Abstract
Background: During the COVID-19 pandemic, telehealth became a widely used method of delivering treatment for substance use disorders (SUD), but its impact upon treatment engagement and dropout remains unknown. Methods: We conducted a retrospective analysis of adult SUD patients (n = 544) between October 2020 and June 2022 among a cohort of treatment-seeking patients at a nonprofit community behavioral health center in Southwestern Ohio. We estimated the likelihood of treatment dropout using survival curves and Cox proportional hazard models, comparing patients who used telehealth with video, telephone, or solely in-person services within the first 14 days of diagnosis. We also compared the likelihood of early treatment engagement. Results: Patients who received services through telehealth with video in the initial 14 days of diagnosis had a lower hazard of dropout, compared to patients receiving solely in-person services (0.64, 95% CI [0.46, 0.90]), while there was no difference in hazards of dropout between patients who received telephone and in-person services. Early use of telehealth, both via video (5.40, 95% CI [1.92, 15.20]) and telephone (2.12, 95% CI [1.05, 4.28]), was associated with greater odds of treatment engagement compared to in-person care. Conclusion: This study adds to the existing literature related to telehealth utilization and engagement in care and supports the inclusion of telehealth in SUD treatment programs for treatment-seeking individuals.
Introduction
Telehealth is defined as “the use of electronic information and telecommunication technologies to support long-distance clinical health care, patient and professional health-related education, health administration, and public health,” and includes provision of care either synchronously or asynchronously via a range of technologies including telephone, written communication, video conferencing, and store-and-forward media (Health Resources & Services Administration, Citation2021). Prior to the COVID-19 pandemic, telehealth had been gradually increasing in its popularity for the treatment of psychiatric disorders (O’Brien & McNicholas, Citation2020; Spivak et al., Citation2020). Compared to other areas of behavioral health, however, substance use disorder (SUD) treatment has been slower to adopt telehealth (Uscher-Pines et al., Citation2020). Substance use disorders are conditions in which an individual experiences cognitive, behavioral and physiological symptoms and significant impairment in functioning as a result of recurrent use of a substance (American Psychiatric Association, Citation2022) and numerous barriers to treatment exist for patients (Priester et al., Citation2016). The pandemic abruptly accelerated the adoption of telehealth for SUD treatment, enabling many patients to continue receiving life-saving care despite ongoing community spread of COVID-19.
Offering SUD treatment services via telehealth may improve access to care by the removal of traditional barriers to care, for example by allowing patients to receive treatment services if they lack transportation, childcare, time off work, or time needed to commute to an appointment. Video visits may offer some benefits over telephone visits, such as allowing broader assessment of a patient’s mental and physical state, but may also not be accessible to all individuals. To take advantage of telehealth with video for a visit, however, patients must have access to technology, such as a smartphone, WiFi, or data plan. While evidence suggests that many individuals with SUD may benefit from obtaining care through technology (Perri et al., Citation2021), disparities continue to exist (Campos-Castillo & Anthony, Citation2021; Chunara et al., Citation2021) and those who are older or homeless are more likely to experience barriers to accessing technology (Collins et al., Citation2016). Furthermore, there is evidence that the COVID-19 pandemic has amplified existing inequalities within society (Perry et al., Citation2021), and one way in which this has manifested is through the implementation of telehealth, further marginalizing patients who lack resources to access these services (Koonin et al., Citation2020; Lott et al., Citation2022).
SUD treatment has traditionally included a broad range of services, some of which (e.g. group therapy, urine drug screens) relied on in-person and facility-based care. The onset of the COVID-19 pandemic, however, posed increased risk to the millions of Americans with SUDs, who suddenly faced a potential disruption of care, particularly related to access to medications, and many providers shifted services to telehealth (Molfenter et al., Citation2021). An enforcement waiver of both federal and state telehealth rules during the COVID-19 public health emergency enabled the use of ongoing telehealth and services that were previously prohibited by law (e.g., first-dose prescribing of controlled substances without a face-to-face physical exam; psychotherapy by telephone) (Hare et al., Citation2020).
Prior to the pandemic, a systematic review from 2019 suggested that telehealth provides high levels of patient satisfaction, but due to limited data, the authors were unable to determine effectiveness of telehealth for SUD (Lin et al., Citation2019). A retrospective cohort study examined 28,791 Veterans diagnosed with opioid use disorder (OUD) and treated with buprenorphine across all facilities within the Veterans Health Administration between 2008 and 2017 (Vakkalanka et al., Citation2021). This study found a lower risk for treatment discontinuation in those who engaged in telehealth compared to in-person visits, but this is not generalizable to other SUDs. One large Canadian study found that patients treated via telehealth were more likely to remain in methadone treatment (Eibl et al., Citation2017; Lin et al., Citation2022), compared to those treated in-person, but other studies have failed to demonstrate superiority of telehealth (Mark et al., Citation2022; Uhl et al., Citation2022).
During the pandemic, OUD-related telehealth services among Medicare beneficiaries were associated with increased treatment retention for medication services and a lower likelihood of experiencing a medically treated overdose (Jones et al., Citation2022). A survey of treatment providers conducted by Molfenter and colleagues revealed that many clinicians preferred video services over telephone services, but perceived that telephone services were more accessible to patients. The authors suggested that both modes of delivery may play a role in providing treatment (Molfenter et al., Citation2021) and proposed that various delivery approaches should be a topic for future studies.
Unfortunately, there is currently not enough evidence related to the optimal implementation of telehealth for SUD treatment. Furthermore, among individuals who receive SUD treatment via telehealth, there is not enough data to determine whether one mode of care delivery is superior to another (telehealth with video or telephone-based services). Additionally, a gap in the literature exists regarding the engagement and retention of patients being treated for SUD following the implementation of telehealth. Although treatment engagement and retention remain a significant challenge in the field of addiction medicine, particularly as many patients have non-linear use trajectories (Fishman et al., Citation2020), existing literature suggests that higher levels of engagement and retention are associated with improved patient outcomes (Harris et al., Citation2010; Naeger et al., Citation2016). While telehealth has improved access prior to (Lin et al. Citation2019) and during the pandemic, and this alone may warrant its continued use even long after the resolution of the pandemic, there remains an urgent need to further investigate its impact on treatment engagement and retention for SUD.
Objectives
The primary aims of the study are to (1) characterize treatment-seeking patients who engage in various modes of treatment services for SUD treatment (telehealth with video, telephone, and in-person care) and (2) examine the association between telehealth mode (video or telephone) and time to dropout. Secondarily, we aimed to evaluate the impact of telehealth mode on early treatment engagement (i.e., completion of two treatment visits within 34 days of the treatment initiation visit).
Methods
Treatment setting
This retrospective cohort study was conducted using electronic health record (EHR) data on a cohort of treatment-seeking patients enrolled in a SUD treatment program provided by OneFifteen / Samaritan Behavioral Health, Inc., a nonprofit community behavioral health center in Southwestern Ohio. Services provided include outpatient care, intensive outpatient, crisis stabilization services, inpatient withdrawal management, and a residential treatment unit, though not all of these services were available throughout the entire duration of the study. Patients are referred to this treatment facility through a variety of sources, including social programs, criminal justice programs, community sources, medical providers and self-referrals.
We developed a technology platform offering real-time, synchronous audiovisual telehealth capabilities to address patient access to care, engagement, and outcomes. During the course of usual treatment, clinicians and patients had the option to deliver or receive SUD services either remotely by telephone or by telehealth with video, or to deliver or receive services in-person. Treatment programs were considered essential services and remained open for in-person care, while both telehealth with video and telephone-based services were also offered to promote access to care. Clinical workflows were updated to include an option for telehealth with video and telephone-based services among patients receiving outpatient, intensive outpatient, and ambulatory withdrawal management services.
The model of care was guided by the principle that some treatment services could be safely delivered remotely, while other services had to remain on site (including laboratory and medication injection services). The setting where this study was conducted promotes harm reduction and shared decision-making as evidence-based practice. While clinical team members offered patients access to care via telehealth with video and/or telephone services throughout the duration of treatment, patients and staff mutually selected the modality of care that best fit their practical and clinical needs. For example, medical services involving a new prescription of a controlled substance were required to occur either in-person or via telehealth with video due to regulatory restrictions, and were not permitted via telephone. Urine drug screens (UDS) were collected in-person but could be obtained asynchronously; for example, an individual could have a remote treatment encounter and present at a different date and time to provide a urine sample for UDS. While telehealth with video and telephone-based services were offered beginning in March 2020, this retrospective analysis includes data beginning in October 2020 because the telehealth system was upgraded at that time to provide reliable data identifying patients with specific telehealth encounters, and thus inclusion of dates before October 2020 was not possible due to gaps in the data.
Cohort sample and eligibility criteria
Patients were eligible for inclusion in the analysis if they were self-referred, 18 years or older at the time of admission into the treatment program, and completed a diagnostic evaluation between October 1, 2020 and June 1, 2022 which resulted in at least one International Classification of Diseases, Tenth Revision (ICD-10) diagnostic code for a SUD (F10-F19 prefixes) as a primary diagnosis. Individuals were defined as being self-referred if they sought treatment services independent of external program requirements; individuals who were required to receive an SUD assessment or complete a treatment program as a part of pretrial services, a court or probationary sentence, or an open Children’s Services case were not included in this cohort. The analysis was restricted to a treatment-seeking population to reduce heterogeneity within the sample and to observe the impact of telehealth among treatment-seeking individuals. Additionally, the cohort included patients who were in treatment for at least 14 days and completed at least one additional treatment visit after the initial diagnostic evaluation for an appointment type that could have been offered by either telehealth with video, telephone, or in-person; individuals were excluded if their only additional visit was to obtain a UDS, since a UDS can only occur in person.
Data were collected between October 1, 2020 and June 1, 2022. All patients were followed from their diagnostic evaluation until either treatment dropout (defined as the last kept appointment prior to a 60 day or more absence in follow-up appointments) or the end of the analysis period. To ensure our results would not be biased by those who had multiple episodes of care, only the data associated with each patient’s most recent episode of care were included in this analysis. The study used a retrospective cohort design, where groups were designated in a nesting strategy as shown in . We implemented a 14-day "run-in" period to define which group individuals were classified to in order to decrease the risk of reverse causality. Excluding UDS, all treatment visits (e.g., individual and group therapy, care coordination, peer support or medical services) were used to define the groups during the run-in period. The telehealth with video group was identified as having at least one remote video visit, which could be an individual appointment with a therapist, physician, nurse practitioner, or other clinical staff member, or a video-based group therapy session; patients of this group could also receive telephone and in-person visits. The telephone group was defined as having at least 1 visit that occurred by telephone; patients in this group could receive in-person but no telehealth with video visits. The in-person group was defined as having visits solely conducted in person. Completion of at least one visit was used to define the two telehealth groups because a single visit reflects an early signal that patients have the capacity and are comfortable using those technologies for care.
Figure 1. Modes of care: A nested model for group assignment. Note. Group assignment was made based on a nested model in the first 14 days of index date. The “in-person group” received their care solely in person. The “telephone group” received care by telephone and in-person. The “telehealth with video” group received at least one visit by telehealth with video and could also receive care by telephone and in-person.
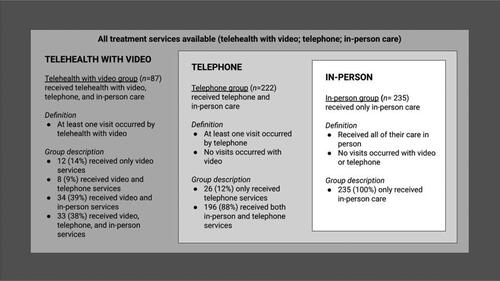
The study was determined to be exempt by the WCG IRB to be able to conduct this research retrospectively. All data used in this analysis had already been collected during standard patient care procedures.
Covariates
The main predictor of interest was use of telehealth-based services, defined as whether the patient had at least one remote telehealth with video or telephone appointment after the initial diagnostic evaluation during the 14-day run-in period. In order to remove confounding effects from personal characteristics on treatment dropout, several variables measuring demographics, socioeconomic status, substance use history and other clinical characteristics were adjusted as covariates in multivariable modeling. Covariate selection was performed based on available EHR data, findings reported in the scientific literature, and the authors’ clinical experience, leading to the identification of age, sex, race, highest education completed, housing status, employment status, primary SUD diagnosis, and presence of mental health comorbidities (defined as F2-F6 ICD-10 diagnoses at intake). Given racial distributions (), race was modeled as White or Nonwhite to support a more parsimonious model. Because severity could influence receipt of hybrid care, we also included indicators for whether the patient had received previous episodes of SUD treatment and whether medical services were received (defined as attending at least one treatment visit with a physician or nurse practitioner) during the 14-day run-in period, and binary variables for whether the Brief Addiction Monitor (BAM) use subscale score was ≥ 1, indicating recent substance use, and whether the risk subscale score was ≥12, indicating high risk factors for use (Cacciola et al., Citation2013). To account for the intensity of treatment services, the number of treatment visits completed during the run-in period was also included. We included all covariates in the final model regardless of baseline group differences ().
Table 1. Characteristics of patients.
Statistical analyses
All analyses had a p < .05 significance level unless otherwise specified and were performed using Python (v3.9.5). Survival analysis was conducted using the Python lifelines (v0.26.4) package. Chi-square and Kruskal-Wallis tests were used to evaluate differences in categorical and continuous variables, respectively, by group. When these tests indicated overall significance, follow-up pairwise comparisons were conducted to determine which differences between care groups were significant. For each variable with overall significance, the Bonferroni correction was applied in the pairwise comparisons to control for multiple comparisons.
Survival analysis
To examine the primary outcome of time to dropout, both visual and statistical methods were employed. Survival curves were assessed using the Kaplan-Meier method, with a log rank test of group differences. We implemented a 14-day “run-in” period to define what group individuals were classified to, and survival analysis was conducted from this date forward. Thus, time in treatment was defined as the number of days elapsed between the index date (14 days after the diagnostic evaluation) and treatment dropout. Patients were censored if they were still in treatment at the end of the analysis period. To analyze the influence of telehealth-based services and other potential predictors on time to drop out, multivariable Cox proportional hazard regression analysis was conducted. The proportionality of hazards assumption was investigated through Schoenfeld residuals. To minimize the risk of bias by excluding patients with missing data, covariates with missing data were imputed using the median value in the sample. The proportions of missing values ranged from 0.2 to 15% ().
Treatment engagement analysis
To supplement our primary analysis evaluating time to treatment dropout, a logistic regression model predicting early treatment engagement was developed, where initiating treatment (defined as at least 1 treatment visit within 14 days of the diagnostic evaluation) and completing at least two additional treatment visits within 34 days of the initiation date was the binary outcome variable. These definitions were used in accordance with the Healthcare Effectiveness Data and Information Set (HEDIS) definitions of treatment initiation and treatment engagement. All treatment visits (e.g., individual and group therapy, care coordination, peer support or medical services) counted toward the treatment engagement definition. Covariates were the same as those used in the Cox model.
Results
Description of the sample
presents descriptive characteristics of the sample (N = 544). Forty-five percent of patients were female. Most patients identified as White (79.1%), while 18.4% identified as Black or African American, 0.2% as Asian, 0.2% as American Indian or Alaska Native, and 1.3% as Other Race. Nearly half of patients reported opioid (49.1%) as their primary substance, followed by alcohol/sedative-hypnotics (28.9%), stimulants (15.3%) or cannabis (6.8%). Due to the small number of patients presenting with primary sedative-hypnotics (SH) use disorder (N = 6), we combined these patients with those with primary alcohol use disorder given physiological similarities. Five patients with a primary diagnosis of nicotine, inhalants, hallucinogens, or other psychoactive substance related-disorders were excluded from the analysis due to insufficient numbers for modeling.
The three groups (telehealth with video, telephone, and in-person) differed in various characteristics, including demographics, primary diagnoses, and substance use history. For example, in-person patients were more likely to be diagnosed with opioid use disorder, have higher BAM Use and Risk scores, and receive medical services more often compared to patients in the telehealth with video and telephone groups. Patients who utilized telehealth with video were more likely to be diagnosed with cannabis use disorder compared to the other groups, while patients in both the telephone and telehealth with video groups were more likely to be Nonwhite compared to the in-person group. While the distribution of group or individual therapy services did not differ between groups, in-person patients were less likely to receive care coordination services than the other two groups (see the Appendix).
The largest group comprised patients who received services in-person (43.2%), followed by telephone (40.8%) and telehealth with video (16%). Among those in the telephone group, 88% received both telephone and in-person services, while 12% only received telephone services. Among those in the telehealth with video group, 38% received a combination of telehealth with video, telephone, and in-person services, while 14% received only telehealth with video services, 9% received video and telephone services only, and another 39% received telehealth with video and in-person care but no telephone services ().
Kaplan-Meier estimates
illustrates unadjusted Kaplan-Meier estimates of treatment retention among the sample by group. Dropout rates at 30, 90, and 180 days for the telehealth with video group were 26, 55 and 69%, respectively, while dropout rates for the telephone group were 32, 57, and 72%, respectively, and 36, 61, and 73%, respectively, for the in-person group. The curves were not significantly different from one another according to the unadjusted log rank test.
Figure 2. Kaplan-Meier estimates of time to dropout by group. Note. Unadjusted Kaplan-Meier survival curves presented as rate of retention (no dropout) plotted against time until dropout in days. Blue line signifies patients who had at least one remote telehealth with video visit, green line signifies patients who used telephone but no telehealth with video, and the orange line signifies those who had solely in person visits within the first 14 days from the diagnostic evaluation. Dropout rates at 30, 90, and 180 days for the telehealth with video group were 26, 55 and 69%, respectively, while dropout rates for the telephone group were 32, 57, and 72%, respectively, and 36, 61, and 73%, respectively, for the in-person group.
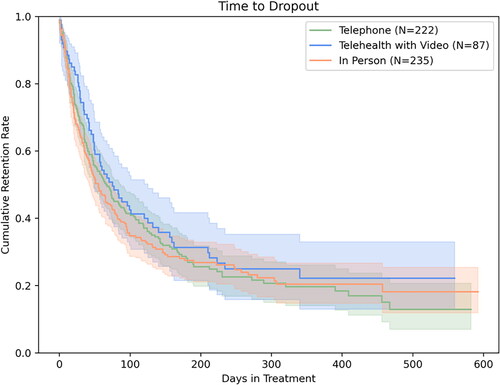
Cox regression analyses and hazard ratios
All significant predictors in the full Cox model satisfied the proportional hazard assumption according to the goodness-of-fit test. As shown in , patients who used telehealth with video in the first 14 days of care had a significantly lower hazard of dropout compared to those receiving solely in-person treatment (0.64, 95% CI [0.46, 0.90]). Hazards of dropout among patients who used telephone did not differ from patients receiving in-person services. Compared to older patients (i.e., age 50 or older), patients aged 18-30 (1.50, 95% CI [1.07, 2.10]) had a higher hazard of dropout. Being unemployed (1.37, 95% CI [1.11, 1.70]) was also associated with an increased hazard of dropout. Compared to patients with primary OUD, those with primary alcohol/SH, cannabis, or stimulants use disorder had higher hazards of dropout. Finally, receipt of medical services during the first 14 days of care had an independent protective effect on likelihood of dropout (0.63, 95% CI [0.45, 0.88)].
Table 2. Cox proportional hazards regression model of time to dropout.
Table 3. Factors associated with treatment engagement.
Factors associated with treatment engagement
In addition to evaluating the impact of telehealth and other factors on time to treatment dropout, we also assessed whether similar variables were associated with early treatment engagement (defined as initiating treatment and completing at least two treatment visits within 34 days of the initiation visit). Both telehealth with video (5.40, 95% CI [1.92, 15.20]) and telephone (2.12, 95% CI [1.05, 4.28]) were independently associated with greater odds of treatment engagement (). In addition, higher number of treatment visits within the first 14 days were also associated with increased odds of treatment engagement (3.50, 95% CI [2.74, 4.47]). Compared to patients with primary OUD, those with AUD had lower odds of treatment engagement (0.40, 95% CI [0.19, 0.88]).
Discussion
The COVID-19 pandemic had the potential to impact individuals with substance use disorders and their treatment disproportionately (Farhoudian et al., Citation2020; Radfar et al., Citation2021), thereby presenting clinicians with the opportunity to implement changes in the delivery of treatment. In this study, patients and staff were presented with options to deliver and receive care in person or remotely by telephone or by telehealth with video, or a combination of modalities. With the exception of a new prescription of a controlled substance, which required an in-person or video-based visit and could not be done by telephone, the choice of treatment mode was largely based on preferences of the patient and provider.
This study describes an evaluation of the impact of early (first 14 days) use of telehealth on treatment engagement and dropout within a sample of treatment-seeking individuals with SUD. When compared to patients who received in-person care, we observed a positive association between early use of telehealth (both via video and telephone) and treatment engagement, and a negative association between early use of telehealth with video and treatment dropout. While the findings of this study cannot determine causation, these data add to the existing literature related to telehealth utilization and engagement in care (Eibl et al., Citation2017; Lin et al., Citation2022; Vakkalanka et al., Citation2021) and support the inclusion of telehealth in SUD treatment programs. This is also the first study, to our knowledge, that observed a difference in outcomes between telehealth with video and telephone. Future studies are needed, however, to elucidate the relationship between optimal treatment delivery modes and outcomes, while attending to the needs of patients.
While engagement and retention in services comprise just a portion of an individual’s recovery, research suggests that longer duration in treatment can result in improved outcomes (NIDA, Citation2020). Telehealth is not the same as in-person treatment, as it provides care through a different experience for both providers and patients. However, the superiority of one or the other in SUD treatment delivery has not been definitively established or refuted (Mark et al., Citation2022; Uhl et al., Citation2022), and our study supports telehealth as another mode by which patients can access care, which has historically been inherently limited for many individuals with a substance use disorder (Madras et al., Citation2020).
The regulatory landscape of telehealth continuously updates as governing agencies weigh the return to pre-COVID telehealth-related practice requirements with the experience and lessons learned by practitioners who have used telehealth as a mode of care delivery over the past two years (Lin et al., Citation2022). Within our treatment environment, practitioners and patients shifted to collaborative decision-making to determine whether to use telehealth for delivery of care—an approach that potentially requires a paradigm shift in thinking. Existing studies have suggested that providers may appreciate the benefits of telehealth, including alleviation of transportation or childcare barriers, reduction in anxiety and the opportunity for an improved emotional connection (Uscher-Pines et al., Citation2020). While telehealth may reduce barriers for some, it may not be an option for everyone who presents for SUD treatment. For example, the patients receiving in-person services in our study were more likely to be homeless, unemployed, and have higher BAM scores, but we do not have information about why they chose to receive services in person, such as whether it was due to lack of access to technology, poor digital literacy or preference. While the use of telehealth may improve access to SUD treatment at the population level and reduce barriers to treatment, it risks further marginalization of those in need by exacerbating the digital divide (Early & Hernandez, Citation2021; Saeed & Masters, Citation2021). Providers who offer telehealth to patients must therefore be aware of the systemic, social, and economic factors that impact every individual’s access to technology.
Telehealth readiness should be assessed at the onset of, and continuously throughout treatment. In this study, we used a 14-day run-in period to define which group individuals were classified to, but patients were not randomized or required to continue using the same mode of treatment that they used in the first 14 days of treatment. The majority of patients in the telehealth with video group used a combination of modalities to receive treatment; only 14% received solely telehealth with video services. Similarly, the majority of patients in the telephone group received care in-person and by telephone. We propose that offering telehealth-based services at the beginning of individuals’ treatment may help patients with access to telehealth engage in the therapeutic relationship and contribute to improved outcomes.
Limitations
While patients have differing levels of comfort and familiarity with technology, staff members also have varying degrees of knowledge and experience in using telehealth platforms; this should be considered when interpreting these results. The setting where this study was conducted promotes shared decision-making as evidence-based practice and patients and staff mutually selected the modality of treatment that best fit their needs. If conflict arose, patients and staff typically worked together to find a resolution. However, patients may have been encouraged to utilize telehealth options by their providers at varying rates, particularly as COVID-19 transmission in the community varied. Furthermore, patients within this study sample did not have an equal opportunity to participate in telehealth due to a variety of reasons, including unequal access, severity of SUD, logistical/practical needs, provider preferences, or the type of therapy services offered to the patient (see the Appendix). It is possible that the patients within the telehealth with video group had favorable outcomes because they had the greatest number of treatment delivery options available to them, suggesting that efforts to reduce the digital divide should be continued.
As a retrospective study, selection bias must be considered, as the decision to use a particular modality may represent underlying differences in access or SUD acuity. We also make the assumption that all patients are given the opportunity to participate in telehealth-based services, but we are unable to confirm this. Existing literature suggests that compared to in-person visits, the use of telehealth is associated with positive provider satisfaction (Volcy et al., Citation2021) and improves engagement between health care providers and patients (Langabeer et al., Citation2021). However, because telehealth was presented to staff as an option, and not a requirement, to deliver care when patients were located offsite, we propose that a driver of the large number of patients in the telephone group (compared to the video group) may be staff preference, as telephone-based care for non-medical services is familiar and did not require use of novel technology.
Our study focuses on treatment-seeking individuals, thus findings may not apply to populations referred from other sources (e.g., court-mandated referrals). Generalizability of our findings may also be limited by the lack of variability in race/ethnicity within this sample, and by data collection from a single treatment program in one location using a proprietary telehealth platform. In addition, the data was collected retrospectively, which is dependent on accurate documentation and coding in the medical record. Unmeasured confounding is also possible; for example, we did not include the severity of SUD or non-mental health comorbidities into the model due to incomplete data. Although it does not equate to severity, we did include the BAM use and risk subscales to serve as a proxy for severity, as this overlaps with several DSM-5 criteria for substance use disorders. We also included receipt of medical services as another proxy for severity, as patients who require medical services often have more severe symptoms. Furthermore, our study was exploratory and observational in nature and our findings cannot determine causality. While our results suggest that early engagement in treatment through telehealth with video may reduce the risk of treatment dropout, more research is needed to understand the impact of telehealth in SUD treatment, from a variety of geographic locations. Future studies should also consider testing interactions to assess the potentially differential impacts of telehealth among different subgroups (e.g., primary SUD, race, gender).
Conclusion
The COVID-19 pandemic has catalyzed the transition to telehealth for many SUD treatment organizations, and the benefit of improved engagement and decreased risk of dropout from early use of telehealth options may prove to be just one reason for its continued use. As the pandemic’s negative impact upon substance use continues (Czeisler et al., Citation2021), the use of telehealth should be considered beyond this current public health crisis. Despite the ongoing pandemic, many states have begun to roll back the telehealth flexibilities that were instated early in 2020, and the return to pre-pandemic telehealth rules may jeopardize continued access to treatment for some individuals with SUD (Armour & Whelan, Citation2021) at a time when unintentional overdose deaths have never been higher (Ahmad et al., Citation2021). State and federal policies related to telehealth, specifically to the Ryan Haight Online Pharmacy Consumer Protection Act of 2008, must be updated, particularly as mandatory in-person requirements create undue challenges and barriers to individuals who would benefit from SUD treatment. Telehealth readiness should be assessed at the onset of treatment and the fidelity of telehealth treatment must be continually assessed. SUD treatment providers should be encouraged by these results as they consider adopting flexible care models that offer telehealth and other changes to traditional care, such as the allowance of asynchronous drug testing and virtual group therapy. Lastly, future studies should include a further investigation of the outcomes, barriers and facilitators associated with longitudinal telehealth use among both patients and providers, and across different subgroups of patients.
Declaration of interest
Financial support for this research was provided by Verily Life Sciences. Employees of Verily Life Sciences have participated in the conception, analysis, and dissemination of results from this study, and collaborated on writing/editing the article with employees of OneFifteen. Verily Life Sciences developed and owns the technology platform examined in this study.
Additional information
Funding
References
- Ahmad, F. B., Rossen, L. M., & Sutton, P. (2021). Provisional drug overdose death counts. National Center for Health Statistics. Retrieved January 4, 2022, from https://www.cdc.gov/nchs/nvss/vsrr/drug-overdose-data.htm.
- American Psychiatric Association. (2022). Diagnostic and statistical manual of mental disorders (5th ed., Text rev.). American Psychiatric Association.
- Armour, S., & Whelan, R. (2021, November 22). Telehealth rollbacks leave patients stranded, some doctors say. Wall Street Journal. https://www.wsj.com/articles/telehealth-rollbacks-leave-patients-stranded-some-doctors-say-11637577001
- Cacciola, J. S., Alterman, A. I., Dephilippis, D., Drapkin, M. L., Valadez, C., Jr., Fala, N. C., Oslin, D., & McKay, J. R. (2013). Development and initial evaluation of the Brief Addiction Monitor (BAM). Journal of Substance Abuse Treatment, 44(3), 256–263. https://doi.org/10.1016/j.jsat.2012.07.013
- Campos-Castillo, C., & Anthony, D. (2021). Racial and ethnic differences in self-reported telehealth use during the COVID-19 pandemic: A secondary analysis of a US survey of internet users from late March. Journal of the American Medical Informatics Association: JAMIA, 28(1), 119–125. https://doi.org/10.1093/jamia/ocaa221
- Chunara, R., Zhao, Y., Chen, J., Lawrence, K., Testa, P. A., Nov, O., & Mann, D. M. (2021). Telemedicine and healthcare disparities: A cohort study in a large healthcare system in New York City during COVID-19. Journal of the American Medical Informatics Association, 28(1), 33–41. https://doi.org/10.1093/jamia/ocaa217
- Collins, K. M., Armenta, R. F., Cuevas-Mota, J., Liu, L., Strathdee, S. A., & Garfein, R. S. (2016). Factors associated with patterns of mobile technology use among persons who inject drugs. Substance Abuse, 37(4), 606–612. https://doi.org/10.1080/08897077.2016.1176980
- Czeisler, M. É., Lane, R. I., Wiley, J. F., Czeisler, C. A., Howard, M. E., & Rajaratnam, S. (2021). Follow-up survey of US adult reports of mental health, substance use, and suicidal ideation during the COVID-19 pandemic, September 2020. JAMA Network Open, 4(2), e2037665. https://doi.org/10.1001/jamanetworkopen.2020.37665
- Early, J., & Hernandez, A. (2021). Digital disenfranchisement and COVID-19: Broadband internet access as a social determinant of health. Health Promotion Practice, 22(5), 605–610. https://doi.org/10.1177/15248399211014490
- Eibl, J. K., Gauthier, G., Pellegrini, D., Daiter, J., Varenbut, M., Hogenbirk, J. C., & Marsh, D. C. (2017). The effectiveness of telemedicine-delivered opioid agonist therapy in a supervised clinical setting. Drug and Alcohol Dependence, 176, 133–138. https://doi.org/10.1016/j.drugalcdep.2017.01.048
- Farhoudian, A., Baldacchino, A., Clark, N., Gerra, G., Ekhtiari, H., Dom, G., Mokri, A., Sadeghi, M., Nematollahi, P., Demasi, M., Schütz, C. G., Hash-Emian, S. M., Tabarsi, P., Galea-Singer, S., Carrà, G., Clausen, T., Kouimtsidis, C., Tolomeo, S., Radfar, S. R., & Razaghi, E. M. (2020). COVID-19 and substance SSE disorders: Recommendations to a comprehensive healthcare response. An international society of addiction medicine practice and policy interest group position paper. Basic and Clinical Neuroscience, 11(2), 133–150. https://doi.org/10.32598/bcn.11.covid19.1
- Fishman, M., Vo, H. T., Burgower, R., Ruggiero, M., Rotrosen, J., Lee, J., & Nunes, E. (2020). Treatment trajectories during and after a medication trial for opioid use disorder: Moving from research as usual to treatment as usual. Journal of Addiction Medicine, 14(4), 331–336. https://doi.org/10.1097/ADM.0000000000000592
- Hare, N., Bansal, P., Bajowala, S. S., Abramson, S. L., Chervinskiy, S., Corriel, R., Hauswirth, D. W., Kakumanu, S., Mehta, R., Rashid, Q., Rupp, M. R., Shih, J., & Mosnaim, G. S. (2020). Work group report: COVID-19: Unmasking telemedicine. The Journal of Allergy and Clinical Immunology. In Practice, 8(8), 2461–2473.e3. https://doi.org/10.1016/j.jaip.2020.06.038
- Harris, A. H., Humphreys, K., Bowe, T., Tiet, Q., & Finney, J. W. (2010). Does meeting the HEDIS substance abuse treatment engagement criterion predict patient outcomes? The Journal of Behavioral Health Services & Research, 37(1), 25–39. https://doi.org/10.1007/s11414-008-9142-2
- Health Resources & Services Administration. (2021, December 14). What is telehealth? https://www.hrsa.gov/rural-health/telehealth/what-is-telehealth
- Jones, C. M., Shoff, C., Hodges, K., Blanco, C., Losby, J. L., Ling, S. M., & Compton, W. M. (2022). Receipt of telehealth services, receipt and retention of medications for opioid use disorder, and medically treated overdose among medicare beneficiaries before and during the COVID-19 pandemic. JAMA Psychiatry, 79(10), 981. https://doi.org/10.1001/jamapsychiatry.2022.2284
- Koonin, L., Hoots, B., Tsang, C., Leroy, Z., Farris, K., Jolly, T., Antall, P., McCabe, B., Zels, C., Tong, I., & Harris, A. (2020). Trends in the use of telehealth during the emergence of the COVID-19 pandemic—United States, January–March 2020. MMWR - Morbidity and Mortality Weekly Report, 2020(69), 1595–1599. https://doi.org/10.15585/mmwr.mm6943a3
- Langabeer, J. R., II, Yatsco, A., & Champagne-Langabeer, T. (2021). Telehealth sustains patient engagement in OUD treatment during COVID-19. Journal of Substance Abuse Treatment, 122, 108215. https://doi.org/10.1016/j.jsat.2020.108215
- Lin, L. A., Casteel, D., Shigekawa, E., Weyrich, M. S., Roby, D. H., & McMenamin, S. B. (2019). Telemedicine-delivered treatment interventions for substance use disorders: A systematic review. Journal of Substance Abuse Treatment, 101, 38–49. https://doi.org/10.1016/j.jsat.2019.03.007
- Lin, L. A., Zhang, L., Kim, H. M., & Frost, M. C. (2022). Impact of COVID-19 telehealth policy changes on buprenorphine treatment for opioid use disorder. American Journal of Psychiatry, 179(10), 740–747. https://doi.org/10.1176/appi.ajp.21111141
- Lott, A., Campbell, K. A., Hutzler, L., & Lajam, C. M. (2022). Telemedicine utilization at an academic medical center during COVID-19 pandemic: Are some patients being left behind? Telemedicine Journal and e-Health, 28(1), 44–50. https://doi.org/10.1089/tmj.2020.0561
- Madras, B. K., Ahmad, N. J., Wen, J., & Sharfstein, J., The Prevention, Treatment, and Recovery Working Group of the Action Collaborative on Countering the U.S. Opioid Epidemic. (2020). Improving access to evidence-based medical treatment for opioid use disorder: Strategies to address key barriers within the treatment system. NAM Perspectives. Discussion Paper. https://doi.org/10.31478/202004b
- Mark, T. L., Treiman, K., Padwa, H., Henretty, K., Tzeng, J., & Gilbert, M. (2022). Addiction treatment and telehealth: Review of efficacy and provider insights during the COVID-19 pandemic. Psychiatric Services, 73(5), 484–491. https://doi.org/10.1176/appi.ps.202100088
- Molfenter, T., Roget, N., Chaple, M., Behlman, S., Cody, O., Hartzler, B., Johnson, E., Nichols, M., Stilen, P., & Becker, S. (2021). Use of telehealth in substance use disorder services during and after COVID-19: Online survey study. JMIR Mental Health, 8(2), e25835. https://doi.org/10.2196/25835
- Naeger, S., Mutter, R., Ali, M. M., Mark, T., & Hughey, L. (2016). Post-discharge treatment engagement among patients with an opioid-use disorder. Journal of Substance Abuse Treatment, 69, 64–71. https://doi.org/10.1016/j.jsat.2016.07.004
- National Committee for Quality Assurance. (2022, January 4). Initiation and engagement of alcohol and other drug abuse or dependence treatment (IET). https://www.ncqa.org/hedis/measures/initiation-and-engagement-of-alcohol-and-other-drug-abuse-or-dependence-treatment/
- National Institute on Drug Abuse (NIDA). (2020). Principles of effective treatment. https://nida.nih.gov/publications/principles-drug-addiction-treatment-research-based-guide-third-edition/principles-effective-treatment
- O’Brien, M., & McNicholas, F. (2020). The use of telepsychiatry during COVID-19 and beyond. Irish Journal of Psychological Medicine, 37(4), 250–255. https://doi.org/10.1017/ipm.2020.54
- Perri, M., Guta, A., Gagnon, M., Bonn, M., Leece, P., Bayoumi, A. M., Rai, N., Touesnard, N., & Strike, C. (2021). Developing a digital health strategy for people who use drugs: Lessons from COVID-19. Digital Health, 7, 20552076211028404. https://doi.org/10.1177/20552076211028404
- Perry, B. L., Aronson, B., & Pescosolido, B. A. (2021). Pandemic precarity: COVID-19 is exposing and exacerbating inequalities in the American heartland. Proceedings of the National Academy of Sciences, 118(8), e2020685118. https://doi.org/10.1073/pnas.2020685118
- Priester, M. A., Browne, T., Iachini, A., Clone, S., DeHart, D., & Seay, K. D. (2016). Treatment access barriers and disparities among individuals with co-occurring mental health and substance use disorders: An integrative literature review. Journal of Substance Abuse Treatment, 61, 47–59. https://doi.org/10.1016/j.jsat.2015.09.006
- Radfar, S. R., De Jong, C., Farhoudian, A., Ebrahimi, M., Rafei, P., Vahidi, M., Yunesian, M., Kouimtsidis, C., Arunogiri, S., Massah, O., Deylamizadeh, A., Brady, K. T., Busse, A., Potenza, M. N., Ekhtiari, H., & BaldAcchino, A. M., ISAM-PPIG Global Survey Consortium. (2021). Reorganization of substance use treatment and harm reduction services during the COVID-19 pandemic: A global survey. Frontiers in Psychiatry, 12, 639393. https://doi.org/10.3389/fpsyt.2021.639393
- Saeed, S. A., & Masters, R. M. (2021). Disparities in health care and the digital divide. Current Psychiatry Reports, 23(9), 61. https://doi.org/10.1007/s11920-021-01274-4
- Spivak, S., Spivak, A., Cullen, B., Meuchel, J., Johnston, D., Chernow, R., Green, C., & Mojtabai, R. (2020). Telepsychiatry use in U.S. mental health facilities, 2010-2017. Psychiatric Services (Washington, D.C.), 71(2), 121–127. https://doi.org/10.1176/appi.ps.201900261
- Uhl, S., Bloschichak, A., Moran, A., McShea, K., Nunemaker, M. S., McKay, J. R., & D’Anci, K. E. (2022). Telehealth for substance use disorders: A rapid review for the 2021 U.S. Department of Veterans Affairs and U.S. Department of Defense guidelines for management of substance use disorders. Annals of Internal Medicine, 175(5), 691–700. https://doi.org/10.7326/M21-3931
- Uscher-Pines, L., Cantor, J., Huskamp, H. A., Mehrotra, A., Busch, A., & Barnett, M. (2020). Adoption of telemedicine services by substance abuse treatment facilities in the U.S. Journal of Substance Abuse Treatment, 117, 108060. https://doi.org/10.1016/j.jsat.2020.108060
- Vakkalanka, J. P., Lund, B. C., Ward, M. M., Arndt, S., Field, R. W., Charlton, M., & Carnahan, R. M. (2021). Telehealth utilization is associated with lower risk of discontinuation of buprenorphine: A retrospective cohort study of US veterans. Journal of General Internal Medicine, 37(7), 1610–1618. https://doi.org/10.1007/s11606-021-06969-1
- Volcy, J., Smith, W., Mills, K., Peterson, A., Kene-Ewulu, I., McNair, M., Kelsey, R., & Mbaezue, N. (2021). Assessment of patient and provider satisfaction with the change to telehealth from in-person visits at an academic safety net institution during the COVID-19 pandemic. Journal of the American Board of Family Medicine: JABFM, 34(Suppl), S71–S76. https://doi.org/10.3122/jabfm.2021.S1.200393
Appendix
Table A1. Distribution of appointment types during the 14-day run-in period by group.