Abstract
When annual average PM2.5 (fine particulate matter sized 2.5 microns and less) data for 2005 became available in April 2006 and the 3-yr average PM2.5 concentration in an area just north of the Houston Ship Channel reached 15.0 µg/m3, the Texas Commission on Environmental Quality (TCEQ) initiated daily collection of quartz fiber as well as Teflon PM2.5 filter samples for chemical speciation analysis. The purpose of the chemical speciation analysis was to use the speciation data, together with meteorological data and hourly TEOM (tapered element oscillating microbalance) PM2.5 mass data, to identify the causes of the high PM2.5 concentrations affecting the monitoring site and the neighborhood. The ultimate purpose was to target emission reduction efforts to sources contributing to the high measured PM2.5 concentrations. After a year of data collection, it was recognized that a specific source, unpaved driveways and loading areas along the Ship Channel and dirt tracked onto Clinton Drive, the main artery running east-west north of the Ship Channel, were the primary cause for the Clinton Drive site's measuring PM2.5 concentrations significantly higher than other sites in Houston. The source characterization and remediation steps that have led to sustained reduced concentrations are described in this paper.
With PM2.5 exceedances it can be essential to have or develop chemical speciation data as part of the process of identifying the source types causing exceedances of an annual standard. Positive matrix factorization (PMF) analysis proved to be a powerful tool that identified the two locally emitted species contributing to exceedances, which did not occur at other sites in the region. They were calcium sulfate (gypsum), an industrial by-product, and soil minerals. Other data analysis approaches were necessary to distinguish North African dust events, which PMF failed to identify.
Introduction
Numerous studies have shown health effects including excess mortality rates from exposure to fine particulate matter (CitationLaden et al., 2000; CitationDockery, 2001; CitationPope and Dockery, 2006). Since 1997, the U.S. Environmental Protection Agency (EPA) has regulated PM2.5 (fine particulate matter sized 2.5 microns and less) as sampled by a federal reference or federal equivalent monitor (CitationEPA, 1997). Until 2012, the National Ambient Air Quality Standard (NAAQS) required that the 3-yr average PM2.5 concentration not exceed 15.0 micrograms per cubic meter (μg/m3). When annual average PM2.5 data for 2005 became available in April 2006 and the 3-yr average PM2.5 concentration in an area just north of the Houston Ship Channel reached 15.0 µg/m3 at one monitoring site, the Texas Commission on Environmental Quality (TCEQ) initiated daily collection of quartz fiber as well as Teflon PM2.5 filter samples for chemical speciation analysis at that site. The purpose of the chemical speciation analysis was to use the speciation data, together with meteorological data and hourly TEOM (tapered element oscillating microbalance) PM2.5 mass data, to identify the causes of the high PM2.5 concentrations affecting the monitoring site and the neighborhood. The ultimate purpose was to target emission reduction efforts to sources contributing to the local-scale high measured PM2.5 concentrations.
Earlier research had been conducted to characterize the nature of particulate matter within the meso-scale Southeast Texas and Houston metropolitan area. A study preceding the 1999 NAAQS promulgation in 1997–1998 provided initial characterization of organic species and an assessment of partitioning between sulfates, organics, and crustal material (CitationFraser et al., 2002). The 1999 EPA-funded Gulf Coast Aerosol Research and Characterization Program (Houston Supersite) (CitationRussell et al., 2004) and the 2005–2006 Second Texas Air Quality Study (CitationParrish et al., 2009) were two large-scale studies that helped to quantify the transport of aerosols and precursors and the formation and size distribution of particles in the Houston area. Specific factors found to affect the area included North African dust and ocean-going ship emissions (CitationBates et al., 2008), and local industrial emissions (CitationBahreini et al., 2009).
In contrast to earlier meso-scale studies to characterize PM2.5, this project involved a local-scale issue. The Clinton Drive monitoring site is the site that was measuring the high PM2.5 concentrations and is at the south edge of Galena Park, the community just north of the Houston Ship Channel, the Port of Houston Authority property, and the industrial areas along the Houston Ship Channel. This monitoring site has historically measured the highest total suspended particulate matter (TSP), respirable particulate matter (PM10; sized 10 micron and less), and PM2.5 concentrations of any monitoring site in North, East, or South Texas. The area was designated attainment for both the 24-hr and the annual PM2.5 NAAQS after the first few years of sampling. However, the 15.0 μg/m3 3-yr average for 2003–2005 at the Clinton Drive site was at the level of the annual PM2.5 NAAQS. In addition, continuous PM2.5 monitoring data collected by a TEOM at the site indicated that the PM2.5 concentrations were continuing to increase.
A characterization of PM2.5 composition from data collected over a 12-month period during 1997–1998 described by CitationFraser et al. (2003) suggested that the Clinton Drive site had higher exposure to vehicle sources and fuel-oil combustion than other Houston sites. As is shown in this paper, the characterization of composition factors has changed over time. Specifically, after a year of speciated data collection starting in 2005 at Clinton Drive under the current study, we recognized that specific sources—unpaved driveways and loading areas along the Ship Channel and dirt tracked onto Clinton Drive, the main artery running east-west north of the Ship Channel—were the primary cause that the Clinton Drive site was measuring PM2.5 concentrations significantly higher than other sites in Houston. The source characterization and remediation steps that have led to sustained reduced concentrations are described below.
Methods and Results
The approach taken in this study began with augmented sampling at the Clinton Drive site in 2005. As data accumulated, multivariate source apportionment using reconstruction formulas suggested in the literature, as well as original principal component analysis, positive matrix factorization, meteorological assessments, and between-site comparisons, was conducted. The sampling and the data analyses led to the targeted remediation and subsequent improvement in air quality at the site.
Field sampling and monitoring
There are three federal reference method (FRM) Rupprecht & Patashnick (R&P) automated, sequential 2025 samplers at the Clinton Drive sampling site operated by the TCEQ. The first one, for our purposes labeled FRM-1, has collected daily, 24-hr samples on a Teflon filter for gravimetric analysis and comparison of the PM2.5 concentrations with the PM2.5 NAAQS since the beginning of routine PM2.5 monitoring in Texas in 1999. The second FRM, labeled FRM-QC, is a collocated, quality control (QC) sampler that collects samples on Teflon filters on the national every-sixth-day schedule. The third FRM sampler, labeled FRM-Spec, which was installed for the special study PM2.5 chemical speciation work in 2006, collects daily quartz fiber filter samples for analysis of organic carbon (OC) and elemental or black carbon (EC). Since the 2006 installation of the FRM-Spec sampler, all valid samples from either FRM-1 or FRM-QC on the EPA's every-sixth-day sampling schedule were analyzed for elements and ions, and if there was a corresponding valid sample from FRM-Spec, for OC and EC as well. In addition, each quarter of the year, a number of additional valid FRM-1 or FRM-QC samples and corresponding valid FRM-Spec samples were selected for speciation based on elevated FRM-1 mass or FRM-1 mass higher than surrounding PM2.5 samplers in the Houston area, or for other reasons such as to assess background concentrations or the influence of special events such as fires or transported dust. All of the special study gravimetric and chemical speciation analyses for samplers FRM-1, FRM-QC, and FRM-Spec were carried out by the Desert Research Institute (DRI) in Reno Nevada, a part of the Nevada System of Higher Education (CitationTropp et al., 2006). R&P FRM 2025 samplers operate at a 16.7 L/min flow rate onto 47-mm filters, which allows collection on Teflon or quartz filters. The FRM-1 and FRM-QC instruments took Teflon filter samples, which were used for determining mass by gravimetric analysis, 48 elements by X-ray florescence (XRF) and six ions by ion chromatography (IC). The FRM-Spec instrument took quartz filter samples, which were used to measure elemental carbon, organic carbon, and carbon fractions. From 2000 through 2009 the standard EC/OC analysis method was a thermo-optical transmittance (TOT) method. Beginning in 2010, carbon analysis has been performed using the thermo-optical reflectance (TOR) method (CitationTropp, 2009).
Additional instruments at the Clinton Drive site include a continuous TEOM analyzer operated by the City of Houston providing PM2.5 mass at a 1-hr time resolution; a PM10 HiVol 24-hr sampler operated by The City of Houston on an every-third-day sampling schedule, for which the filters are analyzed using XRF for elemental composition; and a meteorological station operated by The City of Houston providing 5-min and 1-hr time resolution wind data (resultant speed and direction).
shows a Google Earth map of the Clinton Drive monitoring site location and the surrounding area.
Data interpretations
The data available for interpretation included the FRM-1 PM2.5 total mass collected since 2000, total PM2.5 mass and speciation data from five other local area samplers, total PM2.5 mass for nine local area TEOMs, and meteorological data. The multivariate nature of the PM2.5 speciation data makes it amenable to multivariate statistical methods of principal component analyses and positive matrix factorization (Watson et al., 2006; CitationReff et al., 2007).
shows a line graph of the mean PM2.5 concentration at Clinton Drive by quarter from 2000 through June 2012 using daily FRM mass. The data presented this way show the seasonal periodicity of concentrations with summer peaks and winter troughs, as well as the rise in mean concentrations peaking in 2004–2006, followed by a decline. shows a similar presentation of PM10 concentration at Clinton Drive. The data show the same seasonal periodicity with mean concentrations peaking in 2006, followed by a decline in concentrations. This seasonal variation has also been observed in the PM2.5 data at other samplers in the Texas monitoring network, and matches similar findings by CitationChen et al. (2012), and likely factors include the higher levels of transported U.S. continental haze in the summer, contributions of transported sulfate from higher levels of activity at coal-burning power plants, and transported North African dust.
We merged the TEOM hourly PM2.5 and PM10 24-hr average mass concentrations with hourly wind speed and direction data. By averaging the mean concentrations as a function of direction and wind speed, we were able to show that higher concentrations were present under higher wind speeds, and that southerly winds were associated with higher concentrations for both PM2.5 and PM10. The TEOM PM2.5 mass was averaged by hour of the day and day of the week, which determined that the high PM2.5 concentrations were occurring during workday hours on weekdays. All of these results were consistent with wind-blown dust from unpaved driving surfaces.
PM2.5 monitoring with TEOM analyzers is more common in Texas (although not a federal equivalent method) than filter sampling, because TEOM data are relatively easier and less expensive to obtain and thus such data can be compared across more monitoring sites. shows a comparison in the mean concentration of the PM2.5 from the TEOM instruments at Clinton Drive and eight other monitoring sites in the Houston metro area for 2006. The difference between the 15.8 μg/m3 annual mean at Clinton Drive in 2006 and the next highest site, the Houston East monitoring site 3 miles to the northeast of Clinton Drive, was 2.6 μg/m3 and the average difference between Clinton Drive and all eight sites was 4.1 μg/m3.
Figure 4. Mean TEOM (not an FEM) PM2.5 concentration at Clinton Drive compared with other sites, calendar year 2006.
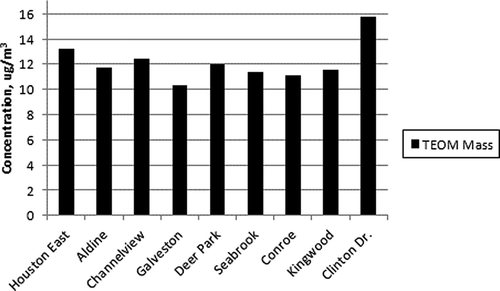
Using the PM2.5 speciation data and the DRI PM2.5 mass reconstruction equations initially provided key information about the difference between PM2.5 composition at the Clinton Drive site and other PM2.5 chemical speciation monitoring sites in the Houston area. The DRI soil reconstruction equation (CitationTropp et al., 2006) is given in Equationeq 1:
EquationEquation 1 uses oxides in the forms of Al2O3, SiO2, CaO, and Fe2O3, respectively; modified from CitationMacias et al. (1981a, 1981b), CitationSolomon et al. (1989), and CitationChow et al. (1994). CitationChow et al. (2012) discuss the assumptions and validity of this and several alternative methods to determine the soil component for mass reconstruction. The reconstructed soil-related portion of PM2.5 at the Clinton Drive site was the major contributor to the monitor having higher PM2.5 measurements. shows a comparison of the reconstructed soil factor using speciation sampling data from the summer of 2005 for five Houston-area sites with the summer of 2006 data at Clinton Drive. Funding for EPA's nominal speciation monitoring network was suspended in 2005, limiting the ability to perform coincident comparisons with the early Clinton speciation data. Using data from two time periods creates a risk that the difference between Clinton Drive and the other sites could be due to a temporal factor. However, the reconstructed soil results from are supported by coincident intercomparisons with total mass in is a map of monitoring sites for PM2.5 in 2006 used in these intercomparisons.
Figure 5. Mean reconstructed soil component at Clinton Drive, summer 2006, compared with other sites from summer 2005.
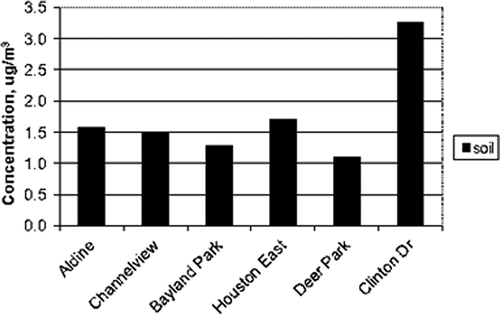
The fact that the reconstructed soil factor stood out as 1.6–2.1 mg/m3 higher than other sites (though separated by a year) suggested a local source of wind-blown dust. The Clinton Drive site is across the street to the north from a large unpaved area used by heavy-duty trucks to move goods to and from the port. Given that prevailing southerly winds would transport dust a short distance to the site, this configuration would appear to be the main issue. However, this configuration of a dust source in the prevailing upwind direction of a sampler had existed for several years, and this alone did not explain the increase in PM2.5 and PM10.
The DRI reconstruction formula in Equationeq 1 had been developed using data from 23 sites in the Texas monitoring network from 2001 to 2004. Because we suspected a local source that could be different from the wind-blown crustal material associated with the more general regional soil component, we chose to take a closer look at the elemental and ion constituents derived from the Teflon filters that were analyzed beginning in January 2005. Again, every-sixth-day and additional filters were analyzed. shows average percentage composition for days from 2005 to 2006 with Teflon filter analysis for 121 days with a predominant wind direction from the south, west, east, or north averaged together by direction. Fifteen days had been identified as North African dust days both by satellite imagery and high silicon and aluminum contents. A single day, December 31, 2006, was identified by date and composition as a day impacted by particulate matter from fireworks. The south and west quadrants have a higher sulfate-to-ammonium ratio than filters associated with the east and north quadrants. The ratio of average sulfate-to-ammonium mass by wind direction quadrant in is 2.83 for “east” and 2.71 for “north,” nearly exactly matching the ammonium sulfate stoichiometric ratio. In contrast, the ratio is 4.35 for “south” and 4.30 for “west,” showing excess sulfate to ammonium in the same quadrants with higher than expected calcium concentrations and indicating that sources with emissions of calcium and sulfate might play a role in the higher concentrations at the site.
Figure 7. Teflon filter 2005–2006 average composition—121 days with a predominant wind direction, 15 North African dust days, and 1 fireworks day, December 31, 2006.
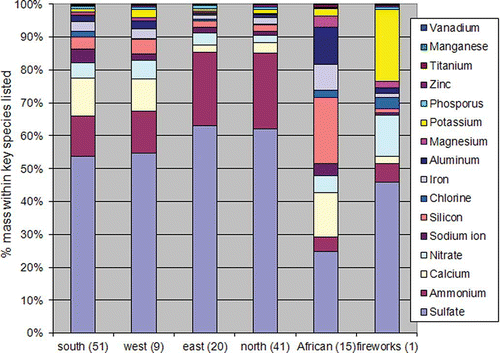
As more speciated data were collected, more complicated forms of source apportionment analysis were performed. A simple principal component analysis (PCA) using the initial 2005 data showed that six factors could be easily identified. These were an ammonium sulfate factor, a crustal material factor, a sea salt factor, an oil combustion factor, and a motor vehicle factor. Positive matrix factorization (PMF) analysis is a more powerful method with more data input requirements, and in 2006 enough data had been collected to employ it. PMF methods and applications to air pollution research (CitationPaatero and Tapper, 1994; CitationHopke, 2000; CitationKim et al., 2003; CitationReff et al., 2007) and additional results on this research are discussed in a previous conference paper (CitationSullivan et al., 2008). PMF is an optimization method that uses the observed multivariate concentration data (x) and a corresponding estimation of the uncertainty (u) in each data point to estimate a combination of source profiles and source contributions that explain each observation. EPA provides a downloadable version of PMF version 3.0, although an earlier version was used earlier in this project. In PMF, the matrix formed from the sample data is decomposed into a factor contribution matrix and a factor profile matrix, which then need to be interpreted as to what source types are present. A speciated data set can be viewed as a data matrix x with rows (samples) indexed as i and columns (chemical species) by j, in which the number of samples is n with m different chemical species. The goal of multivariate receptor modeling with PMF is to identify a number of factors p, the species profile f of each source, and the amount of mass g contributed by each factor to each individual sample. This is expressed in Equationeq 2:
Here eij is the residual for each sample/species between the observed value and the value estimated by the inner product of a row in g and a column in f. All estimates of f and g component are constrained to be non-negative. The uncertainty value uij associated with each xij observation can be an explicit one-to-one input variable or a set of values calculated by a model based on a method detection limit and stated relative error proportional to concentration. For this paper, an uncertainty file was constructed by the DRI laboratory based on the evaluation of sample blanks, duplicate samples, and equipment sensitivities so that an individual uncertainty was attached to every reported concentration. The PMF program minimizes the objective function Q ( Equationeq 3), based upon the error term e from Equationeq 1 and the uncertainties (u).
A value of Q is calculated via the optimization algorithm in PMF version 3.0 and a good solution is judged to have Q ≈ nm − p(n + m); however, a user is guided through several other quality assurance procedures to select a parsimonious and internally consistent solution that makes practical sense when compared with local emission sources and observed meteorological effects.
Using EPA's PMF version 1.1 (version available in 2006) with both Teflon and quartz filter chemical speciation samples from 2006 provided substantially more information about the nature of the sources contributing to the high PM2.5 concentrations in the area. The initial PMF analysis of 57 chemical speciation samples collected and analyzed from May 27, 2006, through December 2006 identified two factors containing soil-related elements: a crustal factor similar to Equationeq 1, and a calcium plus sulfate factor, which might have implicated local gypsum piles or land cover containing primarily calcium sulfate, a by-product from industrial production of hydrofluoric acid from fluorspar. Upon inquiry, we learned that this calcium sulfate waste product was being added to work yards used for storage and trans-shipment as a surface treatment in the hope of suppressing dust. There is only enough sulfate in this factor to account for 41% of the calcium in the factor as calcium sulfate. The additional calcium may have come from oyster shell and/or crushed concrete, both of which had been used as road and work area materials in the region. PMF analysis of 2005 data from a site 3 miles away did not identify a similar calcium plus sulfate factor (CitationHendler, 2006). and show the contribution of these factors to PM2.5 mass as a function of wind direction. The directionality of the calcium plus sulfate factor's contribution ruled out gypsum piles as a significant source of PM2.5 at the site.
Remediation efforts
Voluntary efforts to reduce PM2.5 in the area began in 2007 and included the following measures. The City of Houston installed traffic barriers to keep trucks on the roadway from driving on dirt shoulders on Clinton Drive, including the part of the road next to the property the PM2.5 monitor is on. The City also installed a light to facilitate traffic into the port and increased the density of vegetation along Clinton Drive. The TCEQ and Union Pacific Railroad reduced locomotive emissions in the area by 4.8 tons per year (tpy) using Texas Emission Reduction Program (TERP) funds to install upgrades on 13 of 52 new locomotive engines (genset units) in the Houston area. The Port Transit Rail Authority upgraded switcher engines and stopped steel loading on dirt areas under its control. The Port of Houston required all its work yard lessees to remove calcium sulfate treated soil, bring in fresh soil, periodically grade and compact it, and apply emulsified asphalt to the compacted soil to reduce dust emissions. EPA, the Port of Houston, and industry reduced port-related diesel emissions using $3.4 million in an EPA National Clean Diesel Campaign that provided funding through the Diesel Emission Reduction Act (DERA). DuPont implemented new dust control best management practice at its fluorspar unloading and storage facility. Valero Asphalt paved its large work yard that is just across Clinton Drive to the southeast of the monitor and normally upwind of Galena Park.
Follow-on data collection and data analysis
As data collection at the site has continued, a retrospective look back at concentration changes is possible. Using a more robust 6-yr data set of speciation data and PMF analysis coupled with two heuristic techniques, 12 sources of PM2.5 affecting Clinton Drive can be resolved. In examining the multiyear data set, two factors stood out without the use of statistical methods. (1) As was the case with the “fireworks” sample composition in , unusually high values of potassium on dates near July 4 and January 1 each year suggested that celebratory fireworks had been a major source of this species. (2) The seasonal behavior of crustal-material-related elements (e.g., silicon and aluminum) coupled with satellite images of dust clouds advected over the Atlantic Ocean, and the well-correlated region-wide changes in PM2.5 mass as the dust clouds came on shore, suggested North African dust as an obvious factor. Initial applications of multivariate statistical methods including PMF did not separate fireworks from fires or North African dust from local/regional wind-borne crustal material as factors. A heuristic to handle fireworks is straightforward: elevated potassium near holidays.
To differentiate the North African dust from more-local dust, a more complicated path was developed using all of the available PM2.5 speciation data from East Texas. As a first step, the time-series and statistical distribution of silicon data from Clinton Drive were examined. shows the time series for all elemental silicon collected at Clinton Drive from January 2005 through June 2010. The pattern for elevated concentrations appearing during each summer is clear. A highly skewed distribution for silicon concentrations is shown in two graphs in An examination of the log-Q-Q plot for the silicon data suggests that the 81% of data that fall below 0.6 μg/m3 have an approximate lognormal distribution, but above this concentration the graph has a highly skewed shape. This suggests two different populations are mixed.
The TCEQ provided a list of 70 dates from the summers of 2006–2009 of suspected North African dust (NAD) events based on satellite imagery interpretation and the characteristics of elevated PM2.5 levels across the state during the events. To this list we added two additional dates (based on elevated crustal components measured at several sites) to one multiday event. The subset of the 72 dates for which speciation data are available varied from 12 to 53 days at seven sites in the Dallas/Fort Worth, Houston, and Corpus Christi areas. All other dates were classified as “Not-NAD” days. Among the data collected in this project, the highest Pearson correlations among all elemental species at Clinton Drive were 0.973, 0.970, and 0.969 between titanium and aluminum, between silicon and aluminum, and between silicon and titanium, respectively. Silicon and aluminum are the two elements that stand out in relatively high concentration in the NAD events, and are the two principal constituents for soil in Equationeq 1. As has been shown, calcium, a soil constituent in Equationeq 1, has another local source, and as a result the correlation is relatively low (0.432) with silicon. Iron, the fourth constituent in Equationeq 1, has a high correlation with silicon, aluminum, and titanium (0.881, 0.889, 0.909), though lower than the earlier stated correlations between those three elements. This raised the hypothesis that iron may carry some unique information. The ratios of silicon to each of three other tracer elements for soil (aluminum, iron, and titanium) were calculated in both the NAD and “Not-NAD” data sets for all observations with silicon concentration greater than 0.0001 μg/m3. Time-series graphs were made of the resulting ratios, and the ratios for the NAD days appeared to be more tightly clustered about their mean ratio, which was reasonable given the higher signal-to-noise associated with the higher concentrations.
The simple statistics for mean, median, standard deviation, and percentiles by site were calculated for the two data sets. The results show close relationships between mean and median ratios for all species at all sites, close relationships among all the sites by data set, with similar mean and median ratios between the two data sets for aluminum and titanium ratios to silicon. However, consistent differences were found between the two data sets for the iron-to-silicon ratios. For the Not-NAD data set, the range of means for the iron-to-silicon ratios was 0.72–1.39, with an average across sites of 1.1. For the NAD data set, the range of means was 0.30–0.50, with an average across sites of 0.40. These results for the sample mean calculations are summarized in . A two-sided paired t test on differences between the NAD and Not-NAD shows the difference is statistically significant only for the iron-to-silicon ratio.
Table 1. Mean ratios of crustal elements to silicon from six PM2.5 speciation sites in three urban areas, North African dust versus other days, and paired t test for differences
The conclusion is that we propose a new heuristic method to isolate transported North African dust events based solely on speciation results and time of year: the ratio of iron to silicon in the range of 0.30–0.50 is a good indicator for transported North African dust given elevated silicon concentration and summertime period. Possible explanations for this result may be that iron may tend to be richer in larger particles that tend to deposit out of the finer particles that reach the United States, or it may simply be the case that the crustal material has a different composition in North Africa compared with Texas. Earlier we suggested that the statistical distributions of silicon data above and below 0.6 μg/m3 may be different. So as to limit the risk of misclassifying a nontransport candidate day as a transport day, we split the data into subsets based on summertime measurements with silicon concentrations above and below 0.8 μg/m3 and partitioned the data on iron-to-silicon ratio less than 0.5. The end result is a possible classification of 86 samples that are believed to have been strongly influenced by transported North African dust.
The complete 2005–2012 Clinton Drive speciated data set was filtered to remove eight “fireworks” dates, and 86 North African dust dates. The 2005 and early 2006 data for which no quartz filter/carbon data are available were removed. An additional set of data from late 2009 to early 2010 has been excluded over quality assurance concerns. As a final step, only data from every third day from the national sampling schedule were used to reduce the bias created by having selectively analyzed many non-every-sixth-day filters. Using every third day then balances the reduced number of “random” samples on every sixth day, with some additional samples potentially biased high in concentration. The resulting data set contained 579 observations. The PMF analysis used 23 species, adding 5% added uncertainty to account for potential reactivity in the air, and numerous iterations suggested 10 factors, shown in . The results were very similar to those found earlier and describe in CitationSullivan et al. (2008), but the robustness of the newer results are superior. The robustness of the result was tested with the bootstrapping application in PMF version 3.0, which had 100% success in matching all 10 factors on each of 100 runs using randomly selected subset of 36 samples and based on a 0.6 correlation in matching.
Table 2. Ten factors derived with PMF version 3.0 using Clinton Drive data
shows the final results of apportioning the mass at Clinton Drive to 10 sources using PMF. The North African dust and fireworks sources are not included.
Table 3. Ten factors’ contribution to total mass at Clinton Drive, 2005–2012
The PMF factor results were sequestered into two sets, one from 2006 to 2008, and the second from 2009 to first quarter 2012. The results were merged with the hourly wind data at Clinton Drive. The results for the calcium sulfate factor and the soil factor for the two periods appear in and Under south-southwest winds, the calcium sulfate factor contributes 2.5 μg/m3 less and the soil factor contributes 0.8 μg/m3 less mass from the key upwind direction in recent years compared with earlier years.
Several of these factors have been declining in addition to soil and calcium sulfate. shows DRI reconstructions for seven factors, showing that soil (which overlaps calcium sulfate in the calcium component) declined by more than 2 μg/m3 from 2006 to 2011. Ammonium sulfate also shows a significant decline. One way to assess regional versus local changes is to look across other monitoring sites. shows a graph like for the Aldine monitoring site located 12 miles north-northwest of Clinton Drive. There, ammonium sulfate declined in lock-step with the concentrations at Clinton by approximately 0.8 μg/m3 from 2007 to 2008. and are offered as a simple way to compare across monitors using the same DRI reconstruction formulas on speciation data without doing a local multivariate source apportionment analysis.
Summary
When 2005 PM2.5 data became available in April 2006 and the 3-yr average PM2.5 concentration just to the north of the Houston Ship Channel reached 15.0 µg/m3, the TCEQ initiated daily collection of quartz fiber as well as Teflon PM2.5 filter samples for chemical speciation analysis. Data analysis methods included positive matrix factorization (PMF), wind direction analysis for total mass, for species, and for PMF factors. The PMF analyses identified two mineral factors as the principal difference between this site (Clinton Drive) and other sites in the area. One was composed of typical soil elements. The other consisted of calcium sulfate, which was a by-product of an industrial process, plus additional calcium, which may have come from oyster shell or crushed concrete or both. Voluntary efforts by local businesses, the Port of Houston Authority, and city and county governments took hold and reduced PM2.5 concentrations significantly by the end of 2007. The paper describes these voluntary efforts and the resulting changes in concentration of the species making up PM2.5 impacting the Clinton Drive site and the Galena Park neighborhood north of the Houston Ship Channel area. The 2005–2007 PM2.5 annual averages were 15.9, 16.0, and 15.6 µg/m3, respectively. The 2008–2011 averages were 14.0, 12.6, 12.3, and 12.3 µg/m3. In 2006, the PM2.5 average at Clinton Drive was 2.6 μg/m3 higher than a similar site 3 miles away; by 2009 the difference was reduced to 0.5 µg/m3.
References
- Bahreini , R. , Ervens , B. , Middlebrook , A. M. , Warneke , C. , de Gouw , J. A. , DeCarlo , P. F. , Jimenez , J. L. , Brock , C. A. , Neuman , J. A. , Ryerson , T. B. , Stark , H. Atlas , E. 2009 . Organic aerosol formation in urban and industrial plumes near Houston and Dallas, Texas . J. Geophys. Res. , 114 : D00F16 doi: 10.1029/2008JD011493
- Bates , T.S. , Quinn , P. K. , Coffman , D. , Schulz , K. , Covert , D. S. , Johnson , J. E. , Williams , E. J. , Lerner , B. M. , Angevine , W. M. , Tucker , S. C. , Brewer , W. A. and Stohl , A. 2008 . Boundary layer aerosol chemistry during TexAQS/GoMACCS 2006: Insights into aerosol sources and transformation processes . J. Geophys. Res. , 113 : D00F01 doi: 10.1029/2008JD010023
- Chen , Y. , Zheng , M. , Edgerton , E.S. , Ke , L. , Sheng , G. and Fu , J. 2012 . PM2.5 source apportionment in the southeastern U.S.: Spatial and seasonal variations during 2001–2005 . J. Geophys. Res. , 117 : D08304 doi: 10.1029/2011JD016572
- Chow , J.C. , Watson , J.G. , Lowenthal , D.H. , Chen , L.-W.A. and Wang , X. 2012 . “ Reformulation of PM2.5 mass reconstruction assumptions for the San Joaquin Valley ” . In Desert Research Institute final report prepared for San Joaquin Valley Air Pollution Study Agency, June 28, 2012
- Chow , J.C. , Watson , J.G. , Lowenthal , D.H. , Solomon , P.A. , Magliano , K.L. , Ziman , S.D. and Richards , L.W. 1994 . “ PM10 and PM2.5 chemical characteristics and source apportionment in the San Joaquin Valley ” . In Planning and Managing Regional Air Quality, Modeling and Measurement Studies , Edited by: Solomon , P.A. 687 – 698 . Boca Raton , FL : CRC Press .
- Dockery , D.W. 2001 . Epidemiologic evidence of cardiovascular effects of particulate air pollution . Environ. Health Perspect. , 109 ( 4 ) : 483 – 486 . doi: 10.1289/ehp.01109s4483
- Fraser , M.P. , Yue , Z.W. and Buzcu , B. 2003 . Source apportionment of fine particulate matter in Houston, TX, using organic molecular markers . Atmos. Environ. , 37 : 2117 – 2123 . doi: 10.1016/S1352-2310(03)00075-X
- Fraser , M.P. , Yue , Z.W. , Tropp , R.J. , Kohl , S.D. and Chow , J.C. 2002 . Molecular composition of organic fine particulate matter in Houston, TX . Atmos. Environ. , 36 : 5751 – 5758 . doi: 10.1016/S1352-2310(02)00725-2
- Hendler , A. 2006 . “ URS Corp ” . In personal communication based on work performed for the Houston Regional Monitoring Network, an industry-funded voluntary association
- Hopke, P.K. 2000. A guide to positive matrix factorization. EPA workshop proceedings. Materials from the Workshop on UNMIX and PMF as Applied to PM2.5, Research Triangle Park, February 14–16, 2000 http://www.epa.gov/ttnamti1/files/ambient/pm25/workshop/laymen.pdf (http://www.epa.gov/ttnamti1/files/ambient/pm25/workshop/laymen.pdf)
- Kim , E. , Larson , T.V , Hopke , P.K. , Slaughter , C. , Sheppard , L.E. and Claiborn , C. 2003 . Source identification of PM2.5 in an arid northwest U.S. city by positive matrix factorization . Atmos. Res. , 66 : 291 – 305 . doi: 10.1016/S0169-8095(03)00025-5
- Laden , F. , Neas , L.M. , Dockery , D.W. and Schwartz , J. 2000 . Association of fine particulate matter from difference sources with daily mortality in six U.S. cities . Environ. Health Perspect. , 108 : 941 – 947 . doi: 10.1289/ehp.00108941
- Macias , E.S. , Zwicker , J.O. , Ouimette , J.R. , Hering , S.V. , Friedlander , S.K. , Cahill , T.A. , Kuhlmey , G.A. and Richards , L.W. 1981a . Regional haze case studies in the southwestern United States—I. Aerosol chemical composition . Atmos. Environ. , 15 : 1971 – 1986 . doi: 10.1016/0004-6981(81)90231-6
- Macias , E.S. , Zwicker , J.O. and White , W.H. 1981b . Regional haze case studies in the southwestern United States—II. Source contributions . Atmos. Environ. , 15 : 1987 – 1997 . doi: 10.1016/0004-6981(81)90232-8
- Paatero , P. and Tapper , U. 1994 . Positive matrix factorization: A non-negative factor model with optimal utilization of error estimates of data values . Environmetrics , 5 : 111 – 126 . doi: 10.1002/env.3170050203
- Parrish , D. D. , Allen , D. T. , Bates , T. S. , Estes , M. , Fehsenfeld , F. C. , Feingold , G. , Ferrare , R. , Hardesty , R. M. , Meagher , J. F. , Nielsen-Gammon , J. W. Pierce , R. B. 2009 . Overview of the Second Texas Air Quality Study (TexAQS II) and the Gulf of Mexico Atmospheric Composition and Climate Study (GoMACCS) . J. Geophys. Res. , 114 : D00F13 doi: 10.1029/2009JD011842
- Pope , C.A. III and Dockery , D.W. 2006 . Health effects of fine particulate air pollution: Lines that connect . J. Air Waste Manage. Assoc. , 56 : 709 – 742 . doi: 10.1080/10473289.2006.10464485
- Reff , A. , Eberly , S.I. and Bhave , P.V. 2007 . Receptor modeling of ambient particulate matter data using positive matrix factorization: Review of existing methods . J. Air Waste Manage. Assoc. , 57 : 146 – 154 . doi: 10.1080/10473289.2007.10465319
- Russell , M. M. , Allen , D.T. , Collins , D.R and Fraser , M.P. 2004 . Daily, seasonal and spatial trends in fine particulate matter mass and composition in Southeast Texas . Aerosol Sci. Technol. , 38 : 14 – 26 . doi: 10.1080/02786820390229318
- Solomon , P.A. , Fall , T. , Salmon , L.G. , Cass , G.R. , Gray , H.A. and Davidson , A. 1989 . Chemical characteristics of PM10 aerosols collected in the Los Angeles area . J. Air Pollut. Control Assoc. , 39 : 154 – 163 . doi: 10.1080/08940630.1989.10466515
- Sullivan , D.W. , Tropp , R.J. and Price , J.H. 2008 . Source apportionment for particulate matter at the Houston Clinton Drive monitoring site . Proceedings of the Air and Waste Management Association Annual Conference and Exhibition, Portland, OR, June 24–27, 2008, Extended Abstract 710. ,
- Tropp , R.J. , Chow , J.C. , Watson , J.G. , Kohl , S.D. , Countess , R.J. and Countess , S. 2006 . PM2.5 chemical speciation results of Texas—2001–2004 . Proceedings of the Air & Waste Management Association 99th Annual Conference and Exhibition, New Orleans, LA, June 20–23 2006, Paper 519. ,
- Tropp , R. 2009 . Assessment of data quality indicators for PM2.5 chemical speciation compounds using data from Texas . Proceedings of the Air & Waste Management Association 102th Annual Conference and Exhibition, Detroit, MI, June 16–19 June 2009 , 2009-A-700-AWMA.
- Watson , J.G. , Xhu , T. , Chow , J.C. , Engelbrecht , J. , Fujita , E.M. and Wilson , W.E. 2002 . Receptor modeling application framework for particle source apportionment . Chemosphere , 49 : 1093 – 1136 . doi: 10.1016/S0045-6535(02)00243-6
- U.S. Environmental Protection Agency . 1997 . National Ambient Air Quality Standards for Particulate Matter; Final Rule . Fed. Regist. , 62 : 38652 – 38760 .