Abstract
The recent COVID-19 outbreak has highlighted the importance of effective communication strategies to control the spread of the virus and debunk misinformation. By using accurate narratives, both online and offline, we can motivate communities to follow preventive measures and shape attitudes toward them. However, the abundance of misinformation stories can lead to vaccine hesitancy, obstructing the timely implementation of preventive measures, such as vaccination. Therefore, it is crucial to create appropriate and community-centered solutions based on regional data analysis to address mis/disinformation narratives and implement effective countermeasures specific to the particular geographic area.
In this case study, we have attempted to create a research pipeline to analyze local narratives on social media, particularly Twitter, to identify misinformation spread locally, using the state of Pennsylvania as an example. Our proposed methodology pipeline identifies main communication trends and misinformation stories for the major cities and counties in southwestern PA, aiming to assist local health officials and public health specialists in instantly addressing pandemic communication issues, including misinformation narratives. Additionally, we investigated anti-vax actors’ strategies in promoting harmful narratives. Our pipeline includes data collection, Twitter influencer analysis, Louvain clustering, BEND maneuver analysis, bot identification, and vaccine stance detection. Public health organizations and community-centered entities can implement this data-driven approach to health communication to inform their pandemic strategies.
The recent COVID-19 pandemic has prompted researchers to consider effective communication strategies that could leverage people’s motivation to alter their behavior and control the spread of the virus. Previous research findings demonstrated the importance of community engagement in health and risk communication during this pandemic (Ahinkorah et al., Citation2020; French et al., Citation2020; Riley et al., Citation2021; World Health Organization, Citation2020). Based on a report by the World Health Organization (WHO), it is crucial to evaluate certain specific requirements for each community and establish locally-driven, collaborative initiatives in response to COVID-19. The report highlights the risk of misinformation, confusion, and mistrust undermining efforts to promote the use of vital resources, services, and information without active community engagement (World Health Organization, Citation2020, p. 7).
To empower communities and help people take control of their lives, it is essential to build a strategy that creates opportunities for them to participate in the COVID-19 response and develop locally appropriate, community-centered solutions. To implement this approach, it is important to develop a data-driven framework that can identify the key challenges that need to be addressed. This framework will help improve communication quality and provide adequate information to assist people in their decision-making processes.
According to the WHO report, the strategy for fighting COVID-19 disinformation and vaccine hesitancy should be community-led, data-driven, and collaborative while also reinforcing capacity and local solutions (World Health Organization, Citation2020). This effort requires the participation of state and local government, universities, religious organizations, libraries, media newsrooms, and other community-centered entities. To accelerate the coordinated communication strategy and community response, a research pipeline is suggested to inform the strategy for effective health communication using a data-driven analysis of social media conversations about COVID-19 with a local focus on state discourse. Our case study of southwestern Pennsylvania provides an example of how this data-driven approach was implemented by considering the local conversations on social media, particularly Twitter.
Literature Review
Previous research about health communication and coverage of the pandemic was dedicated to the in-depth analysis of social media usage (Allington et al., Citation2020; Bonnevie et al., Citation2021; Puri et al., Citation2020; Scannell et al., Citation2021; Vos & Buckner, Citation2016), as well as the health risk communication strategies during pandemics (Chou & Budenz, Citation2020; Crouse Quinn, Citation2008; Vaughan & Tinker, Citation2009). Numerous studies about the ongoing COVID-19 outbreak investigated the impact of various factors on people’s intentions to follow pandemic prevention measures. The list of those factors includes exposure to mis/disinformation (Allington et al., Citation2020; Hornik et al., Citation2021), trust in scientific knowledge (Brzezinski et al., Citation2020), partisanship (Allcott et al., Citation2020; Barrios & Hochberg, Citation2020; Cornelson & Miloucheva, Citation2020; Painter & Qiu, Citation2020), as well as the role of social media (Bonnevie et al., Citation2021; Hernandez et al., Citation2021; Scannell et al., Citation2021).
One of the main challenges for health officials during the COVID-19 pandemic was to find an effective communication strategy to encourage people to stay home, wash hands, practice social distancing, wear a mask, and use the available vaccines - practices that were implemented to help to eliminate or minimize the negative consequences of the pandemic (Mummert & Weiss, Citation2013). Constantly growing vaccine hesitancy, mis/disinformation spread about the pandemic and the vaccines, news avoidance, and the usage of alternative media brought even more issues and complications (Allington et al., Citation2020; Bonnevie et al., 2022; Chou & Budenz, Citation2020; Hornik et al., Citation2021; Puri et al., Citation2020).
Dubé et al. (Citation2013) indicate that vaccine hesitancy is a complex phenomenon that is difficult to define. Attitudes toward vaccination are viewed on a continuous scale, ranging from a positive stance and active demand for vaccines to a negative stance and complete refusal to receive them. Vaccine-hesitant individuals fall somewhere in the middle of this continuum. They are often hesitant to receive vaccines that are safe and recommended. At the same time, people with anti-vaccination attitudes express more vaccine skepticism and relate to the strongly negative side of the continuum (Lindeman, Svedholm-Häkkinen, & Riekki, Citation2022). Several factors can influence vaccine hesitancy and decision-making, including past experiences, political beliefs, the information environment, media literacy, trust in government, and public health communication strategies.
Furthermore, vaccination has been the subject of numerous controversies and scares, such as the fraudulent link between COVID-19 vaccines and infertility (Wesselink et al., Citation2022) and others. Media and the Internet, particularly social media, provide a platform for anti-vaccination advocates and conspiracy theorists to disseminate mis/disinformation. Although the terms mis/disinformation are used interchangeably in this paper, there is a difference between the two. Misinformation refers to the spread of false information, regardless of whether there was an intent to deceive (Sherman, Citation2018), while disinformation contains false information intentionally created to mislead and misinform (Fallis, Citation2015). However, identifying intent can be challenging, especially for users on social media platforms.
During the COVID-19 pandemic, misinformation, disinformation, and vaccine hesitancy narratives spread rapidly online among anti-vax social media users. These trends have jeopardized efforts to promote health communication aimed at persuading people to get vaccinated against COVID-19. According to previous research, highly polarized and active anti-vaccine conversations were mainly influenced by political and nonmedical Twitter users, while less than 10% of the tweets stemmed from the medical community (Hernandez et al., Citation2021). One of the most significant contributing factors to vaccine hesitancy is considered to be the vast proliferation of mis/disinformation that led the WHO to declare an “infodemic.” According to Scannell et al. (Citation2021), the nonstop propagation of mis/disinformation has sparked confusion, suspicion, and negative sentiment toward the COVID-19 vaccine. To counter those issues, we need to attract the attention of society and healthcare professionals to the growing number of disinformation stories and ensure the presence of medical fact-checking information that would debunk disinformation narratives. A failure to target COVID-19 social media anti-vax discourse may continue to disrupt the mass-vaccination plans worldwide, including in the US.
As a result, one of the necessary steps in addressing vaccine hesitancy could be debunking the disinformation stories disseminated online. To combat these harmful narratives, it is necessary to develop a systemic approach that enables health practitioners and public health communicators to quickly identify online disinformation and address it promptly at the community level. To reach this goal, we propose a methodological pipeline that utilizes computational methods to gather social media data about COVID-19 within a specific geographical area. This data is then used to identify particular disinformation narratives being spread within the community at the state level. Therefore, we will be able to facilitate the implementation of misinformation debunking approaches and target local communities with more effective health communication strategies. For our case study, we use an example of southwestern Pennsylvania. To address the issues outlined, we have formulated several research questions:
RQ1: What trends in social media discourse are demonstrated on Twitter regarding the propagation of pro-vaccination and anti-vaccination narratives in southwestern Pennsylvania?
RQ2: What trends in social media discourse could be identified on Twitter regarding the propagation of narratives by bots and authentic users?
RQ3: What types of disinformation stories in Pennsylvania can be identified through computational methods?
RQ4: What strategies are used to spread those disinformation stories throughout the area?
Methodology
We have collected Twitter data for the southwestern Pennsylvania COVID-19 Vaccine Project since the beginning of April 2021 to compile a set of tweets that capture conversations about the vaccine in southwestern Pennsylvania. Our initial collection of streamed tweets used keywords such as “Moderna,” “vaccine,” and “Pfizer,” which did not limit the location where the tweets originated. Additionally, we started collecting tweets using a geographic bounding box for tweets with geolocation information to find tweets originating from specific locations in Pennsylvania. Spatial bounding boxes let users select tweets by placing squares on maps or using geolocation coordinates (Landwehr & Carley, Citation2014). Data collection resulted in weekly reports for the local healthcare professionals, including medical groups, religious organizations, and community service groups. The vaccine keyword data has a hierarchical location prediction neural network to extract locations for each tweet. This process results in tweets from the larger southwestern Pennsylvania cities: Philadelphia, Pittsburgh, Erie, Norristown, Chester, Bethlehem, and Allentown. These comprise the bulk of our final processed tweets.
The bounding box set is processed via keywords to limit tweets to those concerning the vaccine, as we know the tweets originate from southwestern Pennsylvania. Most of these tweets are from Pittsburgh and Philadelphia, but we have also collected tweets from 1275 other identifiable locations in Pennsylvania. These other tweets come from outlying suburbs, townships, and boroughs in rural areas and a few very specific user-defined locations (e.g., “Interstate 80 Rest Area: Danville”). Cities and counties have similar percentages of tweets collected since July 2021, with Philadelphia and Pittsburgh (and Allegheny County) representing most of the set (See ).
Table 1. Percent of tweets from various PA cities
Table 2. Percent of tweets from various PA counties
The raw tweets collected, including all locations for the keyword stream and all conversations from the geolocation stream, total approximately 4.3 terabytes of data since April 2021. Once processed to limit the data to Pennsylvania-located tweets concerning vaccines, we have 32 gigabytes of data, or about 6 million tweets from almost 1.4 million users. During our analysis, we applied the principles of social cybersecurity, which aims to analyze, comprehend, and predict changes in human behavior, as well as social, cultural, and political outcomes that result from cyber-mediated activities. The main goal of cybersecurity research is to develop the necessary cyberinfrastructure that would enable society to maintain its essential character in a cyber-mediated information environment, even when faced with changing conditions or social cyber threats (Carley, Citation2020). The main guidelines of this field highlight the significance of analyzing the communication strategies used in social media and exploring effective countermeasures (Beskow & Carley, Citation2019; Carley, Cervone, Agarwal, & Liu, Citation2018).
Our pipeline consists of data collection, data filtering, bot detection with BotHunter (Beskow & Carley, Citation2018), Twitter analysis of influencers such as super spreaders and super friends, as well as analysis of BEND maneuvers (Blane, Bellutta, & Carley, Citation2022), and stance detection analysis (See ). Super spreaders and super friends possess high-ranking centrality scores on communication, meaning their messages are widely spread or they have many friends with substantial influence in the collected dataset. Other influencers are users with an active network presence, tweeting often or mentioning other users. They also operate in central parts of the conversation, such as by using important hashtags or mentioning important users (Alieva, Ng, & Carley, Citation2022; Uyheng & Carley, Citation2019).
The data was collected using twarc and analyzed with ORA and NetMapper software tools (Carley, Citation2014; Carley, Reminga, & Carley, Citation2018). We have used the BotHunter tool to identify bot activities, a tiered supervised machine learning approach for bot detection and characterization. Afterward, we have used NetMapper to compute language cues and ORA to compute reports and scores. ORA produces several metrics for Twitter data, such as the list of super spreaders (users that generate often shared content and hence spread information effectively) and super friends (users that exhibit frequent two-way communication, facilitating large or strong communication networks).
The Louvain method was used to identify network communities participating in the COVID-19 discussion in southwestern PA (Blondel et al., Citation2008). The Louvain algorithm is a widely adopted method for community detection that allows a more granular rendering of the network (Alieva & Carley, Citation2021; Alieva, Moffitt, & Carley, Citation2022; Hagen, Neely, Keller, Scharf, & Vasquez, Citation2022; Uyheng & Carley, Citation2019). We have also used stance detection analysis to compute positive and negative stances about vaccination in PA for users and messages. To identify the communication strategies of anti-vax spreaders, we have implemented BEND maneuver analysis which includes 16 categories of maneuvers for online persuasion and manipulation (Beskow & Carley, Citation2019). The BEND framework serves as a tool for deciphering strategic engagement and information maneuvers (Carley, Citation2020). The framework divides maneuvers in information space regarding positive and negative actions related to actions affecting narrative or network structure. Narrative maneuvers focus on the content of the message, while network maneuvers show network communities and structures. We have used ORA software to compute BEND analysis (see for an overview of BEND maneuvers).
Table 3. Categories for BEND maneuvers
For stance detection analysis, we have compiled a list of common hashtags and URLs where each hashtag and URL has a stance code: either negative, positive, or neutral. We have used this list with ORA software to code tweets in our dataset. As a result, we have implemented a mixed-method approach using quantitative analysis of the most influential stories and narratives (e.g., network analytics, Louvain clustering, BEND maneuvers, stance detection) and qualitative observations (e.g., qualitative discourse analysis, textual analysis) of the disinformation trends found in southwestern Pennsylvania with the focus on the major cities and counties (see ). We have compiled weekly reports identifying the trending hashtags, stories, and tweets per week with a deeper focus on popular disinformation stories and narratives. Those weekly reports are available online (Alieva, Robertson, & Carley, Citation2021).
Results
As a result of the data analysis for the April 2021 – February 2022, we found main topics and trends in stories, such as wearing masks; implementing vaccine mandates; COVID-19 new variants; vaccination and masks for children; COVID-19 regulations at schools; coronavirus issues related to sports and athletes, military and the US Army, as well as the trending topic of a booster vaccination. Overall, we have visualized the number of tweets about COVID-19 over time (See ). The number of tweets has increased over the summer of 2021 (see July), reached two peaks in May and September 2021, and started slowly decreasing with occasional spikes. Based on the tweets collected during these dates, the peak in May 2021 is attributed to the new CDC guidelines that allowed vaccinated individuals to forgo masks indoors and outdoors. However, many organizations and schools in Pennsylvania opted to maintain mask mandates. Another peak occurred in September 2021 following the US president’s announcement of vaccine mandates for employees nationwide.
Twitter analysis in ORA computes lists of the main super spreaders and super friends. The leading super spreader list of vaccine hesitancy stories in Pennsylvania includes the Children’s Health Defense website (childrenshealthdefense.org) and the founder of the organization Robert F. Kennedy Jr. (@RobertKennedyJr). Other organizations and websites on the list that actively spread disinformation, anti-vax, and vaccine hesitancy narratives are: One America News Network or One America News (OANN or OAN); Red Voice Media; Daily Caller; video platforms Rumble and Bitchute; Epoch Times; and media organizations such as dailyexpose.uk; www.dailymail.co.uk; theblaze.com; expose.uk; thepostmillennial.com; www.rebelnews.com; and others, as well as multiple Twitter users. YouTube links with disinformation content sometimes were found in our analysis but were eventually deleted by the platform, while services like Rumble and Bitchute do not moderate COVID-19 disinformation. Most of the trends are related to the narratives that occurred nationally; however, we could also identify several local narratives. Most of them were related to the the local numbers of COVID-19 cases and deaths as well as discussions of mask and vaccination mandates by the local groups. We have identified a negative framing of individual stories spread by the media in the state, such as one of them with the headline “518 Fully Vaccinated Pennsylvania Residents Have Died Of COVID-19.” Stories like this do not necessarily contain disinformation but induce negative attitudes about vaccination. We could also find disinformation stories that claim severe side effects after getting the COVID-19 vaccine (a story with the headline “Pennsylvania girl suffers a stroke and brain hemorrhage 7 days after being vaccinated”). We could also identify local media narratives promoting vaccine hesitancy framing in their stories (e.g., a local story with the headline “‘I’m Not Willing To Go Through This Again:’ Woman Diagnosed With Tinnitus After COVID Vaccine”). Previous research found that messages with negative framing result in a more substantial persuasive effect (Block & Keller, Citation1995). Ashwell and Murray (Citation2020) found that negatively framed news is perceived as more credible and, therefore, more easily accepted. For that reason local healthcare professionals should address stories with negative framing of vaccination to avoid detrimental consequences.
Also, among anti-vax and disinformation topics, the users focused on promoting natural immunity and various alternative treatments, including ivermectin. In addition to the previous topics, the emphasis was often on the “experimental” nature of the COVID-19 vaccine and many misrepresentations of data and scientific findings. Local stories would cover the national context, Pennsylvania, and nearby states. Many, if not most, of the influencers spreading disinformation, originate from outside Pennsylvania. Still, we find them and their tweets in our data as they are being retweeted, quoted, and replied to by Twitter users in Pennsylvania. The most popular pro-vaccination tweet in the entire dataset: “So y’all banning abortions while simultaneously saying you can’t force people to get vaccinated because it’s their body … make it make sense,” while the most popular anti-vaccination tweet in the dataset: “They’re not ‘vaccine passports,’ they’re movement licenses. It’s not a vaccine, it’s experimental gene therapy. ‘Lockdown’ is at best completely pointless universal medical isolation and at worst ubiquitous public incarceration. Call things what they are, not their euphemisms.” Our analysis indicates that negative framing of the messages tends to attract people’s attention and motivate them to share it, as observed in both the pro-vaccination message that incorporates negative views on abortion bans and the anti-vax message that employs conspiracy theories such as “experimental gene therapy” and narratives that emphasize a perceived threat to individual freedom. Generally, lockdowns and vaccine mandates received the most negative responses in the tweets.
With BotHunter and stance detection analysis, we could identify the trends in Twitter communication between bots and non-bots and users with positive and negative stances about vaccination. See for a difference between bots and non-bots over time and for a difference between users with positive and negative stances about vaccination. The overall full dataset indicates the prevalence of positive stance messages about vaccination. Nevertheless, starting from October 2021, the number of positive and negative messages became almost equal. This trend suggests that users who support vaccination are becoming less active over time. Although the number of users posting negative messages also decreased over time, the negative stance narratives still formed an increased share of the overall total tweets. This increase in share could result from the decrease in positive stance narratives. However, the overall communication network is characterized by the presence of echo chambers and polarized communities (see ).
Figure 4. Distribution of PA tweets between actors propagating negative stance narratives (red) and positive stance narratives (green) over time.
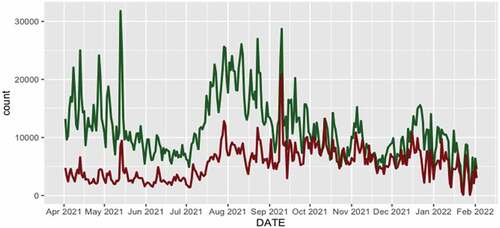
Figure 5. All-communication network for users shows the prevalence of positive stance users (green agents) over negative stance users (red agents).
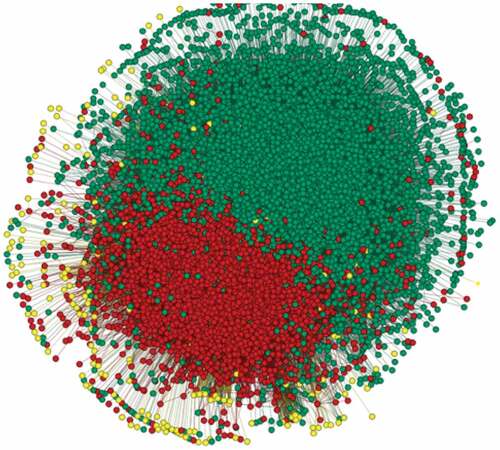
For the next step, we have used Louvain clustering and investigated the most influential groups in the dataset. Since we aimed to investigate all anti-vax users (bots and non-bots identified by the algorithm), we employed a mixed-method approach to qualitatively examine top influencers in each Louvain group and identify a group with the most influential anti-vax users.
The anti-vax users are either real users, bots, trolls, or cyborgs that spread anti-vax messages, vaccine hesitancy, and COVID-19 disinformation narratives online. In this context, “bot” is an account fully managed by computer software and programmed to produce automated messages, while “cyborg” can refer to either a human aided by a bot or a bot aided by a human. A “troll” uses social media to intentionally provoke an emotional reaction from as many users as possible by posting offensive and emotionally charged content (Paavola, Helo, Jalonen, Sartonen, & Huhtinen, Citation2016). The diverse nature of the accounts highlights why relying solely on computational methods is not always possible. As a result, we used a qualitative approach to manually check each list of the most influential users and identify anti-vax users. Next, we examined the Louvain clusters where those users were present. After conducting a stance detection analysis, we identified that the same group was leading in negative stance messaging about vaccination. Therefore, we ran a BEND maneuvers report for the group with the most influential anti-vax and negative stance users to investigate the narratives and strategies used to amplify the anti-vax discourse.
After investigating the lists of influencers, we discovered that anti-vax users tend to be disseminated by groups of other users. They also engage in frequent two-way communication and facilitate large or strong communication networks. Furthermore, they have high values of degree centrality (linked to many users), communicate in groups, and demonstrate their intent to influence other users by frequently retweeting, replying, mentioning, and quoting. The following analysis provides an overview of the BEND analysis, highlighting examples of harmful communication maneuvers.
BEND Maneuvers in Pennsylvania COVID-19 Twitter Discourse
BEND maneuver analysis includes 16 categories of maneuvers for online persuasion and manipulation. We found that primarily positive narrative maneuvers (Explain, Excite, Engage), positive network maneuvers (Back, Build, Boost, Bridge), and certain negative narrative maneuvers (Dismiss, Dismay and Distract) prevailed in the conversation, while Enhance and Neglect were not actively implemented (see ).
We will discuss each group of the maneuvers we found and provide examples. The negative narrative maneuvers, namely Dismiss, Distract, Distort, and Dismay, are employed to amplify disinformation. The Dismiss maneuver is used to express denial of facts, the Distort maneuver is used to change or reinterpret information, and the Distract maneuver is used to create noise and confusion. Meanwhile, the Dismay maneuver causes attitudes of sadness, fear, anxiety, or anger (see for examples).
Table 4. Examples for dismiss, distract, distort, and dismay
Anti-vax users also utilize positive narrative maneuvers such as Explain, Enhance, Excite, and Engage. Explain provides additional details and context, while Enhance covers the views of others and provides more information about the discourse. Excite is used to attract the audience through positive expression, and Engage provides more arguments for better associations with a particular idea. See examples of these maneuvers from anti-vax users in .
Table 5. Examples for explain, enhance, excite, and engage
Negative network maneuvers, such as Neutralize, Nuke, Narrow, and Neglect, attempt to eliminate the impact of counternarratives in the conversation. Neutralize targets a particular influential opinion, while Nuke is used to split the community. Narrow polarizes and isolates groups, while Neglect is used to reduce the community (see examples in ).
Table 6. Examples of neutralize, nuke, narrow, and neglect
Positive network maneuvers, including Build, Back, Boost, and Bridge, strengthen connections between actors in a community network. Build creates communities, while Back supports the opinions of a group. Boost maneuver enhances the connections between network actors, and Bridge adds linkages between various groups (see examples in ).
Table 7. Examples for build, back, boost, and bridge
Generally, we observe that B maneuvers (positive network maneuvers), E maneuvers (positive narrative maneuvers), and D maneuvers (Dismiss, Dismay, Distract) are predominantly present in discussions on vaccine hesitancy and anti-vax sentiments on Twitter in southwestern Pennsylvania. These maneuvers aim to promote vaccine hesitancy and anti-vax discourse by building a community around anti-vax narratives and engaging the target audience with more controversial discourse.
Conclusion
Addressing vaccine hesitancy requires debunking disinformation that is spread online. To combat these harmful narratives, we have developed a systematic approach that enables health practitioners and public health communicators to identify and address online disinformation at the community level. Our computational pipeline gathers social media data about COVID-19 in a specific geographic area and uses it to identify disinformation narratives being spread within the community at the state level.
Our analysis revealed that negative messaging often attracts people’s attention and encourages them to share it. This pattern was observed in both pro-vaccination and anti-vaccination messages. Tweets expressing negativity toward lockdowns and vaccine mandates were the most prevalent. Furthermore, we identified that anti-vaccination users employ positive network and narrative maneuvers to promote vaccine hesitancy and anti-vaccination beliefs on Twitter in southwestern Pennsylvania by building a community around anti-vax narratives and engaging the target audience with controversial discourse.
Limitations
By focusing on a specific geographic location, such as a state, city, or county, we were able to identify and analyze disinformation being spread at the local level, providing a more effective approach to understanding the problem and developing countermeasures. While this method may limit our ability to extrapolate our results to other locations and states, it can be applied by various organizations in different locations. Moreover, this study’s focus on a smaller geographic area can also be a limitation, as it may hinder our ability to observe more significant trends in disinformation. The smaller number of tweets in these areas could make it difficult to draw conclusions about disinformation patterns and compare them with larger cities where more tweets originate. Furthermore, this study only covers Twitter, and a multi-platform approach would provide richer data and enhance our analysis.
Recommendations
To ensure effective health communication strategies, it is crucial to prioritize official and timely communication, shape attitudes toward vaccination beforehand, and reframe social media as a valuable resource. Healthcare organizations should implement social media strategies to counter disinformation. Positive messages on platforms like Twitter can promote behavior change and build positive attitudes about vaccination. Communication strategies should be adjusted based on the audience and the epidemiological situation. This data-driven approach can guide communication strategies for public health organizations, civil society, mass media, and other community-centered groups during the pandemic.
Acknowledgments
This paper is the outgrowth of research in the Center for Computational Analysis of Social and Organizational Systems (CASOS) and the Center for Informed Democracy and Social Cybersecurity (IDeaS) at Carnegie Mellon University. This work was supported in part by both centers, the Henry L. Hillman Foundation, and the Knight Foundation. The views and conclusions contained in this document are those of the authors and should not be interpreted as representing the official policies, either expressed or implied, of the sponsoring organizations.
Disclosure statement
No potential conflict of interest was reported by the author(s).
Correction Statement
This article has been republished with minor changes. These changes do not impact the academic content of the article.
Additional information
Funding
References
- Ahinkorah, B. O., Ameyaw, E. K., Hagan, J. E., Jr, Seidu, A. A., & Schack, T. (2020). Rising above misinformation or fake news in Africa: Another strategy to control COVID-19 spread. Frontiers in Communication, 5, 45. doi:10.3389/fcomm.2020.00045
- Alieva, I., & Carley, K. M. (2021). Internet trolls against Russian opposition: A case study analysis of twitter disinformation campaigns against Alexei Navalny. In 2021 IEEE International Conference on Big Data (Big Data), Orlando, FL, USA. (pp. 2461–2469). IEEE.
- Alieva, I., Moffitt, J. D., & Carley, K. M. (2022). How disinformation operations against Russian opposition leader Alexei Navalny influence the international audience on Twitter. Social Network Analysis and Mining, 12(1), 80. doi:10.1007/s13278-022-00908-6
- Alieva, I., Ng, L. H. X., & Carley, K. M. (2022). Investigating the spread of Russian disinformation about biolabs in Ukraine on Twitter using social network analysis. In 2022 IEEE International Conference on Big Data (Big Data), Osaka, Japan (pp. 1770–1775). IEEE.
- Alieva, I., Robertson, D., & Carley, K. M. (2021). PA vaccine conversations and misinformation on Twitter. Center for Informed Democracy & Social - cybersecurity (IDeaS). https://www.cmu.edu/ideas-social-cybersecurity/research/pa-vaccine-hesitancy-project.html.
- Allcott, H., Boxell, L., Conway, J., Gentzkow, M., Thaler, M., & Yang, D. (2020). Polarization and public health: Partisan differences in social distancing during the coronavirus pandemic. Journal of Public Economics, 191, 104254. doi:10.1016/j.jpubeco.2020.104254
- Allington, D., Duffy, B., Wessely, S., Dhavan, N., & Rubin, J. (2020). Health-protective behaviour, social media usage and conspiracy belief during the COVID-19 public health emergency. Psychological Medicine, 9(10), 1–7. doi:10.1017/S003329172000224X
- Ashwell, D., & Murray, N. (2020). When being positive might be negative: An analysis of Australian and New Zealand newspaper framing of vaccination post Australia’s No Jab No Pay legislation. Vaccine, 38(35), 5627–5633. doi:10.1016/j.vaccine.2020.06.070
- Barrios, J. M., & Hochberg, Y. V. (2020). Risk perception through the lens of politics in the time of the COVID-19 pandemic. University of Chicago, Becker Friedman Institute for Economics Working Paper No. 2020-32. 10.2139/ssrn.3568766
- Beskow, D. M., & Carley, K. M. (2018). Bot-hunter: A tiered approach to detecting & characterizing automated activity on Twitter. SBP-BRiMS: International Conference on Social Computing, Behavioral-Cultural Modeling and Prediction and Behavior Representation in Modeling and Simulation, Pittsburgh, PA, USA, vol. 3.
- Beskow, D. M., & Carley, K. M. (2019). Social cybersecurity: An emerging national security requirement. Military Review, 99(2), 117–127.
- Blane, J. T., Bellutta, D., & Carley, K. M. (2022). Social-cyber maneuvers analysis during the COVID-19 vaccine initial rollout. Journal of Medical Internet Research, 24(3), e34040. doi:10.2196/34040
- Block, L. G., & Keller, P. A. (1995). When to accentuate the negative: The effects of perceived efficacy and message framing on intentions to perform a health-related behavior. Journal of Marketing Research, 32(2), 192–203. doi:10.1177/002224379503200206
- Blondel, V. D., Guillaume, J. L., Lambiotte, R., & Lefebvre, E. (2008). Fast unfolding of communities in large networks. Journal of Statistical Mechanics: Theory & Experiment, 2008(10), P10008. doi:10.1088/1742-5468/2008/10/P10008
- Bonnevie, E., Gallegos-Jeffrey, A., Goldbarg, J., Byrd, B., & Smyser, J. (2021). Quantifying the rise of vaccine opposition on Twitter during the COVID-19 pandemic. Journal of Communication in Healthcare, 14(1), 12–19. doi:10.1080/17538068.2020.1858222
- Brzezinski, A., Kecht, V., Van Dijcke, D., & Wright, A. L. (2020). Belief in science influences physical distancing in response to COVID-19 lockdown policies. University of Chicago, Becker Friedman Institute for Economics Working Paper No. 2020-56. 10.2139/ssrn.3587990
- Carley, K. M. (2014). ORA: A toolkit for dynamic network analysis and visualization. In R. Alhajj & J. Rokne (Eds.), Encyclopedia of social network analysis and mining (pp. 1219–1228). New York, NY: Springer. doi:10.1007/978-1-4614-6170-8_309.
- Carley, K. M. (2020). Social cybersecurity: An emerging science. Computational & Mathematical Organization Theory, 26(4), 365–381. doi:10.1007/s10588-020-09322-9
- Carley, K. M., Cervone, G., Agarwal, N., & Liu, H. (2018). Social cyber-security. In SBP-BRiMS: International conference on social computing, behavioral-cultural modeling and prediction and behavior representation in modeling and simulation (pp. 389–394). Springer, Cham.
- Carley, L. R., Reminga, J., & Carley, K. M. (2018). ORA & NetMapper. SBP-BRiMS: International conference on social computing, behavioral-cultural modeling and prediction and behavior representation in modeling and simulation, Pittsburgh, PA, USA, Springer, vol. 3, no. 3.3, p. 7.
- Chou, W. Y. S., & Budenz, A. (2020). Considering emotion in COVID-19 vaccine communication: Addressing vaccine hesitancy and fostering vaccine confidence. Health Communication, 35(14), 1718–1722. doi:10.1080/10410236.2020.1838096
- Cornelson, K., & Miloucheva, B. (2020). Political polarization, social fragmentation, and cooperation during a pandemic. Toronto, ON, Canada: University of Toronto, Department of Economics.
- Crouse Quinn, S. (2008). Crisis and emergency risk communication in a pandemic: A model for building capacity and resilience of minority communities. Health Promotion Practice, 9(4_suppl), 18S–25S. doi:10.1177/1524839908324022
- Dubé, E., Laberge, C., Guay, M., Bramadat, P., Roy, R., & Bettinger, J. A. (2013). Vaccine hesitancy: An overview. Human Vaccines & Immunotherapeutics, 9(8), 1763–1773. doi:10.4161/hv.24657
- Fallis, D. (2015). What is disinformation? Library Trends, 63, 401–426. doi:10.1353/lib.2015.0014
- French, J., Deshpande, S., Evans, W., & Obregon, R. (2020). Key guidelines in developing a pre-emptive COVID-19 vaccination uptake promotion strategy. International Journal of Environmental Research and Public Health, 17(16), 5893. doi:10.3390/ijerph17165893
- Hagen, L., Neely, S., Keller, T. E., Scharf, R., & Vasquez, F. E. (2022). Rise of the machines? Examining the influence of social bots on a political discussion network. Social Science Computer Review, 40(2), 264–287. doi:10.1177/0894439320908190
- Hernandez, R. G., Hagen, L., Walker, K., O’Leary, H., & Lengacher, C. (2021). The COVID-19 vaccine social media infodemic: Healthcare providers’ missed dose in addressing misinformation and vaccine hesitancy. Human Vaccines & Immunotherapeutics, 17(9), 2962–2964. doi:10.1080/21645515.2021.1912551
- Hornik, R., Kikut, A., Jesch, E., Woko, C., Siegel, L., & Kim, K. (2021). Association of COVID-19 misinformation with face mask wearing and social distancing in a nationally representative US sample. Health Communication, 36(1), 6–14. doi:10.1080/10410236.2020.1847437
- Landwehr, P. M., & Carley, K. M. (2014). Social Media in Disaster Relief. In W. Chu (Ed.), Data Mining and Knowledge Discovery for Big Data. Studies in Big Data (Vol. 1.). Berlin, Heidelberg: Springer. doi:10.1007/978-3-642-40837-3_7
- Lindeman, M., Svedholm-Häkkinen, A. M., & Riekki, T. J. (2022). Searching for the cognitive basis of anti-vaccination attitudes. Thinking & Reasoning, 29(1), 1–26. doi:10.1080/13546783.2022.2046158
- Mummert, A., & Weiss, H. (2013). Get the news out loudly and quickly: The influence of the media on limiting emerging infectious disease outbreaks. PLos One, 8(8), e71692. doi:10.1371/journal.pone.0071692
- Paavola, J., Helo, T., Jalonen, H., Sartonen, M., & Huhtinen, A. -M. (2016). Understanding the trolling phenomenon: The automated detection of bots and cyborgs in the social media. Journal of Information Warfare, 15(4), 100–111. https://www.jstor.org/stable/26487554
- Painter, M. O., & Qiu, T. (2020). Political belief affect compliance with COVID-19 social distancing orders. Covid Economics: Vetted and Real-Time Papers, 4, 103–123. doi:10.2139/ssrn.3569098
- Puri, N., Coomes, E. A., Haghbayan, H., & Gunaratne, K. (2020). Social media and vaccine hesitancy: New updates for the era of COVID-19 and globalized infectious diseases. Human Vaccines & Immunotherapeutics, 16(11), 2586–2593. doi:10.1080/21645515.2020.1780846
- Riley, A. H., Sangalang, A., Critchlow, E., Brown, N., Mitra, R., & Campos Nesme, B. (2021). Entertainment-education campaigns and COVID-19: How three global organizations adapted the health communication strategy for pandemic response and takeaways for the future. Health Communication, 36(1), 42–49. doi:10.1080/10410236.2020.1847451
- Scannell, D., Desens, L., Guadagno, M., Tra, Y., Acker, E., Sheridan, K. … Rosner, M. (2021). COVID-19 vaccine discourse on Twitter: A content analysis of persuasion techniques, sentiment and mis/disinformation. Journal of Health Communication, 26(7), 443–459. doi:10.1080/10810730.2021.1955050
- Sherman, E. (2018). Dictionary.Com’s word of the year is “Misinformation”: A slap at high tech. http://fortune.com/2018/11/26/misinformation-dictionary-com-word-year-misinformation-social-media-tech
- Uyheng, J., & Carley, K. M. (2019). Characterizing Bot Networks on Twitter: An Empirical Analysis of Contentious Issues in the Asia-Pacific. In R. Thomson, H. Bisgin, C. Dancy, & A. Hyder (Eds.), Social, Cultural, and Behavioral Modeling. SBP-BRiMS 2019. Lecture Notes in Computer Science (Vol. 11549). Cham: Springer. doi:10.1007/978-3-030-21741-9_16
- Vaughan, E., & Tinker, T. (2009). Effective health risk communication about pandemic influenza for vulnerable populations. American Journal of Public Health, 99(S2), (October 1, 2009), S324–332. doi:10.2105/AJPH.2009.162537.
- Vos, S. C., & Buckner, M. M. (2016). SociaL media messages in an emerging health crisis: Tweeting Bird Flu. Journal of Health Communication, 21(3), 301–308. doi:10.1080/10810730.2015.1064495
- Wesselink, A. K., Hatch, E. E., Rothman, K. J., Wang, T. R., Willis, M. D., Yland, J. … Crowe, H. M. (2022). A prospective cohort study of COVID-19 vaccination, SARS-CoV-2 infection, and fertility. American Journal of Epidemiology, 191(8), 1383–1395. doi:10.1093/aje/kwac011
- World Health Organization. (2020). COVID-19 global risk communication and community engagement strategy, December 2020-May 2021: interim guidance, 23 December 2020 (No. WHO/2019-nCoV/RCCE/2020.3). World Health Organization. https://www.who.int/publications/i/item/covid-19-global-risk-communication-and-community-engagement-strategy