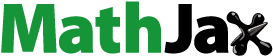
ABSTRACT
Commonly used cut-points may misclassify physical activity (PA) in people with cystic fibrosis (CF). The aim of this study was to develop and cross-validate condition-specific cut-points in children and adolescents with CF. Thirty-five children and adolescents with CF (15 girls; 11.6 ± 2.8 years) and 28 controls (16 girls; 12.2 ± 2.7 years), had their energy expenditure and triaxial acceleration measured during six daily activities of varying intensities. Euclidean Norm Minus One (ENMO) and Mean Amplitude Deviation (MAD) were extracted using both GENEActiv (both wrists) and ActiGraph GT9X (both wrists and right waist) accelerometers. ROC curves were used to determine healthy and CF-specific raw acceleration cut-points for sedentary time (SED), moderate physical activity (MPA) and vigorous physical activity (VPA). The PA cut-points were generally lower in CF compared to controls for both ENMO (60.2–73.1 vs. 63.5–86.8 mg) and MAD (58.9–85.2 vs. 75.9–93.7 mg). These substantial inter-cut-point differences support the need for disease-specific cut-points.
KEYWORDS:
Introduction
Physical activity (PA) is associated with many benefits in those with cystic fibrosis (CF), such as the reduction of exacerbations and the improvement of both life expectancy and quality of life (Hebestreit et al., Citation2014). Accelerometers measure velocity over time, which can subsequently be translated into PA intensities by using prediction equations, cut-points, and, more recently, machine learning models (Arvidsson et al., Citation2019). However, cut-points or prediction equations that were developed for the general population may lead to inaccurate PA estimations when applied to those with a chronic condition such as CF (Mackintosh et al., Citation2018). For example, non-disease specific moderate-to-vigorous PA (MVPA) cut-points were found to underestimate PA in youth with CF (Stephens et al., Citation2016). Such discrepancies may be related to the pathophysiology of the disease, as children and adolescents with CF have a higher resting metabolic rate (RMR) and energy expenditure (EE) for a given task compared to healthy peers (Moudiou et al., Citation2007). Consequently, the cut-points used to assess PA in children with CF may need to be tailored to estimate relative PA levels.
To develop CF-specific cut-points, accelerometers need to be calibrated using a protocol comprising a range of activities that span the intensity spectrum and are representative of daily life (Welk, Citation2005). In healthy populations, calibration studies have typically utilized either laboratory-based or free-living protocols (Welk, Citation2005). Whilst highly structured activities, such as walking or running on a treadmill, included in laboratory-based protocols are generally associated with superior predictive accuracy, they lack ecological validity (Welk, Citation2005). Consequently, free-living protocols are widely recommended to generate cut-points reflecting the unique, sporadic nature of children’s PA patterns (Mackintosh et al., Citation2012). However, despite the associated advantages, a free-living protocol precludes the measurement of a biological reference criterion, such as EE, which is pivotal when calibrating accelerometry, especially in clinical populations (Mackintosh et al., Citation2012). Indeed, a recent systematic review found that while the type of protocol greatly impacts PA classification, studies calibrating accelerometry in pediatric clinical cohorts should account for the pathophysiology of the disease and integrate EE measurements in the protocol (Bianchim et al., Citation2020).
Earlier studies developing cut-points in healthy children have utilized waist-worn accelerometers due to the proximity of this location to the body’s center of gravity (Welk, Citation2005). Although the waist is known to provide accurate estimations of whole-body movement, this placement is associated with poor compliance, which can lead to misclassification of PA intensities and bias (Fairclough et al., Citation2016). Similarly, there is no consensus regarding the optimal accelerometer brand to derive PA levels in children, though recent studies have relied on brands that provide raw acceleration data, such as the GENEActiv and ActiGraph (GT3X+ and GT9X; Hildebrand et al., Citation2014; Hurter et al., Citation2018).
The aim of this study was to develop disease-specific cut-points for children and adolescents with CF using different accelerometer placements (wrist and waist) and brands (GENEActiv and ActiGraph), in comparison to a healthy comparative group. Different accelerometer brands and placements were compared.
Materials and methods
Participants
Thirty-five children and adolescents with CF (15 girls) and 28 healthy controls (16 girls), aged 7–17 years, participated in the study. Participants with CF were recruited from pediatric CF clinics in South Wales and had been diagnosed as having CF according to a newborn screening test, and/or presenting with CF-typical symptoms and either two pathological sweat tests or the identification of two CF-relevant mutations. Those with multi-resistant bacteria, an acute exacerbation at the time of the assessments, co-morbidities such as cardiovascular or musculoskeletal issues that compromise exercise performance, or who were less than two weeks post antibiotic treatment for an exacerbation or awaiting a transplant, were excluded from the study. Healthy participants were recruited through a university in Wales and from the friends and families of the CF participants. The health status of the healthy control group was confirmed by a short clinical anamnesis. Written informed consent was obtained from parents/guardians and assent from the participants prior to the study commencement. Ethics approval was obtained from the National Health Service (NHS) Research Ethics Committee (18/WS/0032).
Protocol
Participants were asked to attend the laboratory on three occasions, with the first two visits separated by seven days. The first visit involved baseline measures of anthropometry, RMR, and lung function. The second and third visits consisted of the daily-life activity protocol and a treadmill-based exercise test, respectively. Participants were asked to arrive at least two hours postprandial and to have avoided caffeine and vigorous exercise for 24-hours. For participants with CF, information regarding medication, any associated comorbidities, and the frequency of exacerbations was extracted from their medical records.
Measurements
Anthropometry
Body mass, stature and sitting height were measured to the nearest. 1 kg, .1 cm and .1 cm, respectively. Body mass index (BMI) and age- and sex-specific z-scores were determined according to the World Health Organization reference data (De Onis et al., Citation2004). Pubertal stage was estimated according to time pre- or post-peak height velocity (PHV; Mirwald et al., Citation2002), with pre- considered >-1 years from PHV, circa- as −1 to +1 years and post-PHV as >+1 years post PHV.
Resting metabolic rate
The assessment of RMR was performed for 20 minutes via indirect calorimetry through a facemask and calibrated metabolic cart following at least 10 minutes at rest on supine (MetaMax Cortex 3B, CORTEX Biophysik GmbH, Germany). This measure was performed in a quiet room and all participants were instructed to remain supine and to avoid talking and/or sleeping. To calculate RMR, the first five minutes and the last two and a half minutes were removed. Subsequently, RMR was calculated according to the Weir equation (Weir, Citation1949).
Aerobic capacity
A standard Bruce protocol was used to assess peak oxygen uptake (O2peak). Gas exchange variables were measured on a breath-by-breath basis (Metamax 3B, Cortex Biophysik GmbH, Germany). Oxygen saturation and heart rate were measured throughout the exercise protocol using a pulse oximeter (Nonin® WristOx® Model 3150, Nonin® Medical Inc., USA) and a three-lead electrocardiogram (ECG; Custo Guard ECG, custo med GmbH, Germany), respectively. Peak oxygen uptake was defined as the highest 10-s moving average during the exercise test.
Lung function
All participants were asked to complete a spirometry assessment at the start of the first session using a forced vital capacity maneuver to determine forced expiratory volume in one second (FEV1) in accordance with the European Respiratory Society standards (Moore, Citation2012). The FEV1%predicted was estimated using a reference equation (Quanjer et al., Citation2012) for age, sex and height, and used to categorize disease severity in those with CF as mild (>70%), moderate (40–69%) or severe (<40%; Davies et al., Citation2009).
Accelerometry
The ActiGraph GT9X Link (ActiGraph, Pensacola, FL) and GENEActiv (ActivInsights Ltd., Cambridge, UK) were used to measure raw acceleration at 100 Hz. Both accelerometers have a dynamic range of approximately 8 g. ActiGraph GT9X Link monitors were placed on each wrist and the right waist with GENEActiv monitors placed on each wrist. The wrist monitors were placed beside one another in a randomized order across participants. The ActiGraph low frequency extension was activated.
Daily-life calibration protocol
The daily-life calibration protocol consisted of activities mimicking the participants’ daily lives. During their first laboratory visit, participants were given a spreadsheet of common activities from the compendium of physical activities (Ainsworth et al., Citation2011) and asked to select any that they would typically do at least once a day. Suggestions of additional activities were also integrated, with the six most commonly selected activities, stratified by behavior type (i.e., sedentary), chosen to be integrated into the daily-life protocol (). Over 50 minutes, participants performed the same six activities for three to ten minutes each, in a randomized order, interspersed by three minutes rest, whilst wearing the accelerometers, metabolic system and pulse oximeter.
Table 1. Activities included in the daily-life protocol.
Data reduction
The raw acceleration data were extracted as .gt3x files and .bin files using ActiLife V 6.10.2 and GENEActiv PC software V2.2, respectively. All .gt3x files were converted to time-stamp free .csv files and subsequently imported along with the .bin files into R statistical software (V3.1.2; R Foundation for Statistical Computing, Vienna, Austria) for the extraction of raw acceleration data. Specifically, the GGIR package (V1.2–0; Migueles, Rowlands et al., Citation2019) was used to auto-calibrate and extract the Euclidean Norm Minus One (ENMO) and Mean Amplitude Deviation (MAD) metrics in 5-s epochs (Migueles, Rowlands et al., Citation2019; Van Hees et al., Citation2014).
Metabolic equivalent of task (MET) values for each activity were calculated by dividing the O2 (ml·min−1·kg−1) for each activity by the measured resting
O2. Data from the first and last minute of each activity were excluded to avoid transitional movements. Subsequently, MET values were aligned with the acceleration metrics for each activity. MET values were then used to code the corresponding 5-s raw accelerometer data as sedentary (≤1.5 METs), moderate (4.0–6.9 METs) or vigorous (≥7.0 METs; Troiano et al., Citation2008).
Statistical analyses
Descriptive analyses were performed using SPSS Statistics, version 23.0 (IBM Corp., USA) with data presented as mean ± standard deviation (SD) or frequencies for continuous and categorical variables, respectively. Participants with missing data were not included in the analysis. A two-way ANOVA and Kruskal-Wallis test were utilized for parametric and non-parametric data, respectively, to investigate inter-group comparisons of participant demographics, accelerometer outputs and EE data. A three-factorial, repeated-measures ANOVA test with Bonferroni correction was used to investigate the effect of activity type, accelerometer placement and accelerometer brand, and their interaction according to disease status.
Disease-specific cut-points for SED, MPA and VPA were generated using Receiver operating characteristic (ROC) with its respective area under the curve (AUC) in MedCalc (version 19.2.1; Ostend, Belgium). The ROC analyses were interpreted according to the sensitivity (the number of true positives) and specificity (the number of false positives). For the ROC-AUC, values of ≥.90 were considered excellent, .80–.90 good, .70–.80 fair, and <.70 poor. The code generated from the MET values, for each intensity, was used as the dependent variable for the ROC curve, with the cut-points generated selected to optimize both sensitivity and specificity. An iterative leave-one-out approach was conducted in R to cross-validate the cut-points. Bland-Altman plots were used to assess the mean bias and limits of agreement between monitors for each placement in both groups (Bland & Altman, Citation1986). Significance was accepted at p ≤ .05.
Results
From the initial 64 participants that were screened, one participant was unable to attend the second visit and was therefore excluded from further analyses. In total, the study included 63 children, 35 with CF and 28 healthy controls (). Most CF participants were homozygous (55%) for the ΔF508 mutation. Fifty-five children were right-handed (30 CF) and 8 were left-handed (5 CF). The two-way ANOVA revealed that those with CF had significantly lower body mass (p = .02) and zBMI (p = .006) than the healthy participants, but there were no significant differences in age, stature, RMR, lung function or O2peak.
Table 2. Participant characteristics.
During walking at a comfortable pace, the CF group had significantly higher accelerometer outputs in comparison to the healthy group for both ENMO (p = .04) and MAD (p = .05), but there were no differences in METs for any activity (). present the cut-points derived from ENMO and MAD, respectively. The cross-validation data for each cut-point are presented as Supplementary Table 1.
Table 3. Mean energy expenditure (METs) and ENMO and MAD (mg) during each activity within the daily-life protocol.
Table 4. Cut-points derived from ENMO raw accelerometer data in mg.
Table 5. Cut-points derived from MAD raw accelerometer data in mg.
The factorial ANOVA showed a significant effect of activity type on both ENMO and MAD (F2.58,82.74 = 64.30, F3.24,103.77 = 161.96, respectively, both p < .0001) in those with CF. Whilst no significant main effect was observed for placement (F1,32 = .30, p = .58), there was a significant effect of brand for MAD metrics in both CF (F1,32 = 6.17, p < .05) and healthy participants (F1,32 = 6.17, p < .05), with significantly higher outputs from GENEActiv. Significant two-way interactions were observed for activity*brand (F2.53,81.18 = 5.47, p < .0001) and brand*placement (F1,32 = 4.35, p < .05) for ENMO. Specifically, the ENMO output from the wrist-worn GENEActiv was higher in comparison with the waist-worn ActiGraph GT9X, particularly during vigorous activities. Furthermore, there was a significant interaction between activity*placement for both ENMO and MAD (F3.04,97.41 = 11.51, F1,120 = 7.13, respectively, both p < .05), with significantly lower outputs for waist-worn monitors during intense activities.
A significant three-way interaction of activity*placement*accelerometer brand was observed for ENMO (F2.49,79.72 = 15.27, p < .0001). Post hoc analyses showed a significant effect of brand during stairs and coloring, with GENEActiv producing higher outputs than the ActiGraph GT9X for both ENMO and MAD (F1,32 = 14.51, F1,32 = 5.96, respectively, both p < .05). Finally, wrist- and waist-worn monitors showed a significant effect for playing on a handheld device (F5,28 = 31.66; F5,28 = 98.24, p < .0001), free-games (F5,28 = 98.24; F5,28 = 43.30, p < .0001) and stairs (F5,28 = 52.99; F5,28 = 75.23, p < .0001) for ENMO and MAD, respectively. Specifically, ActiGraph GT9X yielded lower outputs when placed at the waist, whilst GENEActiv produced higher outputs when placed on the dominant wrist, particularly during these three activities.
The Bland-Altman plots (Supplementary Figure 1) indicated high agreement between the dominant wrist and waist for MAD in the CF group. In the healthy children, ENMO from the non-dominant wrist and waist displayed the best agreement. A visual inspection of the Bland-Altman plots showed heteroscedasticity for ENMO and MAD metrics, irrespective of placement and condition, and for most physical activity intensities. A negative correlation was found between the average ENMO values and the difference in values for waist-worn devices (−.24, p = .003) in the CF group. Moreover, a negative correlation was found between the average raw acceleration values and the difference in values for both ENMO and MAD from the dominant wrist in the healthy group (−.42, −.45, respectively, both p = .0001).
Discussion
This original study developed raw acceleration SED, MPA and VPA cut-points from ActiGraph GT9X and GENEActiv accelerometers placed at the waist and wrist in children and adolescents with and without CF. All cut-points demonstrated fair to excellent accuracy, sensitivity and specificity, and low error. Overall, the GENEActiv provided significantly higher outputs, in comparison with ActiGraph, irrespective of placement and population.
Overall, the disparity in the cut-points developed for youth with CF, relative to an apparently healthy population, both in the present study and in previous literature (Stephens et al., Citation2016), further supports the contention that cut-points developed in healthy children and adolescents are not suitable to estimate PA levels in youth with CF (Bianchim et al., Citation2020). Most importantly, earlier studies estimating PA levels in pediatric CF cohorts from nonspecific cut-points have potentially underestimated total MVPA in these populations. Indeed, previous research reported that those with CF spent less time in MVPA and more in LPA when compared with healthy participants (Aznar et al., Citation2014; Selvadurai et al., Citation2004); the present research shows that such findings may have been due to the cut-points applied, and subsequently a misclassification of PA. Therefore, the potential implications of utilizing these CF-specific cut-points, not least in terms of health outcomes or prognostic indicators, cannot be understated.
Among other factors, the use of count-based cut-points is reported to be responsible for misclassifying PA intensities 33–68% (Trost et al., Citation2010). Consequently, more recent calibration studies have utilized raw acceleration metrics, such as ENMO and MAD (De Almeida Mendes et al., Citation2018). However, the majority of studies developing cut-points from raw acceleration metrics have focused on adults, with few generating thresholds for children (Hildebrand et al., Citation2014; Migueles, Cadenas-Sanchez et al., Citation2019). Importantly, whilst our study found that the MPA and VPA cut-points developed for healthy children were largely comparable to these earlier studies, the MPA and VPA CF-specific cut-points were substantially lower. Such discrepancies may be due to the ventilatory and muscular impairments associated with the pathophysiology of CF (Stephens et al., Citation2016). Specifically, given the enhanced cost of breathing and the pathologic exercise intolerance associated with the condition, daily-life activities are likely to be more energetically costly for those with CF in comparison with their healthy counterparts (Matel & Milla, Citation2009). As such, it is expected that those with CF will have higher EE at lower raw accelerations than healthy participants during given activities. In accord with this, those in the CF group expended more energy during all the sedentary tasks and had significantly higher accelerometer outputs and EE during walking. Differences during walking may reflect altered muscle and metabolic function even in youth with mild CF (Erickson et al., Citation2015), reinforcing the crucial need for condition-specific cut-points. It is important to highlight, however, that there were no differences in METS or RMR between CF and healthy controls.
A unique aspect of this study was the comparison of outputs from two different accelerometer brands, across wrist and waist placements. Overall, GENEActiv generated higher values for both ENMO and MAD across all placements and participants, in accord with previous studies (Hildebrand et al., Citation2014; Hurter et al., Citation2018). Such inter-brand differences could be attributed to multiple factors including, but not limited to, a difference in the magnitude of acceleration signals, proprietary filters, signal to noise ratios, and data resolution (Hildebrand et al., Citation2014). It is well known that ActiGraph has an inbuilt low-pass filter of an unknown cutoff frequency, which is considered proprietary information (John et al., Citation2012). Previous studies have shown that outputs from different accelerometer brands are not interchangeable, despite that this difference does not seem to compromise the accuracy of the PA measurement (John et al., Citation2013; Montoye et al., Citation2016). However, it is crucial that future studies should use brand-specific cut-points to avoid misclassification.
Intra-brand comparisons showed that the dominant wrist-worn GENEActiv yielded higher ENMO and MAD values, particularly during free-games. This is particularly important considering that this activity was designed to replicate children’s normal daily-life routines. Indeed, congruent with previous research in healthy children (Hildebrand et al., Citation2014), the agreement between placements across brands varied greatly by activity, with more pronounced differences shown for coloring, playing on a handheld device, free-games and stairs. Interestingly, Hildebrand et al. (Citation2014) did not find such differences in adults. Of importance, MPA cut-points from the dominant wrist performed slightly better amongst all placements in CF, whilst the non-dominant wrist and waist performed better in healthy participants. Indeed, research in healthy children recommended that the non-dominant wrist should be the placement of choice to prioritize compliance (Hildebrand et al., Citation2014). However, as both wrist placements performed well, and to allow for greater standardization across studies, the non-dominant wrist placement is recommended in youth with CF.
The cross-validation found that the cut-points developed in children and adolescents accurately classified SED, MVPA and VPA most of the time, irrespective of condition, brand and placement. In particular, cut-points derived from ENMO achieved superior accuracy for all placements and brands, in comparison with MAD, independent of health status. Nonetheless, further validation of the CF-specific cut-points is warranted to ensure validity in free-living conditions (Mackintosh et al., Citation2012).
Despite numerous strengths, this study is not without its limitations. Our sample consisted of children and adolescents with mild CF and the developed cut-points may therefore not be applicable to those with a more severe condition. However, it is important to highlight that with the advent of the triple-combination therapy Kaftrio (tezacaftor*elexacaftor*ivacaftor; Jaques et al., Citation2020) it could be argued that mild CF is going to be more common in the near future, and therefore these cut-points may be applicable to a wider proportion of the population with CF. Additionally, due to the COVID-19 lockdown, it was not possible to recruit as many healthy age- and sex-matched healthy participants. Finally, it is paramount to recognize that the statistical approach used to develop the cut-points can greatly impact the prediction accuracy (Welk, Citation2005). Specifically, whilst ROC curve analyses presents advantages in relation to linear-models (Bianchim et al., Citation2020), it may not be optimal for clinical populations, where adjusting for health-related confounding factors may enhance accuracy and account for inter-patient variability. Given the complexity and non-linear nature of PA, future research should consider using machine learning to further enhance the accuracy for PA classification in those with CF (Bianchim et al., Citation2020).
This is the first study to calibrate and cross-validate cut-points from raw accelerometer data for children and adolescents with CF. The newly developed CF-specific cut-points demonstrated high sensitivity and specificity, fair to excellent accuracy, and a low error. Most importantly, the majority of the CF-specific cut-points, particularly MPA and VPA, were lower than those developed for healthy controls and previously reported cut-points. Therefore, the newly developed CF-specific cut-points highlight the need to reevaluate PA levels and associated health outcomes in children and adolescents with CF.
Supplemental Material
Download PDF (696.4 KB)Acknowledgments
The research team acknowledges Mari Powell from the Pediatric Department, Morriston Hospital, Swansea, UK, for her support on patient recruitment and all the families that volunteered to this study.
Disclosure statement
No potential conflict of interest was reported by the author(s).
Data availability statement
The datasets generated and/or analysed during the current study are not publicly available due to GDPR regulations and to protect individual privacy but are available from the corresponding author on reasonable request.
Supplementary material
Supplemental data for this article can be accessed on the publisher’s website
Additional information
Funding
References
- Ainsworth, B. E., Haskell, W. L., Herrmann, S. D., Meckes, N., Bassett, D. R., Tudor-Locke, C., Greer, J. L., Vezina, J., Whitt-Glover, M. C., & Leon, A. S. (2011). Compendium of physical activities: A Second update of codes and MET values. Medicine and Science in Sports and Exercise, 43(8), 1575–1581. https://doi.org/10.1249/MSS.0b013e31821ece12
- Arvidsson, D., Fridolfsson, J., Börjesson, & Börjesson, M. (2019). Measurement of physical activity in clinical practice using accelerometers. Journal of Internal Medicine, 286(2), 137–153. https://doi.org/10.1111/joim.12908
- Aznar, S., Gallardo, C., Fiuza-Luces, C., Santana-Sosa, E., López-Mojares, L. M., Santalla, A., Rodríguez-Romo, G., Pérez, M., Garatachea, N., & Lucia, A. (2014). Levels of moderate–vigorous physical activity are low in Spanish children with cystic fibrosis: A comparison with healthy controls. J Cyst Fibros, 13(3), 335–340. doi:10.1016/j.jcf.2013.10.004
- Bianchim, M. S., McNarry, M. A., Larun, L., Barker, A. R., Williams, C. A., & Mackintosh, K. A. (2020). Calibration and validation of accelerometry using cut-points to assess physical activity in paediatric clinical groups: A systematic review. Preventive Medicine Reports, 19, 101142. https://doi.org/10.1016/j.pmedr.2020.101142
- Bland, J. M., & Altman, D. G. (1986). Statistical methods for assessing agreement between two methods of clinical measurement. Lancet (London, England), 1(8476), 307–10. https://www.ncbi.nlm.nih.gov/pubmed/2868172
- Davies, J. C., Alton, E. W., & Alton, E. W. (2009). Monitoring respiratory disease severity in cystic fibrosis. Respir Care, 54(5), 606–617. https://doi.org/10.4187/aarc0493
- de Almeida Mendes, M., da Silva, I. C. M., Ramires, V. V., Reichert, F. F., Martins, R. C., & Tomasi, E. (2018). Calibration of raw accelerometer data to measure physical activity: A systematic review. Gait & Posture, 61, 98–110. https://doi.org/10.1016/j.gaitpost.2017.12.028
- de Onis, M., Onyango, A. W., Van den Broeck, J., Chumlea, W. C., & Martorell, R. (2004). Measurement and standardization protocols for anthropometry used in the construction of a new international growth reference. Food and Nutrition Bulletin, 25(1 Suppl), S27–S36. https://doi.org/10.1177/15648265040251S104
- Erickson, M. L., Seigler, N., McKie, K. T., McCully, K. K., & Harris, R. A. (2015). Skeletal muscle oxidative capacity in patients with cystic fibrosis. Exp Physiol, 100(5), 545–552. doi:10.1113/EP085037
- Fairclough, S. J., Noonan, R., Rowlands, A. V., Van Hees, V., Knowles, Z., & Boddy, L. M. (2016). Wear compliance and activity in children wearing wrist- and hip-mounted accelerometers. Medicine and Science in Sports and Exercise, 48(2), 245–253. https://doi.org/10.1249/MSS.0000000000000771
- Hebestreit, H., Schmid, K., Kieser, S., Junge, S., Ballmann, M., Roth, K., Hebestreit, A., Schenk, T., Schindler, C., Posselt, H. G., & Kriemler, S. (2014). Quality of life is associated with physical activity and fitness in cystic fibrosis. BMC Pulm Med, 14(1), 26. https://doi.org/10.1186/1471-2466-14-26
- Hildebrand, M., van Hees, V. T., Hansen, B. H., & Ekelund, U. (2014). Age group comparability of raw accelerometer output from wrist- and hip-worn monitors. Medicine and Science in Sports and Exercise, 46(9), 1816–1824. https://doi.org/10.1249/MSS.0000000000000289
- Hurter, L., Fairclough, S. J., Knowles, Z. R., Porcellato, L. A., Cooper-Ryan, A. M., & Boddy, L. M. (2018). Establishing raw acceleration thresholds to classify sedentary and stationary Behaviour in Children. Children (Basel, Switzerland), 5(12), 172. doi:10.3390/children5120172.
- Jaques, R., Shakeel, A., & Hoyle, C. (2020). Novel therapeutic approaches for the management of cystic fibrosis. Multidisciplinary Respiratory Medicine, 15(1), 690. https://doi.org/10.4081/mrm.2020.690
- John, D., Miller, R., Kozey-Keadle, S., Caldwell, G., & Freedson, P. (2012). Biomechanical examination of the ‘plateau phenomenon’ in actigraph vertical activity counts. Physiological Measurement, 33(2), 219–230. doi:10.1088/0967-3334/33/2/219
- John, D., Sasaki, J., Staudenmayer, J., Mavilia, M., & Freedson, P. S. (2013). Comparison of raw acceleration from the GENEA and ActiGraph™ GT3X+ activity monitors. Sensors (Basel, Switzerland), 13(11), 14754–14763. https://doi.org/10.3390/s131114754
- Mackintosh, K. A., Fairclough, S. J., Stratton, G., & Ridgers, N. D. (2012). A calibration protocol for population-specific accelerometer cut-points in Children. PLoS One, 7(5), e36919. doi:10.1371/journal.pone.0036919
- Mackintosh, K. A., Ridgers, N. D., Evans, R. E., & McNarry, M. A. (2018). Physical activity and sedentary time patterns in children and adolescents with cystic fibrosis and age- and sex-matched healthy controls. Journal of Physical Activity & Health, 15(2), 82–88. https://doi.org/10.1123/jpah.2017-0011
- Matel, J. L., & Milla, C. E. (2009). Nutrition in cystic fibrosis. Semin Respir Crit Care Med, 30(5), 579–586. Epub 2009 Sep 16. https://doi.org/10.1055/s-0029-1238916
- Migueles, J. H., Cadenas-Sanchez, C., Rowlands, A. V., Henriksson, P., Shiroma, E. J., Acosta, F. M., Rodriguez-Ayllon, M., Esteban-Cornejo, I., Plaza-Florido, A., Gil-Cosano, J. J., Ekelund, U., van Hees, V., & Ortega, F. B. (2019). Comparability of accelerometer signal aggregation metrics across placements and dominant wrist cut points for the assessment of physical activity in adults [OriginalPaper]. Scientific Reports, 9(1), 1–12. https://doi.org/10.1038/s41598-019-54267-y
- Migueles, J. H., Rowlands, A. V., Huber, F., Sabia, S., & van Hees, V. T. (2019). GGIR: A research community–driven open source r package for generating physical activity and sleep outcomes from multi-day raw accelerometer data. Journal for the Measurement of Physical Behaviour Journal for the Measurement of Physical Behaviour, 2(3), 188–196. https://doi.org/10.1123/jmpb.2018-0063
- Mirwald, R. L., Baxter-Jones, A. D., Bailey, D. A., & Beunen, G. P. (2002). An assessment of maturity from anthropometric measurements. Medicine and Science in Sports and Exercise, 34(4), 689–694. https://doi.org/10.1097/00005768-200204000-00020
- Montoye, A. H. K., Pivarnik, J. M., Mudd, L. M., Biswas, S., & Pfeiffer, K. A. (2016). Validation and comparison of accelerometers worn on the hip, thigh, and wrists for measuring physical activity and sedentary Behavior. AIMS Public Health, 3(2), 298–312. https://doi.org/10.3934/publichealth.2016.2.298
- Moore, V. C. (2012). Spirometry: Step by step. Breathe, 8(3), 232–240. https://doi.org/10.1183/20734735.0021711
- Moudiou, T., Galli-Tsinopoulou, A., Vamvakoudis, E., & Nousia-Arvanitakis, S. (2007). Resting energy expenditure in cystic fibrosis as an indicator of disease severity. J Cyst Fibros, 6(2), 131–136. doi:10.1016/j.jcf.2006.06.001
- Quanjer, P. Q., Sanja, S., Tim, J. C., Xaver, B., Graham, L. H., Bruce, H. C., Paul, L. E., John, L. H., Mary, S. M. I., Jinping, Z., & Stocks, J. (2012). Multi-ethnic reference values for spirometry for the 3–95-yr age range: The global lung function 2012 equations. European Respiratory Journal. https://doi.org/10.1183/09031936.00080312
- Selvadurai, H. C., Blimkie, C. J., Cooper, P. J., Mellis, C. M., & Van Asperen, P. P. (2004). Gender differences in habitual activity in children with cystic fibrosis. Arch Dis Child, 89(10), 928–933. https://doi.org/10.1136/adc.2003.034249
- Stephens, S., Takken, T., Esliger, D. W., Pullenayegum, E., Beyene, J., Tremblay, M., Schneiderman, J., Biggar, D., Longmuir, P., McCrindle, B., Abad, A., Ignas, D., Van Der Net, J., Feldman, & Feldman, B. (2016). Validation of accelerometer prediction equations in children with chronic disease. Pediatric Exercise Science, 28(1), 117–132. https://doi.org/10.1123/pes.2014-0185
- Troiano, R. P., Berrigan, D., Dodd, K. W., Mâsse, L. C., Tilert, T., McDowell, & MCDOWELL, M. (2008). Physical activity in the United States measured by accelerometer. Medicine and Science in Sports and Exercise, 40(1), 181–188. https://doi.org/10.1249/mss.0b013e31815a51b3
- Trost, S. G., Loprinzi, P. D., Moore, R., & Pfeiffer, K. A. (2010). Comparison of accelerometer cut points for predicting activity intensity in youth. Med Sci Sports Exerc, 43(7), 1360–1368. doi:10.1249/MSS.0b013e318206476e
- van Hees, V. T., Fang, Z., Langford, J., Assah, F., Mohammad, A., da Silva, I. C. M., Trenell, M. I., White, T. B., Wareham, N. J., & Brage, S. (2014). Autocalibration of accelerometer data for free-living physical activity assessment using local gravity and temperature: An evaluation on four continents. Journal of applied physiology, 117(7), 738–744. https://doi.org/10.1152/japplphysiol.00421.2014
- Weir, J. B. (1949). New methods for calculating metabolic rate with special reference to protein metabolism. The Journal of Physiology, 109(1–2), 1–9. https://doi.org/10.1113/jphysiol.1949.sp004363
- Welk, G. J. (2005). Principles of design and analyses for the calibration of accelerometry-based activity monitors. Med Sci Sports Exerc, 37(11 Suppl), S501–511. doi:10.1249/01.mss.0000185660.38335.de