Abstract
Air quality impacts of volatile organic compound (VOC) and nitrogen oxide (NOx) emissions from major sources over the northwestern United States are simulated. The comprehensive nested modeling system comprises three models: Community Multiscale Air Quality (CMAQ), Weather Research and Forecasting (WRF), and Sparse Matrix Operator Kernel Emissions (SMOKE). In addition, the decoupled direct method in three dimensions (DDM-3D) is used to determine the sensitivities of pollutant concentrations to changes in precursor emissions during a severe smog episode in July of 2006. The average simulated 8-hr daily maximum O3 concentration is 48.9 ppb, with 1-hr O3 maxima up to 106 ppb (40 km southeast of Seattle). The average simulated PM2.5 (particulate matter with an aerodynamic diameter <2.5 μm) concentration at the measurement sites is 9.06 μg m−3, which is in good agreement with the observed concentration (8.06 μg m−3). In urban areas (i.e., Seattle, Vancouver, etc.), the model predicts that, on average, a reduction of NOx emissions is simulated to lead to an increase in average 8-hr daily maximum O3 concentrations, and will be most prominent in Seattle (where the greatest sensitivity is −0.2 ppb per % change of mobile sources). On the other hand, decreasing NOx emissions is simulated to decrease the 8-hr maximum O3 concentrations in remote and forested areas. Decreased NOx emissions are simulated to slightly increase PM2.5 in major urban areas. In urban areas, a decrease in VOC emissions will result in a decrease of 8-hr maximum O3 concentrations. The impact of decreased VOC emissions from biogenic, mobile, nonroad, and area sources on average 8-hr daily maximum O3 concentrations is up to 0.05 ppb decrease per % of emission change, each. Decreased emissions of VOCs decrease average PM2.5 concentrations in the entire modeling domain. In major cities, PM2.5 concentrations are more sensitive to emissions of VOCs from biogenic sources than other sources of VOCs. These results can be used to interpret the effectiveness of VOC or NOx controls over pollutant concentrations, especially for localities that may exceed National Ambient Air Quality Standards (NAAQS).
The effect of NOx and VOC controls on ozone and PM2.5 concentrations in the northwestern United States is examined using the decoupled direct method in three dimensions (DDM-3D) in a state-of-the-art three-dimensional chemical transport model (CMAQ). NOx controls are predicted to increase PM2.5 and ozone in major urban areas and decrease ozone in more remote and forested areas. VOC reductions are helpful in reducing ozone and PM2.5 concentrations in urban areas. Biogenic VOC sources have the largest impact on O3 and PM2.5 concentrations.
Introduction
Ground-level ozone (O3) and fine particulate matter (aerodynamic diameter <2.5 μm; PM2.5) are major constituents of urban and regional smog and are suspected of affecting human (CitationBell et al., 2005; CitationIto et al., 2005; CitationSchwartz et al., 1996; CitationZhou et al., 2011) and ecosystem health (CitationLikens et al., 1996; CitationMauzerall and Wang, 2001). Emissions of oxides of nitrogen (NOx) and volatile organic compounds (VOCs) drive a complex series of chemical and physical transformations that result in the formation of ozone and secondary particulate matter, and both ambient O3 and PM2.5 concentrations can be reduced by controls on emissions of NOx or VOCs, depending on which is the limiting precursor (CitationDodge, 1987; CitationSeinfeld and Pandis, 2006). Emissions from industrial facilities and electric utilities, motor vehicle exhaust, gasoline vapors, and chemical solvents are some of the major sources of NOx and/or VOCs.
The National Ambient Air Quality Standards (NAAQS) for 8-hr O3 and 24-hr PM2.5, set by the U.S. Environmental Protection Agency (EPA), are 75 ppb and 35 μg m−3, respectively (http://www.epa.gov/air/criteria.html), whereas the annual PM2.5 standard is currently 15 μg m−3. Moreover, the Canadian standard for daily maximum 8-hr average O3 concentration is set at 65 ppb and the 24-hr average PM2.5 standard is set at 30 μg m−3. Eulerian-grid-based air quality chemical transport models (CTMs) are typically used for understanding the factors leading to ozone and PM2.5 exceedances and to test emission control strategies to lower ozone and PM2.5 to meet standards. The Community Multiscale Air Quality model (CMAQ) (CitationByun and Schere, 2006) and the Comprehensive Air Quality model with extensions (CAMx) (CitationEnviron, 2003) have been applied and evaluated over different regions (CitationEder and Yu, 2006; CitationKarydis et al., 2007; CitationTesche et al., 2006; CitationTong and Mauzerall, 2006; CitationZhang et al., 2004, Citation2005). A few modeling studies (CitationBarna and Lamb, 2000; CitationBarna et al., 2000, Citation2001; CitationElleman and Covert, 2009; CitationJiang et al., 2003; CitationO'Neill and Lamb, 2005) have investigated ozone and aerosol production in the northwestern United States. Although the region is not generally known for having a photochemical smog problem, it has a history of episodes of elevated ozone (CitationBarna and Lamb, 2000). CitationBarna et al. (2000) investigated the air pollution in the Cascadia region of the Pacific Northwest, highlighting several features of this area that are different than those in other areas typically evaluated in ozone modeling studies, including (1) complex topographic and land–sea features; (2) an anthropogenic emission inventory that consists primarily of mobile sources, with relatively few large industrial point sources; (3) biogenic emissions dominated by coniferous forests; and (4) a predominant inflow consisting of relatively clean maritime air. CitationJiang et al. (2003) used process analysis to determine the relative importance of chemical production, advection, diffusion, and deposition to ozone concentration downwind from urban Seattle, Washington. This analysis showed that ozone concentrations increase during the day as chemical production exceeds the net effects of deposition and vertical diffusion but decrease after mid-afternoon when horizontal advection begins to dominate the other processes. Within the same study, the importance of VOC/NOx effects versus differences in meteorology was also considered. CitationBarna et al. (2001) investigated the sensitivity of ozone formation to VOC and NOx reductions within the Cascadia airshed of the Pacific Northwest. For this particular case, reductions in VOC emissions alone, or a combination of reduced VOC and NOx emissions, were generally found to be most effective, whereas reducing NOx emissions alone resulted in increased ozone in the Seattle area.
CTMs are widely used to calculate the response of ambient concentrations of various gaseous and aerosol pollutants in the atmosphere to changes in emissions (CitationBlanchard and Stoeckenius, 2001; CitationChock et al., 1999; CitationMendoza-Dominguez et al., 2000; CitationPun et al., 2008; CitationTsimpidi et al., 2007, Citation2008). The nonlinear relationship between ozone and secondary particulate matter components with their precursors suggests potential strategies for obtaining lower ambient concentrations, and the levels of control required are not directly known. The decoupled direct method in three dimensions (DDM-3D) has proven to be a powerful and efficient approach to identifying how sources impact ozone and particulate matter air quality for use in policy development (CitationDunker, 1984; CitationDunker et al., 2002; CitationMendoza-Dominguez et al., 2000; CitationOdman et al., 2002). DDM is found to be in good agreement with brute force emission reduction simulations when calculating the sensitivity of the 3D model to perturbations up to 30% from the base case (CitationDunker et al., 2002; CitationYang et al., 1997). DDM has been also used in CMAQ to estimate PM2.5 sensitivities and source impact analysis (CitationNapelenok et al., 2006), and for PM2.5 source apportionment (CitationBoylan and Russell, 2006; CitationKoo et al., 2009).
In this work, we investigate photochemical ozone and PM2.5 production in the Puget Sound region of the northwestern United States during a period with exceedances of the current 8-hr NAAQS for ozone. The Pacific Northwest is home to several large urban areas surrounded by forests, mountains, and agriculture areas. Recent high levels of O3 suggest that the area may be at risk of falling in nonattainment, particularly if the standard is tightened or if transported O3 increases background levels. Here CMAQ with DDM-3D is used to identify the impact of NOx and VOC sources on O3 and PM2.5 formation in the area. The analysis focuses on the parallel sensitivities of ozone and PM2.5 concentrations to mobile, nonroad, point, area, and biogenic sources of VOCs and NOx.
Modeling Approach
Air quality model
The Community Multiscale Air Quality model (CMAQ version 4.7) (CitationFoley et al., 2010) is run on various grid resolutions to simulate the transformation and fate of air pollutants. Gas-phase chemistry is modeled using the SAPRC-99 chemical mechanism (CitationCarter, 2000). CMAQ version 4.7 includes the following: (i) updates to the heterogeneous N2O5 hydrolysis parameterization (CitationDavis et al., 2008); (ii) improved treatment of secondary organic aerosol (SOA) formation (CitationCarlton et al., 2008, Citation2009, Citation2010); and (iii) a new treatment of gas-to-particle mass transfer for coarse aerosol (CitationKelly et al., 2010).
CMAQ is applied here using a nested-grids approach. The modeling domain uses a Lambert Conformal Projection centered at 40°N, 97°W with true latitudes of 33°N and 45°N. The innermost domain covers a 192 × 312-km region focusing on the Seattle area, with 4-km horizontal grid-spacing nests inside a 432 × 576-km region, with a 12-km horizontal grid-spacing domain that covers the state of Washington and portions of Oregon and British Columbia (). The outer domain uses a 36-km horizontal grid-spacing that covers the entire continental United States as well as portions of Canada and Mexico (5328 × 4032 km). All three grids have 13 vertical layers extending ∼15.9 km above ground, with seven layers below 1 km and the first layer is 18 m thick. Key features of the inner domains include rugged terrain and a complex coastline. The period modeled is 12–24 July 2006, which captures a pollution event when high O3 concentrations were observed across the region. The highest O3 level observed was 134 ppb at Mud Mountain, near Enumclaw (outside of Seattle, Washington), on 21 July. This period is characterized by high temperatures and relatively little cloud cover. The default profiles of CMAQ version 4.7 with slight revisions are used to prepare initial conditions (ICs) and boundary conditions (BCs) for the 36-km domain. Because the 36-km-resolution domain extends beyond most populated and industrial areas (except for some parts of Mexico), concentrations typical of a “clean” background atmosphere are used at the boundaries in the base simulation. The initial condition for O3 is 35 ppb and the value of the O3 concentrations at the boundaries of the 36-km domain is 35 ppb at the north and west boundaries, and 30 ppb at the south and east boundaries. The simulated coarse-grid species concentrations are used as initial and boundary conditions for the finer grids. DDM-3D is used to identify the impact of boundary conditions on the predicted O3 concentrations and found that boundary conditions contribute approximately 17 ppb to the predicted average O3 concentration in the Puget Sound region. The impact of initial conditions on the predicted ozone concentration in the Puget Sound area was found to be close to zero after a spin-up period of 2 days.
Figure 1. Top: Modeling domains with horizontal grid-spacing resolutions of 36 and 12 km, respectively. Bottom: Modeling domains with horizontal grid-spacing resolutions of 12 and 4 km, respectively. Black dots represent the location of the 4-km-domain ozone monitoring sites: (a) Issaquah, (b) North Bend, (c) Enumclaw, (d) Jackson Visitor Center, (e) Pack Forest, (f) Tahoma Woods Admin, (g) Casino Drive/North End, and (h) Custer/Loomis. Urbanized U.S. areas are shown in gray.
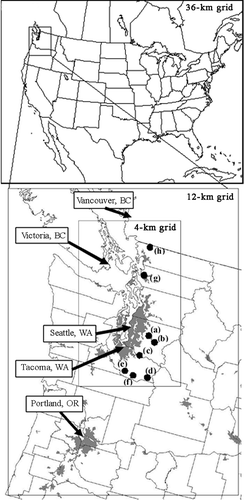
Sensitivity analysis method
The decoupled direct method in three dimensions (DDM-3D; CitationDunker, 1980) is used to calculate the seminormalized sensitivity, , of the ambient concentrations of a pollutant, to perturbations in an input parameter (e.g., emission rate, initial condition, or boundary condition):
DDM-3D has been successfully integrated into CMAQ by CitationCohan et al. (2005) and updated to include algorithms for computing particulate matter (PM) sensitivity coefficients (CitationNapelenok et al., 2006). Second-order sensitivities have also been successfully calculated by differentiating the governing sensitivity equations with respect to the parameter of interest (CitationHakami et al., 2003). Higher-order sensitivity coefficients are useful when considering large perturbations away from the base case, up to 50% (CitationHakami et al., 2004), whereas first-order sensitivities are typically accurate for up to 30% perturbations in input parameters (CitationDunker et al., 2002). Our focus is on calculating ambient O3 and PM2.5
to emissions of NOx and VOCs from area (small businesses, offices and residences, wild fires, dirt roads), biogenic (vegetation, soils), mobile (roadway vehicles), nonroad (construction, industrial and agricultural equipment, trains, airplanes), and point (factories, power plants, refineries, and other large facilities) source emission categories in CMAQ, as well as the impact of Canadian anthropogenic emissions on potential nonattainment areas.
Meteorological fields
The Weather Research and Forecasting model (WRF version 3.1.1) was used to generate meteorological fields for the 12–24 July 2006 episode. The configuration of physics schemes is as follows: long-wave Rapid Radiative Transfer Model (RRTM) (CitationMlawer et al., 1997) and Dudhia scheme (CitationDudhia, 1989) are used for long-wave and short-wave radiation, respectively; Yonsei University (YSU) scheme (CitationHong et al., 2006) is used for the planetary boundary layer; Noah scheme is used for land surface model (LSM); a revised version of Kain-Fritsch scheme (CitationKain and Fritsch, 1990) is used to represent the effects of both deep and shallow cumulus clouds; and the scheme proposed by CitationLin et al. (1983) is chosen as the microphysics option. A summary of the various schemes and their uses can be found CitationSkamarock et al. (2005). The initial and boundary conditions were taken from North American Mesoscale (NAM) model analysis products at a 12-km spatial resolution at 6-hr intervals.
WRF simulations use three nested grids with one-way nesting. The 36-, 12-, and 4-km domains extend over 165 × 129, 43 × 55, and 55 × 85 grids, respectively, with 40 layers vertically with the top pressure of 5000 Pa, and are chosen to overlap with the corresponding CMAQ model domains. Four-dimensional data assimilation grid nudging is employed for the 36- and the 12-km domain solutions. For the inner domain (4-km resolution), observational nudging is used. The 4-km model domain is dominated by several complex features and the meteorology within this region is strongly influenced by orographic effects and land water interactions (CitationMass, 1982). CitationBarna and Lamb (2000) recommended using observational nudging rather than other meteorological model configurations for predicting ozone spatial patterns over this region.
Simulated surface meteorological fields were examined against surface hourly observations from the United States and Canada (), with performance well within the typical range for air quality modeling (CitationEmery et al., 2001; CitationHanna and Yang, 2001). The WRF hourly output data were processed with the Meteorology-Chemistry Interface Processor (MCIP) version 3.4.1 for CMAQ. The highest predicted temperature during the simulation period occurred on 21 July 2006 (303 K). This is in agreement with measurements that also report the highest temperature on that day (not shown).
Table 1. Bias and error in WRF-generated meteorological parameter fields during 14–24 July 2006 with respect to the Techniques Development Laboratory (TDL) surface observations
Emission inventory
The Sparse Matrix Operator Kernel Emissions (SMOKE version 2.6) model (Carolina Environmental Program Citation[CEP], 2003) was used to generate hourly, gridded and speciated emissions for input to CMAQ. The emission inventory contains county-level emissions from different emission source categories for the contiguous United States, southern Canada, and northern Mexico. SMOKE simulations are based on the 2005-based modeling platform version 4.0 using the 2005 version 2 National Emissions Inventory (NEI) data (http://www.epa.gov/ttn/chief/emch/index.html#2005) and ancillary data for temporal, spatial, and chemical allocation of emissions. Within this version of the modeling platform, nonroad sources were simulated using the NONROAD 2004 model via National Mobile Inventory Model (NMIM). Mobile sources were simulated using MOBILE6 model via NMIM (not including refueling emissions). The Continuous Emissions Monitor (CEM) data for year 2006 were obtained from the EPA's Clean Air Markets Division and supplied to provide hourly emissions for electric generating utilities (EGUs) for 2006. The database contains the NOx and SO2 emissions that replaced the 2005 NEI NOx and SO2 emissions for EGU sources. Biogenic emissions were estimated by using the Biogenic Emissions Inventory System 3.14 (BEIS3.14) with the Biogenic Emissions Landcover Database 3.0 (BELD3), which includes 232 vegetation classes (except Canada and Mexico where only the standard 19 U.S. Geological Survey [USGS] categories are available). Canadian and Mexican anthropogenic emissions used in the SMOKE modeling are the 2006 National Pollutant Release Inventory (NPRI) and the 1999 Mexico National Emissions Inventory (MNEI), respectively, coming from the modeling platform too. A uniform monthly temporal profile is used for allocating emissions from wood-burning sources such as fireplace and wood fuel facilities in Canada. This means that these wood-smoke emissions are uniformly distributed throughout the year but, in reality, at least fireplaces are operated in winter, less likely in summer. SMOKE uses spatial surrogates (e.g., population and land use distributions), allocating the NEI's county-level emissions to the modeling grid cells. The resulting inventory consists of pollutants emitted from area, mobile, point, fire, ocean, biogenic, and agricultural sources ().
Table 2. Criteria air pollutants emissions summary in tons day−1 for the 4-km domain
CMAQ Predictions and Evaluation
The average domain-wide, simulated 8-hr daily maximum O3 concentration for the 14–24 July 2006 episode is 48.9 ppb (). High O3 concentrations during this episode are simulated south of the Seattle urban center over Pierce County with 1-hr O3 peak value of 106 ppb simulated at 4 p.m. local time on 21 July (). Time series of the observed and simulated surface O3 mixing ratios at eight monitoring sites in the region suggest that the calculation tracks the observations well, although peak levels are not fully captured (). The mean bias (MB), mean absolute gross error (MAGE), normalized mean bias (NMB), normalized mean error (NME), and the root mean square error (RMSE) were also calculated () to quantify the model skill:
Table 3. CMAQ ozone surface mixing ratios performance using surface observations from the continental United States for the episode of 14–24 July 2006
Figure 2. (a) Average simulated surface concentration of 8-hr daily maximum O3 during 14–24 July 2006. (b) Surface concentration of O3 at 11:00 p.m. (23:00) coordinated universal time (UTC) or 4:00 p.m. (16:00) local time (LT) on 21 July, when the overall maximum peak occurred.
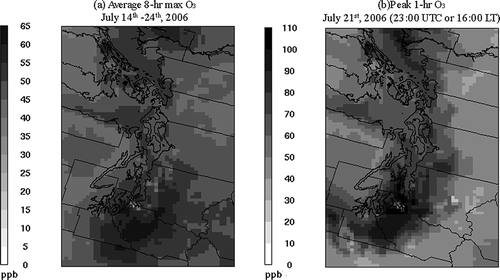
Figure 3. Time series of hourly observed (dotted line) and predicted (full line) ozone concentrations at the Puget Sound monitoring sites: (a) Issaquah, (b) North Bend, (c) Enumclaw, (d) Jackson Visitor Center, (e) Pack Forest, (f) Tahoma Woods Admin, (g) Casino Drive/North End, and (h) Custer/Loomis in coordinated universal time (UTC) during 14–24 July. The correspondence with the local time (LT) is LT = UTC − 7 hr.
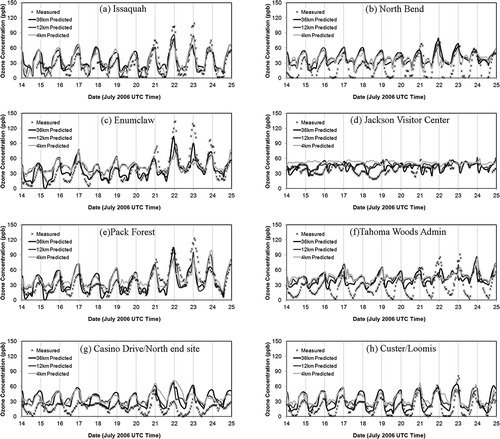
The average simulated O3 concentration over the observational stations in the 4-km resolution domain is 43.1 ppb, whereas the average measured value is 30.2 ppb. The NMB, excluding the observations that are below 40 ppb, is −7.9% compared with 42.7% when all the data points are taken into account. This difference indicates that the model has a low bias compared with high ozone levels but has a high bias at the low concentrations of ozone, which occur mainly during nighttime (). This overprediction during nighttime has to do with the difficulty in capturing the vertical diffusivity coefficient in the CMAQ model for application in this area (CitationZhang et al., 2006). EPA has established model performance goals for 1-hr ozone NMB and NME, for which the observed ozone is 60 ppb or greater, of ≤±15% and ≤35%, respectively (CitationEPA, 1991). These performance goals have been used for over two decades to assist in evaluating ozone models. Model performance for 1-hr ozone concentration over the 4-km domain is −7.9% (NMB) and 25.1% (NME), achieving the suggested performance goals. NMB and NME for the 12- and 36-km domains are also well within these limits (). It is worth mentioning that the cutoff point used in this study is 40 ppb, instead of 60 ppb proposed by EPA, because the O3 concentration in the Puget Sound region rarely exceeds the 60 ppb during the modeling episode. Similar performance has been reported by a large variety of CMAQ applications in different locations within the continental United States (CitationTong and Mauzerall, 2006; CitationZhang et al., 2006). Across these studies the tendency of CMAQ to underpredict the peak ozone values and overestimate the nighttime ozone concentration has been also highlighted. In individual sites, the best agreement is found in “Jackson visitor center” mountainous forest area (RMSE = 8.6 ppb). The model, however, tends to be biased high in the northern part of the domain. The MB and the RMSE in the “Casino Drive/North End” site are 9.9 and 14.7 ppb, respectively. The highest mean ozone concentration is observed in the Enumclaw area (38.5 ppb), where CMAQ tends to be biased slightly high until 20 July, whereas during the last 4 days the observed concentrations increase (up to 120 ppb), resulting in a low bias in ozone from the model. The overall MB in that area is 7.9 ppb.
The average simulated domain-wide PM2.5 surface concentration is 5.6 μg m−3, with the highest concentrations occurring around Vancouver (). Nevertheless, PM2.5 concentrations over Vancouver are overestimated due to the uniform monthly temporal profile used for Canadian wood-burning emission sources and uncertainties in Canadian biogenic emissions. The most abundant component of PM in the domain is organic matter (OM), accounting for 56% (3.14 μg m−3) of the PM mass during the simulated period (). Simulated OM concentrations tend to be higher in Canada, especially Vancouver and Victoria, than in the United States, possibly due to the difference in biogenic isoprene and monoterpene emissions estimated in the United States and Canada. This discrepancy is originated from the bulk land use description of Canadian forests, compared with the United States, used to prepare the biogenic emissions. Sulfate, soil, elemental carbon, ammonium, and nitrate account for 16% (0.89 μg m−3), 12% (0.65 μg m−3), 6% (0.36 μg m−3), 6% (0.35 μg m−3), and 3% (0.16 μg m−3) of total PM2.5 mass, respectively (–f), with the rest 1% (0.015 μg m−3) being sodium chloride transported from the ocean. Simulated and measured values of daily average surface PM2.5 concentrations are compared for every third day of the simulation period, beginning on 14 July (). The average simulated PM2.5 concentration during the days when measurements are available is 9.06 μg m−3, whereas the average measured concentration is 8.26 μg m−3. The NMB is 9.6%, indicating that the results are biased slightly high for PM2.5 concentrations. Speciated PM2.5 measurements were only available from two sites during 2 days within the simulated period (resulting only in four comparisons). According to these observations, the OM is indeed the most abundant component of PM in the domain, as predicted by the model, with sulfate being the second most important. The average measured concentrations of organic mass, sulfate, elemental carbon, ammonium, and nitrate were 7.4, 2.5, 0.9, 0.6, and 0.5 μg m−3, respectively, and the corresponding predicted MB were −0.8, −0.8, 0.15, −0.05, and −0.4 μg m−3.
Table 4. CMAQ PM2.5 surface concentrations (μg m−3) performance using surface observations for the episode of 14–24 July 2006
DDM Results
The first-order, seminormalized sensitivities calculated by DDM in this study represent the sensitivity of O3 and PM2.5 concentrations to small perturbations in NOx and VOC emissions. As such, first-order sensitivities describe the linear response of the model and are expressed in ppb per % for O3 and μg m−3 per % for PM2.5. Positive values represent a decrease in concentration after a 1% decrease of emissions and, correspondingly, negative values represent an increase. The response of O3 and PM2.5 concentrations to NOx and VOC emission changes can be calculated by multiplying the DDM coefficient by the magnitude of emission perturbation and is typically accurate for up to 30% perturbations (CitationDunker et al., 2002). The DDM results presented in this study express the impact of NOx and VOC emissions on the average 8-hr daily maximum ozone and 24-hr PM2.5 concentrations. This representation will be more useful to policymakers given that control strategies should focus on reducing the 8-hr daily maximum ozone and longer averages PM2.5 concentrations that contribute to NAAQS exceedances.
O3 sensitivity to NOx emissions
The average simulated sensitivity of 8-hr daily maximum O3 concentrations to total, domain-wide, NOx emission changes during 14–24 July 2006 in the Seattle urban area is about −0.27 ppb per % (), although with significant spatial variability throughout the modeling domain. This behavior is typical for urban areas, such as Seattle, which are characterized by high NOx-to-VOC ratios (NOx-saturated areas) (CitationDuncan et al., 2010), particularly in cooler areas with lower ultraviolet (UV) radiation fluxes. Similarly, reducing NOx emissions would increase O3 concentrations in Vancouver. On the peak ozone day (21 July), the highest 1-hr O3 sensitivity is observed in Tacoma (−1 ppb per %), which is located 40 km southwest of Seattle (). In Seattle, during the same day, the impact of NOx emissions on 1-hr O3 concentrations is predicted to be up to −0.3 ppb per %. Decreased NOx emissions would decrease 1-hr O3 concentrations in more remote and forested areas (i.e., 0.2 ppb per % close to Enumclaw where the maximum O3 concentration is observed). In such areas, biogenic VOC emissions during 21 July were high due to higher temperatures and solar insolation predicted by WRF, resulting in a low NOx-to-VOC ratio. In these NOx-limited areas, a control of NOx emissions would result in decreased O3 concentrations. Average sensitivities of 8-hr daily maximum O3 concentrations to changes of mobile, point, biogenic, nonroad, and area NOx emissions were calculated for the simulation period. 8-Hour daily maximum O3 concentrations in the Seattle urban area are found to be most negatively sensitive to mobile (up to −0.2 ppb per %) NOx emissions followed by point (up to −0.1 ppb per %) and nonroad (up to −0.05 ppb per %) sources (). Biogenic and area NOx emissions are predicted to have almost no impact on O3 concentrations (less than −0.01 ppb per %; not shown) because they correspond only to 5% of total NOx emissions (). Mobile NOx emissions impact the 8-hr daily maximum O3 concentrations over a large portion of the modeling domain (). On the other hand, point NOx emissions can affect 8-hr daily maximum O3 concentrations only in areas close to sources at Seattle and Tacoma as well as close to the refineries located on the west coast of Washington near the “Custer/Loomis” and the “Casino Drive/North End” monitoring sites (). 8-Hour daily maximum ozone sensitivities to Canadian anthropogenic emissions of NOx were also simulated (). On average, Canadian emissions of NOx have a negative impact on 8-hr daily maximum O3 concentrations around Vancouver (up to −0.2 ppb per %) but do not have a significant impact on 8-hr daily maximum O3 concentrations in Seattle during most of the simulated period (). However, during the peak ozone event on 21 July, Canadian NOx emissions is simulated to lead to a change of −0.05 ppb per % in 1-hr O3 concentration over Enumclaw, where the peak O3 concentrations were observed (). Back trajectories, using the Hybrid Single-Particle Lagrangian Integrated Trajectory (HYSPLIT) model of the National Oceanic and Atmospheric Administration (NOAA), also support this conclusion, because the air mass in Puget Sound region does not originate from Canada during the simulation period (except the period of 20–22 July 2006), particularly since ozone is very effectively transported over cooler water.
Figure 5. Spatial distributions of the predicted sensitivity of O3 to total NOx emissions. (a) Average 8-hr daily maximum O3 sensitivity during 14–24 July 2006. (b) Sensitivity during the maximum O3 concentration, 11:00 p.m. (23:00) UTC or 4:00 p.m. (16:00) LT on 21 July.
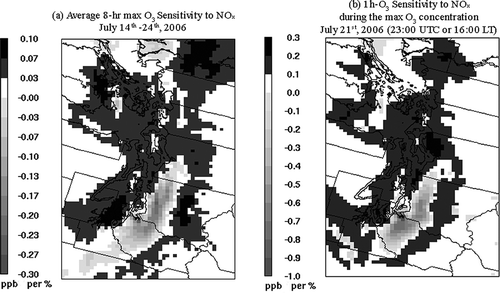
O3 sensitivity to VOC emissions
Spatial distributions of the impact of total VOC emissions on 8-hr daily maximum ozone concentrations during the entire simulation period and on 1-hr maximum ozone concentration during the most polluted day (21 July) were simulated ( and b, respectively). In a NOx-saturated environment (i.e., Seattle, Vancouver), a decrease in VOC emissions will result in decreases of O3 concentrations (CitationSeinfeld and Pandis, 2006; CitationTsimpidi et al., 2008; CitationWhite et al., 1976). The average simulated impact of VOC emissions on 8-hr daily maximum O3 concentrations in Seattle is 0.18 ppb per %. The peak simulated impact of VOC emissions on 1-hr O3 concentrations during 21 July is predicted in Tacoma (0.75 ppb per %). In Seattle, during the same day, the maximum simulated positive impact is 0.4 ppb per %. Average sensitivities of 8-hr daily maximum O3 concentrations to mobile, point, biogenic, nonroad, and area VOC emissions were calculated for the simulation period (). Biogenic sources impact O3 concentrations (up to 0.05 ppb per %) over a wide area that covers most of the fine-scale model domain (). Mobile, nonroad, and area VOC emissions impact O3 concentrations (up to 0.05 ppb per %, each) in the urban areas of the western part of the King and Pierce counties. Finally, ozone is typically insensitive to point VOC emissions (less than 0.002 ppb per %; not shown), primarily due to the low VOC emissions from point sources (). This suggests that a potential control of the VOC emissions coming from the refineries, which are located on the northwest coast of Washington state, will have a negligible impact on ozone concentration over the Puget Sound region. On average, Canadian anthropogenic VOC emissions is simulated to impact O3 concentrations in Vancouver and the northwest corner of Whatcom County by up to 0.05 ppb per % (). However, ozone levels in the remaining areas of the United States within the domain are not very sensitive to Canadian anthropogenic VOC emissions. During the peak O3 event, Canadian anthropogenic VOC emissions are simulated to impact O3 concentrations in Vancouver by 0.06 ppb per % (). Simulated O3 sensitivities to Canadian VOC emissions are less than 0.01 ppb per % in Seattle and the Enumclaw monitoring station, where the peak O3 concentrations were observed.
Figure 8. Spatial distributions of the predicted sensitivity of O3 to total VOC emissions. (a) Average 8-hr daily maximum O3 sensitivity during 14–24 July 2006. (b) Sensitivity during the maximum O3 concentration, 11:00 p.m. (23:00) UTC or 4:00 p.m. (16:00) LT on 21 July.
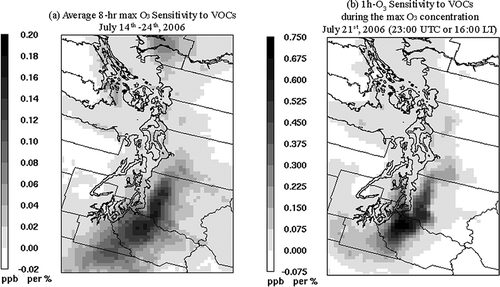
O3 sensitivity to VOC and NOx emissions at the maximum monitored ozone location
At the location with the highest observed ozone concentration (Mud Mountain, near Enumclaw), the sensitivity of 1-hr ozone is always positive to total VOC emission changes (up to 0.03 ppb per %), whereas the impact of total NOx emission changes can be either positive (up to 0.02 ppb per %) or negative (up to −0.02 ppb per %), depending on the relative availability of NOx and VOCs and the time of day (). During the day with the highest ozone concentration in Enumclaw (21 July), reduction of either NOx or VOC emissions results in reductions of ozone concentration (sensitivities are both positive: 0.17 and 0.28 ppb, respectively). In particular, O3 concentrations during that day are most sensitive to mobile NOx and biogenic VOC emission sources. The sensitivities of the average 1-hr maximum O3 concentrations to VOC emissions from biogenic, mobile, area, and nonroad sources are 0.15, 0.06, 0.04, and 0.03 ppb per %, respectively. The corresponding sensitivities to NOx emissions from mobile, nonroad, and area sources are 0.11, 0.05, and 0.01 ppb per %, respectively.
PM2.5 sensitivity to VOC and NOx emissions
The sensitivity of PM2.5 to emissions of VOCs and NOx was investigated for Seattle, Portland, Vancouver (Canada), and Victoria (Canada) (). NOx has the greatest impact on PM2.5 in Vancouver, where simulation results suggest that lowering NOx emissions would increase the average PM2.5 concentrations. PM2.5 concentrations in Victoria, Portland, and Seattle are also negatively sensitive to NOx emissions. The negative sensitivity of PM2.5 to NOx occurs in urban areas with high NOx-to-VOC ratios (NOx-saturated environments), where the decrease of NOx leads to an increase of O3 and OH radical concentrations, which play a role in the oxidation of SO2 to sulfate aerosol and VOCs to secondary organic aerosols (SOA). Therefore, whereas nitrate concentrations decrease with decreasing NOx emissions, sulfate and SOA increase. For instance, in this application, nitrate decreases over Seattle by 0.0008 μg m−3 per % on average in response to NOx emission decreases, whereas sulfate and SOA increase by 0.0002 and 0.0022 μg m−3 per %, respectively. This results in an overall increase of PM2.5 by 0.0016 μg m−3 per % on average. Increases of PM2.5 from decreasing NOx emissions are driven by mobile and nonroad sources in Seattle and Portland. Decreased emissions of VOCs always decrease PM2.5 concentrations. The greatest impacts are observed in Victoria and Vancouver where PM2.5 sensitivities are 0.037 and 0.034 μg m−3 per %, respectively, followed by Seattle and Portland (0.012 μg m−3 per %, each). The decrease of VOC emissions in NOx-saturated environments decreases oxidant levels, which in turn decrease sulfate and organic aerosols, and can also decrease nitrate. In Portland, Seattle, and Victoria, PM2.5 concentrations are more sensitive to emissions of VOCs from biogenic sources than other sources of VOCs. In Vancouver, PM2.5 concentrations are sensitive to biogenic emissions of VOCs and Canadian anthropogenic emissions of VOCs. The differences in biogenic emissions of isoprene and monoterpene in Canada and the United States, due to inconsistencies on the vegetation classes used to prepare the biogenic emissions, also play a role in the simulated elevated impacts of VOC emissions in the Canadian cities compared with the U.S. cities.
Table 5. CMAQ PM2.5 surface sensitivities to emissions of NOx and VOCs from various source categories predicted at major cities within the modeling domain (biogenic emissions include emissions from the United States and Canada)
Conclusions
This paper describes the simulated sensitivity of air pollutants to their sources by using a nested regional modeling system that simulates the air quality over the northwestern United States during a summer smog episode of 2006. The system consists of the WRF mesoscale meteorological model, SMOKE emission model, and CMAQ air quality model. Model evaluation is conducted to assess its skill for meteorology, O3, and PM2.5 over the northwestern United States. The meteorological model performance is within the typical range for air quality modeling. For the 14–24 July 2006 period, the average simulated 8-hr daily maximum O3 concentration is 48.9 ppb. Comparing model performance with observations reveals that O3 is underpredicted for observed concentrations above 40 ppb (NMB = −7.9%) but is biased high at low O3 levels that occurred during nighttime. The positive bias during nighttime, though, cannot affect the DDM calculations, as the sensitivity analysis is focused only during the hours that contribute to the 8-hr daily maximum. Overall, the model does well in representing the daily ozone trend and meets the recommended criteria by EPA for a good performance of 1-hr ozone simulation (NMB = −7.9%, NME = 25.1). The average simulated domain-wide fine particulate matter (PM2.5) surface concentration is 5.6 μg m−3, with the highest concentrations occurring around Vancouver. Organic mass, sulfate, soil, elemental carbon, ammonium, and nitrate account for 56%, 16%, 12%, 6%, 6%, and 3% of PM2.5 mass, respectively. Compared with measurements, the NMB is 9.6%, indicating that the model results for PM2.5 are biased slightly high.
DDM-3D is applied to compute sensitivities of 8-hr daily maximum O3 and PM2.5 ambient concentrations to VOC and NOx emissions. The impact of each source category (area, point, mobile, nonroad, and biogenic) as well as the Canadian anthropogenic VOC and NOx emissions on 8-hr daily maximum O3 and PM2.5 concentrations over the northwestern United States is also investigated. In urban areas, which are characterized by high NOx-to-VOC ratios (NOx saturated), a reduction of NOx emissions will lead to an increase of O3 concentration (negative impact). For instance, in Seattle, the average simulated sensitivity of 8-hr daily maximum O3 concentration to anthropogenic NOx emissions is −0.27 ppb per %. In this case, mobile NOx emissions have the largest impact on O3 concentration (up to −0.2 ppb per %), followed by point and nonroad sources (up to −0.1 and −0.05 ppb per %, respectively). In more remote and forest areas, the NOx-to-VOC ratio is low (NOx limited) due to the high biogenic VOC concentrations. In such areas, a decrease of NOx emissions will result in a decrease of O3 concentrations (positive impact). In NOx-saturated environments (urban areas), a decrease in VOC emissions will lead to increased O3 concentrations. The average simulated sensitivity of 8-hr daily maximum O3 concentrations in Seattle to VOC emissions is 0.18 ppb per %, with maxima up to 1.8 ppb per %. The impact of mobile, biogenic, nonroad, and area emission sources on average 8-hr daily maximum O3 concentrations is up to 0.05 ppb per %, each. Canadian anthropogenic VOC emissions also have a positive impact on O3 concentrations in the northwest corner of Whatcom County, by up to 0.03 ppb per %.
The sensitivity of PM2.5 to emissions of VOCs and NOx is investigated at four major cities (Seattle, Portland, Vancouver, and Victoria). PM2.5 concentration is negatively sensitive to NOx emissions. In urban areas (NOx-saturated environments), a reduction of NOx concentrations results in an increase of OH and O3 concentrations and, as a result, aerosol concentration (mainly sulfate and SOA) in such areas can increase. On the other hand, the decrease of VOC emissions results in lower OH radical and ozone concentrations and consequently a decrease of sulfate and SOA concentration levels. Sensitivity analysis finds that decreased biogenic VOC emissions lead to a decrease of PM2.5, up to 0.028 μg m−3 per %. As a result, a positive impact is observed in all four cities, with the largest PM2.5 sensitivities predicted in Victoria and Vancouver (0.037 and 0.034 μg m−3 per %, respectively), followed by Seattle and Portland (0.012 μg m−3 per %, each). PM2.5 concentrations are more sensitive to emissions of VOCs from biogenic sources than other sources of VOCs.
Acknowledgments
This research was supported by ConocoPhillips.
References
- Barna , M. and Lamb , B. 2000 . Improving ozone modeling in regions of complex terrain using observational nudging in a prognostic meteorological model . Atmos. Environ. , 34 : 4889 – 4906 .
- Barna , M. , Lamb , B. , ’Neill , S. O , Westberg , H. , Figueroa-Kaminsky , C. , Otterson , S. , Bowman , C. and DeMay , J. 2000 . Modeling ozone formation and transport in the Cascadia region of the Pacific Northwest . J. Appl. Meteorol. , 39 : 349 – 366 . doi: 10.1175/1520-0450(2000)0392.0.co;2
- Barna , M. , Lamb , B. and Westberg , H. 2001 . Modeling the effects of VOC/NOx emissions on ozone synthesis in the Cascadia airshed of the Pacific Northwest . J. Air Waste Manage. Assoc. , 51 : 1021 – 1034 . doi: 10.1080/10473289.2001.10464330
- Bell , M.L. , Dominici , F. and Samet , J.M. 2005 . A meta-analysis of time-series studies of ozone and mortality with comparison to the national morbidity, mortality, and air pollution study . Epidemiology , 16 : 436 – 445 . doi: 10.1097/01.ede.0000165817.40152.85
- Blanchard , C.L. and Stoeckenius , T. 2001 . Ozone response to precursor controls: Comparison of data analysis methods with the predictions of photochemical air quality simulation models . Atmos. Environ. , 35 : 1203 – 1215 . doi: 10.1016/s1352-2310(00)00400-3
- Boylan , J.W. and Russell , A.G. 2006 . PM and light extinction model performance metrics, goals, and criteria for three-dimensional air quality models . Atmos. Environ. , 40 : 4946 – 4959 . doi: 10.1016/j.atmonsenv.2005.09.087
- Byun , D. and Schere , K.L. 2006 . Review of the governing equations, computational algorithms, and other components of the models—3 Community Multiscale Air Quality (CMAQ) modeling system . Appl. Mech. Rev. , 59 : 51 – 77 . doi: 10.1115/1.2128636
- Carlton , A.G. , Bhave , P.V. , Napelenok , S.L. , Edney , E.D. , Sarwar , G. , Pinder , R.W. , Pouliot , G.A. and Houyoux , M. 2010 . Model representation of secondary organic aerosol in CMAQv4.7 . Environ. Sci. Technol. , 44 : 8553 – 8560 . doi: 10.1021/es100636q
- Carlton , A.G. , Turpin , B.J. , Altieri , K.E. , Seitzinger , S.P. , Mathur , R. , Roselle , S.J. and Weber , R.J. 2008 . CMAQ model performance enhanced when in-cloud secondary organic aerosol is included: Comparisons of organic carbon predictions with measurements . Environ. Sci. Technol. , 42 : 8798 – 8802 . doi: 10.1021/es801192n
- Carlton , A.G. , Wiedinmyer , C. and Kroll , J.H. 2009 . A review of secondary organic aerosol (SOA) formation from isoprene . Atmos. Chem. Phys. , 9 : 4987 – 5005 .
- Carter, W.P.L. 2000. Documentation of the SAPRC-99 chemical mechanism for VOC reactivity assessment. California Air Resources Board, contract nos. 92–329 and 95–308. http://www.arb.ca.gov/research/apr/past/92-329a_v2.pdf (http://www.arb.ca.gov/research/apr/past/92-329a_v2.pdf)
- Carolina Environmental Program . 2003 . Sparse Matrix Operator Kernel Emissions Modeling System (SMOKE) User Manual , Chapel Hill , NC : www.smoke-model.org .
- Chock , D.P. , Chang , T.Y. , Winkler , S.L. and Nance , B.I. 1999 . The impact of an 8 h ozone air quality standard on ROG and NOx controls in Southern California . Atmos. Environ. , 33 : 2471 – 2485 . doi: 10.1016/s1352-2310(98)00381-1
- Cohan , D.S. , Hakami , A. , Hu , Y.T. and Russell , A.G. 2005 . Nonlinear response of ozone to emissions: Source apportionment and sensitivity analysis . Environ. Sci. Technol. , 39 : 6739 – 6748 . doi: 10.1021/es048664m
- Davis , J.M. , Bhave , P.V. and Foley , K.M. 2008 . Parameterization of N2O5 reaction probabilities on the surface of particles containing ammonium, sulfate, and nitrate . Atmos. Chem. Phys. , 8 : 5295 – 5311 .
- Dodge , M. 1987 . Chemistry of Oxidant Formation: Implications for Designing Effective Control Strategies , Washington , DC : U.S. Environmental Protection Agency . EPA/600/D-87/114 (NTISPB87179990)
- Dudhia , J. 1989 . Numerical study of convection observed during the winter monsoon experiment using a mesoscale two-dimensional model . J. Atmos. Sci. , 46 : 3077 – 3107 . doi: 10.1175/1520-0469(1989)0462.0.co;2
- Duncan , B.N. , Yoshida , Y. , Olson , J.R. , Sillman , S. , Martin , R.V. , Lamsal , L. , Hu , Y.T. , Pickering , K.E. , Retscher , C. , Allen , D.J. and Crawford , J.H. 2010 . Application of OMI observations to a space-based indicator of NOx and VOC controls on surface ozone formation . Atmos. Environ. , 44 : 2213 – 2223 . doi: 10.1016/j.atmosenv.2010.03.010
- Dunker , A.M. 1980 . The response of an atmospheric reaction-transport model to changes in input functions . Atmos. Environ. , 14 : 671 – 679 . doi: 10.1016/0004-6981(80)90051-7
- Dunker , A.M. 1981 . Efficient calculation of sensitivity coefficients for complex atmospheric models . Atmos. Environ. , 15 : 1155 – 1161 . doi: 10.1016/0004-6981(81)90305-x
- Dunker , A.M. 1984 . The decoupled direct method for calculating sensitivity coefficients in chemical-kinetics . Abstracts of Papers of the American Chemical Society , 188(AUG):88-PHYS
- Dunker , A.M. , Yarwood , G. , Ortmann , J.P. and Wilson , G.M. 2002 . Comparison of source apportionment and source sensitivity of ozone in a three-dimensional air quality model . Environ. Sci. Technol. , 36 : 2953 – 2964 . doi: 10.1021/es011418f
- Eder , B. and Yu , S.C. 2006 . A performance evaluation of the 2004 release of models—3 CMAQ . Atmos. Environ , 40 : 4811 – 4824 . doi: 10.1016/j.atmosenv.2005.08.045
- Elleman , R.A. and Covert , D.S. 2009 . Aerosol size distribution modeling with the community multiscale air quality modeling system in the Pacific Northwest: 1. Model comparison to observations . J. Geophys. Res. Atmos. , D11206 doi: D11206:10.1029/2008jd010791
- Emery , C. , Tai , E. and Yarwood , G. Enhanced meteorological modeling and performance evaluation for two Texas ozone episodes . Prepared for the Texas Natural Resource Conservation Commissions by Environ International Corporation . Novato , CA .
- Environ . 2003 . User's guide to the comprehensive air quality model with extensions (CAMx). Version 4.02 , Novato , CA : Report prepared by ENVIRON International Corporation .
- Foley , K.M. , Roselle , S.J. , Appel , K.W. , Bhave , P.V. , Pleim , J.E. , Otte , T.L. , Mathur , R. , Sarwar , G. , Young , J.O. , Gilliam , R.C. , Nolte , C.G. , Kelly , J.T. , Gilliland , A.B. and Bash , J.O. 2010 . Incremental testing of the Community Multiscale Air Quality (CMAQ) modeling system version 4.7 . Geosci. Model Dev. , 3 : 205 – 226 .
- Hakami , A. , Odman , M.T. and Russell , A.G. 2003 . High-order, direct sensitivity analysis of multidimensional air quality models . Environ. Sci. Technol. , 37 : 2442 – 2452 . doi: 10.1021/es020677h
- Hakami , A. , Odman , M.T. and Russell , A.G. 2004 . Nonlinearity in atmospheric response: A direct sensitivity analysis approach . J. Geophys. Res. Atmos. , : D15303 doi: D15303:10.1029/2003jd004502
- Hanna , S.R. and Yang , R.X. 2001 . Evaluations of mesoscale models’ simulations of near-surface winds, temperature gradients, and mixing depths . J. Appl. Meteorol. , 40 : 1095 – 1104 . doi: 10.1175/1520-0450(2001)0402.0.co;2
- Hong , S.Y. , Noh , Y. and Dudhia , J. 2006 . A new vertical diffusion package with an explicit treatment of entrainment processes . Mon. Weather Rev. , 134 : 2318 – 2341 . doi: 10.1175/mwr3199.1
- Ito , K. , De Leon , S.F. and Lippmann , M. 2005 . Associations between ozone and daily mortality—Analysis and meta-analysis . Epidemiology , 16 : 446 – 457 . doi: 10.1097/01.ede.0000165821.90114.7f
- Jiang , G.F. , Lamb , B. and Westberg , H. 2003 . Using back trajectories and process analysis to investigate photochemical ozone production in the Puget Sound region . Atmos. Environ. , 37 : 1489 – 1502 . doi: 10.1016/s1352-2310(02)01027-0
- Kain , J.S. and Fritsch , J.M. 1990 . A one-dimensional entraining detraining plume model and its application in convective parameterization . J. Atmos. Sci. , 47 : 2784 – 2802 . doi: 10.1175/1520-0469(1990)0472.0.co;2
- Karydis , V.A. , Tsimpidi , A.P. and Pandis , S.N. 2007 . Evaluation of a three-dimensional chemical transport model (PMCAMx) in the eastern United States for all four seasons . J. Geophys. Res. Atmos. , D ( 14211 ) doi: D14211:10.1029/2006jd007890
- Kelly , J.T. , Bhave , P.V. , Nolte , C.G. , Shankar , U. and Foley , K.M. 2010 . Simulating emission and chemical evolution of coarse sea-salt particles in the Community Multiscale Air Quality (CMAQ) model . Geosci. Model Dev. , 3 : 257 – 273 .
- Koo , B. , Wilson , G.M. , Morris , R.E. , Dunker , A.M. and Yarwood , G. 2009 . Comparison of source apportionment and sensitivity analysis in a particulate matter air quality model . Environ. Sci. Technol. , 43 : 6669 – 6675 . doi: 10.1021/es9008129
- Likens , G.E. , Driscoll , C.T. and Buso , D.C. 1996 . Long-term effects of acid rain: Response and recovery of a forest ecosystem . Science , 272 : 244 – 246 .
- Lin , Y.L. , Farley , R.D. and Orville , H.D. 1983 . Bulk parameterization of the snow field in a cloud model . J. Clim. Appl. Meteorol. , 22 : 1065 – 1092 . doi: 10.1175/1520-0450
- Mass , C. 1982 . The topographically forced diurnal circulations of western Washington State and their influence on precipitation . Mon. Weather Rev. , 110 : 170 – 183 . doi: 10.1175/1520-0493(1982)1102.0.co;2
- Mauzerall , D.L. and Wang , X.P. 2001 . Protecting agricultural crops from the effects of tropospheric ozone exposure: Reconciling science and standard setting in the United States, Europe, and Asia . Annu. Rev. Energy Environ. , 26 : 237 – 268 . doi: 10.1146/annurev.energy.26.1.237
- Mendoza-Dominguez , A. , Wilkinson , J.G. , Yang , Y.J. and Russell , A.G. 2000 . Modeling and direct sensitivity analysis of biogenic emissions impacts on regional ozone formation in the Mexico-US border area . J. Air Waste Manage. Assoc. , 50 : 21 – 31 . doi: 10.1080/10473289.2000.10463987
- Mlawer , E.J. , Taubman , S.J. , Brown , P.D. , Iacono , M.J. and Clough , S.A. 1997 . Radiative transfer for inhomogeneous atmospheres: RRTM, a validated correlated-k model for the longwave . J. Geophys. Res. Atmos. , 102 ( D14 ) : 16663 – 16682 . doi: 10.1029/97jd00237
- Napelenok , S.L. , Cohan , D.S. , Hu , Y.T. and Russell , A.G. 2006 . Decoupled direct 3D sensitivity analysis for particulate matter (DDM-3D/PM) . Atmos. Environ. , 40 : 6112 – 6121 . doi: 10.1016/j.atmosenv.2006.05.039
- O'Neill , S.M. and Lamb , B.K. 2005 . Intercomparison of the Community Multiscale Air Quality model and CALGRID using process analysis . Environ. Sci. Technol. , 39 : 5742 – 5753 . doi: 10.1021/es048403c
- Odman, M.T., J.W. Boylan, J.G.Wilkinson, A.G. Russell, K. Doty,W. Norris, R. McNider, S.F. Mueller, and R.E. Imhoff. 2002. SAMI Air Quality Modeling Report, Final Report, Southern Appalachian Mountains Initiative. http://www.nature.nps.gov/air/pubs/pdf/SAMI_Final_Report_0802.pdf (http://www.nature.nps.gov/air/pubs/pdf/SAMI_Final_Report_0802.pdf)
- Pun , B.K. , Seigneur , C. , Bailey , E.M. , Gautney , L.L. , Douglas , S.G. , Haney , J.L. and Kumar , N. 2008 . Response of atmospheric particulate matter to changes in precursor emissions: A comparison of three air quality models . Environ. Sci. Technol. , 42 : 831 – 837 . doi: 10.1021/es702333d
- Schwartz , J. , Dockery , D.W. and Neas , L.M. 1996 . Is daily mortality associated specifically with fine particles? . J. Air Waste Manage. Assoc. , 46 : 927 – 939 . doi: 10.1080/10473289.1996.10467528
- Seinfeld , J.H. and Pandis , S.N. 2006 . Atmospheric Chemistry and Physics: From Air Pollution to Climate Change , Hoboken , NJ : John Wiley & Sons .
- Skamarock , W.A. , Klemp , J.B. , Dudhia , J. , Gill , D.O. , Barker , D.M. , Wang , W. and Powers , J.G. 2005 . A Description of the Advanced Research WRF Version 2 , Boulder , CO : National Center for Atmospheric Research . Technical note
- Tesche , T.W. , Morris , R. , Tonnesen , G. , McNally , D. , Boylan , J. and Brewer , P. 2006 . CMAQ/CAMx annual 2002 performance evaluation over the eastern US . Atmos. Environ. , 40 : 4906 – 4919 . doi: 10.1016/j.atmosenv.2005.08.046
- Tong , D.Q. and Mauzerall , D.L. 2006 . Spatial variability of summertime tropospheric ozone over the continental United States: Implications of an evaluation of the CMAQ model . Atmos. Environ. , 40 : 3041 – 3056 . doi: 10.1016/j.atmosenv.2005.11.058
- Tsimpidi , A.P. , Karydis , V.A. and Pandis , S.N. 2007 . Response of inorganic fine particulate matter to emission changes of sulfur dioxide and ammonia: The eastern United States as a case study . J. Air Waste Manage. Assoc. , 57 : 1489 – 1498 . doi: 10.3155/1047-3289.57.12.1489
- Tsimpidi , A.P. , Karydis , V.A. and Pandis , S.N. 2008 . Response of fine particulate matter to emission changes of oxides of nitrogen and-anthropogenic volatile organic compounds in the Eastern United States . J. Air Waste Manage. Assoc. , 58 : 1463 – 1473 . doi: 10.3155/1047-3289.58.11.1463
- U.S. Environmental Protection Agency . 1991 . Guidance for Regulatory Application of the Urban Airshed Model (UAM) , Research Triangle Park , NC : Office of Air Quality Planning and Standards, U.S. Environmental Protection Agency .
- White , W.H. , Anderson , J.A. , Blumenthal , D.L. , Husar , R.B. , Gillani , N.V. , Husar , J.D. and Wilson , W.E. 1976 . Formation and transport of secondary air-pollutants—Ozone and aerosols in St-Louis Urban Plume . Science , 194 : 187 – 189 . doi: 10.1126/science.959846
- Yang , Y.J. , Wilkinson , J.G. and Russell , A.G. 1997 . Fast, direct sensitivity analysis of multidimensional photochemical models . Environ. Sci. Technol. , 31 : 2859 – 2868 .
- Yu , S.C. , Eder , B. , Dennis , R. , Chu , S.H. and Schwartz , S.E. 2006 . New unbiased symmetric metrics for evaluation of air quality models . Atmos. Sci. Lett. , 7 : 26 – 34 . doi: 10.1002/asl.125
- Zhang , Y. , Liu , P. , Queen , A. , Misenis , C. , Pun , B. , Seigneur , C. and Wu , S.Y. 2006 . A comprehensive performance evaluation of MM5-CMAQ for the Summer 1999 Southern Oxidants Study episode—Part II: Gas and aerosol predictions . Atmos. Environ. , 40 : 4839 – 4855 . doi: 10.1016/j.atmonsenv.2005.12.048
- Zhang , Y. , Pun , B. , Wu , S.Y. , Vijayaraghavan , K. and Seigneur , C. 2004 . Application and evaluation of two air quality models for particulate matter for a southeastern US episode . J. Air Waste Manage. Assoc. , 54 : 1478 – 1493 . doi: 10.1080/10473289.2004.10471012
- Zhang , Y. , Vijayaraghavan , K. and Seigneur , C. 2005 . Evaluation of three probing techniques in a three-dimensional air quality model . J. Geophys. Res. Atmos. , D ( 02305 ) doi: D0230510.1029/2004jd005248
- Zhou , J.A. , Ito , K. , Lall , R. , Lippmann , M. and Thurston , G. 2011 . Time-series analysis of mortality effects of fine particulate matter components in Detroit and Seattle . Environ. Health Perspect. , 119 : 461 – 466 . doi: 10.1289/ehp.1002613