Abstract
Communities along Utah's Wasatch Front are currently developing strategies to reduce daily average PM2.5 levels to below National Ambient Air Quality Standards during wintertime persistent stable atmospheric conditions, or cold-air pools. Speciated PM2.5 data from the Wasatch Front airshed indicate that wintertime exceedances of the PM2.5 standard are mainly driven by high levels of ammonium nitrate. Stable wintertime conditions foster the formation of ammonium nitrate aerosol when sufficient sources of NOx, ammonia, and oxidative capacity exist. However, this work demonstrates that secondary ammonium chloride aerosol can also be a significant source of secondary wintertime PM2.5 if sufficient sources of atmospheric chlorine exist. Two factor analysis techniques, positive matrix factorization (PMF) and Unmix, were used to identify contributors to PM2.5 at three monitoring stations along Utah's Wasatch Front: Bountiful, Lindon, and Salt Lake City. The monitoring data included chemically speciated PM2.5 data for 227, 227, and 429 days at each location, respectively, during the period from May 2007 through May 2011. PMF identified 10–12 factors and Unmix identified 4–5 factors for each of the locations. The wintertime PMF and Unmix results showed large contributions from secondary PM2.5 when PM2.5 concentrations exceeded 20 μg/m3. PMF identified both ammonium nitrate and ammonium chloride aerosol as significant secondary contributors to PM2.5 (10–15% of total PM2.5 from ammonium chloride) during wintertime pollution episodes. Subsequent ion balance analysis of the monitoring data confirmed the presence of significant ammonium chloride aerosol on these highly polluted days at all three monitoring sites. The directly emitted primary PM2.5 portions of the source attribution results were further compared to county-level emissions inventories and showed generally good agreement for Salt Lake City and Lindon during wintertime except for wood smoke and fugitive dust, which have higher contributions in the receptor modeling results than in the emissions inventories.
The study suggests that secondary ammonium chloride aerosol can be a significant source of wintertime PM2.5 in an ammonia-rich environment, like the Wasatch Front airshed, if sufficient sources of atmospheric chlorine exist. During wintertime, cold-air-pool events, the source attribution results generally agree with the county emission inventories with the exception of wood smoke and cooking sources. At the Salt Lake City monitoring station, the estimated contributions from wood smoke and cooking are nearly double those of the corresponding inventory, suggesting that they are nearly as important as gasoline emissions.
Introduction
Exposure to fine particulate matter (PM2.5, particles with an aerodynamic diameter <2.5 μm) has been linked to adverse human health effects, including increases in cardiovascular and pulmonary disease (CitationBaliff et al., 2000; CitationNicolai et al., 2003), and morbidity and mortality (CitationDockery et al., 1993; CitationPope et al., 1991). PM2.5 also contributes to impaired visibility (CitationWatson 2002) and changes in the global radiative balance (CitationChung and Seinfeld, 2002). In 2006, The U.S. Environmental Protection Agency (EPA) issued an updated 24-hr standard for PM2.5 of 35 μg/m3, and as a result in 2009 it declared three regions in northern Utah along the Wasatch Front as nonattainment areas for 24-hr average PM2.5. The state of Utah is currently developing a plan to bring the PM2.5 concentrations to attainment levels.
The Wasatch Front typically experiences elevated levels of PM2.5 during wintertime, when high-pressure weather systems and a high solar zenith angle lead to cold-air pools that periodically trap aerosols in mountain valleys. These elevated PM2.5 levels cause adverse health effects locally. For example, the state of Utah found that the odds of an emergency department visit in Salt Lake County, with a primary diagnosis of asthma, are 42% greater during days 5–7 of prolonged inversions than for noninversion days (Beard et al., 2012). During a particularly extreme cold-air pool event in 2004, 24-hr average PM2.5 concentrations exceeded 100 μg/m3 (CitationMalek et al., 2006), while more recent maximum daily average concentrations have been in the range of 50–70 μg/m3.
Several previous studies have examined the sources of fine particulate matter along the Wasatch Front; however, compared to this work these studies used data that spanned a brief time period, on the order of weeks. CitationHansen et al. (2010) collected speciated hourly PM2.5 data at the Utah Division of Air Quality (UDAQ) Lindon air monitoring station for 10 days during the winter of 2007 and performed a source apportionment study using the positive matrix factorization (PMF) model. Their model results identified the following four primary sources, mobile diesel, mobile gasoline, wood smoke, and road dust, and the following secondary sources: sulfate, nitrate, organic matter, and aged wood smoke. Grover et al. (2006) performed an intensive air monitoring campaign in August 2002 at the UDAQ Lindon air monitoring station and complementary source apportionment using the Unmix model. From this small sample set, they found three main contributors to PM2.5: gasoline emissions, diesel emissions, and secondary aerosols. Their analysis did not include inorganic species, so it is not surprising that they did not identify sources of crustal material. The limited duration of these previous studies makes it difficult to draw general conclusions about the sources of fine particulate matter along the Wasatch Front.
This study investigates the sources of PM2.5 and the impact of secondary ammonium nitrate and chloride affecting three monitoring sites along the Wasatch Front using ambient data collected from 2007 to 2011. Source apportionment is performed using two receptor models, PMF and Unmix, and model results are compared to each other as well as to emissions inventories. Analysis of chemically speciated PM2.5 in this work and in previous studies (CitationHansen et al., 2010, CitationMangelson et al., 1997) indicates that the largest contribution to PM2.5 on winter days that exceed the National Ambient Air Quality Standard comes from secondary ammonium nitrate aerosol. However, the source apportionment and monitoring data analyses presented in this work also demonstrate that secondary ammonium chloride aerosol is a significant source of wintertime PM2.5 at all three monitoring locations.
The physical and thermodynamic properties of ammonium chloride aerosol have been previously investigated by CitationPio and Harrison (1987a). They found that the thermodynamics of aerosol ammonium chloride are similar to the thermodynamics of ammonium nitrate. Both ammonium nitrate and ammonium chloride aerosols exist in a reversible phase equilibrium with their gaseous precursors (ammonia and the respective acids); however, the volatility of ammonium chloride has a somewhat stronger temperature dependence than ammonium nitrate.
While published observations of ambient ammonium chloride aerosol are unusual, a number of previous studies have reported or inferred its presence. CitationDu et al. (2010) measured chemically speciated hourly PM2.5 concentrations in Shanghai for a 2-day period in 2009. They observed that the hourly sum of ammonium sulfate, ammonium nitrate, and ammonium chloride ranged between 2.6 and 101.2 μg/m3 with ammonium nitrate being the dominant species. Hourly ammonium nitrate and ammonium chloride observations were well correlated, with ammonium chloride being approximately one-tenth the concentration of ammonium nitrate. CitationChang and Allen (2006) compared measured ammonium chloride aerosol with photochemical model predictions during a photochemical episode in southeast Texas from August to September 2000. Both model and measurements indicated that significant ammonium chloride aerosol formation only occurred at times and in areas that were both ammonia rich and had sufficient atmospheric chlorine. They found that anthropogenic chlorine emissions in southeast Texas had the potential to enhance PM2.5 up to 9 μg/m3, but only in localized areas for brief periods, given the summertime temperatures and the mostly ammonia-poor conditions. CitationPossanzini et al. (1992) conducted field experiments in Rome, Italy, and found that ammonium chloride aerosol represented approximately one-fifth of observed ammonium nitrate and one-tenth of the total ammonium species by mass. CitationPio and Harrison (1987b) determined ammonium chloride aerosol concentrations of up to 10 μg/m3 in northwest England, and CitationYoshizumi and Okita (1983) examined aerosol filter data from 1975 collected in Riverside, CA, and determined that ammonium chloride concentrations ranged between 7.76 and 15.5 μg/m3.
In this work we provide evidence that ammonium chloride aerosol adds a significant contribution to PM2.5 in the Wasatch Front airshed during wintertime days when PM2.5 is elevated and the airshed is more generally dominated by ammonium nitrate aerosol.
Materials and Methods
PM2.5 samples
The UDAQ operates three urban PM2.5 chemical speciation monitors as part of the U.S. Environmental Protection Agency (EPA) Speciation and Trends Network (STN). The three STN monitors are located in Salt Lake City, Bountiful, and Lindon, and shows a map indicating the locations of the three monitors along the Wasatch Front. The 24-hr average PM2.5 data used in this study were downloaded from the U.S. EPA Air Quality System (AQS) database of quality-assured data. The date range of data extracted was 5/6/2007 to 5/9/2011, resulting in 429 sample days for Salt Lake City, 227 days for Bountiful, and 228 days for Lindon. The sampling frequency at the Salt Lake City monitor was twice that of the other monitors, which accounts for approximately twice the number of samples available from the Salt Lake City site. The beginning of this date range coincided with the installation of an updated carbon sampler (URG 3000N, May 2007), and the end date represents the latest data available at the time data were extracted (May 2011).
After preprocessing the datasets (described in the following section), the PMF analysis included the following chemical species: Al, Br, Ca, Cr, Cu, Cl, Fe, Pb, Mn, Ni, Mg, Ti, V, Si, Zn, K, Na, NH4, NO3, OC1, OC2, OC3, OC4, OP, EC1, EC2, EC3, and SO4 (Note: OC and EC data were exclusively from the URG 3000N). The organic carbon (OC) and elemental carbon (EC) fractions are determined by the U.S. EPA STN carbon analysis protocol, which uses the IMPROVE_A methodology (CitationDRI, 2008). The Unmix analysis required removal of additional species.
Preprocessing
Prior to analysis, the data sets were preprocessed to correct for field blanks, missing/negative values, incomplete values, and poor signal-to-noise (S/N) ratios.
Analytical data from EPA's STN monitoring network in the AQS database were not blank corrected, but sample blank data were available. Field blanks were generally collected less frequently than the monitoring frequency, so many samples did not have an associated field blank. For samples with coincident field blanks, the measured blank concentration was subtracted from the reported concentration. For samples without coincident blanks, the median value from the previous three blanks was used as an estimate for blank correction.
The PMF model requires that each measurement be assigned an uncertainty. Most measurements reported in the AQS database also report the analytical uncertainty. The overall measurement uncertainty was estimated from a combination of the measured analytical uncertainty and the method detection limit (MDL). The uncertainty of measurements reported below the MDL were set to either 5/6 × MDL or the reported uncertainty, whichever was larger. The uncertainty of measurements above the MDL were set to the measured analytical uncertainty plus 1/3 × MDL (CitationReff et al., 2007). For those chemical species where uncertainties were not available, the uncertainties were estimated based on a fraction of the measured value using the methodology and fractions employed by CitationKim et al. (2005).
If the total PM2.5 mass of a sample was missing or if an entire analytical channel was missing (e.g., carbon data, ions, metals), the sample was removed from the modeling data set for both PMF and Unmix data sets. In instances where individual chemical species were missing, the missing observations in the PMF and the Unmix dataset were replaced with the species median concentration, and the uncertainty for PMF was set to four times the species median concentration to minimize the influence of the replaced data on the model solution. Chemical species were removed from the modeling dataset if more than 50% of the samples had missing data.
PMF and Unmix do not allow negative data, which can sometimes be reported if species concentrations are close to zero. For the PMF data set, negative concentrations were reset to zero. For the Unmix data set, negative or zero values were replaced with ½ × MDL (CitationU.S. EPA 2007).
Data with low S/N can occur when many samples are reported near or below MDLs. Using data with excessive noise has been found to negatively impact the quality of receptor modeling results (CitationPaatero and Hopke, 2003; Reff et al., 2007). In this study, chemical species with S/N < 0.46 were discarded for PMF (<0.5 for Unmix), which removed a number of trace-level chemical species not usually associated with pollution sources and with many measurements at or below their MDLs. The influence of chemical species with S/N less than 2.0 was diminished in PMF by multiplying the uncertainties of these data by a factor of 3.
To avoid overweighting the influence of duplicate chemical species in the model results, one of the duplicate species was removed to avoid double counting. In this analysis, S, Na+, K+, and OP by the thermal optical transmittance method were removed, and SO4, Na, K, and OP by the thermal optical reflectance method were retained. The retained chemical species were chosen based on data completeness and S/N ratio.
In order to obtain a feasible Unmix solution with PM2.5 as the normalizing species, additional days were removed from the analysis based on plots of PM2.5 versus the sum of species (Figure S-1, supplementary material) and an evaluation of days affected by fireworks. The following days were removed from the Unmix analysis:
• | Days when the PM2.5 concentration exceeded the sum of species by more than a factor of 2. | ||||
• | Days when the PM2.5 sum of species exceeded the PM2.5 concentration by more than 20%. These days had low PM2.5 concentrations (less than 7 μg/m3). | ||||
• | Days affected by fireworks. Preliminary Unmix runs were unable to identify a fireworks factor. Thus, the high potassium concentrations associated with fireworks would unnecessarily contribute to noise in this species concentration. Therefore, days were removed when K concentrations exceeded the average concentration by a factor of 5 or more and were within 2 days of New Year's Eve, the Fourth of July, or the state holiday, Pioneer Day (July 24). |
In addition, Unmix could not provide a feasible solution with all of the chemical species included in PMF. The subset of species for the Unmix analysis was selected by first identifying species with an average annual concentration of 0.2 μg/m3 and adding species that are suggestive of potentially relevant sources, such as Si for crustal material and K for biomass combustion. The final Unmix species were then selected by trial and error to yield the best solution. The selection criteria included species that led to a solution: having the best fit to the measured data (R 2 value) with PM2.5 set as the normalizing species, containing most of the species with an annual average concentrations of 0.2 μg/m3 or greater, and including species that helped to identify sources of interest.
PM2.5 emission inventory
UDAQ provided the 2008 winter-adjusted and annual Salt Lake County, Utah County (Lindon monitor location), and Davis County (Bountiful monitor location) PM2.5 emission inventories. UDAQ is currently using these inventories to develop control scenarios as part of the Clean Air Act requirement to develop a state implementation plan to bring the area into attainment of the 24-hr PM2.5 National Ambient Air Quality Standard. Consequently, this inventory has been subject to high levels of review and quality assurance.
In addition to daily emissions, the inventories contained categories for source type (point, mobile, and area), county, source classification code, and a description of the sources. Based on this description, the source types were grouped into five categories of primary PM2.5 sources: gasoline, diesel, industrial/urban dust, wood smoke/cooking, and other. Mobile sources of gasoline and diesel emissions were estimated using MOVES 2010A (on-road emissions) and NONROAD 2008A (nonroad emission). For Salt Lake County, diesel contributed 24% and gasoline contributed 74% to mobile emissions with approximately 2% from other sources (i.e., aircraft and natural gas). The inventories did not identify any stationary point sources of diesel or gasoline emissions.
The emission inventories included the following fugitive dust sources: paved and unpaved roads, mining/mineral processing, sand and gravel, and agricultural operations. They did not include wind-blown dust that is associated with undisturbed land, land outside the county, or other activities. The emission factors for fugitive dust estimates were developed from population census numbers, U.S. EPA emission factors, and UDAQ-developed, state-specific emission factors. The inventories also included emissions from wood stoves, fireplaces, and cooking (i.e., commercial char broiling and frying). These emissions were also based on population census numbers and U.S. EPA emission factors. The inventories did not include emissions from prescribed burns, agricultural burns, wildfires, or secondary formation of PM2.5 from the other products of wood burning, that is, volatile organic compounds.
The inventories do not include fireworks or estimates of secondary sources of PM2.5. In addition, not all categories in the inventory match the five primary source categories from the source attributions, which are discussed in the results section. Emission inventory source categories not matched to the source apportionment results include emissions from the use of natural gas, propane, and unspecified fuels, and they accounted for 11% of the Salt Lake County Inventory, 15% of the Utah County Inventory (Lindon), and 25% of the Davis County Inventory (Bountiful).
Theory/calculation
Both PMF and Unmix solve the general receptor modeling problem, given by:
For airborne particles Cij is the jth species concentration (μg/m3) measured in the ith sample, Sik is the mass contribution from source k in the ith sample (e.g., source contribution, μg/m3), and e is the error, which results from variations in the source contribution and analytical uncertainties.
PMF
PMF source apportionment modeling was performed using U.S. EPA PMF 3.0 (CitationNorris et al., 2008). A thorough discussion of the mathematical equations underlying U.S. EPA PMF can be found in Paatero (1997), CitationPaatero (1999), Paatero and Hopke (2003), Reff et al. (2007), CitationNorris et al. (2008), and CitationWang et al. (2012a, 2012b). The model was run in the robust mode using a nonrandom seed value of 10 (so that results were reproducible) and 20 repeat runs to ensure the model least-squares solution represented a global rather than local minimum. The rotational FPEAK variable was held at the default value of 0.0. The model solution with the optimum number of factors was determined somewhat subjectively based on inspection of the factors in each solution, but also from the quality of the least-squares fit (analysis of QRobust and QTrue values) in the model output. The scaled residuals for final model solutions were normally distributed and generally fell into the recommended range of +3 to −3.
Unmix
The U.S. EPA Unmix model 6.0 (U.S. EPA, 2007) was used in this study. The mathematical details of Unmix can be found in CitationHenry (1997, 2002, 2003). Unmix solves the receptor modeling problem (eq 1) using a self-modeling curve resolution algorithm that searches for “edges” in the data that define the jth species mass contribution from the kth source. These edges occur when some samples lack contributions from at least one source or are dominated by contributions from one source for a group of species. The number and direction of the edges derived from Unmix depend on the set of species used, and the solution is sensitive to the species included in the model. It has been successfully employed in various source attribution studies and generates results that compare well with other techniques (CitationHu et al., 2006; CitationChakraborty and Gupta, 2010; CitationMukerjee et al., 2004; CitationSong et al., 2006).
Results and Discussion
This section primarily focuses on the results for Salt Lake City with a brief discussion of the Lindon and Bountiful locations. The reader should note that factors determined in a source-attribution analysis using factor analysis methods like PMF and Unmix are not necessarily mutually exclusive. For example, a factor identified as predominantly ammonium nitrate aerosol is likely from a combination of primary and secondary sources including gasoline engines, diesel engines, and industrial facilities, even though these sources may also have separately identified factors. Hence, caution should be used in interpreting factor classifications too literally or with exclusivity.
PMF results
illustrates the species contributions to the PMF factors for Salt Lake City, and the following sections detail how each of the PMF factors and associated sources were identified. Seasonal variations played a role in the identification of some factors, and monthly PMF factor contributions can be found in the supplementary material (Figure S-2). The PMF factors account for 98% of the PM2.5 mass in Salt Lake City and Lindon, and 97% of the PM2.5 mass in Bountiful. and present the factor profiles for Bountiful and Lindon, respectively.
Figure 2. Species contributions to PMF factor profiles for Salt Lake City. The y-axis units of μg/μg are micrograms of the species divided by the total PM2.5 mass.
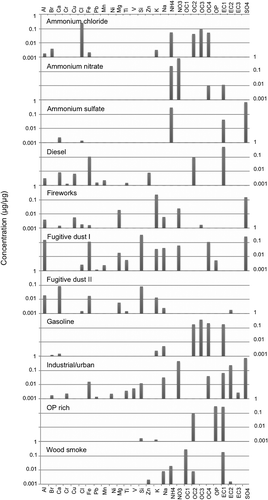
Figure 3. Species contributions to PMF factor profiles for Bountiful. The y-axis units of μg/μg are micrograms of the species divided by the total PM2.5 mass.
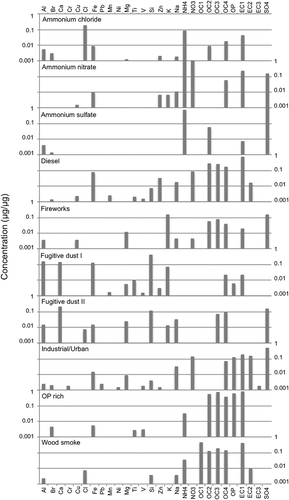
Figure 4. Species contributions to PMF factor profiles for Lindon. The y-axis units of μg/μg are micrograms of the species divided by the total PM2.5 mass.
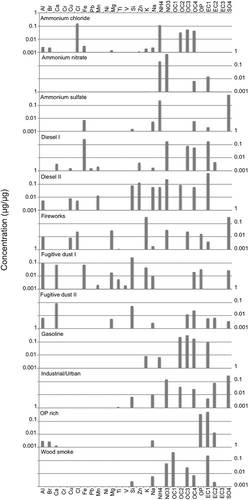
Ammonium chloride factor
A PMF factor predominantly comprising ammonium chloride was identified at all three STN monitoring stations along the Wasatch Front. During winter PM2.5 pollution episodes this factor was significant, contributing 10–15% of total PM2.5 on days when the 24-hr PM2.5 exceeded 30 μg/m3. The presence of significant concentrations of ammonium chloride aerosol in the Wasatch Front airshed has not previously been identified. The study by CitationHansen et al. (2010) did identify elevated aerosol chlorine in their winter 2007 measurements, but lacking cation measurements, they made the assumption that the chlorine was associated with aerosolized sodium chloride from entrained road salt. In the work presented here, we determine that the majority of the chlorine is associated with secondary ammonium chloride rather than primary sodium chloride.
Independent of the PMF results, we performed an ion charge balance analysis on each STN data set that confirmed the presence of ammonium chloride aerosol. This analysis showed that the total measured cationic charge in each sample (sum of charge from Na+, K+, NH4 +) was closely balanced by the total measured anionic charge (sum of charge from Cl-, NO3 -, and SO4 2-), indicating there were no major missing ionic species (). Note that the chloride ion was not directly measured, but total chlorine was assumed to be mostly chloride ion. The ion balance on days with high chlorine mass indicated that the overwhelming majority of chlorine was associated with ammonium due to very low concentrations of both Na+ and K+ (see for the Salt Lake City results and Figures S-3 and S-4 in supplementary materials for the Bountiful and Lindon results, respectively).
Figure 5. Aerosol change balance between anions and cations at three STN monitoring locations along Utah's Wasatch Front. Anion equivalence was calculated as the sum [Cl-]/35.453 + [NO3 -]/62.005 + [SO4 2-]/48.03, and cation equivalence was calculated as the sum [Na+]/23.0 + [K+]/39.098 + [NH4 +]/18.04.
![Figure 5. Aerosol change balance between anions and cations at three STN monitoring locations along Utah's Wasatch Front. Anion equivalence was calculated as the sum [Cl-]/35.453 + [NO3 -]/62.005 + [SO4 2-]/48.03, and cation equivalence was calculated as the sum [Na+]/23.0 + [K+]/39.098 + [NH4 +]/18.04.](/cms/asset/c32a239b-1072-4278-b188-a8035a88d297/uawm_a_774819_o_f0005g.gif)
Figure 6. Cation and anion charge equivalence for the highest measured aerosol Cl days in Salt Lake City.
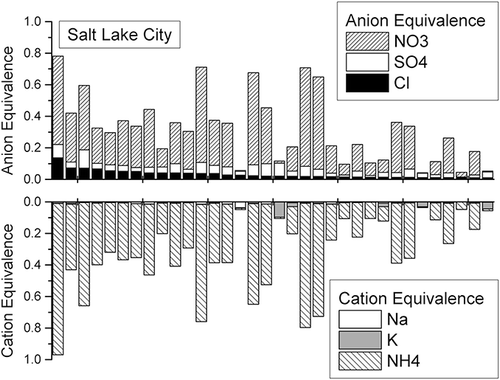
Chang and Allen (2006) describe the equilibrium partitioning between the gas and particle phases when ammonia, nitric acid, sulfuric acid and chloride are all present in an airshed. Ammonia-poor conditions occur when there is insufficient ammonia to neutralize all acid components, and under these conditions equilibrium partitioning will favor the formation of ammonium sulfate over other ammonium salts. Ammonia-rich conditions occur when there is excess ammonia, and there is sufficient ammonia to neutralize all of the acids in the aerosol phase. Several studies have demonstrated that the airshed in and near Salt Lake City is ammonia-rich during winter PM2.5pollution episodes (UDAQ, 2011; CitationMartin, 2006; Mangelson et al., 1997). Also, the charge balance between aerosol anions and cations described earlier indicates that the majority of aerosol acids were neutralized by ammonium.
As previously noted, the work of Pio and Harrison (1987a) reported that the thermodynamics of ammonium chloride aerosol are similar to the thermodynamics of ammonium nitrate. They also estimated that under typical atmospheric concentrations of gaseous ammonia and hydrogen chloride, ammonium chloride aerosol should not be prevalent at temperatures above 10°C. The Wasatch Front results reported here are consistent with those estimates. In our results the majority of ammonium chloride factor mass above 1 μg/m3 occurred when the maximum daily temperature was below 10°C, and all instances of this factor above 3 μg/m3 occur below this temperature ().
Possible sources for chlorine include wintertime road salting, industrial emissions, and biological activity linked to the Great Salt Lake and surrounding marshes. Of the possible sources, road salting was deemed an unlikely source of chlorine given the relative absence of sodium in the observations as well as the absence of reports of ammonium chloride aerosol in other urban areas that conduct road salting in wintertime. Sodium chloride is, however, a well-known source of gas-phase chlorine in coastal and marine areas through the reaction of nitric acid with sodium chloride and the release of hydrogen chloride. Because measured levels of ammonia in the atmosphere during wintertime along the Wasatch Front are high (UDAQ, 2011), atmospheric nitrate is expected to be fully neutralized by ammonia, and nitric acid levels should be extremely low, making the reaction of nitric acid with sodium chloride unlikely.
To address possible sources of chlorine from industrial activity, regional emissions inventories were evaluated and indicated one significant industrial source of chlorine in the Wasatch Front airshed: US Magnesium, Inc., a magnesium production facility that lies approximately 70 km due west of Salt Lake City. The 2008 emissions estimates for US Magnesium, Inc., compiled by the state of Utah for its PM2.5 state implementation plan, reported 579 tons/yr of chlorine air emissions, which made up 96.6% of the total reported anthropogenic chlorine emissions for the airshed. At this reported emission level, screening-level plume dispersion modeling using the National Oceanic and Atmospheric Administration (NOAA) ARL Hysplit online modeling system (CitationDraxler and Rolph, 2012) indicates that chlorine emissions from US Magnesium, Inc., might be a significant contributor to aerosol chlorine observed at the three STN PM2.5 samplers along the Wasatch Front. While more refined plume dispersion modeling is beyond the scope of this study, these screening-level results suggest that a more refined modeling investigation is warranted.
A third possible source for the atmospheric chlorine is biological activity related to the Great Salt Lake. Methyl chloride has been found to be a product of microbial activity in salt marshes and the ocean. CitationRhew et al. (2000) reported significant fluxes of bromomethane and chloromethane in two coastal salt marshes and suggested that salt marshes may contribute up to 10% of the global emissions budget for these methyl halides. While chlorine emission from salt marshes associated with the Great Salt Lake is an intriguing hypothesis for a contributing source of atmospheric chlorine in the Salt Lake airshed, more investigation is necessary to quantify all the contributing sources of chlorine.
Ammonium nitrate factor
This factor was identified by the dominance of nitrate and ammonium. Ammonium nitrate accounts for a significant portion of the average winter PM2.5, approximately 50%, and contributions were as high as 75% on some highly polluted winter days. Figure S-2 indicates that both ammonium nitrate mass and mass fraction were elevated during the winter months and mostly absent during summer. UDAQ has performed monitoring to determine whether ammonia is a limiting factor in the formation of ammonium nitrate and other inorganic secondary PM and has determined that ammonia is present in excess during wintertime, cold-air pool events along the Wasatch Front (UDAQ, 2011).
Ammonium sulfate
This factor was identified by the dominance of sulfate and ammonium. Figure S-2 shows the average monthly mass impacts from this factor and indicates no strong seasonal pattern. This suggests that primary sulfate emissions may be playing a significant role in this factor, rather than secondary photochemical production of sulfate where one would expect a pattern of summertime maxima and winter minima. The most likely sources of sulfate along the Wasatch Front include the petroleum and metals industries, off-highway vehicles, solid-waste incineration, aircraft, and railway equipment. Based on the 2008 inventories, none of these sources are expected to exhibit significant seasonal emission variations.
Diesel and gasoline factors
Both gasoline and diesel factors were identified by the relative contribution of OC and EC to the total mass. For gasoline vehicles, the factor was dominated by OC2, OC3, and OC4, with the EC component mostly EC1. Previous work has also linked similar factors to gasoline vehicles (CitationHwang and Hopke, 2007; CitationKim et al., 2004; CitationMaykut et al., 2003; CitationZhao and Hopke, 2004, 2006; CitationKim and Hopke, 2006; CitationKim and Hopke, 2008b). For diesel emissions, the relatively high proportions of Mn and Fe along with OC and EC are similar to findings in other major urban areas (Kim et al., 2004; CitationMaykut et al., 2003; CitationRamadan et al., 2000; CitationKim and Hopke, 2008a; CitationWu et al., 2007; Amato and Hopke 2011; CitationKim et al., 2010; CitationLewis et al., 2003). The diesel factor showed a statistically significant higher average mean contribution on weekdays versus weekend (Student t-test 90% confidence level).
The monthly impact (Figure S-2) shows that both factors have highest impacts during the cold months of the year: November, December, and January. However, these impacts are likely due to the higher frequency of air-stagnation events and stable atmospheric boundary layers in winter and are not associated with an actual increase in gasoline and diesel emissions in those months. The monthly average fractional contribution of the diesel factor to PM2.5 (Figure S-2) fluctuated around 10% throughout the year. The monthly average fractional contribution of the gasoline factor to PM2.5 ranged between 10 and 20%.
Fugitive Dust I and II factors
Two factors possessing markers associated with airborne crustal materials were identified as Fugitive Dust I and II (Figures ). Although these factors exhibit variation in the contributions of SO4, NO3, and OC4, both factors contained a large fraction of Al, Si, Mg, Ca, Fe, and Ti—the soil elements. Fugitive Dust II was enriched in Ca compared to Fugitive Dust I. While there are similarities in Fugitive Dust I and Fugitive Dust II chemical signatures, Fugitive Dust I and II factors tended to be present in different degrees depending on the time of year. Fugitive Dust I tended to be most active in July–September, while Fugitive Dust II was mostly active during March through July (Figure S-2). Superimposing the wind-speed data on the time-series graphs of the fugitive dust factors shows a moderate correlation between elevated wind speeds and the magnitudes of the factor values. For example, when the average hourly wind speed was 5.4 m/sec (12 mph) or greater, the average fugitive dust contribution was significantly greater than on days with an average hourly wind speed below 5.4 m/sec (Student t-test 95% confidence level). It is worth noting, however, that the exceptionally high wind speeds recorded during winter months resulted in no increase in elevated fugitive dust factor. This is consistent with the understanding that snow cover and high soil-moisture levels prevent significant entrainment of crustal material.
The presence of two separate factors associated with the entrainment of fugitive dust material is most likely associated with the seasonal change in the wind pattern. Similar to the results presented in this work, CitationChen et al. (2010) reported finding two separate dust factors, one calcium-rich and one not, in PMF modeling for receptors in southeastern Minnesota. In their work they attributed potential sources of calcium-rich aerosol to limestone outcroppings in the karst areas of southeastern Minnesota and/or concrete dust from construction activities. In a separate study, PMF modeling conducted by Desert Research Institute (DRI) as part of the Causes of Haze Assessment project (http://www.coha.dri.edu) found two separate soil factors, also one Ca-rich and one not, for monitoring sites at Nevada's Great Basin National Park and Jarbidge Wilderness Area and Utah's Lone Peak Wilderness Area. The findings of two soil factors at the three STN monitors in the greater Salt Lake area in this study as well as DRI's results for three remote locations in Utah and Nevada suggest these two crustal factors represent different soil types, possibly from differing source regions.
Fireworks factor
The chemical fingerprint of the fireworks factor had significant concentrations of potassium, copper, and magnesium, which are metals commonly used in pyrotechnics (CitationVecchi et al., 2008; CitationJoly et al., 2010). The time series of PM2.5 associated with this factor also matched that expected for fireworks use. The largest contributions from this factor fell on sampling days on or near the national holidays of New Year's Day (Janurary 1), Independence Day (July 4), and the state holiday of Pioneer Day (July 24).
Industrial/urban factor
This factor is dominated by a mixture of OC, EC, SO4, and NO3, with trace but significant contributions from Na, V, Ni, Pb, and Cr. The EC2 fraction was particularly important in the model's identification of this factor. Assigning the source of this factor is unclear. Previous work has linked similar factors to a variety of sources including railroad traffic or other combustion-related emissions (Kim et al., 2004; Zhao and Hopke, 2004; Kim and Hopke, 2006; CitationHan et al., 2007). It is likely that this factor is in some way connected to fuel combustion. This factor's time series of monthly average impacts shows a bimodal shape with the two maxima in March and October.
OP-rich factor
This factor was identified by the dominance of the OP species. Numerous other PMF studies that have used OC and EC fraction data based on the thermal evolution protocols developed under the IMPROVE program have also found OP-rich factors (Hwang and Hopke, 2007; CitationZhao and Hopke, 2006; Kim and Hopke, 2006) and have suggested this factor is linked to secondary organic aerosol production (CitationJang et al., 2003).
Wood-smoke factor
The wood-smoke factor was identified by its large OC content, smaller EC content, and majority of mass impacts occurring during the winter months of December and January. It is interesting to note that potassium, commonly associated with combustion of biomaterials, was not identified as a part of this factor. It is possible that potassium's role in other factors (e.g., fugitive dust, fireworks) combined with the relatively small contribution of wood smoke in the overall data set influenced the models lack of attribution of potassium to the wood-smoke factor.
Unmix results
For Salt Lake City, the Unmix solution included 411 observations and 13 species: Fe, Ni, Si, Zn, K, NH4, NO3, OC1, OC2, OC3, OP, EC1, and PM2.5. This solution had a minimum R 2 of 0.92, indicating that at least 92% of the variance of each species can be explained by 5 sources. The Unmix factors accounted for 99% of the PM2.5 mass in Salt Lake City, 98% of the mass in Lindon, and 97% of the mass in Bountiful. summarizes the species contributions to each of the five factors in Salt Lake City. Seasonal variations played a role in the identification of some factors, and monthly Unmix factor contributions can be found in the supplementary material (Figure S-6). and summarize the species contributions for Bountiful and Lindon, respectively. The standardized residuals were normally distributed and generally fell into the recommended range of +3 to −3. Note that chlorine was not included in the Unmix analysis because either Unmix could not find a feasible solution or other important species, like nitrate or several of the OC fractions, had to be omitted in order to obtain a feasible solution. Thus, Unmix could not identify an ammonium chloride factor for any of the Wasatch Front locations.
Figure 7. Species contributions to Unmix factor profiles for Salt Lake City. The y-axis units of μg/μg are micrograms of the species divided by the total PM2.5 mass.
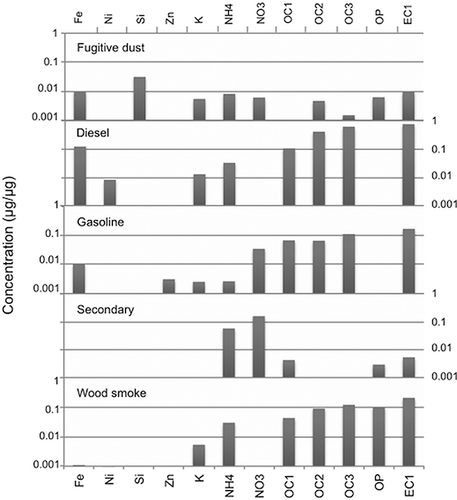
Figure 8. Species contributions to Unmix factor profiles for Bountiful. The y-axis units of μg/μg are micrograms of the species divided by the total PM2.5 mass.
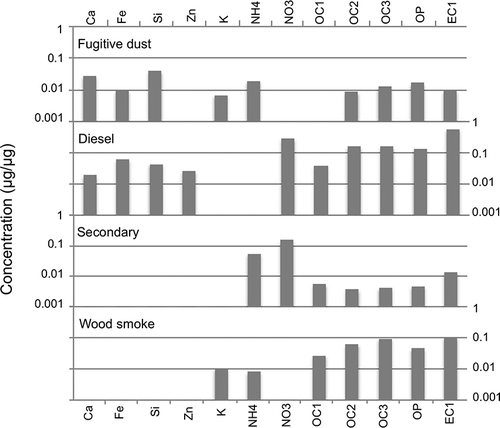
Figure 9. Species contributions to Unmix factor profiles for Lindon. The y-axis units of μg/μg are micrograms of the species divided by the total PM2.5 mass.
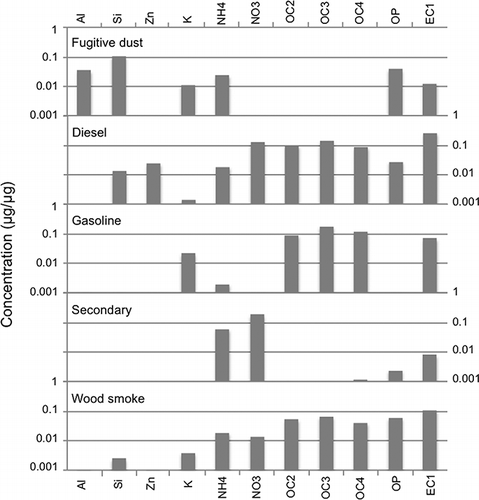
Diesel factor
This factor comprises EC and OC with trace elements of Fe, Zn and/or Ca, depending on the species included in the analysis (Figures ). The EC/OC ratio ranged from 0.67 to 1.1 at the three locations. This factor was slightly more prevalent during winter. However, the weekday contributions from this factor were significantly higher than the weekend contributions (90% confidence interval, unpaired t-test, equal variance). Furthermore when examining the most important days for this factor, none of the top 20 days occurred on a Sunday, suggesting that this factor is related to vehicle traffic.
Fugitive dust factor
This factor comprises Si, small amounts of K, Fe, and Al, and some EC and OC. Because the best solution for each location required a slightly different mix of species, the factor profile for fugitive dust differed slightly at the three monitoring stations. Days when this factor was most important were somewhat associated with higher wind speeds. When the average hourly wind speed was 5.4 m/sec (12 mph) or greater, the average fugitive dust contribution was significantly greater than on days with an average hourly wind speed below 5.4 m/sec (Student t-test 95% confidence level). Based on the factor composition and the seasonal pattern, showing low contributions during the winter, this factor appears to be mostly wind-driven fugitive dust from natural sources, paved roads, and potentially industrial sources. The composition and the seasonal pattern of this factor agree with the PMF factors for Fugitive Dust I and Fugitive Dust II.
Gasoline factor
This factor contained EC and OC with trace amounts of Zn (). It contained less Fe and Ca compared to the diesel factor, and it had a lower EC/OC ratio, ranging from 0.18 to 0.65. It was more prevalent during winter and exhibited a weekday/weekend trend. Although the differences were not statistically significant, when examining the days when this factor is most important, none of the top 20 days included Sundays, suggesting that this factor has some relationship to traffic.
Secondary inorganic aerosol factor
Based on the factor composition, with significant contributions of ammonium and nitrate (), and the strong seasonal pattern (much more common in winter, Figure S-6), this factor appears to represent secondary inorganic pollutant formation, primarily ammonium nitrate. Both the composition and the seasonal contribution of this factor agree with the PMF results.
Wood smoke/aged wood smoke
The importance of K, EC, OC and OP () and the seasonal pattern suggest that this factor is related to wood smoke. The potassium contribution to this factor was 1–2%, and the EC contribution was 14–20%. However, this factor shows an OP contribution of 6–10%, which does not agree with the PMF results. It is possible that this factor represents wood smoke and a portion of the PMF OP-rich factor, which may include aged wood smoke. OP can be a marker for wood smoke and biomass burning; CitationChow et al. (2004) report OP factors for a variety of wood and vegetative burns ranging from 2 to 25%. CitationJeong et al. (2011) identified a biomass-burning factor based on high concentrations of K, OP, and EC1. Chow et al. also suggest that the K/Fe ratio can be used as a tracer for wood smoke, with a range of 3–230. In Salt Lake City, the ratio of K/Fe is 7, which is at least a factor of 10 greater than the other four factors. In Lindon, the ratio is higher than any of the other four factors but was difficult to quantify because the Fe contribution was very close to zero.
The seasonal pattern was also important in identifying this factor (Figure S-6). This factor tended to be important on days associated with wildfires. The contribution of this factor was also higher in the summers of 2007 and 2008 when high wildfire activity occurred in Utah and California. In the summer of 2010, wildfire activity was much lower in this region as was the contributions from this factor. The species found in this factor may also be indicative of wood burning for wintertime heating, cooking, charbroiling/smoking, and frying foods (Chow et al., 2004).
Comparison of PMF, Unmix, and the inventory results
The source attribution results were in close agreement with respect to the large contributions of inorganic secondary PM to PM2.5 along the Wasatch Front. During winter when PM2.5 concentrations exceeded 20 μg/m3 the PMF and Unmix results showed that secondary PM was the largest contributor to PM2.5 (60–67% for Salt Lake City, 72–73% for Lindon, and 64–80% for Bountiful). Examining the year-round results also showed that secondary PM was important, with contributions ranging from 41–43% in Salt Lake City, 41–44% in Lindon, and 40–45% in Bountiful.
Figures 10 and 11 compare the source contributions of primary PM2.5 obtained with PMF, Unmix, and the county emission inventories during winter when PM2.5 concentrations exceeded 20 μg/m3 and for the year-round results, respectively. Note that Salt Lake, Bountiful, and Lindon are located in different counties. The county inventories included only primary PM2.5 emissions and did not attempt to estimate secondary PM2.5. Consequently in order to compare the source-attribution results to the inventories, the source-attribution results were normalized to remove the secondary PM2.5 factors (including the PMF factors ammonium chloride, ammonium nitrate, and ammonium sulfate, and the secondary Unmix factor). Inventory sources that could not be categorized into one of the five primary PM2.5 categories were grouped into the category “other.” The PMF firework factor was grouped in the “other” category.
Although the Unmix solution could not resolve as many factors as the PMF solution, once the PMF factors are grouped, the wintertime Salt Lake City results agreed well with each other, with the exception of the contribution from wood smoke and other. Both PMF and Unmix suggested a greater contribution from wood smoke and OP than the inventory. For the purpose of this analysis, aged wood smoke is considered to be the main contributor to the wintertime OP-rich PMF factor mass. So the wood-smoke contribution here is the sum of the PMF wood smoke and OP-rich factors in order to make the PMF results comparable to the Unmix results. The absence of any secondary PM contributions from wood smoke in the inventory partially, but not fully, explains the discrepancy. It is possible that cooking could be related to the wood-smoke factors. However, even if one sums the wood-smoke emissions and the cooking emissions from the inventory, the inventory accounts for less than half of the PMF- or Unmix-predicted wood-smoke and OP contribution. It is also possible that the location of the Salt Lake City monitor in an older residential area is more influenced by wood burning than is the county as a whole. As part of Utah's plan for meeting the PM2.5 National Ambient Air Quality Standard, it adopted additional restrictions on residential wood burning in December 2012. It will be interesting to see whether the wood-smoke contributions to PM2.5 decrease in future years. When comparing the year-round results (), both PMF and Unmix identified a greater contribution from fugitive dust than the inventory, which is understandable because the inventory does not include wind-blown dust. The inventory also predicts greater contributions from diesel and gasoline vehicles than the source-attribution methods.
Comparison of primary PM2.5 results for Lindon and Bountiful
Unmix and PMF identified similar factors in Salt Lake, Bountiful, and Lindon; however, neither PMF nor Unmix identified primary PM2.5 gasoline exhaust contributions in Bountiful. Note that Unmix was not able to resolve as many factors as PMF.
Lindon
During winter when PM2.5 concentrations exceeded 20 μg/m3, the Unmix, PMF, and inventory results for primary PM2.5 agreed reasonably well with contributions from fugitive dust of approximately 11% of primary PM2.5, from diesel emissions 18–30%, and from gasoline 31–41% (). In addition, PMF results suggest larger contributions from wood smoke (40% of primary PM2.5) compared to the inventory (21%) or Unmix (19%). When considering the year-round emissions, PMF and Unmix suggest greater contributions from fugitive dust compared to the inventory, likely the result of wind-blown dust not being included in the inventory ().
Figure 10. Comparison of primary PM2.5 factor contributions (%) from PMF, Unmix, and the UDAQ inventory, during winter when the average contributions to PM2.5 on days with PM2.5 > 20 μg/m3 (October–March). Because the inventory does not include secondary PM2.5, for this comparison the PMF and Unmix results were normalized to remove the secondary PM2.5 contributions. This inventory represents a typical winter day, that is, January or February (C. Pennell, personal communication, 2012). Note that cooking includes frying and charbroiling.
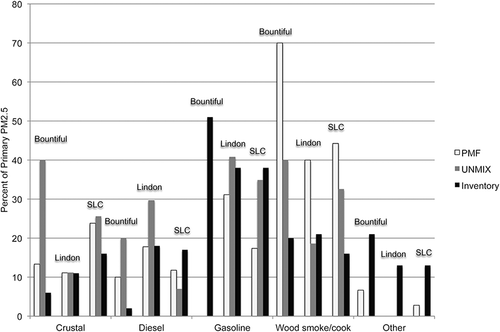
Bountiful
The Bountiful results for primary PM2.5 (PMF, Unmix, and inventory) do not agree as well as either the Salt Lake City or Lindon locations. During winter when PM2.5 concentrations exceeded 20 μg/m3, the contributions had ranges of 6–40% of the primary portion of PM2.5 for fugitive dust, 2–20% for diesel, 0–51% for gasoline, and 20–70% for wood smoke (). It is unclear whether the lack of agreement is due to the inability to include OP among the species in the Unmix solution, or the need to remove more dates to obtain a feasible solution with PM2.5 as the normalizing species. Neither Unmix nor PMF could identify a primary gasoline factor at this location, although the inventory identifies 50% and 38% of contributions from gasoline sources during the winter and year-round, respectively. The source attribution methods suggest a greater contribution from fugitive dust sources, ranging from double to greater than a factor of 6 (year-round) ().
Conclusion
Two factor analysis techniques, PMF and Unmix, were used to identify contributors to PM2.5 at three monitoring stations along Utah's Wasatch Front: Bountiful, Lindon, and Salt Lake City. During wintertime cold-pool events, both source attribution methods identified inorganic secondary aerosols as the main contributors to PM2.5 concentrations (60–80%, all locations), with secondary ammonium nitrate being the dominant contributor. In addition to ammonium nitrate, PMF identified a separate factor linked to secondary ammonium chloride, and this factor was a significant contribution to the total PM2.5 aerosol mass during wintertime PM2.5 pollution events along the Wasatch Front (10–15% of total PM2.5 on days when the 24-hr PM2.5 exceeded 30 μg/m3). Subsequent ion balance analysis of the monitoring data confirmed the presence of significant ammonium chloride aerosol on these highly polluted days at all three monitoring sites. The presence of significant ammonium chloride aerosol is unusual and has not previously been reported for this airshed. Sources of chlorine in the airshed are not well understood, but could be related to industrial activity and/or biogenic emissions from the Salt Lake.
Although Unmix identified fewer factors than PMF, after grouping some of the PMF factors, the source attribution results for the primary portion of PM2.5 generally agreed with the emissions inventories for the region. The source attributions for primary PM2.5 suggest that wood smoke and cooking may be as important as gasoline emissions during the wintertime cold-pool events in Salt Lake City, perhaps due to the location of the sampling station in a residential area. During spring through fall, fugitive dust emissions may be more important contributors to primary PM2.5 emissions than the inventory suggests. This may be due to the omission of wind-blown dust in the inventories and the difficulty in obtaining accurate estimates of fugitive dust emissions in an arid region when emissions are highly dependent on meteorological conditions as well as activity levels.
Supplementary Material
Download MS Word (13.4 MB)Acknowledgment
Thanks to Joel Karmazyn and Barbara McMurtrie, UDAQ, who provided the 2008 emission inventory and guidance on its interpretation. Many thanks to Dr. Cristina Jaramillo, University of Utah Department of Chemical Engineering, for her insights on factor analysis.
References
- Amato , F. and Hopke , P.K. 2012 . Source apportionment of the ambient PM2.5 across St. Louis using constrained positive matrix factorization . Atmos. Environ , 46 : 329 – 37 . doi: 10.1016/j.atmosenv.2011.09.062
- Baliff , M. , Oh , J. , Allen , J.A. , Monahan , G. and Dockery , D.W. 2000 . Air pollution and incidence of cardiac arrhythmia . Epidemiology , 11 : 11 – 17 .
- Begum , B.A. , Hopke , P.K. and Zhao , W. 2005 . Source identification of fine particles in Washington, DC, by expanded factor analysis modeling . Environ. Sci. Technol , 39 : 1129 – 37 . doi: 10.1021/es049804v
- Beard , J.C. , Beck , C. , Graham , R. , Packham , S.C. , Traphagan , M. , Giles , R.T. and Morgan , J. G. 2012 . Winter temperature inversions and emergency department visits for asthma in Salt Lake City, Utah, 2003–2008 . Environ. Health Perspect , 120 ( 10 ) : 1385 – 90 .
- Brown, S.G., and H.R. Hafner. 2005. Multivariate Receptor Modeling Workbook. Sonoma Technology, Inc., Petaluma, CA, prepared for the U.S. Environmental Protection Agency, Office of Research and Development, Research Triangle Park, NC, September http://www.sonomatechdata.com/sti_workbooks/#MVRMWB (http://www.sonomatechdata.com/sti_workbooks/#MVRMWB)
- Chang , S. and Allen , D.T. 2006 . Chlorine chemistry in urban atmospheres: Aerosol formation associated with anthropogenic chlorine emissions in southeast Texas . Atmos. Environ. , 40 : S512 – 23 . doi: 10.1016/j.atmosenv.2006.04.070
- Chen , L.W.A. , Watson , J.G. , Chow , J.C. , DuBois , D.W. and Herschberger , L. 2010 . Chemical mass balance source apportionment for combined PM2.5 measurements from U.S. non-urban and urban long-term networks . Atmos. Environ. , 44 : 4908 – 18 .
- Chung , S.H. and Seinfeld , J.H. 2002 . Global distribution and climate forcing of carbonaceous aerosols . J. Geophys. Res , 107 : 4407 – 40 . doi: 10.1029/2001JD001397
- Chakraborty , A. and Gupta , T. 2010 . Chemical characterization and source apportionment of submicron (PM1) aerosol in Kanpur region, India . Aerosol Air Quality Res. , 10 : 433 – 45 . doi: 10.4209/aaqr.2009.11.0071
- Chow , J.C. , Watson , J.G. , Kuhns , H. , Etyemezian , V. , Lowenthal , D.H. , Crow , D. , Kohl , S.D. , Engelbrecht , J.P. and Green , M.C. 2004 . Source profiles for industrial, mobile, and area sources in the Big Bend regional aerosol visibility and observational study . Chemosphere , 54 : 185 – 208 . doi: 10.1016/j.chemosphere.2003.07.004
- Draxler, R.R., and G.D. Rolph, 2012. HYSPLIT (HYbrid Single-Particle Lagrangian Integrated Trajectory) model access via NOAA ARL READY website. NOAA Air Resources Laboratory, Silver Spring, MD http://ready.arl.noaa.gov/HYSPLIT.php (http://ready.arl.noaa.gov/HYSPLIT.php) (Accessed: Oct2012) ).
- DRI [Desert Research Institute]. 2008. DRI model 2001 thermal/optical carbon analysis (TOR/TOT) of aerosol filter samples—Method IMPROVE_A. DRI SOP #2-216r2, revised July 2008 http://www.epa.gov/ttnamti1/files/ambient/pm25/spec/DRI_SOPforIMPROVEAFINAL.pdf (http://www.epa.gov/ttnamti1/files/ambient/pm25/spec/DRI_SOPforIMPROVEAFINAL.pdf) (Accessed: 15 December 2012 ).
- Dockery , D.W. , Pope , A.C. , Xiping , X. , Spengler , J.D. , Ware , J.H. , Fay , M.E. , Ferris , B.G. and Speizer , F.E. 1993 . An association between air pollution and mortality in six U.S. cities . N. Engl. J. Med , 329 : 1753 – 59 . doi: 10.1056/NEJM199312093292401
- Du , H. , Kong , L. , Cheng , T. , Chen , J. , Yang , X. , Zhang , R. , Han , Z. , Yan , Z. and Ma , Y. 2010 . Insights into ammonium particle-to-gas conversion: Non-sulfate ammonium coupling with nitrate and chloride . Aerosol Air Quality Res. , 10 : 589 – 95 . doi: 10.4209/aaqr.2010.04.0034
- Grover , B.D. , Carter , C.B. , Kleinman , M.A. , Richards , J.S. , Eatough , N.L. , Eatough , D.J. , Dasgupta , P.K. , Al-Horr , R. and Rahmat Ullah , S.M. 2003 . Monitoring and source apportionment of fine particulate matter at Lindon, Utah . Aerosol Sci. Technol. , 40 (10) : 941 – 51 . doi: 10.1080/02786820600815424
- Han , Y. , Cao , J. , Chow , J.C. , Watson , J.G. , An , Z. , Jin , Z. , Fung , K. and Liu , S. 2007 . Evaluation of the thermal/optical reflectance method for discrimination between char- and soot-EC . Chemosphere , 69 : 569 – 74 . doi: 10.1016/j.chemosphere.2007.03.024
- Hansen , J.C. , Woolwine , W.R. III , Bates , L. , Clark , B. , Kuprov , R.Y. , Mukherjee , P. , Murray , J.A. , Simmons , M.A. , Waite , M.F. , Eatough , N.L. , Eatough , D.L. , Long , R. and Grover , B.D. 2010 . Semicontinuous PM2.5 and PM10 mass and composition measurements in Lindon, Utah, during winter 2007 . J. Air Waste Manage. Assoc. , 60 : 346 – 55 . doi: 10.3155/1047-3289.60.3.346
- Henry , R.C. 1997 . History and fundamentals of multivariate air quality receptor models . Chemometr. Intell. Lab. , 37 : 37 – 42 . doi: 10.1016/S0169-7439(96)00048-2
- Henry , R.C. 2002 . Multivariate receptor models—Current practice and future trends . Chemometr. Intell. Lab. , 60 : 43 – 48 . doi: 10.1016/S0169-7439(01)00184-8
- Henry , R.C. 2003 . Multivariate receptor modeling by N-dimensional edge detection . Chemometr. Intell. Lab. , 65 : 179 – 89 . doi: 10.1016/S0169-7439(02)00108-9
- Hu , S. , McDonald , R. , Martuzevicius , D. , Biswas , P. , Grinshpun , S.A. , Kelley , A. , Reponen , T. , Lockey , J. and LeMasters , G. 2006 . UNMIX modeling of ambient PM2.5 near an interstate highway in Cincinnati, OH, USA . Atmos. Environ , 40 : S378 – 95 . doi: 10.1016/j.atmosenv.2006.02.038
- Hwang , I.J. and Hopke , P.K. 2007 . Estimation of source apportionment and potential source locations of PM 2.5 at a west coastal IMPROVE site . Atmos. Environ , 41 : 506 – 18 . doi: 10.1016/j.atmosenv.2006.08.043;
- Jang , M. , Lee , S. and Kamens , R.M. 2003 . Organic aerosol growth by acid-catalyzed heterogeneous reactions of octanal in a flow reactor . Atmos. Environ , 37 : 2125 – 38 . doi: 10.1016/S1352-2310(03)00077-3
- Jeong , C.H. , McGuire , L.M. , Herod , D. , Dann , T. , Dabek Zlotorzynska , E. , Wang , D. , Ding , L. , Celo , V. , Mathieu , D. and Evans , G. 2011 . Receptor model based identification of PM2.5 sources in Canadian cities . Atmos. Pollut. Res. , 2 : 158 – 71 . doi: 10.5094/APR.2011.021
- Joly , A. , Smargiassi , A. , Kosatsky , T. , Fournier , M. , Dabek-Zlotorzynska , E. , Celo , V. , Mathieu , D. , Servranckx , R. , D'amours , R. , Malo , A. and Brook , J. 2010 . Characterisation of particulate exposure during fireworks displays . Atmos. Environ. , 44 : 4325 – 29 . doi: 10.1016/j.atmosenv.2009.12.010
- Kim , E. , Hopke , P.K. and Edgerton , E.S. 2004 . Improving source identification of Atlanta aerosol using temperature resolved carbon fractions in positive matrix factorization . Atmos. Environ. , 38 : 3349 – 62 . doi: 10.1016/j.atmosenv.2004.03.012
- Kim , E. , Hopke , P.K. and Qin , Y. 2005 . Estimation of organic carbon blank values and error structures of the speciation trends network data for source apportionment . J. Air Waste Manage. Assoc. , 55 : 1190 – 99 . doi: 10.1080/10473289.2005.10464705
- Kim , E. and Hopke , P.K. 2006 . Characterization of fine particle sources in the Great Smoky Mountains area . Sci. Tot. Environ , 368 : 781 – 94 . doi: 10.1016/j.scitotenv.2006.02.048
- Kim , E. and Hopke , P.K. 2008a . Source characterization of ambient fine particles at multiple sites in the Seattle area . Atmos. Environ , 42 : 6047 – 56 . doi: 10.1016/j.atmosenv.2008.03.032
- Kim , E. and Hopke , P.K. 2008b . Characterization of ambient fine particles in the northwestern area and Anchorage. Alaska . J. Air Waste Manage. Assoc. , 58 : 1328 – 40 . doi: 10.3155/1047-3289.58.10.1328
- Kim , E. , Turkiewicz , K. , Zulawnick , S.A. and Magliano , K.L. 2010 . Sources of fine particles in the South Coast area, California . Atmos. Environ , 44 : 3095 – 100 . doi: 10.1016/j.atmosenv.2010.05.037
- Lewis , C.W. , Norris , G.A. , Henry , R.C. and Conner , T.L. 2003 . Source apportionment of Phoenix PM2.5 aerosol with the Unmix receptor model . J. Air Waste Manage. Assoc. , 53 : 325 – 38 .
- Malek , E. , Davis , T. , Martin , R.S. and Silva , P.J. 2006 . Meteorological and environmental aspects of one of the worst National air pollution episodes (January, 2004) in Logan, Cache Valley, Utah, USA . Atmos. Res , 79 : 108 – 22 . doi: 10.1016/j.atmosres.2005.05.003
- Martin, R. 2006. Cache Valley air quality studies. August http://www.airquality.utah.gov/Public-Interest/Current-Issues/cache-valley-PM/Outreach/Cache%20Valley%20Air%20Quality%20Studies%202006.pdf (http://www.airquality.utah.gov/Public-Interest/Current-Issues/cache-valley-PM/Outreach/Cache%20Valley%20Air%20Quality%20Studies%202006.pdf) (Accessed: October 2012 ).
- Mangelson , N.F. , Lewis , L. , Joseph , J.M. , Cui , W. , Machir , J. , Eatough , D.J. , Rees , L.B. , Wilkerson , T. and Jensen , D.T. 1997 . The contribution of sulfate and nitrate to atmospheric fine particles during winter inversion fogs in Cache Valley, Utah . J. Air Waste Manage. Assoc , 47 : 167 – 75 . doi: 10.1080/10473289.1997.10464429
- Maykut , N. , Lewtas , J. , Kim , E. and Larson , T. 2003 . Source apportionment of PM2.5 at an urban IMPROVE site in Seattle, Washington . Environ. Sci. Technol. , 37 : 5135 – 42 .
- Mukerjee , S. , Norris , G.A. , Smith , L.A. , Noble , C.A. , Neas , L.M. , Ozkaynak , A.H. and Gonzales , M. 2004 . Receptor model comparisons and wind direction analyses of volatile organic compounds and submicrometer particles in an arid, binational, urban air shed . Environ. Sci. Technol , 38 : 2317 – 27 . doi: 10.1021/es0304547
- Nicolai , T. , Carr , D. , Weiland , S.K. , Duhme , H. , von Ehrenstein , O. , Wagner , C. and von Mutius , E. 2003 . Urban traffic and pollutant exposure related to respiratory outcomes and atopy in a large sample of children . Eur. Respir. J , 21 : 956 – 63 . doi: 10.1183/09031936.03.00041103a
- Norris, G., R. Vedantham, K.Wade, S. Brown, J. Prouty, and C. Foley. 2008. EPA Positive Matrix Factorization (PMF) 3.0 fundamentals & user guide. EPA 600/R-08/108 http://www.epa.gov/heasd/products/pmf/pmf.html (http://www.epa.gov/heasd/products/pmf/pmf.html)
- Paatero , P. 2007 . Least squares formulation of robust non-negative factor analysis . Chemometr. Intell. Lab , 37 : 23 – 35 . doi: 10.1016/S0169-7439(96)00044-5
- Paatero , P. 1999 . The multilinear engine e a table-driven least squares program for solving multilinear problems, including the n-way parallel factor analysis model . J. Comput. Graph. Stat. , 8 ( 4 ) : 854 – 88 .
- Paatero , P. and Hopke , P.K. 2003 . Discarding or downweighting high-noise variables in factor analytic models . Anal. Chim. Acta , 490 : 277 – 89 . doi: 10.1016/S0003-2670(02)01643-4
- Pio , C. and Harrison , R. 1987a . The equilibrium of ammonium chloride aerosol with gaseous hydrochloric acid and ammonia under tropospheric conditions . Atmos. Environ , 21 : 1243 – 46 . doi: 10.1016/0004-6981(87)90253-8
- Pio , C. and Harrison , R. 1987b . Vapor pressure of ammonium chloride aerosol: effect of temperature and humidity . Atmos. Environ. , 21 : 2711 – 15 .
- Pope , C.A. , Dockery , D.W. , Spengler , J.D. and Raizenne , M.E. 1991 . Respiratory health and PM10 pollution. A daily time series analysis . Am. Rev. Respir. Dis , 144 : 668 – 74 . doi: 10.1164/ajrccm/144.3_Pt_1.668
- Possanzini , M. , Masia , P. and Palo , V.D. 1992 . Speciation of ammonium-containing species in atmospheric aerosols . Atmos. Environ , 26A : 1995 – 2000 . doi: 10.1016/0960-1686(92)90084-X
- Ramadan , Z. , Song , X.-H. and Hopke , P.K. 2000 . Identification of sources of Phoenix aerosol by positive matrix factorization. J . Air Waste Manage. Assoc , 50 : 1308 – 20 . doi: 10.1080/10473289.2000.10464173
- Reff , A. , Eberly , S.I. and Bhave , P.V. 2007 . Receptor modeling of ambient particulate matter data using positive matrix factorization: review of existing methods . Air Waste Manage. Assoc , 57 : 146 – 54 . doi: 10.1080/10473289.2007.10465319
- Rhew , R.C. , Miller , B.R. and Weiss , R.F. 2000 . Natural methyl bromide and methyl chloride emissions from coastal salt marshes . Nature , 403 : 292 – 95 .
- Song , Y. , Shaodong , X. , Yuanhang , Z. , Limin , Z. , Lynn , G.S. and Mei , Z. 2006 . Source apportionment of PM2.5 in Beijing using principal component analysis/absolute principal component scores and UNMIX . Sci. Total Environ , 372 : 278 – 86 . doi: 10.1016/j.scitotenv.2006.08.041
- U.S. Environmental Protection Agency . 2007 . EPA Unmix 6.0 fundamentals and user guide , Research Triangle Park , NC : U.S. Environmental Protection Agency . EPA/600/R-07/089
- U.S. Environmental Protection Agency. 2012. Chemical management—Data management and reporting http://www.epa.gov/ttn/amtic/specdat.html (http://www.epa.gov/ttn/amtic/specdat.html) (Accessed: 8 February 2012 ).
- Utah Division of Air Quality. 2011. PM2.5 state implementation plan modeling protocol, Final version 2.2 www.airquality.utah.gov/Public-Interest/Current-Issues/pm2.5/pdf/June-2011/Protocol-V.2.2.pdf (http://www.airquality.utah.gov/Public-Interest/Current-Issues/pm2.5/pdf/June-2011/Protocol-V.2.2.pdf) (Accessed: 15 July 2012 ).
- Vecchi , R. , Bernardoni , V. , Cricchio , D. , D'Alessandro , A. , Fermo , P. , Lucarelli , F. , Nava , S. , Piazzalunga , A. and Valli , G. 2008 . The impact of fireworks on airborne particles . Atmos. Environ , 42 : 1121 – 32 . doi: 10.1016/j.atmosenv.2007.10.047
- Wang , Y. , Hopke , P.K. , Xia , X. , Rattigan , O.V. , Chalupa , D.C. and Utell , M.J. 2012a . Multiple year black carbon measurement and source apportionment using Delta-C in Rochester, NY . J. Air Waste Manage. Assoc , 62 ( 8 ) : 880 – 87 . doi: 10.1080/10962247.2012.671792
- Wang , Y. , Hopke , P.K. , Xia , X. , Rattigan , O.V. , Chalupa , D.C. and Utell , M.J. 2012b . Source apportionment of airborne particulate matter using inorganic and organic species as tracers . Atmos. Environ , 55 : 525 – 32 . doi: 10.1016/j.atmosenv.2012.03.073
- Watson , J.G. 2002 . Visibility: science and regulation . J. Air Waste Manage. Assoc , 52 : 628 – 713 . doi: 10.1080/10473289.2002.10470813
- Watson, J.G., J.C., Chow, and L.-W. A. Chen. 2008. Assessment of carbon sampling artifacts in the IMPROVE, STN/CSN, and search networks. For U.S. EPA, Office of Air Quality Planning and Standards, August 22 http://www.epa.gov/airtrends/specialstudies/20080822_sampling_artifact_rev.pdf (http://www.epa.gov/airtrends/specialstudies/20080822_sampling_artifact_rev.pdf) (Accessed: 12 December 2012 ).
- Wu , C.-F. , Larson , T.V. , Wu , S.-Y. , Williamson , J. , Westberg , H.H. and Liu , L.-J. 2007 . Source apportionment of PM2.5 and selected hazardous air pollutants in Seattle . Sci. Tot. Environ , 386 : 42 – 52 . doi: 10.1016/j.scitotenv.2007.07.042
- Yoshizumi , K. and Okita , T. 1983 . Quantitative estimation of sodium- and ammonium-nitrate, ammonium chloride, and ammonium sulfate in ambient particulate matter . J. Air Pollut. Cont. Assoc , 33 : 224 – 26 . doi: 10.1080/00022470.1983.10465570
- Zhao , W. and Hopke , P.K. 2004 . Source apportionment for ambient particles in the San Gorgonio wilderness . Atmos. Environ , 38 : 5901 – 10 . doi: 10.1016/j.atmosenv.2004.07.011
- Zhao , W. and Hopke , P.K. 2006 . Source identification for fine aerosols in Mammoth Cave National Park . Atmos. Res , 80 ( 4 ) : 309 – 322 . doi: 10.1016/j.atmosres.2005.10.002
Supplemental Materials
Supplemental materials are available for this paper. Go to the publisher's online edition of the Journal of the Air & Waste Management Association.