Abstract
Public distrust in official sources of health information and uncertainty about novel guidelines may discourage engagement in recommended disease prevention practices. The current study tests the hypothesis that building confidence in positive behavioral outcomes may support recommendation following even in the context of low trust in recommendation sources. This set of longitudinal studies examines the main and interaction effects of trust in official sources and behavioral beliefs in their prediction of recommended COVID-19 prevention behaviors (facemask wearing, social distancing, vaccination). Repeated measurement data were collected from a nationally representative sample of U.S. adults in May/June 2020 (T1; n = 1074), July 2020 (T2; n = 889), and April/June 2021 (T3; n = 750). All five tests, using lagged linear regression, found negative interactions between trust and behavioral beliefs, as hypothesized; three were significant (T1-T2: facemask wearing B=-0.10, SE = 0.04; T1-T3: social distancing B=-0.20, SE = 0.06; T2-T3 vaccination B=-0.27, SE = 0.10) and two were consistent albeit not significant (T1-T3: social distancing B=-0.13, SE = 0.08; T1-T3: facemask wearing B=-0.11, SE = 0.06). Supporting hypotheses, trust in recommendation sources predicted behavior most among those who were less certain about behavioral outcomes and confidence in behavioral benefits predicted behavior most among those with low trust in recommendation sources. Theoretical and practical implications are discussed.
Over the past two decades, public trust in media, governments, and scientific experts has declined in the United States (U.S.) and in many other countries (Flew, Citation2021; Latkin et al., Citation2020). For example, in the U.S., trust in scientists increased early in the COVID-19 pandemic, but has remained below pre-pandemic levels since the end of 2020 (Kennedy, Tyson, & Funk, Citation2022). Between 2020 and 2022, confidence in the scientific community decreased from 48% to 38% overall and, although trust varies sharply contingent on political affiliation, confidence has declined among both Republicans and Democrats (Davern, Bautista, Freese, Herd, & Morgan, Citation2023). Low trust in experts may contribute to the rejection of scientifically-supported prevention behaviors (Bargain & Aminjonov, Citation2020). While rebuilding trust in official information channels is an important long-term priority, research is also needed to identify effective strategies for communicating time-sensitive guidelines when trust is low. The current study considers one potential communication approach that may be effective in the context of low trust in recommendation sources: targeting beliefs about the outcomes of recommended behaviors (i.e., behavioral beliefs).
Behavioral beliefs may not always align with trusted sources. Individuals encounter health information from a variety of sources (Research Center, Citation2021; Shafiq et al., Citation2021). Positive coverage of recommended behaviors may lead to pro-behavioral beliefs, regardless of trust in official recommendation sources. Complementarily, negative or conflicting coverage may lead to anti-behavioral beliefs, despite trust in official sources (Nagler, Citation2014). In these contexts—when these two cognitive predictors of behavior conflict—what are the implications for behavior?
Drawing from behavior change and dual-processing theories, this study examines how trust in official sources and behavioral beliefs impact recommended COVID-19 prevention behaviors and whether believing in the benefits of these behaviors mitigates reliance on source trust to inform decisions. If so, communication interventions that emphasize positive behavioral outcomes may be especially persuasive among those with low trust in recommendation sources. This study strikes new ground as it examines a nationally representative sample, tests over time predictions, measures predictors and outcomes in a natural context, and establishes effects of three distinct behaviors of public health importance.
Literature Review
Behavioral Beliefs as Predictors of Recommended Behaviors
Based on theory and prior research, prevention behaviors are likely to be substantially informed by behavioral beliefs. According to the integrative model of behavior prediction (IMBP), behavior-specific beliefs influence behavioral attitudes, which—along with, self-efficacy, and perceived norms—predict intention to engage in a behavior (Fishbein & Ajzen, Citation2011). Intention, in turn, predicts behavior (Fishbein & Ajzen, Citation2011). There is strong empirical evidence supporting the theorized relationship between behavioral beliefs and behavioral outcomes (Ajzen, Citation2011; Armitage & Conner, Citation2001; McEachan, Conner, Taylor, & Lawton, Citation2011; Schulze & Wittmann, Citation2003; Sheeran, Orbell, & Trafimow, Citation1999). Consistent with this broader literature, recent studies have found associations between positive behavioral beliefs and adoption of COVID-19 prevention behaviors (Chu & Liu, Citation2021; Guillon & Kergall, Citation2020; Head, Kasting, Sturm, Hartsock, & Zimet, Citation2020; Hornik et al., Citation2021; Reiter, Pennell, & Katz, Citation2020; Woko, Siegel, & Hornik, Citation2020). The current study expands prior work by testing the effects of behavioral beliefs on three distinct behaviors over time in the context of trust in public health sources. The following was hypothesized:
H1: Positive behavioral beliefs will predict more frequent engagement in recommended prevention behaviors (facemask wearing, social distancing, vaccination).
Source Trust as Predictor of Recommended Behaviors
Trust in recommendation sources is another potential predictor of prevention behavior, which is distinct from behavioral beliefs. Broadly, trust is defined as the willingness to accept vulnerability with the expectation of positive intentions and behavior of another (Rousseau, Sitkin, Burt, & Camerer, Citation1998). In contrast to behavioral beliefs, trust encompasses perceptions pertaining to a source of information, or channel, rather than the information itself. An individual may defer the decision to engage in a behavior to a trusted source, without personally assessing the safety and benefits of the behavior. In the context of a public health recommendation, trust is the expectation that expert sources are providing guidelines that serve one’s best interest, while behavioral beliefs assess the benefits and costs of following the guidelines themselves. The current study focuses specifically on trust in public health officials as sources of information about COVID-19.
Given public health agencies are official sources of COVID-19 guidelines, one would expect trust in these agencies to have an overall impact on the protective behaviors they recommend [Centers for Disease Control and Prevention (CDC), Citation2023; World Health Organization (WHO), Citation2021]. Trust in a recommendation source has the potential to increase attention to messages (Jones, Sinclair, & Courneya, Citation2003), validate message arguments, and influence behavior-specific beliefs (Chaiken, Lieberman, & Eagly, Citation1989; Heesacker, Petty, & Cacioppo, Citation1983; Hovland & Weiss, Citation1951). For example, a panel study by Kim and Tandoc (Citation2021) found initial trust in the government was associated with subsequent pro-facemask wearing beliefs and later behavior. This prior study highlights the potential for trust in official guideline sources to influence behavior through behavior-specific beliefs. Based on prior studies, the current study hypothesized the following for all three behaviors measured:
H2: Trust in public health information sources will predict more frequent engagement in recommended prevention behaviors (facemask wearing, social distancing, vaccination).
Dual Processing and the Interaction of Behavioral Beliefs and Source Trust
According to dual-processing theories, believing in the benefits of recommended behaviors may attenuate the effects of distrust in recommendation sources. Specifically, the heuristic systematic model (HSM)’s attenuation hypothesis holds that when heuristics and message arguments conflict, beliefs derived from systematic processing can outweigh the impact of heuristics (Chaiken & Maheswaran, Citation1994; Todorov, Chaiken, & Henderson, Citation2002). The HSM and other dual-processing models posit that individuals make decisions based on one of the two types of processes: automatic (i.e., Type 1, peripheral, or heuristic processing) and deliberate (i.e., Type 2, central, or systematic processing) (Chaiken, Lieberman, & Eagly, Citation1989; Evans, Citation2012; Petty & Cacioppo, Citation1986; Todorov, Chaiken, & Henderson, Citation2002). While heuristic processing usually involves reliance on cognitive shortcuts to inform decisions, systematic processing usually involves attention to the quality of message arguments (Petty & Cacioppo, Citation1986; Todorov, Chaiken, & Henderson, Citation2002). The notion that experts are (or are not) trustworthy may serve as a heuristic, biasing judgments only when one is unable or unmotivated to engage in systematic processing (Carpenter, Citation2015; Petty & Cacioppo, Citation1986; Todorov, Chaiken, & Henderson, Citation2002). However, if people form reasoned judgments about the benefits of engaging in a recommended behavior, they may follow the recommendation regardless of trust in the source (Todorov, Chaiken, & Henderson, Citation2002). While the current study does not specifically test dual-processing theories, these models provide an explanation as to why trust may have only conditional effects on behavior.
Supporting the heuristic model, prior work has found that deliberative processing of strong message arguments can override the influence of trust in the message source (Mak, Schmitt, & Lyytinen, Citation1997; Rosen, Citation2000; Stephenson, Benoit, & Tschida, Citation2009). In the COVID-19 context, experimental studies have tested dual-process models in accepting recommendations (Swami & Barron, Citation2021; Thoma, Weiss-Cohen, Filkuková, & Ayton, Citation2021) and examined the interaction of exposure to messages from low versus high trusted sources and with stronger versus weaker behavioral belief messages (CDC, Citation2020a; Citation2020b). These studies support the potential interaction effect of perceptions pertaining to a message channel (i.e., source trust) and beliefs pertaining to the message content (i.e., behavioral beliefs). However, this work is largely experimental, relies on attitudes rather than behaviors as outcomes, and does not operate in real contexts.
In contrast, survey studies have identified associations between trust and behavioral beliefs on real-world COVID-19 prevention behavior (e.g., Chu & Liu, Citation2021; Head, Kasting, Sturm, Hartsock, & Zimet, Citation2020; Kim & Tandoc, Citation2021). These studies offer support for the main effects of each predictor on behavior and for the mediating role of beliefs in the relationship between source trust and behavior (Kim & Tandoc, Citation2021). However, they do not measure the potential moderating effects of trust. Further, most of these survey studies are cross-sectional, limiting the ability to sort causal order between variables.
To the author’s best knowledge, this is the first longitudinal survey study to measure whether trust in recommendation sources moderates the relationship between behavioral beliefs and behavior. The use of observational data and examination of three behavioral outcomes strengthens external validity laboratory studies assessing attitudinal outcomes. Longitudinal analysis supports stronger claims than a cross-sectional analysis might; it establishes causal order (since the predictors precede the outcome behavior) and adjusts for baseline behavior (reducing concern about potential confounding variables).
The current study assesses whether trust (or distrust) in recommendation sources and beliefs about recommended behaviors may substitute for one another in their effects on subsequent behavior when the other is low. In particular, of relevance to public health campaigns, the interpretation focuses on the effects of behavioral beliefs when trust is low. Based on prior literature and dual-processing theories, the following was hypothesized:Footnote1
H3: There will be a negative interaction effect of level of trust in public health information sources and behavioral beliefs on engagement in recommended prevention behaviors (facemask wearing, social distancing, vaccination), such that each will more strongly predict behavior when the other is low.
Methods
Sample
The current study uses data from a larger project focused on identifying predictors of COVID-19 prevention behaviors during the first year of the pandemic. A three-wave nationally representative probability sample of U.S. residents was recruited between 05/26–6/12/20 (T1; n = 1074; cooperation rate 54%), 07/15–07/21/20 (T2; n = 889; follow-up response rate 83%), and 04/12/21–04/20/21 or 06/08/21–06/30/21 (n = 750; follow-up response rate 72% from baseline survey). The current work makes secondary use of the baseline data but had some influence on variables measured at follow-up waves. Surveys were conducted in English or Spanish online or by phone through the SSRS Opinion Panel (Social Science Research Solutions SSRS, Citation2020) (recruitment rate for the underlying panel varies from 2% to 4%). This study was approved by the Institutional Review Board of the University of Pennsylvania.
Data for this study were collected as public health guidelines shifted from a primary focus on facemask wearing and social distancing in the summer of 2020 to an additional focus on vaccination in the spring of 2021 (CDC, Citation2021). Reflecting this shift, initial analyses assess how trust and behavioral beliefs measured in May/June 2020 predicted facemask wearing and social distancing behaviors 5–6 weeks later in July 2020 and 11–12 months later in April/May 2021. Parallel analyses examine how trust in public health sources and beliefs about the safety and efficacy of COVID-19 vaccines in July 2020 predicted vaccine intentions/behavior 8–10 months later in April/June 2021 [after vaccines became available in the U.S.]. Survey weights were developed by SSRS to ensure participants matched the U.S. population on important demographic variables. For facemask wearing and social distancing, behavior change between T1 and T2 and between T1 and T3 was assessed; Vaccine-related items were only included in T2 and T3 (therefore, vaccination analyzes focus on change from T2 to T3). Table S1 provides measures for all key variables measured at each wave.
provides descriptive data comparing samples available at each wave. The table shows only the T1 estimates for the samples available at T2 and T3, after weighting, rather than scores on these variables at T2 and T3 to clarify any dropout effects. Overall, and particularly once weights were applied, the retained samples were similar to the baseline sample across demographic variables and T1 substantive variables.
Table 1. Descriptive statistics for time 1 measures comparing samples available at each measurement wave
Dependent Variables
Outcomes of interest included the adoption of three recommended COVID-19 prevention behaviors: facemask wearing, social distancing, and vaccination (CDC, Citation2020a, Citation2020b, Citation2023).
Facemask Wearing Behavior
To measure facemask wearing frequency at T1 and T2, respondents were asked in random order whether they had engaged in seven specific activities in the past week [e.g., Shopping for groceries or other necessities; meeting people socially indoors; working at a job outside your home] (Yes/No) (see Table S1 for full battery). If respondents had participated in any of the seven activities, they were asked the frequency with which they had worn a face covering or mask (1 = Never, 2 = Some of the time, 3 = Most of the time, 4 = Every time). Measures were based on Centers for Disease Control and Prevention (CDC) guidelines during the time of data collection (CDC, Citation2020a, Citation2020b). A facemask wearing scale (1–4) averaged responses to these measures, with high consistency across items (T1 and T2 alphas = 0.88; 0.93) and with all items loading on a single dimension in Principal Components Analyses (PCA). In addition, at all three waves, respondents were asked, “When you went outside your home in the past 7 days, how often did you wear a mask or other face covering?” (1 = Rarely or never, 2 = Sometimes, 3 = Most of the time, 4 = Always). This single item measure was strongly correlated with the facemask wearing scale at both T1 (r = 0.73) and T2 (r = 0.61), showing strong convergent validity. Due to space limitations, only this single-item measure was included at T3.
Social Distancing Behavior
To measure social distancing frequency at T1 and T2, participants were asked in random order whether they had engaged in five activities in the past seven days that would have violated social distancing guidelines at the time of data collection (CDC, Citation2020a, 2020b) [e.g., Gone out to a restaurant, bar, or other indoor place where people gather; Had close contact – within about 6 feet – with people who do not live with you] (Yes = 0; No = 1) (see Table S1 for full battery). These items were combined into a single social distancing scale and coded so the highest score (5) meant engaging in fewer non-compliant activities. There was moderate consistency across measures (T1 and T2 alphas = .65 and .68) and with all items loading on a single dimension in PCA. In addition, at all three waves, respondents were asked, “Social distancing is staying at least 6 feet away from other people in public places. In the past 7 days, how often have you practiced social distancing?” (1 = Rarely or never, 2 = Sometimes, 3 = Most of the time, 4 = Always). This single item measure was moderately correlated with the scales (T1 r = .57; T2 r = .49). Due to space limitations, only this single-item measure was included at T3.
Vaccination Intention/Behavior
Vaccine-related items were included at T2 and T3 only. Given vaccines were not available at T2, vaccination intention (rather than behavior) was measured at this wave. To measure T2 intention, all respondents were asked: “If you were able to get a vaccine for the coronavirus today, what is the likelihood that you would get vaccinated?” (1 = Very unlikely; 2 = Unlikely; 3 = Likely; 4 = Very likely). At T3, COVID-19 vaccines had been approved for all adults in the U.S., allowing for behavior measurement. The T3 questionnaire asked whether respondents had received at least one dose of a coronavirus vaccine (Yes/No). Those who had not received a dose were asked the same intention question as in T2. To create a T3 measure parallel to the T2 intention measure that would include all respondents, T3 intention was merged with T3 behavior, as previously validated (Kikut, Clark, Jesch, & Hornik, Citation2022). Those who were vaccinated at T3 were given the same score as unvaccinated respondents with the highest level of intention (4 = Very likely).Footnote2
Independent Variables
For facemask wearing and social distancing analyses, the independent variables of interest were T1 trust in public health officials as sources of information about COVID-19, T1 behavioral beliefs, and their interaction. For vaccination analyses, independent variables of interest were T2 trust in public health officials as sources of information about COVID-19, T2 vaccine behavioral belief, and their interaction.
T1 Trust in Public Health Information Sources
Items measuring trust in sources of COVID-19 information were adapted from Pew Research Center’s American News Pathways survey instrument (Research Center, Citation2021). Trust and distrust were assessed as the opposite ends of a single variable; participants were asked to rate how much they trust or distrust public health officials or agencies as a source of information about the coronavirus pandemic (1 = Strongly distrust, 2 = Distrust, 3 = Trust, 4 = Strongly trust). This measure was collected at T1 and T3. Single-item measures may raise concerns about measurement quality but have the potential to capture underlying constructs (Matthews, Pineault, & Hong, Citation2022). The case for quality relies on three types of evidence: over time consistency; discrimination of this measure from measures of trust in other sources; and evidence for correlation with other variables as expected. Trust in public health officials was substantially correlated with the same measure at T3 (r = 0.60). To establish discriminant validity, the T1-T3 public health source trust correlation was compared to the correlations between trust in public health sources at T1 and trust in other sources at T3. T1 trust in public health sources was less correlated with T3 trust in other sources, which were likely to be giving consistent messages (mainstream news r = 0.44, doctor r = 0.49, state and local elected officials r = 0.51). Further, supporting nomological validity, T1 trust in public health officials showed strong expected negative associations with T1 trust in President Trump (r = −0.35) and conservative news sources (r = −0.14) and positive associations with T1 trust in progressive and liberal news sources (r = 0.42) and behavioral beliefs (facemask wearing T1 r = 0.42, T2 r = 0.48; social distancing T1 r = 0.42, T2 r = 0.47).
T2 Trust in Public Health Information Sources
Due to space limitations, the measure of trust in public health officials as sources of information about the coronavirus used at T1 and T3 was not included at T2. A different measure, focusing on trust in public health officials as sources of information about coronavirus vaccines was included. In the same battery as the behavioral belief items, respondents were asked their agreement with the statement, “If you were to get vaccinated with the coronavirus, you could trust information you receive from public health officials about the vaccine” (1 = Strongly disagree; 2 = Disagree; 3 = Agree; 4 = Strongly agree). To generate a broader measure of trust parallel to the one used in Study 1, predicted values were taken from regressing the T2 vaccine-specific trust measure on the more general T1 and T3 trust measures. This score removed variance that might be specific to vaccine-related trust.
T1 Facemask Wearing and Social Distancing Behavioral Beliefs
Aligning with the IMBP, behavioral belief items focused specifically on potential outcomes of prevention behaviors (Fishbein & Ajzen, Citation2011). Six-item batteries, validated previously (Hornik et al., Citation2021), were used to measure the anticipated benefits of facemask wearing and social distancing at both T1 and T2. The following stem was used: “How much do you disagree or agree if you [wear a mask or other face covering in public places]/[maintain social distancing] every day for the next two weeks … .” For each behavior, one item pertained to benefits for oneself (you will be less likely to get sick), and four items pertained to benefits for others (e.g., you will protect more vulnerable people in society, it will prevent you from transmitting the coronavirus to others) (See Table S1 for full battery). These items were presented in random order. Response options included: Strongly disagree (1), Disagree (2), Agree (3), Strongly agree (4). Responses were averaged to create a pro-facemask belief scale (alpha = 0.93) and a pro-social distance belief scale (alpha = 0.93). The consistency between T1 and T2 (two months later) was high for both scales (facemask r = 0.75; social distancing r = 0.73).
T2 Vaccination Behavioral Beliefs
Beliefs about COVID-19 vaccines were measured at T2 only. Items were adapted from an extant scale (Larson et al., Citation2015; Shapiro et al., Citation2018). Respondents were given a battery with the stem, “If you were to receive a potential coronavirus vaccine … .” The following statements were presented in random order: you would suffer bad side effects from the vaccine (reverse coded); you would be well protected from getting infected with the coronavirus; you would be protecting others in your community from getting infected (1 = Strongly disagree; 2 = Disagree; 3 = Agree; 4 = Strongly agree). These three items were combined into a pro-vaccine beliefs scale (alpha = 0.80).
Analyses
Five analyses were conducted in Stata15.1 (Stata, Citation2017). Facemask wearing and social distancing behaviors at T2 (and separately at T3) were regressed on the main effects and the interaction between T1 trust in public health information sources and T1 pro-behavior beliefs, controlling for behavior at T1. Vaccination intention/behavior at T3 was regressed on the main effects and the interaction between estimated T2 trust in public health sources and T2 pro-vaccine belief, controlling for T2 vaccine intention.Footnote3
The T1-T2 analyses included the 83% of T1 respondents who provided information at T2 (n = 889) and the T1-T3 analyses included the 72% of T1 respondents who provided information at T3 (n = 750). The sample for vaccine analyses included only respondents who participated in both T2 and T3 (n = 659). Weights were used to represent the U.S. population; analyses used the svy Stata command to correct standard errors. To check for sensitivity to the influence of potential confounders, all analyses were re-run controlling for demographic variables—including sex, age, education, household size, partner status, marital/partner status, job requirements, home ownership, income, region, race/ethnicity, and political party.
Results
Descriptive Data
establishes the baseline similarity of the samples available at each wave, despite dropout. It is also useful to examine changes across waves on the variables of interest. Among respondents retained at all three waves, the proportion who strongly distrusted public health sources increased from 4% to 12% and the proportion who strongly trusted public health sources decreased from 25% to 21%. For both facemask wearing and social distancing, pro-behavioral belief was generally strong—most people agreed or strongly agreed that facemask wearing and social distancing would benefit others and themselves—and was fairly consistent between T1 and T2.
reports the correlations and means for facemask and social distancing analyses. There were significant correlations between T1 trust in public health sources and both behaviors. shows the summary statistics and correlations of primary variables for vaccination analyses. On average, respondents at T2 had positive beliefs about vaccine safety and efficacy (M = 2.72; 1 = most anti-vaccine and 4 = most pro-vaccine). There was a significant correlation between trust and vaccine intention/behavior.
Table 2. Weighted descriptive data and correlations of primary variables for facemask wearing and social distancing analyses
Table 3. Weighted descriptive data and correlations of primary variables for vaccine analyses
Main Effects
It was hypothesized that behavioral beliefs (H1) and trust (H2) would have main effects on adoption of each behavior. All regression results are reported in (facemask wearing and social distancing) and (vaccination). H1 was supported; controlling for behavior at baseline, prior behavioral beliefs had a significant main effect on all follow-up behaviors [T2 facemask wearing (B = 0.15; SE = 0.04) and social distancing (B = 0.30; SE = 0.07); T3 facemask wearing (B = 0.34; SE = 0.06), social distancing (B = 0.38; SE = 0.06), and vaccination intention/behavior (B = 0.65; SE = 0.13)]. Similarly, supporting H2, prior trust had a significant main effect on all T3 behaviors [facemask wearing (B = 0.28; SE = 0.07); social distancing (B = 0.24; SE = 0.07) and vaccine intention/behavior (B = 0.72; SE = 0.12)]. However, the main effect of T1 trust on T2 behaviors was not statistically significant [facemask wearing (B = 0.06; SE = 0.04) and social distancing (B = 0.07; SE = 0.08)]. Therefore, H2 was partially supported.
Table 4. OLS regression: protective behavior on time 1 trust in public health sources and pro-behavioral belief
Table 5. OLS regression: time 3 COVID-19 Vaccine intention/behavior on trust in public health sources and behavioral belief
Interaction Effects
It was hypothesized (H3) that there would be a negative interaction effect of baseline source trust and behavioral beliefs on follow-up behaviors, controlling for baseline behaviors. Supporting the interaction hypothesis, there were negative interaction effects of trust and pro-behavioral beliefs on all three behavioral outcomes. For facemask wearing, this interaction was significant in the T1-T2 analysis (B = -0.10; SE = 0.04) and in the same direction in the T1-T3 analysis (B = -0.11; SE = 0.06; p = 0.08). For social distancing, the interaction was significant in the T1-T3 analysis (B = -0.20; SE = 0.06) and in the same direction in the T1-T2 analysis (B = -0.13; SE = 0.08; p = .09). For vaccination, the interaction between trust and pro-vaccine beliefs was significant (B = -0.27; SE = 0.10). Controlling for potential confounders did not change the significance of interactions (equations not shown).
Predictive margins plots show the linear prediction of T3 facemask wearing (), T3 social distancing (), and T3 vaccination () based on pro-behavioral beliefs for those with lower and higher trust in public health sources. All figures show a steeper upward-slanted slope (i.e., stronger association between pro-behavioral belief and behavior) when trust in public health sources is low and a flatter slope when trust is high.
Figure 1. Predictive margins: facemask wearing at time 3 (T3) on pro-facemask wearing beliefs and trust/distrust in public health sources time 1 (T1).
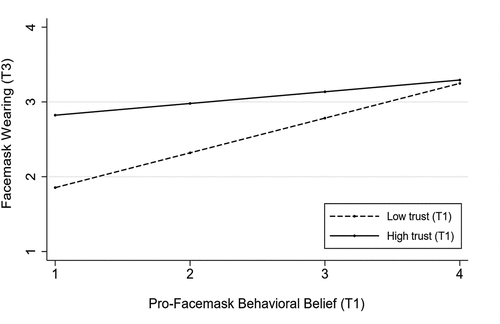
Figure 2. Predictive margins: social distancing at time 3 (T3) on Pro-social distancing beliefs and trust/distrust in public health sources at time 1 (T1).
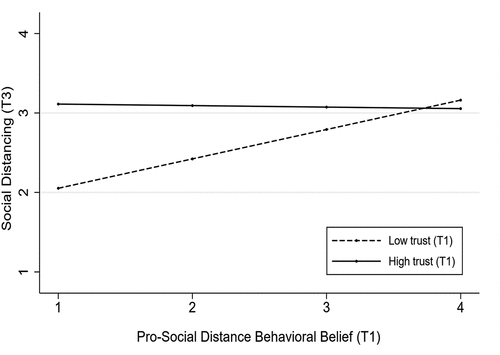
Figure 3. Predictive margins: vaccination behavior/intentions at time 3 (t3) on pro-vaccine beliefs and trust/distrust in public health sources at time 2 (T2).
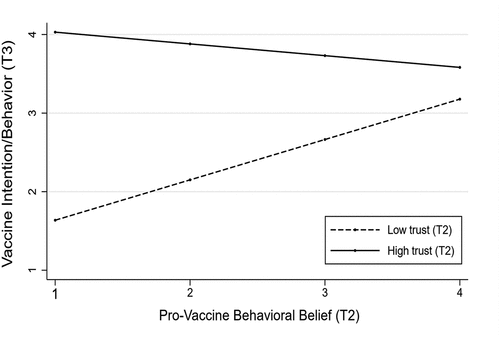
Discussion
Declining trust in institutions and experts may threaten the ability for public health agencies to encourage important disease prevention practices. Understanding the contexts in which trust does and does not influence behavior can inform health communication interventions. The current study used longitudinal survey data and drew from core theories of behavior change and persuasion to assess how source trust interacts with behavioral beliefs to predict engagement in three recommended behaviors.
Consistent with the IMBP (Fishbein & Yzer, Citation2003), and prior COVID-19 survey research (e.g., Fridman, Lucas, Henke, & Zigler, Citation2020; Hornik et al., Citation2021; Kim & Tandoc, Citation2021), evidence was found supporting the main effects of anticipated behavioral benefits at baseline on subsequent behavior for all three behavioral outcomes (facemask wearing, social distancing, and vaccination). Also, aligning with prior work, trust in recommendation sources consistently predicted recommendation behavior (Diament, Kaya, & Magenheim, Citation2021; Kim & Tandoc, Citation2021; Pornpitakpan, Citation2004; Xu, Margolin, & Niederdeppe, Citation2021). The current study offers methodological advancements, exploiting longitudinal data (strengthening causal claims compared to cross-sectional studies) and illustrating consistent main effects across three distinct behaviors and time lags (strengthening generalizability of main effects findings).
Also, extending previous work, a contingent moderation effect of trust and behavioral beliefs was observed. Specifically, behavioral beliefs were most predictive of behavior among those with low trust in public health sources. These results align with dual-processing models, suggesting that trust (and distrust) in recommendation sources may most predict behavior when individuals do not have the information or ability to make informed judgments about recommended behavioral outcomes (Todorov, Chaiken, & Henderson, Citation2002). Meanwhile, belief in the safety and efficacy of recommended behaviors may reduce the need to rely on trusted sources to inform decisions. That is, pro-behavioral beliefs (however learned) may attenuate the influence of trust on recommendation adherence (Todorov, Chaiken, & Henderson, Citation2002).
These findings advance prior literature by supporting the potential role of dual-processing in influencing real-world behavioral outcomes during public health crises. Experimental tests of dual-processing theories have found evidence supporting the notion that strong message arguments and systematic processing decrease the influence of perceived source credibility on persuasive outcomes (Mak, Schmitt, & Lyytinen, Citation1997; Petty & Cacioppo, Citation1986; Rosen, Citation2000; Stephenson, Benoit, & Tschida, Citation2009). However, such studies have been most successful in explaining attitude formation, rather than behavior change (Carpenter, Citation2015), and have been criticized for conflating manipulations of argument quality with processing type (Mongeau & Williams, Citation1996; O’Keefe, Citation2013). The current study did not manipulate or measure processing style nor test the effects of specific message features. Rather, it considered the role of existing beliefs, which align with evidence supporting recommended behaviors, contingent on a broad sense of trust in public health sources. To the best of the author’s knowledge, this is the first longitudinal observational survey study to test or show a negative interaction between behavioral beliefs and source trust in their joint influence on health recommendation adherence. The contribution of the study is enhanced because it relies on nationally representative data and shows parallel results across three recommended behaviors and over three time lags.
Strengths & Limitations
This study has several strengths—including the use of a longitudinal nationally representative panel with high cooperation and retention rates, examination of predictors of multiple behaviors, and integration of two foundational cognitive theories of behavior. Yet, this work is not without limitations. As with any observational survey study, conclusions may be weakened by reliance on self-reported behaviors, possible biases in sampling procedures, and concerns about unmeasured confounders. While the study is controlled for several demographic variables, it is possible that unmeasured variables may have influenced results. However, by controlling for behavior at baseline, this threat was reduced—unmeasured confounders would have had to impact baseline trust and beliefs and subsequent behavior over and above effects on baseline behavior. Threats to inference remain if there are unmeasured confounders that affect beliefs or trust at one time and behavior with some delay. In addition, the current study does not capture within-person effects, which may offer more granular findings on the relationships between trust, beliefs, and behavior change.
It is also worth noting that the results were parallel for all protective behaviors, though conventionally significant for facemask wearing at T2, and social distancing and vaccination at T3 and in the same direction but marginally significant (p < .10) for social distancing at T2 and facemask wearing at T3. In addition, due to space considerations in the larger survey project, trust was operationalized using a single-item measure and did not capture sub-dimensions of trust (i.e., trustworthiness and confidence) (Earle & Siegrist, Citation2008; Fiske, Cuddy, & Glick, Citation2007). Future work should consider the role of each subtype of trust in relation to behavioral beliefs and behavior. Finally, the current study measures already established beliefs and trust on behavior but does not explore the effects of exposure to messaging strategies. More research is needed to examine how COVID-19 recommendation messages can effectively encourage audiences to engage in recommended behaviors when either trust or certainty is low.
Implications & Recommendations
This study was conducted during a public health crisis, in the context of unprecedented uncertainty, proliferation of misinformation, and politicization of expert guidelines. The sociopolitical environment surrounding the COVID-19 pandemic, as well as the novel nature of the virus itself and effective prevention behaviors, called for new research to inform public health messaging. This study offers evidence that trust in public health sources is an important factor influencing prevention behaviors, as both a direct predictor and a moderator of the relationship between beliefs and behavior. For this reason, efforts to re-build and maintain trust in experts should remain a public health priority. However, the findings of the current study also suggest that behavior-specific beliefs are also consistently strong predictors of recommended behaviors, especially among low trusting individuals. That is, individuals who distrust official recommendation sources might still engage in recommended protective behaviors if presented with information that strengthens their belief in the protective benefits. As public health priorities continue to evolve, communication interventions should not overlook the promise of exposure to straightforward messaging highlighting the positive benefits and safety of protective behaviors.
Acknowledgments
Thank you to Robert Hornik, Emma Jesch, and Danielle Clark for their feedback and support on this project.
Disclosure statement
No potential conflict of interest was reported by the author(s).
Correction Statement
This article has been republished with minor changes. These changes do not impact the academic content of the article.
Additional information
Funding
Notes
2 Two factors influenced the decision to combine vaccinated respondents with those who were “very likely” to get vaccinated. First, the T3 sample was collected at two time points (April and June 2021). Respondents who were randomly selected for the June survey had two additional months to become vaccinated than those selected for the April survey. Therefore, vaccination status and intention had different meanings depending on timing of T3 participation. While 54% of respondents in the April sample had been vaccinated, 64% had been vaccinated in the June sample. Second, intention at T2 was strongly associated with behavior at T3, consistent with the IMBP (Fishbein & Ajzen, Citation2011). Of those who responded, they were “very likely” to get a hypothetical COVID-19 vaccine at T2, 82% had received at least one dose at T3; In comparison, only 23% of those who were “very unlikely” at T2 had received a dose at T3. Given nearly all high intenders were vaccinated by T3, it was assumed most unvaccinated high intenders at T3 would soon be vaccinated. This justified the creation of a T3 intention/behavior score that placed intention and behavior on the same continuum.
3 Analyses did not include measurement of the effects of T2 independent variables on T3 facemask and social distance behavior because beliefs relevant to these behaviors were not measured at T2. Similarly, analyses did not include measurement of the effects of T1 vaccination beliefs on T2 or T3 vaccination outcomes because vaccination beliefs were not measured at T1.
References
- Ajzen, I. (2011). The theory of planned behaviour: Reactions and reflections. Psychology & Health, 26(9), 1113–1127. doi:10.1080/08870446.2011.613995
- Armitage, C. J., & Conner, M. (2001). Efficacy of the theory of planned behaviour: A meta-analytic review. British Journal of Social Psychology, 40(4), 471–499. doi:10.1348/014466601164939
- Bargain, O., & Aminjonov, U. (2020). Trust and compliance to public health policies in times of COVID-19. Journal of Public Economics, 192, 104316. doi:10.1016/j.jpubeco.2020.104316
- Carpenter, C. J. (2015). A meta-analysis of the ELM’s argument quality × processing type predictions. Human Communication Research, 41(4), 501–534. doi:10.1111/hcre.12054
- Centers for Disease Control and Prevention (CDC). (2020a, May 12). Media statement from Dr. Robert Redfield, CDC Director; Dr. Steve Hahn, FDA Commissioner; and Dr. Anthony Fauci, NIAID Director. https://www.cdc.gov/media/releases/2020/s0512-statement-redfield-hahn-fauci.html
- Centers for Disease Control and Prevention (CDC). (2020b, June 25). Transcript for the CDC telebriefing update on COVID-19. https://www.cdc.gov/media/releases/2020/t0625-COVID-19-update.html
- Centers for Disease Control and Prevention (CDC). (2021, March 8). CDC issues first set of guidelines on how fully vaccinated people can visit safely with others. https://www.cdc.gov/media/releases/2021/p0308-vaccinated-guidelines.html
- Centers for Disease Control and Prevention (CDC). (2023, July 6). How to protect yourself and others. https://www.cdc.gov/coronavirus/2019-ncov/prevent-getting-sick/prevention.html
- Centers for Disease Control and Prevention (CDC). (2023, May 12). Vaccines for COVID-19. https://www.cdc.gov/coronavirus/2019-ncov/vaccines/index.html
- Chaiken, S., Lieberman, A., & Eagly, A. H. (1989). Heuristic and systematic processing within and beyond the persuasion context. In J. S. Uleman & J. A. Bargh (Eds.), Unintended thought: Limits of awareness, intention, and control (pp. 212–252). New York, NY: Guilford.
- Chaiken, S., & Maheswaran, D. (1994). Heuristic processing can bias systematic processing: Effects of source credibility, argument ambiguity, and task importance on attitude judgment. Journal of Personality and Social Psychology, 66(3), 460. doi:10.1037/0022-3514.66.3.460
- Chu, H., & Liu, S. (2021). Integrating health behavior theories to predict American’s intention to receive a COVID-19 vaccine. Patient Education and Counseling, 104(8), 1878–1886. doi:10.1016/j.pec.2021.02.031
- Davern, M., Bautista, R., Freese, J., Herd, P., & Morgan, S. L. (2023). General Social Survey 1972-2022. [Machine-readable data file]. Principal Investigator, Michael Davern; Co-Principal Investigators, Rene Bautista, Jeremy Freese, Pamela Herd, and Stephen L. Morgan. Sponsored by National Science Foundation. NORC ed. Chicago: NORC, 2023: NORC at the University of Chicago [producer and distributor]. gssdataexplorer.norc.org
- Diament, S. M., Kaya, A., & Magenheim, E. B. (2021). Frames that matter: Increasing the willingness to get the covid-19 vaccines. Social Science & Medicine, 292, 114562. doi:10.1016/j.socscimed.2021.114562
- Earle, T. C., & Siegrist, M. (2008). Trust, confidence and cooperation model: A framework for understanding the relation between trust and risk perception. International Journal of Global Environmental Issues, 8(1–2), 17–29. doi:10.1504/IJGENVI.2008.017257
- Evans, J. S. (2012). Dual-process theories of deductive reasoning: Facts and fallacies. In K. J. Holyoak & R. G. Morrison (Eds.), The Oxford handbook of thinking and reasoning (pp. 115–133). New York, NY: Oxford University Press.
- Fishbein, M., & Ajzen, I. (2011). Predicting and changing behavior: The reasoned action approach. Psychology Press. doi:10.4324/9780203838020
- Fishbein, M., & Yzer, M. C. (2003). Using theory to design effective health behavior interventions. Communication Theory, 13(2), 164–183. doi:10.1111/j.1468-2885.2003.tb00287.x
- Fiske, S. T., Cuddy, A. J. C., & Glick, P. (2007). Universal dimensions of social cognition: Warmth and competence. Trends in Cognitive Sciences, 11(2), 77–83. doi:10.1016/j.tics.2006.11.005
- Flew, T. (2021). The global trust deficit disorder: A communications perspective on trust in the time of global pandemics. Journal of Communication, 71(2), 163–186. doi:10.1093/joc/jqab006
- Fridman, I., Lucas, N., Henke, D., & Zigler, C. K. (2020). Association between public knowledge about COVID-19, trust in information sources, and adherence to social distancing: Cross-sectional survey. JMIR Public Health and Surveillance, 6(3), e22060. doi:10.2196/22060
- Guillon, M., & Kergall, P. (2020). Attitudes and opinions on quarantine and support for a contact-tracing application in France during the COVID-19 outbreak. Public Health, 188, 21–31. doi:10.1016/j.puhe.2020.08.026
- Head, K. J., Kasting, M. L., Sturm, L. A., Hartsock, J. A., & Zimet, G. D. (2020). A national survey assessing SARS-CoV-2 vaccination intentions: Implications for future public health communication efforts. Science Communication, 42(5), 698–723. doi:10.1177/1075547020960463
- Heesacker, M., Petty, R. E., & Cacioppo, J. T. (1983). Field dependence and attitude change: Source credibility can alter persuasion by affecting message‐relevant thinking. Journal of Personality, 51(4), 653–666. doi:10.1111/j.1467-6494.1983.tb00872.x
- Hornik, R., Kikut, A., Jesch, E., Woko, C., Siegel, L., & Kim, K. (2021). Association of COVID-19 misinformation with face mask wearing and social distancing in a nationally representative US sample. Health Communication, 36(1), 6–14. doi:10.1080/10410236.2020.1847437
- Hovland, C. I., & Weiss, W. (1951). The influence of source credibility on communication effectiveness. Public Opinion Quarterly, 15(4), 635–650. doi:10.1086/266350
- Jones, L. W., Sinclair, R. C., & Courneya, K. S. (2003). The effects of source credibility and message framing on exercise intentions, behaviors, and attitudes: An integration of the elaboration likelihood Model and prospect theory 1. Journal of Applied Social Psychology, 33(1), 179–196. doi:10.1111/j.1559-1816.2003.tb02078.x
- Kennedy, B., Tyson, A., & Funk, C. (2022, February 15). Americans’ trust in scientists, other groups declines. Pew Research Center. https://www.pewresearch.org/science/2022/02/15/americans-trust-in-scientists-other-groups-declines/
- Kikut, A., Clark, D., Jesch, E., & Hornik, R. (2022). Strengthened belief in vaccine effectiveness predicted increased COVID-19 vaccination intention and behaviour: Results from a nationally representative longitudinal survey of U.S. adults from July 2020 to April/May 2021. Vaccine: X, 40(42), 6035–6041. doi:10.1016/j.vaccine.2022.08.046
- Kim, H. K., & Tandoc, E. C. (2021). Wear or not to wear a mask? Recommendation inconsistency, government trust and the adoption of protection behaviors in cross-lagged TPB models. Health Communication, 37(7), 833–841. doi:10.1080/10410236.2020.1871170
- Larson, H. J., Jarrett, C., Schulz, W. S., Chaudhuri, M., Zhou, Y., Dube, E., & MacDonald, N. E. (2015). Measuring vaccine hesitancy: The development of a survey tool. Vaccine: X, 33(34), 4165–4175. doi:10.1016/j.vaccine.2015.04.037
- Latkin, C. A., Dayton, L., Strickland, J. C., Colon, B., Rimal, R., & Boodram, B. (2020). An assessment of the rapid decline of trust in US sources of public information about COVID-19. Journal of Health Communication, 25(10), 764–773. doi:10.1080/10810730.2020.1865487
- Mak, B., Schmitt, B. H., & Lyytinen, K. (1997). User participation in knowledge update of expert systems. Information & Management, 32(2), 55–63. doi:10.1016/S0378-7206(96)00010-9
- Matthews, R. A., Pineault, L., & Hong, Y.-H. (2022). Normalizing the use of single-item measures: validation of the single-item compendium for organizational psychology. Journal of Business and Psychology, 37(4), 639–673. doi:10.1007/s10869-022-09813-3
- McEachan, R. R. C., Conner, M., Taylor, N. J., & Lawton, R. J. (2011). Prospective prediction of health-related behaviours with the theory of planned behaviour: A meta-analysis. Health Psychology Review, 5(2), 97–144. doi:10.1080/17437199.2010.521684
- Mongeau, P. A., & Williams, M. L. M. (1996). Argument quality and the elaboration likelihood model of persuasion. Paper presented at the Annual Meeting of the International Communication Association, Chicago, IL.
- Nagler, R. H. (2014). Adverse outcomes associated with media exposure to contradictory nutrition messages. Journal of Health Communication, 19(1), 24–40. doi:10.1080/10810730.2013.798384
- O’Keefe, D. (2013). Elaboration likelihood model. In J. P. Dillard & L. Shen (Eds.), The SAGE handbook of persuasion: Developments in theory and practice (pp. 137–149). Thousand Oaks, CA: SAGE Publications.
- Petty, R. E., & Cacioppo, J. T. 1986. The elaboration likelihood model of persuasion. In Advances in experimental social psychology (Vol. 19, pp. 123–205) Elsevier Science & Technology. 10.1016/S0065-2601(08)60214-2
- Pornpitakpan, C. (2004). The persuasiveness of source credibility: A critical review of five decades’ evidence. Journal of Applied Social Psychology, 34(2), 243–281. doi:10.1111/j.1559-1816.2004.tb02547.x
- Reiter, P. L., Pennell, M. L., & Katz, M. L. (2020). Acceptability of a COVID-19 vaccine among adults in the United States: How many people would get vaccinated? Vaccine: X, 38(42), 6500–6507. doi:10.1016/j.vaccine.2020.08.043
- Research Center, P. (2021). How Americans Navigated the News in 2020: A Tumultuous Year in Review. https://www.journalism.org/2021/02/22/how-americans-navigated-the-news-in-2020-a-tumultuous-year-in-review/
- Rosen, C. S. (2000). Integrating stage and continuum models to explain processing of exercise messages and exercise initiation among sedentary college students. Health Psychology, 19(2), 172–180. doi:10.1037/0278-6133.19.2.172
- Rousseau, D. M., Sitkin, S. B., Burt, R. S., & Camerer, C. (1998). Not so different after all: A cross-discipline view of trust. Academy of Management Review, 23(3), 393–404. doi:10.5465/amr.1998.926617
- Schulze, R., & Wittmann, W. W. (2003). A meta-analysis of the theory of reasoned action and the theory of planned behavior: The principle of compatibility and multidimensionality of beliefs as moderators. In R. Schulze, H. Holling, & D. Böhning (Eds.), Meta-analysis: New developments and applications in medical and social sciences (pp. 219–250). Boston, MA: Hogrefe & Huber Publishers.
- Shafiq, M., Elharake, J. A., Malik, A. A., McFadden, S. M., Anguolu, O. G., & Omer, S. (2021). COVID-19 sources of information, knowledge, and preventive behaviors among the US adult population. Journal of Public Health Management and Practice, 27(3), 278–284. doi:10.1097/PHH.0000000000001348
- Shapiro, G. K., Tatar, O., Dube, E., Amsel, R., Knauper, B. , and Rosberger, Z. (2018). The vaccine hesitancy scale: Psychometric properties and validation. Vaccine, 36(5), 660–667. doi:10.1016/j.vaccine.2017.12.043
- Sheeran, P., Orbell, S., & Trafimow, D. (1999). Does the temporal stability of behavioral intentions moderate intention-behavior and past behavior-future behavior relations? Personality & Social Psychology Bulletin, 25(6), 724–734. doi:10.1177/0146167299025006007
- Social Science Research Solutions (SSRS). (2020, June 27). https://ssrs.com/opinion-panel/
- Stata. 2017. 15.1 (Stata Corporation LLC).
- Stephenson, M. T., Benoit, W. L., & Tschida, D. A. (2009). Testing the mediating role of cognitive responses in the elaboration likelihood model. Communication Studies, 52(4), 324–337. doi:10.1080/10510970109388567
- Swami, V., & Barron, D. (2021). Rational thinking style, rejection of coronavirus (COVID-19) conspiracy theories/theorists, and compliance with mandated requirements: Direct and indirect relationships in a nationally representative sample of adults from the United Kingdom. Journal of Pacific Rim Psychology, 15, 183449092110373. doi:10.1177/18344909211037385
- Thoma, V., Weiss-Cohen, L., Filkuková, P., & Ayton, P. (2021). Cognitive predictors of precautionary behavior during the COVID-19 pandemic. Frontiers in Psychology, 12, 589800. doi:10.3389/fpsyg.2021.589800
- Todorov, A., Chaiken, S., & Henderson, M. D. (2002). The heuristic-systematic model of social information processing. In J. P. Dillard & M. Pfau (Eds.), The persuasion handbook: Developments in theory and practice (pp. 195–211). London: SAGE Publications.
- Woko, C., Siegel, L., & Hornik, R. (2020). An investigation of low COVID-19 vaccination intentions among Black Americans: The role of behavioral beliefs and trust in COVID-19 information sources. Journal of Health Communication, 25(10), 819–826. doi:10.1080/10810730.2020.1864521
- World Health Organization (WHO). (2021, April 9). Coronavirus Disease (COVID-19) advice for the public. https://www.who.int/emergencies/diseases/novel-coronavirus-2019/advice-for-public
- Xu, Y., Margolin, D., & Niederdeppe, J. (2021). Testing strategies to increase source credibility through strategic message design in the context of vaccination and vaccine hesitancy. Health Communication, 36(11), 1354–1367. doi:10.1080/10410236.2020.1751400