300mM iodoacetamide). The salts and detergent were then removed from the samples by methanol/chloroform precipitation. After removal of the supernatant, the protein precipitates were rinsed once in methanol, air dried, and dissolved in 15lL of 100mM NH4HCO3 at pH 8.0, with agitation for up to 45min. Dissolved proteins were digested with trypsin (1:20 w/w) at 26°C initially for 12h, and then fresh trypsin was added at the same ratio and the digestion continued for an additional 12h. The final volume of each sample was 17uL.
Analysis of ProteinChip Array
After thawing and 2 min of centrifugation (10 000 r/min), every 10000 cells were added into 3 µl U7SU2 (7 mol/L Urea, 2 mol/L Thiourea, 4% CHAPS (3[(3-cholamidopropyl) dimethylammonio]-1-propan esulfonate), 50mmol/lDTT (DL-dithiothreitol), 0.2% Protease Inhibitor Cocktail) in a 96-well plate and incubated for 30 min at 4 °C with 600 r/min vigorous shaking. The ProteinChip array cassette was put into a 96-well bioprocessor and 200 µl NaAc (50 mmol/L, pH 4.0) was put into each well, and incubated for 5 min at 4 °C with 600 r/min vigorous shaking. The liquid was removed. The procedure was repeated once. Then 185 µl NaAc was added into each well in the 96-well plate (600 r/min, 2 min) and then 100 µl samples disposed above of different patients were added into different wells separate of the ProteinChip array cassette (600 r/min, 1 h) Citation[11]. After the content from each well was removed, each well was washed with 200 µl NaAc (pH = 4.0, 600 r/min, 5 min). The procedure was repeated two more times. Each spot was washed with 200 µl HPLC water, which was removed immediately. The procedure was repeated once. After air drying, 1 µl SPA (sinapic acid) was applied to each spot. After air drying for 5 min, another 1 µl SPA was applied. Ciphergen SELDI Protein Biology System II plus (PBS II plus) and ProteinChip Software (Version 3.2, Ciphergen Biosystems) were used to read the chips and analyze the data. The following settings were used: laser intensity 165, 65 laser shots per sample, detector sensitivity 7, automatically detected peaks from 2,000 to 30,000 m/z. Mass accuracy was calibrated to less than 0.1% using the All-in-1 peptide molecular mass standard (Ciphergen Biosystems). The peaks were normalized and noises were filtrated (first signal to noise ratio > 2.5). Peak clusters were completed using second-pass peak selection (signal to noise ratio > 2, within 0.3% mass window) and estimated peaks were added. The biomarker wizard of ProteinChip Software 3.2 was used to compare the data of different group, and by Mann-Whitney U test discrepant mass peaks were found.
Statistical Analysis
To establish histopathology diagnostic models for OSCC, we attempted to identify three differential patterns of OSCC biomarkers as follows: pattern 1, a differential pattern of biomarkers for diagnosis of OSCC was identified by comparing OSCC tissue and adjacent healthy controls; pattern 2, another differential pattern of biomarkers for diagnosis of OLK, was identified by comparing OLK with adjacent healthy controls; pattern 3, a differential pattern of biomarkers for OSCC, was identified by comparing OSCC with OLK. The data analysis was implemented by the Zhejiang University – Cancer Institute-ProteinChip Data Analysis System (ZUCI-PDAS), which was designed by Jiekai Yu on the MATLAB Web Server 1.2.4 (The MathWorks, Natick, MA). All these bioinformatics studies were integrated in the ZUCI-PDAS available at www.zlzx.net.
The first step of our data analysis was to use the undecimated discrete wavelet transform (UDWT) method to denoise the signals. The UDWT method is implemented by the Rice Wavelet Toolbox version 2.4. Second, the spectra were subjected to baseline correction by aligning with a monotone local minimum curve and mass calibration (adjusting the intensity scale according to three labeled peaks that appears in all the selected spectra). The proteomic peaks were detected and quantified by an algorithm that takes the maximal height of every denoised, baseline-corrected, and calibrated mass spectrum into account. Third, the peaks were filtered to maintain an S/N of more than three. The S/N of a peak is the ratio of the height of the peak above the baseline to the wavelet-defined noise. Finally, to match peaks across spectra, we pooled the detected peaks if the relative difference in their mass sizes was not more than 0.3%. The minimal percentage of each peak, appearing in all the spectra, is specified to ten. The matched peak across spectra is defined as a peak cluster. If a spectrum does not have a peak within a given cluster, the maximal height within the cluster will be assigned to its peak value. The normalization was performed only with the identified peak clusters.
Further, to distinguish between the different groups of data, we applied a nonlinear support vector machine (SVM) classifier, originally developed by Vladimir Vapnik, with a radial based function kernel, a parameter Gamma of 0.6, and a cost of the constrain violation of 19. The leave-one-out cross-validation approach was applied to estimate the accuracy of this classifier. This approach takes out one sample each time as the test set and keeps the remaining samples as the training set. This process is repeated until each sample has been taken once as a test sample. This SVM classifier was implemented by the shareware program OSU_SVM v.3.00 Toolbox of Junshui Ma and Yi Zhao. The capability of each peak in distinguishing different groups of data was estimated by the p value of Wilcoxson T-test. The top ten peaks with the smallest p value were selected for further analysis. Each of the 1023 combinations of the 10 peaks was analyzed by the leave-one-out crossvalidation SVM Citation[11]. Combinations with the highest accuracy in distinguishing different groups of data were selected as potential biomarkers. The SVM model with the highest Youden's index was selected as the model for detecting OSCC.
RESULTS
Differential Proteomics Pattern of OSCC Tissues Versus Adjacent Normal Mucosal Tissues (Pattern 1)
A total of 187 qualified and discrepant mass peaks were detected in the tissue comparison of OSCC with normal. Through leave-one-out cross-validation, discriminant analysis screening out 3 protein mass peaks (m/z: 3714, 3515, and 4944) to build a tissue diagnosis model, which could develop and determine the tissue peptide patterns for distinguishing OSCC tissues from healthy ones. In this group, the three protein mass peaks were expressed significantly high in OSCC cells (). The contemporaneous changes of these 3 protein mass peaks remarkably instructed an exact tissue diagnosis model. The accuracy of the blind prediction was 90.48% (38/42). The sensitivity and specificity were 90.48% (19/21), respectively ( and ).
Figure 2. Protein mass peak 3714 expressed significantly high in the OSCC tissue, while the healthy tissue showed a remarkably low protein mass peak at 3714 (P=0.0000922061).
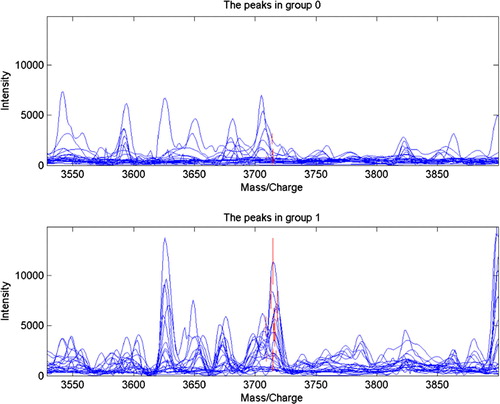
Table 1. The cross-validation results of diagnosis model of OSCC and healthy tissues (cases)
Table 2. The three discrepant tissue protein mass peaks (m/z) in comparison of OSCC with healthy tissues (x±SD)
Differential Proteomics Pattern of OLK Tissues Versus Adjacent Normal Mucosal Tissues (Pattern 2)
A total of 183 qualified peaks were selected after noise filtering and peak cluster identification. The top ten peaks with the smallest p value were selected, randomly combined, and fed into SVM. The accuracy of each combination in distinguishing OLK from normal tissue was analyzed; combinations with the highest accuracy were chosen as potential biomarkers, and the SVM model with the highest Youden's index was used as the diagnostic model. This model, designated pattern 2, comprised 2 potential biomarkers with m/z of 15122 and 7569 Da, respectively. The 2 peaks were highly expressed in OLK but weakly expressed in normal tissues. The descriptive statistics of these 2 peaks are shown in . Pattern 2 has a specificity of 100.00% and a sensitivity of 85.71%, as evaluated by leave-one-out cross validation.
Table 3. The statistics of two candidate biomarkers for screening OLK from normal tissue
Differential Proteomics Pattern of OLK Patients Versus OSCC Patients (Pattern 3)
Pattern 3 was established similarly to distinguish OSCC from OLK. Pattern 3 comprised two potential biomarkers out of 218 peaks with m/z of 3738, 11366 Da. While the peaks with m/z 3738 Da were expressed higher in OSCC than in OLK, the 11366 appeared to be expressed in an opposite way. The descriptive statistics of these two peaks are shown in . This model had a specificity of 100.00% and a sensitivity of 85.71%, as evaluated by leave-one-out cross validation.
Table 4. The statistics of two candidate biomarkers for screening OLK from OSCC.
DISCUSSION
Three integers of tissue proteomic diagnosis models of OSCC were collected and listed in as follows. Researching from pattern 2 and 3, it was indicated that the histologic protein mass/charge wave peaks 15122, 7569, 11366 expressed highly in OLK tissue, while 3714, 3738, 3515, 4944 expressed highly in the OSCC tumor tissue; all these seven protein peaks expressed weakly in normal oral mucous membrane ephithelia. These three histology proteomic diagnosis models of OSCC and OLK are integers, respectively. It seemed that the protein mass/charge peaks in leukoplaque tissue were high over 7000, while they were comparatively lower in normal oral mucous membrane and malignant squamous cell tumor. This technique is promising while it remains to be a following task to enlarge the sample.
Table 5. Three patterns of proteomic diagnosis model of OSCC
We applied SELDI-TOF-MS, which is able to obtain and compare spectra from a significant number of samples in a relatively short time with very little sample preparation or sophisticated chromatography. The analysis of a large number of samples will ideally reveal biomarkers, i.e., protein signals that are unique to, or differently expressed in, one sample set when compared with a different sample set. Current obtained results show that SELDI-TOF-MS provides a simple and high throughput technique to rapidly identify a large number of differently expressed peptides/proteins in histological samples, especially low mass proteins (<10 kDa) that have been difficult to assay effectively with other methods. It is further anticipated Citation[12] that this proteomic technique will be a valuable tool in projects ranging from identification of diagnostic biomarkers for a variety of diseases or description of whole body physiological changes as well as to the study of modification and biomolecular interactions of local tissue peptides and proteins.
We adopted LCM to isolate 10,000–15,000 epithelial cells from clinical tissue samples, combined with SELDI-TOF-MS, to explore the feasibility of establishing a pattern of OLK and expressed cancer-related proteins for OSCC. Our findings indicate that these approaches generate large proteomic datasets from minimal clinical samples that is likely to lead to the identification of novel OSCC and OLK protein biomarkers. Indeed, some of the emerging protein information has already provided evidence of the expression of molecules that might be involved in tumor or OLK as well as clinically useful markers defining the margins of the neoplastic lesions Citation[6].
To gain success, this approach is indeed likely to depend on the use of highly sensitive protein detection systems, together with the accuracy of LCM for the isolation of specific cell types, and the quality and preservation of the tissue samples. To this end, LCM generally ensures approximately 95% purity of the correct cell population from tissues with optimum tissue integrity intact. Thus, before proceeding with the analysis, we have first confirmed the quality and the histopathology of all the samples by microscopic visualization of frozen tissue sections (6um thickness), modified Mayer's hematoxylin stained. Oral squamous epithelium lacking hyperplastic and/or dysplastic cellular features were localized carefully for microdissection. Similarly, all tumor biopsies were assessed to be squamous cell carcinomas with variable histological grades, which ranged from poorly to well-differentiated lesions for progressive research. After this initial assessment, all the samples were considered suitable for LCM and proteome analysis.
CONCLUSION
The combination of ProteinChip array with Time-of-Flight Mass Spectrometry offers the advantages of speed, simplicity, sensitivity, and suitability for a comparative study. The results of this initial study with samples from OSCC patients are promising and encouraging. In this study, the histological diagnosis models of OSCC from these data at a molecular level were found to be significantly sensitive, indicating the potential use of protein fingerprints and LCM for the isolation of specific cell types and the quality as novel approaches for early diagnosis of OSCC. The important point of this study is to demonstrate the feasibility of modern cancer histological diagnostics and establish the basis for incessant biomarker research Citation[12–14]. The progressive study of such prognostic markers based on tumor phenotype and biologic behavior would allow clinicians not only to diagnose a disease involving OSCC but also to select the most efficacious treatment modalities.
It is anticipated that the technological advancements in mammography would yield more reliable detection of small lesions. Further studies with larger samples and protein identification are needed to confirm “molecular fingerprints” for a real clinical benefit to patients with OSCC.
Acknowledgements
This work was supported by the Research and Development Program of Science &Technology in Zhejiang of China (2007C30030, 2008C33026). Hong He and Gang Sun are Co first authors, they contributed equally to this work. Declaration of interest: The authors report no conflicts of interest. The authors alone are responsible for the content and writing of the paper.
References
- Becker M.T., Shores C.G., Yu K.K., Yarbrough W.G. Molecular assay to detect metastatic head and neck squamous cell carcinoma. Arch Otolaryngol. Head Neck Surg 2004; 130: 21–27
- Ferlito A., Shaha A.R., Rinaldo A. The incidence of lymph node micrometastases in patients with pathologically staged N0 in cancer of oral cavity and oropharynx. Oral Oncol 2002; 38: 3–5
- Nemeth Z., Velich N., Bogdan S., et al. The prognostic role of clinical, morphological and molecular markers in oral squamous cell tumors. Neoplasma 2005; 52(2)95–102
- Resing K.A., Ahn N.G. Proteomics strategies for protein identification. FEBS Lett 2005; 579(4)885–889
- Merchant M., Weinberger S.R. Recent advancements in surface-enhanced laser desorption/ionization-time of flight-mass spectrometry. Electrophoresis 2000; 21(6)1164–1177
- Baker H., Patel V., Molinolo, Alfredo A., et al. Proteome-wide analysis of head and neck squamous cell carcinomas using laser-capture microdissection and tandem mass spectrometry. Oral Oncology 2005; 41: 183–199
- Frend F., Emmert-Buck M.R., Chuqui R., et al. Immuno-LCM: Laser capture microdissection of immunostained frozen sections for mRNA analysis. Am J Pathol. 1999; 154(1)61–66
- Lee J.Y., Dong S.M., Kim S.Y., et al. A simple, precise and economical microdissection technique for analysis of genomic DNA from archival tissue sections. Virchows Arch 1998; 433(4)305–309
- Sirivatanauksorn Y., Drury R., Crnogorac-Jurcevic T., et al. Laser-assisted microdissection: applications in molecular pathology. J Patho 1999; 189(2)150–154
- Wu S.L., Hancock W.S., Goodrich G.G., Kunitake S.T. An approach to the proteomic analysis of a breast cancer cell line (SKBR-3). Proteomics 2003; 3(6)1037–1046
- Schipper R., Loof A., de Groot J. SELDI-TOF-MS of saliva: Methodology and pre-treatment effects. J Chromatography B 2007; 847(1)45–53
- Xu, Wen-hong, Chen, Yi-ding, Hu, Yue, Yu, Jie-kai, , et al. 2006. Preoperatively molecular staging with CM10 ProteinChip and SELDI-TOF-MS for colorectal cancer patients. J Zhejiang Univ. Science B, 7(3): 235–240.
- Issaq H.J., Veenstra T.D., Conrads T.P., Felschow D. The SELDI-TOF MS approach to proteomics: protein profiling and biomarker identification. Biochem. Biophys. Res. Commun 2002; 292(3)587–592
- Weinberger S.R., Morris T.S., Pawlak M. Recent trends in protein biochip technology. Pharmacogenomics 2000; 1(4)395–416