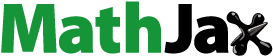
Abstract
The insurance industry is built on risk classification, grouping insureds into homogeneous classes. Through actions such as underwriting, pricing, and so forth, it differentiates, or discriminates, among insureds. Actuaries have responsibility for pricing insurance risk transfers and are intimately involved in other aspects of company actions and so have a keen interest in whether or not discrimination is appropriate from both company and societal viewpoints. This article reviews social and economic principles that can be used to assess the appropriateness of insurance discrimination. Discrimination issues vary by the line of insurance business and by the country and legal jurisdiction. This article examines social and economic principles from the vantage of a specific line of business and jurisdiction; these vantage points provide insights into principles. To sharpen understanding of the social and economic principles, this article also describes discrimination considerations for prohibitions based on diagnosis of COVID-19, the pandemic that swept the globe in 2020.
Insurance discrimination issues have been an important topic for the insurance industry for decades and are evolving in part due to insurers’ extensive use of Big Data; that is, the increasing capacity and computational abilities of computers, availability of new and innovative sources of data, and advanced algorithms that can detect patterns in insurance activities that were previously unknown. On the one hand, the fundamental issues of insurance discrimination have not changed with Big Data; one can think of credit-based insurance scoring and price optimization as simply forerunners of this movement. On the other hand, issues regarding privacy and use of algorithmic proxies take on increased importance as insurers’ extensive use of data and computational abilities evolve.
1. INTRODUCTION
Discrimination is a topic that touches on the daily lives of almost every person. The word “discrimination” generally has negative connotations; people think of discrimination as occurring when we go into a store and are treated differently because of our hair color, when we apply for a job that we are well qualified except for our age, or when we are shunned from a social group because of our ethnicity, heritage, or religious background. From this perspective, discrimination is endemic in our society and a topic that deserves serious consideration.
This article focuses on discrimination in an insurance context. Insurance is particularly interesting because the entire industry is based on discrimination. Here, we use the word discrimination in an entirely neutral way, taking it to mean the act of treating different groups differently, where the groups are distinguished by salient features such as hair color, age, gender, heritage, religion, and so forth, whether such discrimination is justifiable or not. For example, auto insurers often charge younger (presumably riskier) drivers more than older (presumably safer) drivers but do not make a distinction between brown-haired and red-haired drivers (presumably because the two groups are equally risky). So, discrimination based on age is done routinely, whereas discrimination based on hair color is not. In subsequent sections, we present different arguments about whether insurance discrimination is ethical or is “unfair” and morally indefensible in some sense.
1.1. How Insurers Discriminate
Insurers collect information on current and potential customers. They collect information about the customers themselves, the entity being insured (whether a person, organization, or physical object such an auto or home), where the entity is located (that can vary, such as a person or auto), and parameters about the contract desired, among other things. This information, represented as variables or factors, provides the basis that insurers use to form groups and make decisions. By treating groups differently, they discriminate among them. Before describing the process of forming these groups and weighing in on whether or not the use of a specific variable is ethical, we first describe the set of actions that an insurer might take. In short, how do insurers discriminate among customers?
Issuance, renewal, or cancellation
The first stage is the decision to insure. We follow the structure of Avraham (Citation2018), who noted that the harshest form of discrimination is the decision on whether or not to issue a policy because of some characteristic, such as the applicant’s religion or ethnicity. This may be at the underwriting stage or even earlier, at the marketing stage. For example, Subsection 2.3 describes classical issues of so-called redlining where insurers simply did not enter geographic districts with high concentrations of African Americans (who were assumed to be high risk). A similar type of discrimination happens when insurers refuse to renew or when they cancel policies based on some characteristic. Indeed, some jurisdictions have statutes that limit or prohibit the use of a particular characteristic in either issuance, renewal, or cancellation of an insurance contract.
Coverage
Another form of discrimination involves restricting coverage in ways that might harm disadvantaged groups. For example, insurance companies might limit disability insurance coverage for people with disabilities that stem from having HIV.
Pricing
Even without limitations on issuance and coverage, insurance companies can still distinguish among insureds by simply charging different premiums. For example, an insurer may admit people with various diseases and disabilities into their pool, yet charge these people a higher premium. Is this fair or unfair? Some argue that a specific disease––for example, cancer––is no fault of the individual and so they should not bear the additional burden of higher premiums. A counter position is that by admitting high-cost individuals to the pool, this raises the costs for all in the insurer’s pool (even those without the specific disease).
1.2. Insurance Prohibitions
The modern-day insurance industry is founded on the ability to differentiate, or discriminate, among risks, known as risk classification. Thinking of an insurer as a private company, there are strong economic arguments for permitting insurers to discriminate among risks; Section 3 presents economic reasoning for this permission. There is evidence, consistent across lines of business and international jurisdictions, that insurance policyholders believe that some insurance discrimination is fair (cf. Schmeiser, Störmer, and Wagner Citation2014). However, there are also instances where consumers are concerned with “unfair” discrimination. When insurance is mandatory or nearly so, it becomes less of an economic commodity and more of a social good, resulting in different attitudes toward “fairness.” Section 2 describes social justice considerations that underpin notions of fairness. Because of these concerns, regulators and policymakers in many jurisdictions impose restrictions on insurers abilities to discriminate.
Disagreements among stakeholders about what constitutes fairness can arise depending on whether one views insurance as an economic commodity or as a social good. Employees of insurers often think of insurance as an economic quantity and argue that risk classification is morally appropriate (cf. concepts of actuarial fairness in Section 2). Consumer advocates focus on concepts of solidarity and cross-subsidization of risks, thinking of insurance as a social good, or a basic human right. These views depend markedly on the jurisdiction and line of insurance business, as discussed in Subsection 4.2 and Section 5, respectively.
Insurance discrimination, including the prohibitions from regulators that insurers might face and how these prohibitions affect society, has long been an important topic. Issues of fairness have not been resolved nor are they becoming less relevant; its prominence has lately increased with the increasing importance of “Big Data” where massive data sets and increasing computing power have become commonplace (cf. Thouvenin et al. Citation2019). Like other major industries, insurers have at their disposal increasing amounts of information available about current and potential customers. As emphasized in Subsections 5.5 and 6.4, more information gives insurers opportunities to differentiate among potential clients at increasingly granular levels; many are doing so because of real or perceived pressures from competition. However, not all information that could be used should be used. We seek to provide a framework for actuaries and other analysts to think about the use of potentially sensitive information.
2. SOCIAL JUSTICE CONSIDERATIONS
2.1. What Is Actuarial Fairness?
To understand what is unfair discrimination and what insurers should be prohibited from doing, let us start with a discussion of “fair” insurance mechanisms. We begin with a historical context, drawing on the work of Frezal and Barry (Citation2019).
2.1.1. Pooling and Solidarity
In the early 17th century, mathematicians used games of chance such as rolling dice and drawing lotteries to develop a theory of randomness. For example, the fair price for each participant in a lottery was determined by the sum of wagers divided by the number of participants. Fairness was thus understood within the framework of individual equitable contracts, ones that traded a certain present amount for an uncertain future value. Even though the ex post results of the game had winners and losers, because the ex ante probabilities were equal, such games could be accepted as fair.
Much later, in the 19th century, a similar mathematical model was used for insurance pricing. From an individual’s perspective, one could again trade a certain present amount, the premium, for an uncertain future value that would provide compensation for an insured loss. For an insurance pool, the sum of future values was no longer certain (unlike the lottery size) but, with the additional regularity in the sum of future value of losses, there were real benefits in pooling of risks. In the modern language of probability, although the amount that an individual might expect to lose for an insured loss remained unchanged, the amount of uncertainty was vastly reduced.
Insurance pooling offered a novel method for coping with the uncertainty of losses. Before this, the only method of coping with potential loss events was individual prudence. With an insurance pool, losses now became the responsibility of the pool. From a moral perspective, the responsibility for the accident could now be thought of as not due to the behavior of the faulty individual but rather attributed to the collective; in this sense, pooling socializes responsibility (cf. Baker Citation2002).
As further discussed in Lehtonen and Liukko (Citation2011), pooling creates a sense of shared responsibility among a group of people. This, combined with a certain understanding of equality and justice, creates a type of insurance solidarity. It is not the same type of solidarity that one thinks of in political movements, which embody a conscious identification with the group, emotional bonds, shared values and beliefs, and so forth. It is solidarity that emphasizes mutual responsibility, reciprocity, and a particular shared understanding of fairness. Insurance provides a mechanism to transfer an individual’s uncertainty to a pool; to achieve this agreement, the individual must have faith in the pool.
2.1.2. Responsibility and Actuarial Fairness
With the shift of responsibility from the individual to the pool, one can imagine that the sense of fairness shifts and depends upon the nature of the pool. If the pool is formed from a small group of like-minded individuals (think of the classical case of a group of farmers forming a collective to restore a member’s barn in the event of fire), then there will be little difference between notions of fairness for the individual and for the pool collective. However, modern-day insurance is generally sponsored at large levels, either by governments or private corporations, the latter of which can be owned by policyholders (mutual or takaful companies) or by investors (stock companies). Members of the pool may feel a type of (insurance) solidarity but the responsibilities of the pool depend on its nature.
Stock Insurance Company
At one end of the spectrum is the case of the pool of contracts issued to individuals by a for-profit stock company. Here, the pool can be thought of as a sum of bilateral contracts that leaves out the collective dimension of insurance. Actuarially fair pricing is based on the expected value of the uncertain event at stake, taken to be the risk transferred from the insured to the insurer. In this context, fairness means that each customer should pay for their own risk and only their own risk. As will be discussed in Section 3, there is ample basis for this position from economic theory.
Government
At the other end of the spectrum is the case when the pool is owned by a government entity where such contracts constitute social insurance. Subsidies, from one group to another, are common in social insurance. Governments regularly engage in social policy such as the redistribution of risk or income. The use of insurance to subsidize the underprivileged is consistent with what many view as a government’s core mission. In social insurance, there can be a large variation in how strictly the principle of actuarial fairness is followed.
Group Insurance
Between these two ends of the spectrum, there is substantial variation in principles of fairness depending on who owns the pool and the nature of the contractual arrangement. For example, consider a disability income contract issued to a large group, such as a university. Because the employer (university) pays all or a major portion, premiums rated by risk factors are not a major issue (unlike the individual market). In general, in group insurance, the amount of socialization is greater than one would find in the corresponding individual market.
As described in Baker (Citation2002), most people do not think that their premiums will go to pay other people’s claims. Instead, they think of it as a type of savings account and often expect that over the course of a lifetime the deposits made by each person should roughly equal the withdrawals on that person’s insurance account. Thinking of premiums as going to pay others claims emphasizes the social aspect of insurance. That is, the losses, understood as belonging to the collective, are also borne collectively (Lehtonen and Liukko Citation2011).
Mutual Company
In a mutual insurance company, the policyholders are both customers and owners. Unlike stock companies, ownership rights of the mutual policyholders are not transferable. By eliminating stockholders with their separate and sometimes conflicting interests, potential conflicts between owners and customers over dividend, financing, and investment policies are internalized. This is the major benefit of the mutual form of organization. Because the owners of the pool are the policyholder themselves, this suggests that the amounts of cross-subsidies among groups or socialization would be greater than in an organization with a for-profit motive. However, in point of practice, mutuals compete with stock companies and so many of their practices are indistinguishable from those of stock companies. A small academic literature examines differences between stocks and mutuals (cf. Braun, Schmeiser, and Rymaszewski Citation2015). In part due to the policyholder’s owner stake in the company, evidence from this literature suggests that policies offered by stock insurers are overpriced relative to policies of mutuals. Nonetheless, we know of no study that has confirmed or disproved the conjecture that mutuals discriminate differently than stock insurers.
Takaful
As another example, consider modern takaful companies (cf. Maysami and Kwon Citation1999). In many senses there are similarities between takaful and mutual companies. Classical Western insurance appears to violate the Islamic prohibition of gambling (as well as the Islamic prohibition of usury). Instead, takaful insurance offers, not as a bilateral contract, a transfer of a known risk to a collective enterprise by which Muslims pool resources to aid one other in the event of loss. Responsibility of the loss shifts from the individual to the collective and so aspects of fairness shift.
2.1.3. Insurance as a Social Good
Attitudes toward fairness also depend upon whether an insurance product can be viewed as a social or type of public good. A social good is something that benefits the general public such as clean air, clean water, and literacy. One characteristic of a public good is that it is nonexcludable; that is, it cannot be provided unless others can also enjoy it. For example, if you erect a dam to stop flooding, you protect everyone in the area (whether or not they contributed to erecting the dam). Thus, viewing an insurance product as a public good would argue against excluding members of society.
For example, in many countries health insurance is likely to be seen as a social good where access to a certain level of health care is guaranteed for all. This is even true in a country like the United States, which generally has taken longer to improve access to health care than other countries. In contrast, life insurance is more often seen as a private (non-public) economic commodity. Life insurance can enhance the financial security of the family of a policyholder but is voluntary and is not viewed as a necessity. Other insurance lines, such as long-term care and disability insurance, arguably fall somewhere in the spectrum between social and economic private goods (cf. Prince Citation2019).
If an insurance product is thought to be a social good, a related question is whether members of the public have equal access to the product. Specifically, the issue is whether there is an impact that puts members of a select “protected” group at a disproportionate disadvantage compared with members of a similar group. Issues of such disparate impact are difficult for individual insurers to address but are important for public acceptance of the insurance marketplace (cf. Miller Citation2009). Regulators have been grappling with the question of whether laws that prohibit discrimination based on race, religion, or national origin, could or should cover instances of disparate impact on underserved or protected classes of consumers emanating from the use of predictive modeling and analytics.
2.2. Characteristics of Sensitive Variables
Grouping, or classifying, insureds into homogeneous categories for the purposes of risk sharing is at the heart of the insurance function. Many variables that insurers use are seemingly innocuous (e.g., blindness for auto insurance), yet others can be viewed as “wrong” (e.g., religious affiliation), “unfair” (e.g., onset of cancer for health insurance), “sensitive” (e.g., marital status), or “mysterious” (e.g., Artificial Intelligence produced). When regulators and policymakers decide that it is not permitted to use a variable for classification, it is thought of as creating a protected class. By and large, the choice of whether a variable should be used for insurance purposes is a normative one. Although actuaries and other financial analysts determine insurance premiums from the available information, the choice of which variables to use is a societal one in which many actors participate.
Nonetheless, it is helpful to understand what variable attributes influence society’s assessment of whether it is fair for insurance purposes. When identifying whether or not a variable contains sensitive information, we use a structure drawn from Avraham (Citation2018) and Prince and Schwarcz (Citation2020).
Control. If an insured has control over an attribute, it is generally deemed to be an acceptable variable to be used for insurance purposes. For an example from auto insurance, consider a variable that indicates whether the car is high-performance, capable of going at fast speeds and expensive to replace in the event of an accident. An insured chooses whether or not to purchase a high-performance vehicle and so vehicle type is generally deemed to be an acceptable variable. In contrast, race and sex at birth are examples of characteristics over which insureds have no control. Naturally, questions of degree of choice enter; for example, in life insurance, smoking is generally now accepted as a rating factor, whereas religious affiliation is generally prohibited.
Mutability. Does the variable change over time (such as age) or stay fixed? It is possible that rating by age is tolerable because we all get the same chance to be on the winning side and the losing side of it over the course of a lifetime.
Statistical Discrimination. A variable should have some predictive value of an underlying risk. If it does not, then it is generally viewed as unacceptable for insurance purposes. As a rule of thumb, the better predictor of risk the characteristic is, the more tolerable such discrimination becomes. However, some pricing variables may not have such predictive abilities; Subsection 3.3 will sharpen this precept by distinguishing between “risk-based” and “non-risk” price discrimination in insurance.
Causality. It is generally acceptable to use a variable if it is known to cause an insured event. For example, an individual diagnosed with cancer will generally be unable to purchase life insurance. Naturally, establishing causality is a much higher bar than mere correlation, or predictive ability, for a risk. For example, decades were spent building scientific evidence before it was widely established that smoking causes premature deaths (cf. Peto Citation1994).
Limiting or Reversing the Effects of Past Prejudice. Does an insurer’s use of a trait perpetuate negative stereotypes or otherwise subordinate disadvantaged groups? The historical use of the characteristic as a method of discrimination is also relevant; that is, whether the characteristic defines a socially salient group that has been disadvantaged in the past. In that sense, discriminating based on skin color is more problematic than that based on eye color.
Inhibiting Socially Valuable Behavior. Does an insurer’s use of a trait inhibit or prevent socially desirable activities? Subsection 5.1 describes how individuals, fearful of being denied life insurance, avoid participating in genetic testing research. As another example, Prince and Schwarcz (Citation2020) cited U.S. laws that prohibit insurers from discriminating on the basis of intimate partner violence because such reporting could dissuade victims of violence from seeking needed medical care or police intervention.
Whether a characteristic is socially suspect or sensitive is context dependent, depending on the jurisdiction and the line of insurance business, as described in Subsection 4.2 and Section 5.
2.3. Indirect Discrimination
One of the difficult questions in insurance discrimination is the treatment of related variables; use of variables related to a prohibited variable constitutes indirect discrimination. These are variables that, although they do not have the usual characteristics of an unfair variable (Subsection 2.2), have undesirable effects on society. A classic example is redlining, a term that refers to the practice of drawing red lines on a map to indicate areas that insurers will not serve, areas typically containing high proportions of minorities.
Specifically, we can define indirect (insurance) discrimination as consisting of three elements: proxy discrimination, disparate impact, and whether or not the discrimination could be avoided by other means. We address each in turn.
Proxy discrimination, also known as indirect statistical discrimination, occurs when insurers discriminate based on a facially neutral characteristic, such as the size of the car engine, that correlates with a protected class, such as gender (in many studies, men like to drive cars with big engines). In the case of redlining, insurers discriminate based on geographic area (such as an urban area), which is correlated with race. Specifically, by avoiding certain urban areas, they also avoid large groups of potential minority customers; this amounts to at least partially making insurance decisions based on a protected variable.
It will be helpful to think about two types of proxies: one where an identifiable surrogate such as geographic area serves as a substitute for a protected variable such as race and one where the proxy is produced by an algorithm that summarizes the effects of many variables. Subsection 5.5 on big data emphasizes the increasing importance of the second type of proxy discrimination as insurers utilize increasingly sophisticated algorithms and growing sources of data. Empirical aspects of proxy discrimination are discussed further in Section 6.
The second element is disparate impact; that is, whether there is an impact that puts members of a protected group at a disproportionate disadvantage compared with members of a similar group. Returning to the redlining example, this practice puts minority neighborhoods at an economic disadvantage. Baker (Citation2002, p. 41) stated: “A neighborhood redlined by insurance companies is a more risky place for banks to lend. Without good financing opportunities, fewer people invest in the neighborhood, and without investment the neighborhood becomes an even more risky place for banks, causing further decline.”
These notions of proxy discrimination and disparate impact are drawn from the legal literature and it can be sometimes difficult to infer a precise mathematical formulation. For our purposes, we think of proxy association as the relationship between a protected variable, and a set of one or more surrogate variables whereas disparate impact occurs when there is a relationship between a protected variable and an outcome of interest (e.g., insurance purchase). In Subsection 6.4, we supplement these definitions by drawing from the machine learning literature.
The third element is whether the criterion is motivated by a legitimate business necessity. If it is, then discrimination may be legal even in the event of producing a disparate impact. For example, Subsection 5.3 will describe models that optimize insurer’s profitably objectives, a legitimate business motivation, at the expense of disfavoring customers with fewer market options who tend to be low-income and minority consumers.
As another example, the Council of the European Union (EU Citation2012) Directive 2004/113/EC Guidelines on the Application of the Gender Directive, “The use of risk factors which might be correlated with gender […], as long as they are true risk factors in their own right,” is still permitted.
3. ECONOMIC CONSIDERATIONS
One approach to pricing is to think of an insurance contract as a type of financial investment. From this viewpoint, financial investors base risk transfer considerations on a law of one price that is dictated by forces of supply and demand in a competitive market. An advantage of this approach is that many issues of insurance discrimination become moot because prices are given by an external marketplace. Many readers will enjoy thinking about pricing of insurance contracts in the context of financial economics asset pricing theory, summarized by Bauer, Phillips, and Zanjani (Citation2013).
However, even in personal lines (where there is much more homogeneity than in commercial lines), there is substantial heterogeneity among insurance products when considering the variety of contract features (deductibles, limits, coinsurance, and so forth), risk factors of the entity insured (e.g., auto or home), and risk factors of the insured (e.g., attitude toward risk) that exist. Because of this heterogeneity, insurance pricing is focused on the underlying cost of producing the good or service.
Like any firm, the price that an insurer charges is determined by the quantity, where marginal cost equals marginal revenue. However, unlike other industries, determining marginal costs is difficult in insurance. In part this is because the production cost is random. By definition, insurance contracts are based on contingent events whose financial outcomes are uncertain. Further, even when an insured event does occur, the actual cost of an insured claim may not be known for a long period of time.
As further developed in Subsection 4.1, insurance prices are based on the expectation of losses, a concept coined as an actuarially fair price in Arrow (Citation1963). In a simple model, an actuarially fair price is the result of an assumption of zero profits and ensures that the insured will buy full insurance coverage. It is the foundation of insurance pricing.
3.1. Adverse Selection, Moral Hazard, and Incentives
Simple models can provide insights but are naturally limited in addressing the numerous features of real contracts. One feature particularly relevant to potential insurance discrimination is unequal access to information, known as information asymmetry.
Insurers traditionally face adverse selection, a problem that can arise when consumers know more about their own risk characteristics than insurers. Insurers argue that by knowing about risk factors, the entire marketplace is better. Indeed, the entire purpose of risk classification is to mitigate the problem of adverse selection. Extending this line of thought, the more information that insurers have about policyholders, the more effective risk classification is; this in turn results in a better marketplace for all.
Another type of adverse selection can occur when an insurer has less information than other competing insurance companies about the risk levels of its customers (cf. Cather Citation2018). This can result in cream skimming, because the innovative insurer targets the best risks who, like cream in a container of fresh milk, rise, to the top of a pool of policyholders.
Another classic type of information asymmetry is moral hazard. Insurers worry about insureds’ attitudes toward safety; by purchasing insurance, insureds have the incentive to take on more risks (thus increasing the probability of a risky event). For example, after purchasing homeowner’s insurance, the insured may become lax in watching for fires (smoking in bed, not checking for frayed electrical wires). One way to mitigate this risk is through the installation of fire alarms.
Insurers also have to be wary of their own moral hazard. For example, if they acquire a protected variable such as political affiliation, then they have to be careful that this knowledge does not implicitly bias their pricing processes even if they do not use this information actively. One way to mitigate this risk is to simply avoid acquiring such protected information.
An implication of moral hazard is that people tend to increase their risk unless given incentives not to. Conversely, people may also reduce their risks when given incentives to do so. Indeed, much of modern risk management is predicated on introducing risk mitigation tools to reduce the impact of insured events. Classic examples include lower premiums for sprinkling systems in fire insurance and nonsmoker discounts in life insurance (Avraham, Logue, and Schwarcz Citation2014).
Insurers worry about traditional adverse selection and moral hazard because information asymmetries favor policyholders over insurers. In contrast, as emphasized by Schwarcz and Siegelman (Citation2017), much of insurance law is designed to protect policyholders because of information asymmetries that favor insurers over policyholders. For example, policyholders are often insufficiently knowledgeable about an insurance policy’s terms and conditions, the insurer’s financial strength, and the appropriate type of policy for a consumer’s needs. Regulators are concerned that insurers may be able to exploit these deficits in policyholder information or sophistication by providing more limited coverage than policyholders believe they are purchasing or by adopting excessively aggressive claims-handling strategies.
More recently, consumer advocates have been concerned that additional big data information, discussed more in Subsection 5.5, puts consumers at a disadvantage. For consumer advocates, more data information for insurers means that:
Insurers can cherry pick at a granular level.
Insureds do not have equivalent new tools to compare quality of policies and performance of insurance companies.
Consumer advocates argue that mandatory and de facto mandatory purchase of insurance means that free market competition is insufficient to protect policyholders.
3.2. Economic Efficiencies
Economists largely agree that a competitive market is an efficient one (cf. Skipper and Klein Citation2000). Efficiency is achieved because competition forces buyers to pay their maximum demand price and forces sellers to charge their minimum supply price. Competition serves the best interests of consumers in that it provides insurers incentives to attract customers by reducing prices or improving insurance products. Competition policy is about applying rules to make sure that companies compete fairly with each other.
One of the barriers to competition is asymmetric information; the insurance industry uses risk classification to cope with this potential problem. What could happen without risk classification? Because of the price differential we might see a reduced pool of insured individuals; this reflects a decrease in the efficiency of the insurance market. Extending this line of thought to multiple periods suggests an exodus of low risks that can lead to a death spiral of rising premiums and ends up unraveling the entire market (cf. Dionne and Rothschild Citation2014).
However, by and large, insurers are allowed to classify risks. As argued by Tennyson (Citation2007), a large body of academic research supports the conclusion that insurance markets function in a workably competitive manner in the absence of rate regulation. Competition indicators include the number of insurers and their market shares, profitability, and price of their products. At least some markets for some countries (e.g., U.K. auto) generally exhibit the characteristics of a competitive market (Financial Conduct Authority [FCA] Citation2019, Annex 3: International Comparisons).
Rate regulation can limit the insurers ability to classify risks and hence threaten competition. Tennyson (Citation2007) described two types of rate regulation regimes:
rate suppression: reducing average rates for all consumers, and
rate compression: reducing rates for some consumers (usually high-risk) relative to others (usually low-risk).
Both have negative consequences for insurance markets. Rate suppression runs the risk of driving average premiums below competitive levels, reducing insurer returns below a competitive rate of return. Rate suppression will distort insurance supply in the market, reducing competition in the long run. Rate compression can have similar effects by reducing rates for some consumer groups below competitive levels. As an example of evidence of the negative effects of rate regulation, in a classic article, Blackmon and Zeckhauser (Citation1991) documented the negative effects of rate suppression and compression for the automobile market in Massachusetts.
3.3. Price Discrimination
The act of charging different prices for identical products is known in economics as price discrimination. To apply this to insurance, we need to specify that identical products also means identical production costs. We could, for example, have two auto policies that promise to pay exactly the same benefits for a loss, but, their prices may differ depending on risk factors such as the insured’s ability to drive and attitude toward risk, the type of vehicle itself (inexpensive family car versus a pricey sports car), the location where it is being driven (city versus rural), and so forth. So, the expected loss would be different causing the prices to differ. This type of risk-based price discrimination is the norm in insurance pricing. In contrast, Thomas (Citation2012) used the phrase non-risk price discrimination for the insurance situation where prices may differ for the same coverage and underlying risk characteristics.
Price discrimination is common in other industries. For example, airlines regularly charge higher prices for flights during the week (e.g., Monday to Friday) because these are typically taken by business travelers. This is an example of first-degree price discrimination where the price is based on the buyer’s willingness to pay. Second-degree discrimination involves quantity discounts, and third-degree discrimination reflects different prices for different consumer groups; for example, discounts for senior citizens (known as “honored citizens” in Portland). Price discrimination is not possible in a perfectly competitive market because there are many firms competing for the price (cf. Lukacs, Neubecker, and Rowan Citation2016).
In the insurance industry, first-degree price discrimination is common in large commercial insurance where it is assumed that buyers are sophisticated and willing and able to negotiate prices. Third-degree price discrimination is common in personal insurance, at least in some jurisdictions such as within Europe and the United Kingdom. In particular, prices for renewing customers are often distinguished from risk-identical new customers, with different (usually lower) price offers made to new customers: “paying customers to switch.” To illustrate, the work in Adams et al. (Citation2015) on general insurance auto confirms that some consumers pay much higher prices if they stay with the same insurer, particularly for a long period of time. This practice is motivated by so-called price optimization models, which are described in Subsection 5.3.
For personal insurance, some jurisdictions allow price discrimination but others take a dim view of it. For example, in the early to mid-1800s, U.S. voluntary associations of insurers were organized in part to enforce uniform rates among the insurers. Uniform rates were desired so that rates were adequate to protect against insolvencies and were not unfairly discriminatory. From Miller (Citation2009, p. 278):
The primary concern with unfairly discriminatory rates, often stated at the time, was that rich and powerful insureds could unfairly negotiate lower rates than were being charged to less influential insureds, even though their degree of risk and underlying insurance costs did not warrant a lower rate.
Ability to discriminate. Insurers can differentiate prices because of the quality of their data, the general confusion surrounding the pricing process, and the consumer’s inability to “re-sell” the product.
Price discrimination in insurance does not facilitate new markets.
Price discrimination may undermine utmost good faith. Laws mandate that customers provide information about their risks truthfully and, in many jurisdictions, insurers are also permitted to share information for the purposes of preventing fraud. If this information is used for other purposes, then over time this could undermine public acceptance of the doctrine of utmost good faith.
Price discrimination may undermine justifications for risk-related pricing.
Distributional effects of price discrimination. Allowing price discrimination may introduce cross-subsidies and have uneven effects on different parts of society.
Insurers may wish to use non-risk-related factors to achieve legitimate business goals, such as maximizing profit or increasing customer retention. In these cases, firms may unwittingly discriminate, knowing only that a facially neutral practice produces desirable outcomes. Subsection 5.3 describes the specific case of price optimization models where use of non-risk-related factors is prohibited in many jurisdictions.
4. ACTUARIAL ASPECTS OF RATE REGULATION
Subsection 1.1 provides an overview as to ways in which insurers discriminate; the focus of this section is on the pricing function. This is because actuaries are heavily involved, and hence influential, in pricing. Further, many regulations are geared toward pricing prohibitions, known as rate regulation. In addition, one can argue that prices are intimately related to whether or not someone is offered coverage (one could “price someone out of the market”) and the amount of coverage.
4.1. Pricing
Like any business, pricing is critical in insurance. As described in Section 3, one aspect in which insurance differs from other industries is that the cost of the good is random and may not be known for many years after the sale of the product. This has led the actuarial profession to think deeply about what this “cost” entails.
Nonetheless, prices are often based on the cost of insurance. These are the costs of transferring a risk from the policyholder to the insurer. As described in standard actuarial textbooks such as Friedland (Citation2013) and Werner and Modlin (Citation2016; see also Free Citation2018, ch. 7 of the open source Loss Data Analytics), insurance costs consist of the (1) losses (compensation provided by the insurer for the insured claim), (2) expenses associated with the policy and claim, and (3) cost of capital (costs of keeping monies necessary to fund the insurance operation). Prices based on insurance costs are sometimes known as technical prices.
As described in the online supplement (Frees and Huang Citation2021), many jurisdictions are silent on insurance rate regulations and so market prices are influenced by forces of supply and demand. As with other businesses, the cost of a product is an important but may not be the sole determinant of a price. Additional factors include the market availability of alternatives (e.g., costs of self-insurance, prices offered by competing firms) and marketing considerations such as customer loyalty. However, in jurisdictions where rate regulation is prominent, prohibitions are in terms of technical prices. Because our focus is on rate regulations, we focus on cost-based technical prices.
Also discussed in the online supplement, many jurisdictions exhibit insurance rate regulations in one form or another. At the time of this writing, the United States is the country that most actively regulates rates and so we use this to motivate the discussion. From the U.S.-domiciled Casualty Actuarial Society’s Statement of Principles Regarding Property and Casualty Insurance Ratemaking (Principle 4) (https://www.casact.org/sites/default/files/2021-05/Statement-Of-Principles-Ratemaking.pdf), “A rate is reasonable and not excessive, inadequate, or unfairly discriminatory if it is an actuarially sound estimate of the expected value of all future costs associated with an individual risk transfer.”
In the U.S., insurance is regulated at the state level. The National Association of Insurance Commissioners (NAIC) is an organization that provides standards that states may adopt. As described in the model rating law NAIC (Citation2010), the rule is that “rates shall not be excessive, inadequate or unfairly discriminatory.” It further defines an unfair discriminatory rate as “unfair discrimination exists if, after allowing for practical limitations, price differentials fail to reflect equitably the differences in expected losses and expenses.”
As an expectation, cost-based prices are naturally influenced by the choice of rating factors and this is where regulation comes into play. Regulators prohibit the use of certain variables. How do these prohibitions affect rating schemes?
4.2. Extent of Regulation
Insurance regulations may consist of applicable acts, statutes, regulations or any other binding authority (such as accounting standards and any regulatory guidance that is effectively binding), as described within the International Standards of Actuarial Practice of the International Actuarial Association (Citationn.d.). In most jurisdictions, their enforcement is overseen by an insurance supervisor or regulator, many of whom follow the standards developed by the International Association of Insurance Supervisors (Citationn.d.). According to their website, the International Association of Insurance Supervisors “is the international standard-setting body responsible for developing and assisting in the implementation of principles, standards and other supporting material for the supervision of the insurance sector.” These include standards known as insurance core principles: on insurer solvency, sales practices, agent licensing and policy forms, for example. Interestingly, there is no discussion within the insurance core principles on rate regulation.
The extent of insurance rate regulation varies by jurisdiction. At one end of the spectrum, the phrase active rate regulation means that the regulator is heavily involved in determining rates. This could mean government mandated rates in which regulators dictate the rates to be charged. Alternatively, regulators may only require approval of rates, either in advance or concurrent with policy offerings. Friedland (Citation2013, ch. 27) summarized the spectrum of rate regulation. To illustrate, for the United States, Borselli (Citation2011) provided additional details including types of regulatory systems organized by state and line of business. The other end of the spectrum is competitive rating or open competition systems. Borselli (Citation2011) compared active rate regulation to open compensation systems. He noted that historically many European countries operated under active rate regulatory environments but now regulators of European member states do not have the right to regulate insurance prices.
For more concrete descriptions, in the online supplement (Frees and Huang Citation2021) we describe several major regulatory jurisdictions:
The United States is the largest general insurance marketplace. It is also the most actively regulated jurisdiction with a coordinating body (the National Association of Insurance Commissioners) that develops model laws that may be adopted by individual states.
The European Union is the second largest marketplace. It has a coordinating body (the European Commission) that develops legislative directives that must be implemented by member countries.
China, Japan, and Australia are respectively the third, fourth, and tenth largest marketplaces. They illustrate the variety that even single-country regulatory environments may exhibit regarding discrimination issues in insurance.
5. PROHIBITIONS BY LINE OF BUSINESS
In addition to jurisdiction, as emphasized by Avraham (Citation2018), insurance prohibitions vary greatly by line of business. It is notable that the markets in which rate regulation is most common––automobile insurance, health insurance, workers, compensation, medical malpractice, and homeowners insurance––are all markets in which insurance is mandatory or in which universal coverage is thought to be socially desirable (cf. Tennyson Citation2007).
In commercial lines of insurance such as general liability and professional liability, the policyholder is a firm. As a general rule, insurance rate regulation tends to be absent from commercial insurance. In part this is because firms typically have more resources than individuals and so do not suffer the same imbalance of information asymmetry as described in Subsection 3.1. As exceptions to this rule, medical malpractice and workers’ compensation continue to be highly regulated. These two lines provide coverages that tend to be mandated by government regulations. Even though highly regulated, because the purchasers of insurance are generally organizations, discrimination issues are not as relevant. In the following, we focus instead on personal insurance.
5.1. Unisex Risk Classification
The European insurance marketplace was rocked in 2011 when the European Court of Justice concluded that any gender-based insurance discrimination is prohibited (EU Citation2012). Prior to this ruling, gender was regularly routinely used for pricing insurance.
Broadly, what characteristics of this trait would lead society to prohibit its use for insurance discrimination? Referring to the Subsection 2.2 categories, sex at birth is certainly beyond the control of the insured. Insurers have economic motivation for using gender as a predictor because, for many lines of business, it exhibits helpful predictive abilities even though its causal nature can be debated. An important motivation for prohibiting gender as a rating variable is to limit the perpetuation of negative stereotypes, so that men and women would receive equal treatment in the access to and supply of all goods and services. Equality and respect for human dignity and human rights are among the core values of the EU, reflected in several EU directives targeting discrimination. Initially signed in 1957, Article 10 of the Treaty on the Functioning of the European Union states:
In defining and implementing its policies and activities, the Union shall aim to combat discrimination based on sex, racial or ethnic origin, religion or belief, disability, age or sexual orientation.
From the case of Los Angeles Department of Water v. Manhart (1978), employers cannot require women to make larger contributions to a pension plan in order to receive the same monthly benefits as similarly situated men.
From the case of Arizona Governing Committee for Tax Deferred Annuity v. Norris (1983), women cannot receive lower monthly benefits than men who had made the same contributions (this case was based on a defined contribution plan).
These decisions were based on the 1964 Civil Rights Act that prohibits employment discrimination because of an individual’s race, color, religion, sex, or national origin. In 1986, the Equal Employment Opportunity Commission broadened the Supreme Court’s prohibitions by forbidding sex-based differences in any employee benefit, even if justified by differences in cost.
Another major event that preceded the 2011 ruling was a European Union Council Directive in 2000 that prohibited discrimination based on racial and ethnic origin (EU Citation2000). Prior to this directive, some insurers required an additional premium based on the risk criterion of the applicant being a “foreigner” (cf. Schmeiser, Störmer, and Wagner Citation2014). The 2011 ruling was based on European Union Directive 2004/113/EC, (EU Citation2004; this earlier directive required equal treatment of men and women but provided an exception for the insurance industry). This ruling applies to all lines of insurance business; in contrast, for example, gender-based pricing of auto insurance is permitted in all but a handful of U.S. states (the exceptions being Hawaii, Massachusetts, Montana, North Carolina, Pennsylvania, and, as of 2019, California). As part of the guidelines on the application of the 2011 ruling, the use of risk factors that might be correlated with gender, as long as they are true risk factors in their own right, is still permitted.
The retirement systems line of business is interesting because gender is an important predictor of longevity (cf. Lemaire Citation2002). Women, in general, outlive men, so they receive pension benefits over a longer period of time. For other lines of business such as personal auto, it is possible to find variables that provide a suitable substitute for gender (cf. Ayuso, Guillén, and Pérez-Marín Citation2016). Section 6 explores the concept of proxy discrimination in more detail.
5.2. General Insurance and Credit-Based Scoring
An individual’s credit history has long been used in commercial lines of insurance and in life insurance. As described by Brockett and Golden (Citation2007, p. 24), “Although it has been known since at least 1949 that credit history is related to driving accidents, the advent of high capacity, high-speed computers has made massive personal credit files available, and has made it feasible to routinely use this credit information for predicting insurance losses in personal lines of property and casualty insurance.”
From the Federal Trade Commission and others (Citation2007), general insurers in the United States started to use credit history information for automobile pricing in the early 1980s. It became more routine with the development of Fair Isaac Corporation (FICO) scores in the mid 1990s, first for homeowners and then for auto. According to FICO, in the absence of state prohibitions, 95% of automobile insurers and 85% of homeowners insurers employ insurance scores in either the underwriting or rating process (NAIC Citation2021; see also Morris, Schwarcz, and Teitelbaum Citation2017).
Credit-based insurance scores, or simply insurance scores, are similar to widely known credit scores in that both rely upon an individual’s credit history. This credit history includes prior credit performance (e.g., late payments), current levels of indebtedness (e.g., bankruptcy), length of credit history (e.g., age of oldest account, average age of all accounts), pursuit of new credit (e.g., new accounts, mortgages), and types of credit used (e.g., department, travel, major bank credit cards). However, credit scores predict the risk of credit delinquency and so measure the financial well-being of a consumer. In contrast, an insurance score is designed to predict insurance losses and so assesses how well individuals manage their money.
Credit-based insurance scores summarize an individual’s personal financial history; they do not exhibit the characteristics described in Subsection 2.2 that would lead them to being described as sensitive or suspect. However, as emphasized by Morris, Schwarcz, and Teitelbaum (Citation2017), insurance scores are regulated because they potentially correlate with suspect classifications, in particular race and income. For this reason, in the United States, most states regulate insurers’ use of insurance scores in auto and home insurance, and a few states ban their use altogether (Avraham, Logue, and Schwarcz Citation2014; Avraham Citation2019).
Credit-based insurance scores provide a natural example where the ability to predict insurance losses is well established (statistical discrimination) but the causal nature is uncertain. As noted by Brockett and Golden (Citation2007), a poor credit score may not create (cause) an insured loss but it is a measure of underlying biological and psycho-behavioral traits that do affect insured losses. However, from Morris, Schwarcz, and Teitelbaum (Citation2017), this is inconsistent with the fact that two of the major drivers of credit risk are unemployment and health problems, neither of which seems to reflect irresponsible behavior such as reckless driving or lack of fire safety. So, although both sources re-affirm that credit-based insurance scores provide a sound platform for predicting insurance losses, the causal nature remains unclear.
5.3. General Insurance and Price Optimization
Technical prices, which are based on expected claims, provide the foundations for most lines of general insurance, at least on the noncommercial, or personal, side. Traditionally, informal judgment has been used to adjust technical prices to become market prices; these adjustments are (i) for consistency among factors, among plans, and over time; (ii) for competitors’ rates; and (iii) for impact on retention (cf. Casualty Actuarial Society Committee on Ratemaking Citation2014). Price optimization refers to a systematic approach for making adjustments to traditional cost-based technical prices that incorporates customer demand.
Technical prices can be thought of as based on single-period models that focus on costs of insurance including claims and expenses. In contrast, the price optimization approach incorporate models of retention as well as prices of competitors typically by looking over several periods. For example, if an insurer raises prices, then that insurer can expect lower retention; the amounts depend on how sensitive the consumer is to price changes and the availability of the same coverage from the competition. By looking over several periods, price optimization models can tune prices to achieve an insurer’s long-term goals, such as profitability or development of market share.
From an insurer’s point of view, the use of price optimization tools is simply a sound business practice that is widely adopted in many industries, including retail and travel. Price optimization moves insurance pricing beyond expected costs to behavior including price sensitivity. At the individual consumer level, it may be that an insurer prices differently two consumers with the same risk profile because their anticipated price sensitivity differs (cf. Subsection 3.3). Price sensitivity matters because it affects consumer retention and acquisition expenses for new business are generally higher than expenses for retaining a customer (cf. Casualty Actuarial and Statistical Task Force Citation2015).
However, consumers have taken a dim viewpoint of price optimization (see a summary in Casualty Actuarial and Statistical Task Force Citation2015). On the one hand, the ability to identify loyal customers suggests that these are the customers who would enjoy lower premiums because of the lower expenses associated with them. On the other hand, these are exactly the customers who are likely to stay (and remain loyal) when faced with price increases. Some critics argue that price sensitivity practices impose an unfair penalty on customer loyalty. Customers more likely to be loyal are less likely to shop for alternatives. Insurers will identify this tendency and so impose price increases on customers not for their tendency to have high claims but rather for their tendency to be loyal.
Other critics argue that price optimization has been developed to increase insurers’ profits by raising premiums on individuals who are less likely to shop around for a better price, and many of these people are low-income consumers. Consumer advocates assert that deviation from cost-based ratemaking through price optimization will disfavor those consumers with fewer market options, less market power, and less propensity to shop around, in particular, low-income and minority consumers. Thus, although insurers may be optimizing neutral objectives, the result of their actions can result in unintentional proxy discrimination.
Swayed by these arguments, many U.S. insurance state regulators have banned price optimization in personal lines insurance. As another example, price optimization and price discrimination are not illegal in Australia but do give rise to consumer unease and are being scrutinized by regulators (Emergency Services Levy Big Data Project Team Citation2018).
5.4. Life Insurance and Genetic Testing
Genetic testing involves a type of medical test that examines chromosomes, genes, or proteins. The results of a genetic test can confirm or rule out a suspected genetic condition or help determine a person’s chance of developing or passing on a genetic disorder. There are many different purposes for testing, including medical (such as diagnosing a genetic disease or predicting disease risk) and non-medical (such as confirming parentage or forensic investigation). Hundreds of genetic tests are currently in use and more are being developed; see, for example, Born (Citation2019).
Information from genetic tests is potentially sensitive. Following the structure in Subsection 2.2, the main reasons are because they are not under the control of an individual, nor, in most cases, do they change over time. Only in rare instances (such as Huntington’s disease) is a genetic condition known to cause an insured event such as death. When thinking about the standards of perpetuating negative stereotypes and historical precedence, the genetic tests themselves are new (and developing), so the historical impact of a specific test is minimal. Nonetheless, as noted by Avraham, Logue, and Schwarcz (Citation2014, p. 32), “Genetic discrimination in the context of health, life, and disability insurance immediately evokes Nazi Germany and its obsession with promoting the reproduction of more ‘genetically desired’ people and eliminating ‘genetically defective’ individuals.”
Statistical discrimination is another important attribute. Lehtonen and Liukko (Citation2011, p. 40) wrote, “At least for the time being, genetic information is in most cases neither statistically nor economically significant for risk assessment from the insurance companies’ point of view. The exceptions are the rare single-gene diseases, such as Huntington’s disease, which inevitably or very likely result in death.” As of this writing, this remains the case (cf. Vukcevic and Chen Citation2018); today, it is likely that including results from genetic testing will not materially alter an insurer’s prices. However, insurers believe that this will change over time; that is, predictive ability of genetic testing will increase over time and will become salient in at least the life, disability, critical illness, and long-term care insurance marketplaces.
Insurers worry about genetic testing information because of information asymmetry concerns. Like the purchase of life insurance, the decision to undergo genetic testing is voluntary. When a potential policyholder has information about his or her health that is not shared with the insurance company, this could lead to anti-selection, where poorer risks purchase more insurance and better risks purchase little or no insurance. From an insurer’s viewpoint, one solution would be to allow insurers to require genetic testing, just as they are allowed to evaluate other aspects (e.g., weight, hypertension, and so forth) of a person’s health. There is some evidence that prohibitions on using genetic testing information may materially affect insurer’s claim costs; see, for example Lombardo (Citation2018).
In policy debates, arguments have been made for the position that genetic information is special and must therefore be treated differently from other types of medical information (sometimes known as genetic exceptionalism). One way in which genetic testing differs from, for example, blood pressure, is through the impact it has on a person’s willingness to undergo the testing for fear of being denied life insurance. As summarized by Prince (Citation2019, p. 467), “Empirical evidence shows that fear of genetic discrimination has led individuals across the globe to refuse to participate in genetic research projects or to fail to undergo recommended clinical testing.” Nonetheless, this may be simply due to the evolving nature of the science of genetic testing. For an analogy to underscore this point, Born (Citation2019, p. 6) noted that “… over time, other types of medical tests – e.g., tests for cholesterol levels – were first considered controversial when initial evidence showed a wide variation in predicted value.”
The social impact depends on the line of insurance business. In health insurance, the impact of genetic testing is less pronounced because many leading countries in the world offer government-provided health insurance or mandate the purchase of health insurance. Even in the United States, which does not have government-provided health insurance, results of genetic testing are not permitted under the Genetic Information Nondiscrimination Act. This act prohibits covered health insurers (and employers) from discriminating on the basis of genetic information, which includes genetic test results, family medical history, and use of genetic services.
Some international conventions recommend restricting the use of genetic information for insurance purposes. The Council of Europe’s Convention on Human Rights and Biomedicine prohibits the performance of genetic testing as a condition for entering into an insurance contract (cf. Lehtonen and Liukko Citation2011). In 1997, the United Nations Educational, Scientific, and Cultural Organization issued a Universal Declaration on the Human Genome and Human Rights. This was followed by a 2003 declaration that argued that genetic data and biological samples should not be accessible by insurance companies, among other actors (cf. Prince Citation2019).
Though many countries have specific laws covering genetic testing, most of the regulations are not very detailed. summarizes genetic testing regulations, from Klein (Citation2017). Joly et al. (Citation2020) provides another perspective on cross-country comparisons. Not only the science but also the insurance regulation of genetic testing continues to evolve. Several jurisdictions have passed or are considering legislative changes in the use of genetic testing information in underwriting insurance. For example, Canada passed federal laws in 2017 banning the use of all genetic information for business purposes; see, for example, Lombardo (Citation2018). Within the United States, Born (Citation2019) documented recent proposals in the state of Florida. Further, in July 2020, Florida has passed a law that prohibits life insurers and long-term care insurers from canceling, limiting, or denying coverage or establishing differentials in premium rates based on genetic information. As noted in , Australia has been active in considering genetic testing legislation.
Table 1 Genetic Testing Prohibitions by Country
5.5. Big Data
As with all institutions, insurers are redefining how they do business with the increasing capacity and computational abilities of computers, availability of new and innovative sources of data, and advanced algorithms that can detect patterns in insurance activities that were previously unknown. Subsections 6.3 and 6.4 summarize how these advanced algorithms can be used to mitigate discrimination issues.
Conceptually, Big Data does not alter the fundamental issues of insurance discrimination. This point was emphasized in Swedloff (Citation2014). One can think of credit-based scoring and price optimization as simply forerunners of a long-term trend by insurers to gather more and more data about their current and potential customers. One might hope that machine-driven algorithms would eliminate human biases but, as documented by Barocas and Selbst (Citation2016), these algorithms inherit the prejudices of authors of the algorithms and prior decision makers. As another example, Bartlett et al. (Citation2019) found that the use of algorithmic decision making for mortgage loans in the United States results in significant proxy discrimination again Latinx and African American borrowers but significantly reduces discriminatory practices of face-to-face lenders.
Yet, Big Data is changing how insurers do business. With respect to insurance discrimination, Swedloff (Citation2014) argued that the two main aspects of change are privacy and proxy discrimination. On the privacy front, some of this detailed information is provided voluntarily by individuals to insurers and suggests to some that it not be treated as sensitive. This includes information from Global position Systems that we put in our cars that underpin telematics, comparable devices for our homes (the Internet of Things), devices that we wear to improve our health, and so forth.
Still, insurers may also use other information that is not provided directly by individuals. Privacy issues are raised any time a carrier classifies risks on intimate, personal information, like HIV status, marital status, sexual orientation, or genetic information. Although not an insurance case, Swedloff (Citation2014) discussed the highly publicized event where Target, a large U.S. department store, used analytics to predict which of its customers were pregnant. This information was passed on to the marketing arm, which sent coupons for maternity clothing, nursery furniture, and the like, to women who were likely to be expecting a child. That is, without asking any customers about their pregnancy status or harvesting that data in particular, Target was able to predict extremely sensitive and personal information about its customers. Consumer advocates fear that similar information, such as where we go, movies that we watch, and telephone calls and texts that we make, would be of interest to insurers ostensibly to be used for understanding consumers’ attitudes toward risk and the likelihood of making insurance claims.
Proxy discrimination, introduced in Subsection 2.3, occurs when a surrogate, or proxy, is used in place of a prohibited trait. As originally conceived, this proxy is a facially neutral trait, such as the size of an automobile’s engine being used as a proxy for gender. In the world of Big Data, an equally important issue is that complex algorithms are being developed using literally thousands of traits (in the simple Target example, the analyst used only 25 traits to develop an effective pregnancy score). Thus, as emphasized by Prince and Schwarcz (Citation2020), proxy discrimination may be unintentional; moreover, the insurer may not even be aware that it is engaging in discriminatory behavior due to the opaqueness of machine-driven algorithms. Proxy discrimination is particularly important for actuaries and so is further discussed in Section 6.
Although consumers may benefit from a marketplace where insurers can more accurately assess risks, there is also a potential loss of transparency in insurance pricing. There already exists a low level of consumer understanding and a low level of consumer engagement with insurance purchases. More complex data algorithms will impede efforts at transparency. As discussed by Richman, von Rummell, and Wuthrich (Citation2019), machine learning models are often more complex and less transparent than traditional models. Moreover, massive data sets and complex models do not make life easier for regulators. Insurance regulators need to review rating plans that incorporate complex predictive models. Many jurisdictions do not have sufficient in-house actuarial expertise to review such filings.
For a final note on Big Data, some have expressed a concern that highly individualized or personalized rates lose the benefit of risk pooling (e.g., Emergency Services Levy Big Data Project Team Citation2018; Meyers and van Hoyweghen Citation2018). Although this could be a problem for some portfolios, most insurance systems are based on diversification of pools of unrelated (independent) risks. This type of diversification does not go away when the risks are different as long as they are priced properly.
5.6. COVID-19
A pandemic is a global outbreak of disease, and in early 2020 the world saw the onslaught of a new coronavirus dubbed COVID-19. As with other parts of the global economy, the disease has rocked the insurance industry. The lines of business most affected on the commercial side include workers’ compensation, business interruption insurance, cyber liability, general liability, and event cancellation, as well as health and travel insurance on the personal side; see, for example, Fannin Citation2020). Interestingly, automobile insurance claims have dramatically reduced (for the first part of 2020 at the time of this writing) due to travel restrictions; people are driving less and getting into fewer accidents.
Prohibiting Insurance Discrimination Based on COVID-19 Diagnosis
Insurance legislation is being introduced to prohibit discrimination based on the diagnosis of this disease. For example, the state of Wisconsin passed a law on April 15, 2020, that includes the following:
This bill prohibits insurers that offer an individual or group health benefit plan, pharmacy benefit managers, or self-insured governmental health plans from doing any of the following based on a current or past diagnosis or suspected diagnosis of COVID-19: establishing rules for the eligibility of any individual, employer, or group to enroll or remain enrolled in a plan or for the renewal of coverage under the plan; cancelling coverage during a contract term; setting rates for coverage; or refusing to grant a grace period for payment of a premium that would generally be granted.
More broadly, this legislation has several implications. For example, in the absence of this legal restriction, rates may well increase for grocery store workers, due to their exposure and increased suspicion of a diagnosis of COVID-19. Is this in the best interest of society? To sharpen understanding of the social and economic considerations presented in Sections 2 and 3, we now reflect on these principles in terms of COVID-19.
Social Justice Considerations of COVID-19 Insurance Prohibitions
By its very global nature, a pandemic brings out the social responsibility of an insurance pool described in Subsection 2.1.1. The line of business affected by COVID-19 may be viewed as social/public good (e.g., health insurance) or as an economic commodity (e.g., life insurance), but the responses to COVID-19 are certainly social. Just as when you build a dam it benefits everyone who lives in the area (not just those who make contributions toward building it), in the same way societal measures for disease prevention (e.g., social distancing) are borne by the entire population.
Subsection 2.2 introduced variable attributes that influence fairness for insurance purposes. For a COVID-19 diagnosis:
Control. Individuals have few controls as to whether they have disease symptoms due to its widespread impact on society.
Mutability. The variable may change over time but not in a good way.
Causality/Statistical Discrimination. For those who have recently contracted the disease, there is a known pathway to heightened mortality risk and health care costs. For those diagnosed with disease but who have recovered, there are no known additional risks to mortality or morbidity.
Limiting or Reversing the Effects of Past Prejudice. This is not an issue because COVID-19 is a new disease.
Inhibiting Socially Valuable Behavior. If insurers were allowed to rate based on disease symptoms, it is likely that many would refuse testing, which would inhibit scientific progress in addressing the disease, similar to genetic testing.
Proxy Discrimination. In the United States, it is known that COVID-19 affects African Americans more than other ethnic groups and that COVID-19 mortality rates are related to age. Thus, insurer discrimination based on contracting COVID-19 could be viewed as an indirect path to race and, where pertinent, age discrimination.
Economic Considerations of COVID-19 Insurance Prohibitions
Adverse Selection. It is likely that individuals would know whether they had symptoms of COVID-19 without going through formal testing, unknown to the insurer. This creates the potential for adverse selection.
Competition. It is unlikely that any insurer will have private knowledge about the nature of the COVID-19 disease, suggesting that marketplace competition is not an issue. However, some insurers may elect to pull out of the marketplace (such as with travel insurance), meaning that lack of supply may increase prices.
Price Discrimination. This is not likely to be an issue with COVID-19.
Summary of COVID-19 Insurance Prohibitions
For a pandemic, the weight of evidence suggests that societal concerns dominate and that a prohibition based on diagnosis, real or suspected, of COVID-19 is warranted. Because insurers lack data about its predictive abilities, it is unlikely that competition will be affected. Prohibitions of this nature increase consumer confidence in the insurance system. It is hoped that at a not too far date in the future, COVID-19 will lose its pandemic status and become another disease that we have to deal with. At that time, special legislative actions for COVID-19 will lose their appeal.
6. PROXY DISCRIMINATION
Proxy discrimination, when a seemingly innocuous variable is correlated with a protected variable, can be a problem because it produces the same outcomes that would be obtained in the absence of restrictions based on directly predictive traits (cf. Prince and Schwarcz Citation2020). This is true whether or not the surrogate is opaque to the insurer and the regulator.
6.1. Strategies for Mitigating Proxy Discrimination
Historically, the focus has been on introducing regulation that prohibits the use of protected variables, such as race, or surrogates thought to be proxies for protected variables, such as credit-based insurance scores. More recently and providing a greater challenge is how to mitigate proxy discrimination when the proxies are produced by opaque machine learning algorithms based on many variables. There are several strategies that policymakers can use to limit this latter type of discrimination, none of which are ideal.
Community Rating. At one end of the spectrum, proxy discrimination can be completely eliminated by removing the insurer’s ability to discriminate entirely. This is the case in community-rated plans where all policyholders pay the same price such as is common in social insurance schemes.
Approved Variables. Another option is to specify variables that may be used instead of variables that may not be used. This is the strategy taken in the U.S. individual health insurance market under the Affordable Care Act. Specifically, insurers may vary rates based on only four factors: (1) whether a plan covers an individual or family, (2) geographic area, (3) age, and (4) smoking status. As described by Prince and Schwarcz (Citation2020), the Affordable Care Act prohibits discrimination on the basis of prior health history, pre-existing conditions, and sex.
Actuarial Justification. A third alternative is to restrict the use of protected variables, such as race, religion, and political affiliation, and to further limit the use of rating variables to only those that are actuarially justified; that is, statistically discriminatory. This is the case of the U.S. rules on unfair discrimination where variables induce price differentials that “reflect equitably the differences in expected losses and expenses.” There are usually data disclosure requirements for actuarial justification. For example, in the case of insurance based on age discrimination in Australia, “the Commission and the President can require the disclosure of the source of the actuarial or statistical data.” And for the case of insurance based on sex discrimination in Australia, there are clients-related data disclosure provisions; see the online supplement for further details.
Limited Prohibitions. A fourth strategy is to only restrict the use of protected variables (including their proxies) such as gender. This is the model followed by the European rules. However, as noted in the online supplement, European regulation permits the use of risk factors correlated with gender as long as they are risk factors in their own right.
No Restrictions. At the other end of the spectrum, an option is to have no prohibitions. This is the case for most lines of commercial insurance.
Within this broad spectrum, there are many variations that regulators could consider particularly for the third and fourth strategies that permit some insurer discretion. One possible solution is to focus on transparency-oriented reforms that require insurers to disclose information on how their algorithms are working and possibly the sources of their data.
6.2. Linear Model Strategies
Another possible solution is to require insurers to use only variables that contain no protected information. To see how this might work in practice, consider data that we can represent as y, an outcome of interest such as an insurance claim and a set of predictor or rating variables. Further decompose the rating variables into components, those that are permitted, or not protected, by the regulators XNP (nonprotected variables) and those that are potentially contentious or protected, XP. Following the work of Aseervatham, Lex, and Spindler (Citation2016), we can consider y to be an auto insurance claim, XP an indicator for gender, and XNP to be a collection of other variables that includes age, type of car, location, and so forth.
In the absence of regulatory restrictions, the actuary would consider all variables. For ease of interpretation, consider a “full” linear regression model, with predictors of the form
(1)
(1)
In the presence of regulatory restrictions, the actuary could consider a restricted model,
with predictors of the form
(2)
(2)
For a sensitive variable XP to be the subject of contention, it often is correlated with an outcome y. So, one might expect for there to be a drop in the predictive ability when moving from the full information predictors
to the restricted ones,
In point of practice, often there is a strong relationship of XP with the other predictor variables XNP. When XP is dropped, the other variables serve as proxies for the omitted variable. For example, in their study, Aseervatham, Lex, and Spindler (Citation2016) found that this was largely the case, with the interesting exceptions being for younger and older drivers. As another point of practice, if a variable is dropped (such as gender), then it is likely that insurers may seek to incorporate new variables that also serve as proxies (“gender-like”) for the protected variables.
When a variable is dropped, the impact of the other factors changes, as quantified by the regression coefficients moving from b2 to This is not always desirable, so to mitigate this drawback, Pope and Sydnor (Citation2011) proposed an alternative predictor
(3)
(3)
where
is the average over the protected variables. Here, the coefficients b0, b1, and b2 are from the full model. These predictors are blind to the protected variables in that two individuals who differ only in their protected characteristics will receive the same predicted value from the model. However, as with
and
can be correlated with the protected variables. In other words, the average predicted values will vary across protected groups because of differences in other characteristics across groups. Please note that the Pope-Sydnor model is not restricted to linear models, as shown in Lindholm et al. (Citation2020).
As another option, there may be instances when the actuary would like to have a rating scheme that is totally unrelated to any sensitive or protected variables. For example, one can imagine using only that information that is uncorrelated to a set of protected variables under contention.
To this end, create a set of variables that are uncorrelated to XP by defining the projection matrix
and the transformed variables
Then, with the new variables, one uses the usual least squares procedures to get
Some standard matrix algebra shows that
that is, the regression coefficients from the transformed variables equal the regression coefficients in the full model. See, for example, Frees (Citation2009, p. 141). From this, the predictors are
(4)
(4)
By construction, these predictors are uncorrelated with the sensitive, protected variables.
6.3. Empirical Example
To see how these strategies might work in a real insurance context, we analyze 4,624 claims from Australian automobile insurance drawn from De Jong and Heller (Citation2008). So that our work can be easily replicated, we use the data from the R package CASdatasets, which slightly differs from de Jong and Heller in the coding of the variables. Statistical code, using the freely available software R, is provided in the online supplement (Frees and Huang Citation2021).
For this analysis of claims severity, ClaimAmount, we focus on a potential protected variable, Female, indicating if the policyholder is female. Other variables relevant to claims severity for these data are VehValue, the vehicle value in thousands of Australian dollars, and DrivAge, the age and employment status of the policyholder. A preliminary examination of the data (not included here but available, for example, in De Jong and Heller Citation2008), shows that the distribution of claims is skewed. From this and customary industry practice, we fit a gamma distribution with a logarithmic link. The analysis summarized in shows that Female is an important predictor of claim amount.
Table 2 Gamma Regression Model 1 Summary
This model could be readily used for predicting claims severity. For illustrative purposes, the left-hand panel of shows the distribution of fits for the same portfolio of policyholders used to fit the data; the right-hand panel shows the distribution by gender. To address this, the model was refit excluding gender and the fitted values are labeled as Model 2. As another alternative, we fit a model using the orthogonalized versions of the VehValue and DriveAge variables, making each variable uncorrelated with Female. This results in Model 3. Then, we developed a proxy for the probability of being female, using automatic variable selection techniques, with VehValue and DriveAge as inputs but also including additional variables in the dataset that were not helpful predictors of ClaimAmount. We added this predictor to Model 2 and to Model 3, resulting in Models 4 and 5, respectively. Finally, we developed the Pope-Sydnor predictors from Model 1 the results of these fits are labeled as Model 6. This development is detailed in the online supplement.
summarizes results from the first five models (the repetition of Model 1 is included for comparison purposes). From Model 1, females have significantly lower claims. Similarly, from Models 4 and 5, a higher probability of being female implies a lower expected claim amount. From the Akaike information criterion goodness of fit statistics, Model 1 is the best fit. Models 2 and 3 are similar and exhibit a markedly worse fit than Model 1. It is interesting that Model 3 does not perform that much worse than Model 2; that is, removing effects of gender from the other predictor variables does not do that much damage to the overall model fit. Models 2 and 3 are significantly improved by including the proxy for being female, as shown in Models 4 and 5.
Table 3 Gamma Regression Model Summary
shows the means of the fitted values by gender under each model, including the Pope-Sydnor predictors. These fits are rescaled so that they have the same mean, thus promoting comparability. expands upon this by showing the corresponding distributions. The base Model 1 displays the biggest discrepancy between male and female distributions, suggesting that corresponding prices calculated using this procedure would be the largest among the alternatives considered. Model 2 is the only fitting procedure that does not require knowledge of the protected variable Female. Fits from Model 3 are very similar to those of Model 2 (it turns out that the Spearman correlation between fits is 0.994); further, Model 3 is attractive because it only uses predictors that are uncorrelated with the protected variable Female. Model 4 uses the same base variables as Model 1 but replaces the protected variable with a proxy. Coefficients of the proxy are determined using the protected variable but once the coefficients have been determined, the proxy depends only on known covariates, not the protected variable. As with the comparison between Models 2 and 3, Model 5 is similar to Model 4 but uses only covariates uncorrelated to the protected variable (in addition to the proxy variable). Using Model 6, two individuals who differ only in gender will have the same fitted values from the model. However, it does not ensure equal values across the protected groups (two genders) as shown .
Table 4 Comparison of Means by Predictors and Gender
6.4. Machine Learning Approaches
Recent years have seen an increasing trend in using big data and machine learning techniques in various actuarial practices, as introduced in Subsection 5.5. They are usually praised for superior out-of-sample forecasting performance but can also be opaque in insurance discrimination. Mehrabi et al. (Citation2019) identified two potential sources of unfairness in machine learning: biases in data and algorithms. Data (especially big data) can be heterogeneous and create bias in many different ways, which may lead to unfair results when a model learns from biased data. Algorithms may also lead to unfair decisions even when data are unbiased. To the best of our knowledge, there has been limited research in the actuarial/insurance literature discussing how to measure and manage discrimination using machine learning approaches. For example, Loi and Christen (Citation2019) provided an ethical analysis of private insurance discrimination and fairness in machine learning. They distinguished morally permissible and impermissible forms of statistical discrimination in private insurance and derived some ethical implications for the use of machine learning techniques in the insurance context.
Although the motivating applications are typically not insurance related, the machine learning field has seen an explosion of research on fairness. See, for example, the survey papers by Zliobaite (Citation2015), Romei and Ruggieri (Citation2014), Mehrabi et al. (Citation2019), and Chouldechova and Roth (Citation2018). This literature provides discussions and debates on how to define fairness of predictive models and how to measure the performance in terms of discrimination. For example, Kleinberg, Mullainathan, and Raghavan (Citation2016) formalize, three core fairness conditions in algorithmic classification that correspond to notions of fairness: calibration within groups, balance for the negative class, and balance for the positive class. They found that except in highly constrained cases, there is no method that can satisfy the three conditions simultaneously. The results suggest thinking about the trade-offs between the notions of fairness.
Following Mehrabi et al. (Citation2019), we summarize three ways for discrimination prevention: preprocessing, in-processing, and postprocessing. Data preprocessing removes the discrimination information from the historical data (target or input variables) and then applies regular machine learning approaches for model estimation; see, for example, Kamiran and Calders (Citation2012) and Calders and Žliobaitė (Citation2013). Most of the linear model strategies introduced in Subsection 6.2 belong to this category. In-processing techniques modify the learning algorithms by incorporating changes into the objective function or adding additional constraints to remove discrimination in the model learning phase; see, for example, Kamishima et al. (Citation2012). Postprocessing modifies a fitted regular model to remove discrimination. For example, Kamiran, Calders, and Pechenizkiy (Citation2010) postprocessed decision trees with discrimination-aware pruning and relabeling of tree leaves. The discrimination-free pricing model introduced in Pope and Sydnor (Citation2011) also belongs to this category.
Machine learning approaches generally require knowledge of both protected and nonprotected variables. However, for legal or commercial reasons, organizations (including insurers) may not hold data on protected variables, such as gender, race and ethnicity, which poses challenges to mitigating discrimination (cf. Miller Citation2009). Veale and Binns (Citation2017) introduced and discussed three potential approaches to deal with this problem, including (1) having trusted third parties to store data necessary for incorporating fairness constraints in modeling, (2) building collaborative online platforms to allow diverse organisations to share and access knowledge required to promote algorithmic fairness, and (3) using unsupervised learning and pedagogically interpretable algorithms to incorporate fairness hypotheses for further selective testing and exploration.
Despite the explosion of interest and volume of work that has been produced and published in recent years, the theory and application of discrimination-aware machine learning is still in a nascent state, especially in the context of insurance practice.
7. CONCLUSIONS
Understanding the insurance prohibitions landscape is important for actuaries and other financial analysts. Actuaries are heavily involved in setting of insurance prices. They are also often influential in determining the scope of insurance contractual coverages as well as whom the company insures, both initially and at renewal. Our position is not that actuaries should dictate whether or not use of information should be restricted or prohibited. Rather, choices regarding insurance prohibitions involve policy choices that should also involve legal and economic scholars, as well as government representatives and advocates for the industry and for consumers. Actuaries can make important contributions to these discussions by quantifying the financial impact of policy alternatives. This article helps actuaries to present financial cost recommendations in a meaningful way by summarizing different perspectives that other participants may entertain when considering insurance prohibitions.
Discussions on this article can be submitted until October 1, 2023. The authors reserve the right to reply to any discussion. Please see the Instructions for Authors found online at http://www.tandfonline.com/uaaj for submission instructions.
Online Supplement to: The Discriminating (Pricing) Actuary
Download PDF (423.3 KB)ACKNOWLEDGMENTS
The authors thank Anthony Asher, Junhao Liu, Xu Shi, Anya Prince, Michael Powers, Merle Weiner, Mario Wüthrich, and two anonymous reviewers for their numerous and insightful remarks.
FUNDING
The authors acknowledge the financial support received from the Research School of Finance, Actuarial Studies and Statistics, Australian National University. F. Huang’s work for this article was mainly done during her employment at the Australian National University.
REFERENCES
- Adams, P., R. Baker, S. Hunt, D. Kelly, and A. Nava. 2015. Encouraging consumers to act at renewal: Evidence from field trials in the home and motor insurance markets. Financial Conduct Authority Occasional Paper 12. https://www.fca.org.uk/publication/occasionalpapers/occasional-paper-12.pdf.
- Arizona Governing Committee for Tax Deferred Annuity and Deferred Compensation Plans versus Norris. 1983. Supreme Court decision 463 (No. 82-52):1073.
- Arrow, K. J. 1963. Uncertainty and the welfare economics of medical care. The American Economic Review 53, 941–73.
- Aseervatham, V., C. Lex, and M. Spindler. 2016. How do unisex rating regulations affect gender differences in insurance premiums? The Geneva Papers on Risk and Insurance-Issues and Practice, 41 (1):128–60.
- Avraham, R. 2018. Discrimination and insurance. In The Routledge handbook of the ethics of discrimination, ed. K. Lippert-Rasmussen, 335–347. Abingdon: Routledge. https://papers.ssrn.com/sol3/papers.cfm?abstract_id=3089946.
- Avraham, R. 2019. Database of state tort law reforms (6.1). University of Texas School of Law, Law and Economics Research Paper e555. https://papers.ssrn.com/sol3/papers.cfm?abstract_id=902711.
- Avraham, R., Logue, K.D. and Schwarcz, D. 2014. Towards a universal framework for insurance anti-discrimination laws. Connecticut Insurance Law Journal 21, 1.
- Ayuso, M., M. Guillén, and A. M. Pérez-Marín. 2016. Telematics and gender discrimination: Some usage-based evidence on whether men's risk of accidents differs from women's. Risks 4 (2):1–10. doi:10.3390/risks4020010
- Baker, T. 2002. Risk, insurance, and the social construction of responsibility. In Embracing risk: The changing culture of insurance and responsibility, ed. T. Baker and J. Simon, 33–51. Chicago: The University of Chicago Press.
- Barocas, S., and A. D. Selbst. 2016. Big data's disparate impact. California Law Review 104:671–732.
- Bartlett, R., A. Morse, R. Stanton, and N. Wallace. 2019. Consumer-lending discrimination in the FinTech era. National Bureau of Economic Research. http://www.phd-finance.uzh.ch/dam/jcr:139b84f5-afb8-40ac-b255-83b5386431ff/FS_spring18_paper_Morse.pdf.
- Bauer, D., R. D. Phillips, and G. H. Zanjani. 2013. Financial pricing of insurance. In Handbook of insurance, ed. G. Dionne, 627–45. New York, NY: Springer.
- Blackmon, B. G., and R. Zeckhauser. 1991. Mispriced equity: Regulated rates for auto insurance in Massachusetts. The American Economic Review 81 (2):65–69.
- Born, P. 2019. Genetic testing in underwriting: Implications for life insurance markets. Journal of Insurance Regulation 38 (5):1–18.
- Borselli, A. 2011. Insurance rates regulation in comparison with open competition. Connecticut Insurance Law Journal 18:109–68.
- Braun, A., H. Schmeiser, and P. Rymaszewski. 2015. Stock vs. Mutual insurers: Who should and who does charge more? European Journal of Operational Research 242 (3):875–89.
- Brockett, P. L., and L. L. Golden. 2007. Biological and psychobehavioral correlates of credit scores and automobile insurance losses: Toward an explication of why credit scoring works. Journal of Risk and Insurance 74 (1):23–63. doi:10.1111/j.1539-6975.2007.00201.x
- Calders, T., and I. Žliobaitė. 2013. Why unbiased computational processes can lead to discriminative decision procedures. In Discrimination and privacy in the information society, ed. B. Custers, T. Calders, B. Schermer, and T. Zarsky, 43–57. Berlin, Heidelberg: Springer.
- Casualty Actuarial and Statistical Task Force. 2015. Price optimization white paper. Kansas City, Missouri, United States: National Association of Insurance Commissioners. https://www.naic.org/documents/committees_c_catf_related_price_optimization_white_paper.pdf.
- Casualty Actuarial Society Committee on Ratemaking. 2014. Price optimization overview. http://www.casact.org/area/rate/price-optimization-overview.pdf.
- Cather, D. A. 2018. Cream skimming: Innovations in insurance risk classification and adverse selection. Risk Management and Insurance Review 21 (2):335–66. doi:10.1111/rmir.12102
- Chouldechova, A., and A. Roth. 2018. The frontiers of fairness in machine learning. arXiv preprint arXiv:1810.08810.
- De Jong, P., and G. Z. Heller. 2008. Generalized linear models for insurance data. Cambridge, UK: Cambridge University Press.
- Dionne, G., and C. Rothschild. 2014. Economic effects of risk classification bans. The Geneva Risk and Insurance Review 39 (2):184–221. doi:10.1057/grir.2014.15
- Emergency Services Levy Big Data Project Team. 2018. Monitoring of insurance premiums in the world of big data. www.eslinsurancemonitor.nsw.gov.au.
- European Union. 2000. Council directive 2000/43/EC. Official Journal of the European Communities L180, 43:22–26. https://eur-lex.europa.eu/legal-content/EN/TXT/?qid=1578080397727&uri=CELEX:32000L0043.
- European Union. 2004. Council Directive 2004/113/EC of 13 December 2004 implementing the principle of equal treatment between men and women in the access to and supply of goods and services. Official Journal of the European Union L373, 47:37–43. https://eur-lex.europa.eu/legal-content/EN/TXT/?uri=celex%3A32004L0113.
- European Union. 2012. Guidelines on the application of Council Directive 2004/113/EC to insurance, in the light of the judgment of the court of justice of the European Union in case c-236/09 (Test-Achats). Official Journal of the European Union C11, 55:1–11. https://eur-lex.europa.eu/legal-content/EN/TXT/?uri=celex%3A52012XC0113%2801%29.
- Fannin, B. A. 2020. COVID-19: The property-casualty perspective. Casualty Actuarial Society. https://www.casact.org/sites/default/files/2021-03/COVID-19_The_PC_Perspective_3-27-2020.pdf.
- Federal Trade Commission and others. 2007. Credit-based insurance scores: Impacts on consumers of automobile insurance. In A report to Congress by the Federal Trade Commission.
- Financial Conduct Authority. 2019. General insurance pricing practices: Interim report. Annex 3: International comparisons. Financial Conduct Authority MS 18/1. 2. https://www.fca.org.uk/publication/marketstudies/ms18-1-2-interim-report.pdf.
- Frees, E. W. 2009. Regression modeling with actuarial and financial applications. Cambridge University Press. https://finance.nankai.edu.cn/_upload/article/files/c6/44/73f40f574e069868d74d52345ece/69e1f4b9-4aa4-48d2-8c63-3e205e73c749.pdf
- Frees, E. 2018. Loss data analytics. arXiv preprint arXiv:1808.06718. Retrieved from https://openacttexts.github.io/
- Frees, E., and Huang, F. 2021. “Online Supplement to: The Discriminating (Pricing) Actuary”. https://papers.ssrn.com/sol3/papers.cfm?abstract_id=3892473.
- Frezal, S., and L. Barry. 2019. Fairness in uncertainty: Some limits and misinterpretations of actuarial fairness. Journal of Business Ethics 1–10. doi:10.1007/s10551-019-04171-2
- Friedland, J. 2013. Fundamentals of general insurance actuarial analysis. Society of Actuaries.
- International Association of Insurance Supervisors (IAIS). n.d. Retrieved from https://www.iaisweb.org/home
- International Standards of Actuarial Practice (ISAP). n.d. International Actuarial Association. Retrieved from https://www.actuaries.org/iaa/IAA/Publications/ISAPs/IAA/Publications/05ISAPs.aspx?hkey=334b21a7-a3ac-4e0e-8294-3cbc755ab14a
- Joly, Y., C. Dupras, M. Pinkesz, S. A. Tovino, and M. A Rothstein. 2020. Looking beyond GINA: Policy approaches to address genetic discrimination. Annual Review of Genomics and Human Genetics 21:491–507. doi:10.1146/annurev-genom-111119-011436
- Kamiran, F., and T. Calders. 2012. Data preprocessing techniques for classification without discrimination. Knowledge and Information Systems 33 (1):1–33. doi:10.1007/s10115-011-0463-8
- Kamiran, F., T. Calders, and M. Pechenizkiy. 2010. Discrimination aware decision tree learning. Paper presented at the 2010 IEEE International Conference on Data Mining, 13–17 Dec. 2010, Sydney, NSW, Australia..
- Kamishima, T., S. Akaho, H. Asoh, and J. Sakuma. 2012. Fairness-aware classifier with prejudice remover regularizer. Paper presented at the Joint European Conference on Machine Learning and Knowledge Discovery in Databases Bristol, United Kingdom, September 23–27 September.
- Klein, R. 2017. Genetics and life insurance. A view into the microscope of regulation. Geneva, Switzerland: The Geneva Association. https://www.genevaassociation.org/research-topics/global-ageing/genetics-and-life-insurance-view-microscope-regulation.
- Kleinberg, J., S. Mullainathan, and M. Raghavan. 2016. Inherent trade-offs in the fair determination of risk scores. arXiv preprint arXiv:1609.05807.
- Lehtonen, T.-K., and J. Liukko. 2011. The forms and limits of insurance solidarity. Journal of Business Ethics 103 (1):33–44. doi:10.1007/s10551-012-1221-x
- Lemaire, J. 2002. Why do females live longer than males? North American Actuarial Journal 6 (4):21–37. doi:10.1080/10920277.2002.10596061
- Lindholm, M., R. Richman, A. Tsanakas, and M. V. Wuthrich. 2020. Discrimination-free insurance pricing. https://papers.ssrn.com/sol3/papers.cfm?abstract_id=3520676
- Loi, M., and M. Christen. 2019. Insurance discrimination and fairness in machine learning: An ethical analysis. https://papers.ssrn.com/sol3/papers.cfm?abstract_id=3438823.
- Lombardo, M. 2018. The impact of genetic testing on life insurance mortality. Society of Actuaries. https://www.soa.org/resources/research-reports/2018/impact-genetic-testing/.
- Los Angeles Department of Water and Power versus Manhart. 1978. Supreme court decision 435 (No. 76-1810), 702.
- Loss Data Analytics. Retrieved from https://openacttexts.github.io/
- Lukacs, P., L. Neubecker, and P. Rowan. 2016. Price discrimination and cross-subsidy in financial services. FCA Occasional Paper 22. https://www.fca.org.uk/publication/occasional-papers/op16-22.pdf.
- Maysami, R. C., and W. J. Kwon. 1999. An analysis of Islamic takaful insurance. Journal of Insurance Regulation 18 (1):109–132.
- McCarthy, D. D., and J. A. Turner. 1993. Risk classification and sex discrimination in pension plans. Journal of Risk and Insurance 60(1):85–104. doi:10.2307/253100
- Mehrabi, N., F. Morstatter, N. Saxena, K. Lerman, and A. Galstyan. 2019. A survey on bias and fairness in machine learning. arXiv preprint arXiv:1908.09635.
- Meyers, G., and I. van Hoyweghen. 2018. Enacting actuarial fairness in insurance: From fair discrimination to behaviour-based fairness. Science as Culture 27 (4):413–38. doi:10.1080/09505431.2017.1398223
- Miller, M. J. 2009. Disparate impact and unfairly discriminatory insurance rates. Paper presented at Casualty Actuarial Society E-Forum, Las Vegas, Nevada, March 9–11. https://citeseerx.ist.psu.edu/viewdoc/download?doi=10.1.1.376.2528&rep=rep1&type=pdf#page=290
- Morris, D. S., D. Schwarcz, and J. C. Teitelbaum. 2017. Do credit-based insurance scores proxy for income in predicting auto claim risk? Journal of Empirical Legal Studies 14 (2):397–423. doi:10.1111/jels.12151
- National Association of Insurance Commissioners. 2010. Property and casualty model rating law (file and use version). https://www.naic.org/store/free/GDL-1775.pdf.
- NAIC. 2021. Credit-based insurance scores. National Association of Insurance Commissioners. Retrieved from https://content.naic.org/cipr_topics/topic_creditbased_insurance_scores.htm
- Peto, R. 1994. Smoking and death: The past 40 years and the next 40. British Medical Journal 309 (6959):937–39. doi:10.1136/bmj.309.6959.937
- Pope, D. G., and J. R. Sydnor. 2011. Implementing anti-discrimination policies in statistical profiling models. American Economic Journal: Economic Policy 3 (3):206–31. doi:10.1257/pol.3.3.206
- Prince, A. E. R. 2019. Political economy, stakeholder voices, and saliency: Lessons from international policies regulating insurer use of genetic information. Journal of Law and the Biosciences 5 (3):461–94. doi:10.1093/jlb/lsz001
- Prince, A. E. R., and D. Schwarcz. 2020. Proxy discrimination in the age of artificial intelligence and big data. Iowa Law Review. https://papers.ssrn.com/sol3/papers.cfm?abstract_id=3347959.
- Richman, R., N. von Rummell, and M. V. Wuthrich. 2019. Believing the bot—Model risk in the era of deep learning. https://papers.ssrn.com/sol3/papers.cfm?abstract_id=3444833.
- Romei, A., and S. Ruggieri. 2014. A multidisciplinary survey on discrimination analysis. The Knowledge Engineering Review 29 (5):582–638. doi:10.1017/S0269888913000039
- Schmeiser, H., T. Störmer, and J. Wagner. 2014. Unisex insurance pricing: Consumers' perception and market implications. The Geneva Papers 39, 322–350.
- Schwarcz, D., and P. Siegelman. 2017. Law and economics of insurance. In The Oxford handbook of law and economics: Vol. 2. Private and commercial law, ed. Francesco Parisi, 481–508. New York, USA: Oxford University Press.
- Skipper, H. D., and R. W. Klein. 2000. Insurance regulation in the public interest: The path towards solvent, competitive markets. The Geneva Papers on Risk and Insurance-Issues and Practice 25 (4):482–504. doi:10.1111/1468-0440.00078
- Swedloff, R. 2014. Risk classification's big data (r) evolution. Connecticut Insurance Law Journal 21:339–373.
- Tennyson, S. L. 2007. Efficiency consequences of rate regulation in insurance markets. Networks Financial Institute Policy Brief 2007-PB, 03. https://www.indstate.edu/business/sites/business.indstate.edu/files/Docs/2007-PB-03_Tennyson.pdf.
- Thomas, R. G. 2012. Non-risk price discrimination in insurance: Market outcomes and public policy. The Geneva Papers on Risk and Insurance-Issues and Practice 37 (1):27–46.
- Thouvenin, F., F. Suter, D. George, and R. H. Weber. 2019. Big data in the insurance industry: Leeway and limits for individualising insurance contracts. Journal of Intellectual Property, Information Technology and E-Commerce Law 10 (2):209–243. https://www.jipitec.eu/issues/jipitec-10-2-2019/4916.
- Veale, M., and R. Binns. 2017. Fairer machine learning in the real world: Mitigating discrimination without collecting sensitive data. Big Data & Society 4 (2). doi:10.1177/2053951717743530
- Vukcevic, D., and J. Chen. 2018. Advances in genetics and their impact on life insurance. https://www.actuaries.asn.au/Library/Events/FSF/2018/VukcevicChenPaper.pdf.
- Werner, G., and C. Modlin. 2016. Basic ratemaking. 5th ed. Casualty Actuarial Society. https://www.casact.org/library/studynotes/werner_modlin_ratemaking.pdf.
- Zliobaite, I. 2015. A survey on measuring indirect discrimination in machine learning. arXiv preprint arXiv:1511.00148.