Abstract
Improvement of air quality models is required so that they can be utilized to design effective control strategies for fine particulate matter (PM2.5). The Community Multiscale Air Quality modeling system was applied to the Greater Tokyo Area of Japan in winter 2010 and summer 2011. The model results were compared with observed concentrations of PM2.5 sulfate (SO42-), nitrate (NO3−) and ammonium, and gaseous nitric acid (HNO3) and ammonia (NH3). The model approximately reproduced PM2.5 SO42− concentration, but clearly overestimated PM2.5 NO3− concentration, which was attributed to overestimation of production of ammonium nitrate (NH4NO3). This study conducted sensitivity analyses of factors associated with the model performance for PM2.5 NO3− concentration, including temperature and relative humidity, emission of nitrogen oxides, seasonal variation of NH3 emission, HNO3 and NH3 dry deposition velocities, and heterogeneous reaction probability of dinitrogen pentoxide. Change in NH3 emission directly affected NH3 concentration, and substantially affected NH4NO3 concentration. Higher dry deposition velocities of HNO3 and NH3 led to substantial reductions of concentrations of the gaseous species and NH4NO3. Because uncertainties in NH3 emission and dry deposition processes are probably large, these processes may be key factors for improvement of the model performance for PM2.5 NO3−.
Implications:
The Community Multiscale Air Quality modeling system clearly overestimated the concentration of fine particulate nitrate in the Greater Tokyo Area of Japan, which was attributed to overestimation of production of ammonium nitrate. Sensitivity analyses were conducted for factors associated with the model performance for nitrate. Ammonia emission and dry deposition of nitric acid and ammonia may be key factors for improvement of the model performance.
Introduction
Particulate matter (PM) with aerodynamic diameter less than 2.5 μm (PM2.5) is an atmospheric pollutant that mainly consists of several major components, such as sulfate (SO42−), nitrate (NO3−), ammonium (NH4+), elemental carbon (EC), and organic aerosol (OA). PM2.5 has been of increasing concern because of its adverse effects on human health (e.g., Ueda et al., Citation2009; Nawahda et al., Citation2012). The Ministry of the Environment of Japan (MOE) introduced an air quality standard for PM2.5 concentration (35 μg m−3 for daily mean and 15 μg m−3 for annual mean) in 2009. Although PM2.5 concentrations have decreased in recent years in Japan, the standard for PM2.5 is not attained in most urban areas (MOE, Citation2012). Among the major components of PM2.5, while EC, which is emitted directly through combustions of fossil fuel and biomass (Bond et al., Citation2004), exhibits a decreasing trend, SO42−, NO3−, and NH4+, which are mainly produced from gaseous precursors via complex chemical processes in the atmosphere, do not exhibit clear decreasing trends (Minoura et al., Citation2006). To design effective PM2.5 control strategies, it is essential to use air quality models (AQMs) that represent detailed physical and chemical processes in the atmosphere. However, current AQMs cannot adequately simulate mass concentrations of PM2.5 and its major components in the Greater Tokyo Area, which includes one of the largest megacities in the world (Morino et al., Citation2010).
The urban air quality model intercomparison study in Japan (UMICS) has been conducted in order to improve AQM performance. In UMICS, the major components of PM2.5 in the Greater Tokyo Area are focused; common data sets, including meteorological, emission, and boundary data, are provided to participating models; and participants conduct sensitivity runs in their fields of expertise. UMICS consist of three phases, with the first phase focusing on EC, the second phase focusing on SO42−, NO3−, and NH4+, and the third phase focusing on OA (UMICS1, UMICS2, and UMICS3). In UMICS1, all the participating models underestimated PM2.5 EC concentrations in the Greater Tokyo Area in summer 2007. Process analyses revealed that emission and vertical diffusion were dominant factors that affect the simulated EC concentrations; it was also shown that improvement of local EC emissions could considerably contribute to improvement of the model performance (Chatani et al., Citation2014).
This paper describes results of UMICS2, which focuses on the major ionic component of PM2.5 in winter 2010 and summer 2011. All the participating models are the Community Multiscale Air Quality modeling system (CMAQ) (Byun and Schere, Citation2006) with different configurations. Because large discrepancies occurred between observed and simulated PM2.5 NO3− concentrations as described in a later section, sensitivity analyses were conducted in order to investigate factors influencing AQM performance for PM2.5 NO3− simulation.
The following processes are closely associated with PM2.5 NO3− concentration. Most of nitrogen oxides (NOX) is emitted as nitrogen monoxide (NO), which is rapidly oxidized to nitrogen dioxide (NO2) in the atmosphere. During daytime, nitric acid (HNO3) is formed by the reaction of NO2 and hydroxyl radical (OH), which is the predominant chemical removal path for NOX. During nighttime, nitrogen trioxide (NO3) is formed by the reaction of NO2 and ozone (O3), and reacts with NO2 to form dinitrogen pentoxide (N2O5). Heterogeneous reactions of N2O5 have a substantial effect on nocturnal HNO3 production (Dentener and Crutzen, Citation1993; Riemer et al., Citation2003). Gaseous HNO3 and ammonia (NH3), and particulate ammonium nitrate (NH4NO3) form a thermodynamic equilibrium that depends on temperature and relative humidity (Kim et al., Citation1993). Additionally, dry deposition is an important removal path for HNO3 and NH3 because they have relatively large dry deposition velocities among various gaseous species (Zhang et al., Citation2003). Sensitivity analyses conducted in this study are related to these processes.
Methodology
Modeling domains
shows common modeling domains prepared for UMICS2. Topography height and land use were obtained from the U.S. Geological Survey data (30-sec resolution). The common domains consist of three domains, from domain 1 (D1), covering a wide area of East Asia, to domain 3 (D3), covering the Greater Tokyo Area. The horizontal resolutions and the numbers of grid cells are 64, 16, and 4 km, and 96 × 80, 56 × 56, and 56 × 56 for D1, domain 2 (D2), and D3, respectively. The vertical layers consist of 30 sigma-pressure coordinated layers from the surface to 100 hPa, with the middle height of the first layer being approximately 28 m. For comparisons between model results, the target area is defined as land areas with elevations <200 m in D3. The target area includes the largest urban and industrial areas in Japan, and therefore is characterized by huge population and air pollutant emissions.
Meteorological fields
Common meteorological fields for UMICS2 were produced using the Weather Research and Forecasting model (WRF) (Skamarock and Klemp, Citation2008), version 3.2.1, for periods from November 15 to December 5, 2010, and July 11 to 31, 2011. Sea surface temperature was derived from the high-resolution, real-time, global sea surface temperature analysis data developed at the U.S. National Centers for Environmental Prediction (NCEP)/Marine Modeling and Analysis Branch. Initial and boundary conditions were derived from the final analysis data of NCEP. WRF was configured with the Kain–Fritsch cumulus parameterization (Kain, Citation2004) in D1 and D2, the Asymmetrical Convective Model version 2 (ACM2) (Pleim, Citation2007) for the planetary boundary layer parameterization, the WRF single-moment 5-class microphysics scheme (Hong et al., Citation2004), the Pleim–Xiu land surface model (Xiu and Pleim, Citation2001), and the rapid radiative transfer model (Mlawer et al., Citation1997) for the longwave radiation and the scheme of Dudhia (Citation1989) for the shortwave radiation processes. Grid nudging was applied to wind, temperature, and humidity in D1 and D2 with nudging coefficient of 1.0 × 10−4 sec−1 for the entire periods.
The common meteorological fields were compared with observations by the Japan Meteorological Agency (JMA) at meteorological observatories in D3 for periods from November 22 to December 5, 2010, and July 18 to 31, 2011. summarizes statistical comparisons between the observations by JMA and the common meteorological fields, and shows time series comparisons of hourly meteorological variables averaged for all the meteorological observatories. These comparisons showed that the common meteorological fields generally agreed well with the observations. The WRF performance evaluated by statistical measures was comparable to the performances of meteorological models in other literatures (Shimadera et al., Citation2009, Citation2013). In winter 2010, precipitation events were due to passages of low-pressure systems on November 23 and 25, and a cold front on December 3, and clear sky conditions prevailed during the other periods. In summer 2011, the typhoon 1106 MA-ON passed south of the main island of Japan during July 19 to 21, and then cloudy and wet conditions prevailed in the Greater Tokyo Area.
Table 1. Statistical comparisons between observations by JMA and common meteorological fields produced by WRF for UMICS2 at the meteorological observatories in D3
Emission data
Common emission data for UMICS2 were produced according to the method described by Chatani et al. (Citation2011). Anthropogenic emissions in D1 were derived from the emission inventory for Asia in the year 2006 estimated by Zhang et al. (Citation2009) for sulfur dioxide (SO2), NOX, carbon monoxide (CO), PM and volatile organic compounds (VOC), and the regional emission inventory in Asia (Ohara et al., Citation2007) for NH3. Anthropogenic emissions in D2 and D3 were estimated with the Japan Auto-Oil Program (JATOP) vehicle emission estimate model and the Georeference-Based Emission Activity Modeling System (Nansai et al., Citation2004). Ship emissions were derived from emission inventories developed by the National Maritime Research Institute (NMRI) and the Ocean Policy Research Foundation (OPRF). Biogenic VOC emissions were estimated by using the Model of Emissions of Gases and Aerosols from Nature (Guenther et al., Citation2006), version 2.04, with the common meteorological fields.
shows horizontal distributions of mean SO2, NOX, and NH3 emission rates in summer 2011 in D3. Major emission sources of SO2 are large point sources, industrial areas along the Tokyo Bay, and ships. Major sources of NOX are vehicles, in addition to those of SO2. Major sources of NH3 are agricultural sources, particularly volatilization from livestock wastes and fertilized fields. The difference in the major sources explains the difference in the distribution patterns. The total SO2, NOX, and NH3 emissions in D3 were 107, 408, and 110 mol sec−1 in the winter period and 110, 371, and 334 mol sec−1 in the summer period, respectively.
Observation data
Observations were conducted using denuder/filter-pack systems at Komae, Kisai, and Maebashi () in winter 2010 and summer 2011 (Hasegawa et al., Citation2012). Concentrations of PM2.5 SO42−, NO3−, and NH4+, and gaseous HNO3 and NH3 were quantified by analyzing 4-hr samples with ion chromatography. From November 25 to December 2, 2010, observation data available for model evaluation are PM2.5 SO42− concentrations at the three sites, concentrations of PM2.5 NO3−, and NH4+, and gaseous HNO3 and NH3 at Kisai. From July 24 to 29, 2011, concentrations of the five chemical species at the three sites are available.
Participating models and boundary conditions
summarizes configurations of models participating in UMICS2. All the five participating models (M0 to M4) are CMAQ with different configurations. M0 is the baseline simulation case of UMICS2, and is compared with the observation data in the next section. The Statewide Air Pollution Research Center version 99 (Carter, Citation2000) was used with the Euler Backward Iterative (EBI) solver (Hertel et al., Citation1993) or the Rosenbrock (ROS3) solver (Sandu et al., Citation1997) for the gas-phase chemistry. The fourth- or fifth-generation CMAQ aerosol module (AERO4 or AERO5) was used for the aerosol process. The thermodynamic equilibrium module used in the aerosol process was ISORROPIA (Nenes et al., Citation1998). The aqueous chemistry and wet deposition process were based on the regional acid deposition model (RADM) (Chang et al., Citation1987) except that M0, M2, M3, and M4 used ACM (Pleim and Chang, Citation1992) for the subgrid cloud process. All the models used ACM2 for the vertical diffusion and the Models-3 routine (Pleim et al., Citation2001) for the dry deposition process.
Table 2. Configurations of models participating in UMICS2
Common initial and boundary concentrations for D1 were obtained from the Model for Ozone and Related Chemical Tracers, version 4 (Emmons et al., Citation2010). M0 was driven by the common data sets in order to produce common boundary concentrations for the inner domains, which were used for M1, M2, M5, and sensitivity runs. CMAQ runs were conducted for periods from November 15 to December 5, 2010, and July 11 to 31, 2011. The first 7 days of each period are initial spin-up periods, and the next 14 days of each period are the target periods for comparisons between model results.
Model Evaluation
shows time series of the observed and M0-simulated hourly concentrations of O3, NO, NO2, and PM2.5 at Kisai the target periods of UMICS2 in winter 2010 and summer 2011. The M0-simulated O3 and NO2 concentrations approximately agreed with the observations in the two periods. The concentration level of O3 was not quite as high in the summer because of the typhoon and prevailing cloudy conditions. M0 approximately captured the daily variation pattern of PM2.5 in the winter, but failed to capture the diurnal variation. M0 also failed to capture the temporal variation of PM2.5. The discrepancies in PM2.5 are related to the model performance for PM2.5 components described in the following.
Figure 4. Time series of observed and M0-simulated hourly concentrations of O3, NO, NO2, and PM2.5 at Kisai in winter 2010 (left panels) and summer 2011 (right panels).
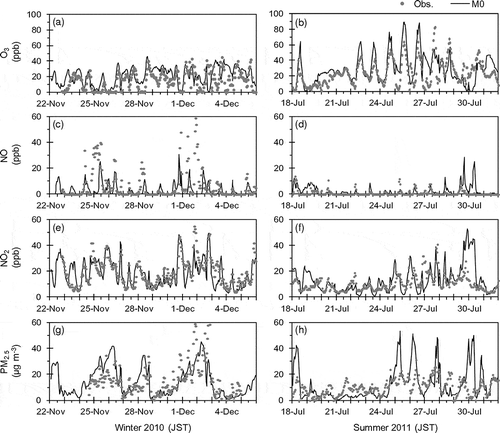
summarizes comparisons between the observed and M0-simulated concentrations of PM2.5 SO42−, NO3−, and NH4+, and gaseous HNO3 and NH3 in winter 2010 and summer 2011. shows time series of the observed and M0-simulated 4-hr concentrations in summer 2011. The M0-simulated mean concentrations of PM2.5 SO42− approximately agreed with the observations. In addition, M0 generally captured the temporal variation patterns of PM2.5 SO42− in both winter 2010 and summer 2011 except on July 28–29 at Komae. During the nighttime on July 28, although the wind was weak in both the observation and the common meteorological field, the prevailing wind directions were the east in the observation and the west in the common meteorological field ( and ). Therefore, the observed concentrations were likely to be affected by large emissions along the Tokyo Bay (). The observed PM2.5 NO3− and NH4+ concentrations in the period at Komae were also much higher than the M0-simulated values, as well as SO42−. The discrepancies seem to be attributed to the common meteorological field rather than to CMAQ itself.
Table 3. Comparisons of observed and M0-simulated concentrations of PM2.5 SO42−, NO3−, and NH4+, and gaseous HNO3 and NH3
Figure 5. Time series of observed and M0-simulated 4-hr concentrations of PM2.5 SO42−, NO3−, and NH4+, and gaseous HNO3 and NH3 at Komae (left panels), Kisai (middle panels), and Maebashi (right panels) in summer 2011.
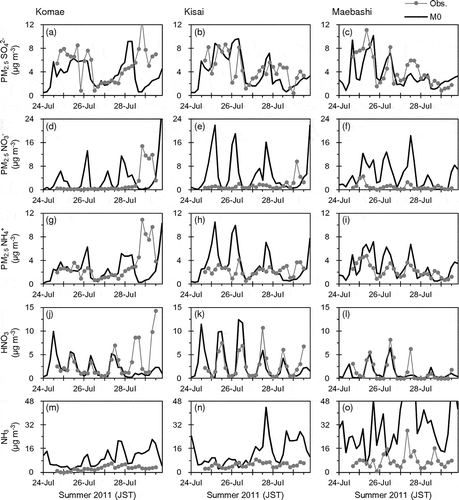
While M0 simulated well the diurnal variation pattern (higher during daytime and lower during nighttime) of HNO3 concentration, it clearly overestimated PM2.5 NO3−. The substantial overestimations of PM2.5 NO3− and overestimations of PM2.5 NH4+ occurred simultaneously. Therefore, the overestimations are likely attributed to overestimation of particulate NH4NO3 concentration. Although the descending order of the mean NH3 concentrations at the observation sites was the same between the observation and M0 (Maebashi > Kisai > Komae), the model clearly overestimated the concentration level. The overestimation of NH3 may have a large effect on the overestimation of PM2.5 NO3−. The model performance for NH3 concentration depends strongly on the accuracy of emission data. However, there are large uncertainties in estimates of the total amount and temporal variations of NH3 emissions because of difficulties in measuring the emission rates from various sources (Pinder et al., Citation2006). In addition, the absence of long-term and widespread monitoring network for NH3 concentration has made it difficult to evaluate NH3 emission inventories in Japan.
Because the performance of M0 for PM2.5 NO3− was much inferior to that for PM2.5 SO42−, sensitivity analyses conducted in the next section focused on the processes associated with PM2.5 NO3− concentration.
Sensitivity Analyses
Integrated process rate analysis
An analysis tool for integrated process rate (IPR) is integrated in CMAQ. The IPR analysis provides quantitative information on the contributions of various individual physical and chemical processes, including advection, diffusion, primary emission, dry deposition, aqueous process, gas-phase chemistry, and aerosol process, to the changes in the simulated concentrations of a pollutant. The IPR analysis was conducted by M0.
shows results of the IPR analysis for ground-level concentrations of gaseous HNO3 and PM2.5 NO3− in the target area during the target periods. The positive contribution of gas-phase chemistry to HNO3 concentration during daytime indicates the production through the photochemical oxidations of NOX. The large negative contribution of dry deposition to HNO3 indicates that the process is the dominant removal path for HNO3 in the atmosphere. The negative (positive) contribution of aerosol process to PM2.5 NO3− (HNO3) during daytime and the positive (negative) contribution during nighttime are mainly due to the gas–aerosol equilibrium of NH4NO3. Low temperature and high relative humidity during nighttime increase particulate NH4NO3 concentration and decrease gaseous HNO3 and NH3 concentrations. The accuracy of the thermodynamic module may have a large effect on AQM performance for PM2.5 NO3−, as well as temperature and humidity. In addition, heterogeneous reactions of N2O5 also contributed to the positive value of aerosol process during nighttime.
Figure 6. Mean diurnal variations of M0-simulated contributions of various individual physical and chemical processes to changes of ground-level HNO3 and PM2.5 NO3− concentrations in the target area during the target periods of UMICS2. The processes include advection (ADV), diffusion (DIF), primary emission (EMIS), dry deposition (DDEP), aqueous process (CLDS), gas-phase chemistry (CHEM), and aerosol process (AERO).
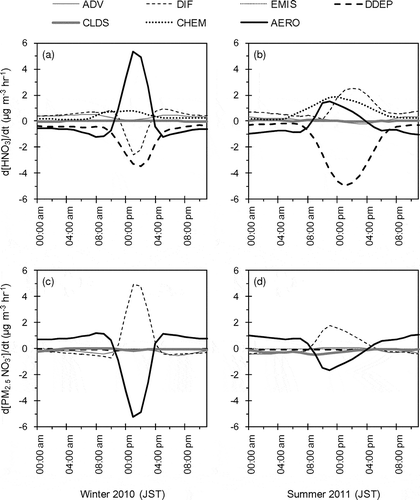
Different CMAQ configurations
The results of the five participating models were compared. Because common input data sets were used, temporal variation patterns of simulated concentrations were quite similar to each other (not shown). In order to emphasize the difference, the results were compared by percentage differences between mean ground-level concentrations simulated by M1 to M4 and those by M0 in the target area during the target periods of UMICS2. The results of sensitivity analyses are also shown in the same way in later chapters.
shows comparisons of the results of M1 to M4 by percentage differences of mean concentrations from M0. Although the differences between the results of M1 and M3, which are CMAQ version 4.7.1 (the same version as M0), and those of M0 are relatively small, they indicate effects of the in-line photolysis rate calculation module. The in-line module uses CMAQ-predicted O3, NO2 and PM concentrations to adjust photolysis rates, rather than relying on a look-up table of static background concentrations (Binkowski et al., Citation2007). Because of large emissions in the Greater Tokyo Area, pollutant concentrations in the area are generally higher than those in background areas. Therefore, photolysis rates calculated in the in-line module in the Greater Tokyo Area were smaller than those of the look-up table, which resulted in smaller productions of HNO3 through the photochemical reactions and PM2.5 NO3− through the subsequent process in M1 and M3. The results of M2 show relatively large differences compared to the others because of various different configurations, such as the version of CMAQ, the gas-phase chemistry solver, and the aerosol and aqueous modules. M4 is CMAQ version 5.0 in which minimum eddy diffusivity reduced from 2.0 to 1.0 m−2 sec−1 in urban areas and from 0.5 to 0.01 m−2 sec−1 in other areas. Consequently, M4 predicted higher ground-level concentrations of species that were strongly affected by ground-level emissions, such as NO2 and NH3, under stable conditions during nighttime.
Temperature and relative humidity
Sensitivity analyses described hereafter were performed using a brute-force method. Sensitivity analyses of temperature and relative humidity, which affect the gas-aerosol equilibrium of NH4NO3, were conducted in D3 by M0. In sensitivity runs, temperature or relative humidity used in aerosol module was uniformly changed by ±2 K (T + 2, T – 2) or by ±10% (RH + 10, RH – 10). Errors of such magnitudes can sometimes occur in meteorological simulations (–). Note that relative humidity used in aerosol module remains within the range of 0.5 to 99%, and changes in temperature and relative humidity also affect other processes, such as heterogeneous reactions of N2O5.
shows results of the sensitivity analyses of temperature and relative humidity. In higher temperature and lower relative humidity, particulate NH4NO3 easily shifts to gaseous HNO3 and NH3. The results indicate even errors of temperature and humidity that sometimes occur can cause over- or underestimation of PM2.5 NO3−. Prediction errors in temperature may have a larger effect than the result of the sensitivity analysis because they lead to prediction errors in relative humidity.
NOX emission
Sensitivity analysis of NOX emission was conducted in D3 by M1. In sensitivity runs, NOX emission was uniformly changed by from −40% to +40% (E – 40, E – 20, E + 20, E + 40). Because the total NOX emissions in Japan in the year 2000 estimated by different researchers are similar (2198 Gg by Streets et al., Citation2003; 1970 Gg by Ohara et al., Citation2007; 2408 Gg by Kannari et al., Citation2007), uncertainty in the total NOX emission is probably smaller than 40%.
shows results of the sensitivity analysis of NOX emission. The sensitivity to NOX emission was higher in summer because of accelerated photochemical reactions. Although the sensitivity of NO2 concentration to NOX emission was high, the sensitivity of PM2.5 NO3− concentration was relatively low, particularly in E + 20 and E + 40 in the winter. In the incremental runs, the increase in consumption of oxidants by the conversion of NO to NO2 led to the lack of oxidants available for further oxidations to produce HNO3. Because uncertainty in NOX emission estimate may be small and PM2.5 NO3− tended to be insensitive to NOX emission, improvement of NOX emission is less likely to have a high priority for improvement of AQM performance for PM2.5 NO3−.
Seasonal variation of NH3 emission
Sensitivity analysis of NH3 emission was conducted in D2 to D3 by M0. The common NH3 emission data was estimated using emission factors in European Environment Agency (Citation2009), in which emissions form livestock solid wastes and from manure applications are treated together. For sensitivity runs, a seasonal variation of NH3 emission was estimated according to processes for nitrous oxide (N2O) emission estimation in Japan (National Institute for Environmental Studies, Citation2012), in which emissions from livestock solid wastes and from manure applications are treated separately. shows the seasonal variations of NH3 emissions from livestock solid wastes and manure applications. Using the modified seasonal variation for sensitivity runs, the total NH3 emission in D3 increased by 52% in the winter and decreased by 42% in the summer.
Figure 10. Seasonal variations of NH3 emissions from livestock solid wastes and agricultural fields fertilized by manures used in common emission data and sensitivity runs.
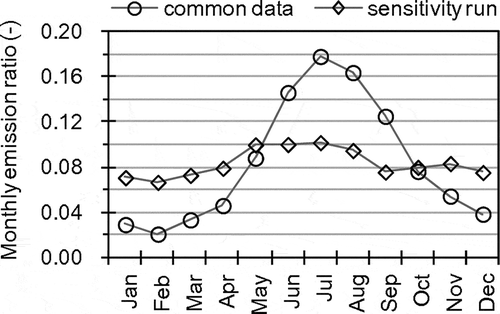
shows results of the sensitivity analysis of seasonal variation of NH3 emission. The change in NH3 emission directly affected NH3 concentration, and substantially affected the formation of NH4NO3. In Japan, observation data of NH3 concentration are not sufficiently available for evaluation of AQM performance. Therefore, uncertainty in NH3 emission estimate is still probably large and improvement of NH3 emission may be one of the key factors for improvement of AQM performance for PM2.5 NO3−.
HNO3 and NH3 dry deposition velocities
Sensitivity analysis of HNO3 and NH3 dry deposition, which is an important removal path for them, was conducted in D3 by M1. During daytime in summer 2011, M1-simulated HNO3 and NH3 dry deposition velocities averaged in the target area generally ranged from 1 to 5 cm sec−1, and mean values were 2.3 cm sec−1 for HNO3 and 1.9 cm sec−1 for NH3. Meanwhile, Neuman et al. (Citation2004) estimated much higher HNO3 dry deposition velocities in daytime in the summer (8 to 26 cm sec−1). In sensitivity runs, HNO3 and NH3 dry deposition velocities were uniformly multiplied by 5 (Vd5), 2 (Vd2), 0.5 (Vd0.5), and 0.2 (Vd0.2). Note that the multiplications of the dry deposition velocities have no theoretical support.
shows percentage differences between M1 and the sensitivity runs for mean concentrations in the target area during the target periods of UMICS2. also shows the percentage differences but plotted against the ratio of HNO3 and NH3 dry deposition velocities in the sensitivity runs to those in M1. A change in concentration at the first layer caused by a change in dry deposition is mitigated by other processes, particularly interactions with upper layers through vertical diffusion. While mean contributions, which were estimated by the IPR analysis, of dry deposition to HNO3 concentration were respectively −0.6, −2.5, and −6.9 μg m−3 hr−1 in Vd0.2, M1, and Vd5 in the target area during daytime in the summer, those of diffusion process were respectively −0.5, +1.2, and +5.1 μg m−3 hr−1. As a result, the changes in concentrations did not linearly respond to the changes in HNO3 and NH3 dry deposition velocities. However, the higher dry deposition velocities of HNO3 and NH3 led to the substantial reductions of concentrations of the gaseous species, and consequently to the substantial reductions of particulate NH4NO3. Because of difficulty in evaluation of the dry deposition process of AQM, uncertainty in simulated dry deposition velocity is probably large. Therefore, the dry deposition of the gaseous species may also be one of the key factors for improvement of AQM performance for PM2.5 NO3−.
Figure 12. Percentage differences between M1 and sensitivity runs with modified HNO3 and NH3 dry deposition velocities for mean concentrations in the target area during the target periods of UMICS2 in (a) winter 2010 and (b) summer 2011.
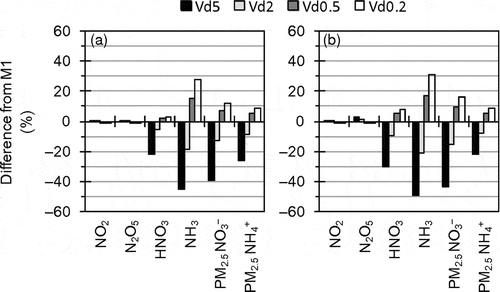
Figure 13. Percentage differences between M1 and sensitivity runs with modified HNO3 and NH3 dry deposition velocities for mean concentrations in the target area during the target periods of UMICS2 in (a) winter 2010 and (b) summer 2011 plotted against ratio of HNO3 and NH3 dry deposition velocities in sensitivity runs to those in M1.
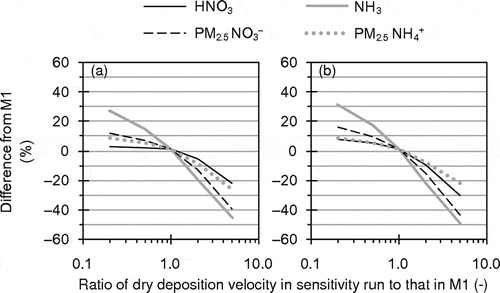
N2O5 heterogeneous reaction probability
Sensitivity analysis of N2O5 heterogeneous reactions, which substantially contribute to nocturnal HNO3 production, was conducted in D3 by M0. In sensitivity runs, the heterogeneous reaction probability of N2O5 (ΓN2O5) was set to 0 (Γ0) and 0.1 (Γ0.1). The value of 0 indicates no N2O5 heterogeneous reactions occur, and the value of 0.1 was recommended by Dentener and Crutzen (Citation1993) and used in CMAQ prior to version 4.3, and is now recognized as an upper estimate (Evans and Jacob, Citation2005). In addition, sensitivity runs were conducted with the parameterizations of ΓN2O5 used in older CMAQ aerosol modules: AERO3 (Γae3) (Riemer et al., Citation2003) and AERO4 (Γae4) (Evans and Jacob, Citation2005). The parameterization in AERO5 (M0) is based on Davis et al. (Citation2008).
shows results of the sensitivity analysis of ΓN2O5. The large ΓN2O5 value in Γ0.1 led to substantial PM2.5 NO3− production from N2O5. The differences between the results of Γ0 and M0 indicate the contribution of N2O5 heterogeneous reactions in M0, which are about 20% in the winter and 10 % in the summer for PM2.5 NO3−. Therefore, uncertainty in the heterogeneous reactions is not a dominant cause of the overestimation of PM2.5 NO3− concentration. While the results of Γae4 are similar to those of M0, the Γae3-simulated PM2.5 NO3− concentrations are slightly smaller than the M0-simulated values. This finding indicates that ΓN2O5 values in Γae3 are smaller than those in M0, which is consistent with Davis et al. (Citation2008). The overestimation of PM2.5 NO3− can be slightly mitigated by using the method in AERO3. Note that it is not certain whether the method is appropriate or not because the parameterizations themselves are not validated in this study.
Modification of multiple factors
Another sensitivity analysis (Mmod), in which modifications of multiple factors that could mitigate the overestimation of PM2.5 NO3− concentration were applied to M0, was conducted in D1 to D3. Mmod is different from M0 in using the in-line photolysis rate calculation, the modified seasonal variation of NH3 emission, fivefold dry deposition velocities of HNO3 and NH3, and the ΓN2O5 parameterization in AERO3. As described in the previous chapters, the changes in NH3 emission and dry deposition velocities had large effects.
shows results of the sensitivity analysis in the target area during the target periods of UMICS2. The Mmod-simulated HNO3 concentration was substantially smaller than the M0-simulated value because of the large contribution of the effect of higher dry deposition velocity and the small contributions of the effects of the in-line photolysis rate calculation and the ΓN2O5 parameterization in AERO3. In the winter, the difference between M0- and Mmod-simulated NH3 concentrations was relatively small because the effect of higher dry deposition velocity was compensated by the effect of larger NH3 emission. Meanwhile in the summer, the Mmod-simulated NH3 concentration was substantially smaller than the M0-simulated value because of the combined effect of higher dry deposition velocity and smaller NH3 emission. The substantial decreases in the gaseous species led to substantial decreases in particulate NH4NO3 concentration.
Figure 15. Percentage differences between M0 and Mmod for mean concentrations in the target area during the target periods of UMICS2 in (a) winter 2010 and (b) summer 2011.
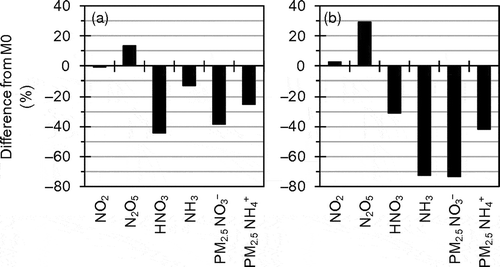
summarizes comparisons between the observed and Mmod-simulated concentrations of PM2.5 SO42−, NO3−, and NH4+, and gaseous HNO3 and NH3 in winter 2010 and summer 2011. shows time series of the observed, M0-simulated and Mmod-simulated 4-hr concentrations in summer 2011. The Mmod-simulated PM2.5 SO42− concentrations were slightly smaller than the M0-simulated values because of the decreases in NH3 concentrations. The Mmod-simulated PM2.5 NO3−, and NH4+ and the HNO3 and NH3 concentrations were substantially smaller than the M0-simulated values as described earlier. Consequently, the Mmod-simulated PM2.5 NO3− and NH3 concentrations were generally closer to the observations than the M0-simulated values. Although using fivefold dry deposition velocities may be unrealistic and has no theoretical support, the results indicate that such large uncertainties still exist in some key processes that control AQM performance. In addition, it is possible that some factors other than those investigated in this study, such as the vertical mixing process, also substantially affect AQM performance for PM2.5 NO3−.
Table 4. Comparisons of observed and Mmod-simulated concentrations of PM2.5 SO42−, NO3−, and NH4+, and gaseous HNO3 and NH3
Summary
This paper described the results of UMICS2, which was conducted in order to improve AQM performance for the major ionic components of PM2.5 in the Greater Tokyo Area of Japan. The common data sets, including meteorological fields, emission data, and boundary concentrations, were prepared for the periods of UMICS2 in winter 2010 and summer 2011. While M0, the baseline simulation case of UMICS2, approximately reproduced the concentration level and temporal variation of PM2.5 SO42−, the model clearly overestimated PM2.5 NO3− concentration because of the overestimation of particulate NH4NO3 production.
In order to investigate factors influencing AQM performance for PM2.5 NO3−, this study conducted sensitivity analyses of the processes that were closely associated with PM2.5 NO3− concentration. The sensitivity analyses of temperature and relative humidity, which affect the gas–aerosol equilibrium of NH4NO3, indicated that prediction errors of temperature and humidity that sometimes occurred in meteorological simulations could cause prediction errors of PM2.5 NO3−. The sensitivity analysis of NOX emission indicated that PM2.5 NO3− concentration tended to be insensitive to NOX emission, and therefore improvement of NOX emission was a low-priority task for improvement of AQM performance for PM2.5 NO3−. The sensitivity analysis of N2O5 heterogeneous reactions, which substantially contribute to nocturnal HNO3 production, indicated that uncertainty in the heterogeneous reactions was not a dominant cause of the overestimation of PM2.5 NO3−. The sensitivity analysis of seasonal variation of NH3 emission showed that the change in NH3 emission directly affected NH3 concentration, and substantially affected the formation of NH4NO3. The sensitivity analysis of HNO3 and NH3 dry deposition, which is an important removal path for them, showed that the higher dry deposition velocities of the gaseous species led to the substantial reductions of concentrations of them and NH4NO3. Because uncertainties in NH3 emission and dry deposition processes are probably large, these processes may be key factors for improvement of AQM performance for PM2.5 NO3−. Abundant observation data of HNO3 and NH3 concentrations, and preferably fluxes, will be required for the purpose.
Funding
This research was supported by the Environment Research and Technology Development Fund (C-1001) of the Ministry of the Environment, Japan.
Acknowledgment
The authors are grateful to NMRI and OPRF for providing the ship emission inventories.
Additional information
Notes on contributors
Hikari Shimadera
Hikari Shimadera is an assistant professor at the Center for Environmental Innovation Design for Sustainability, Osaka University, Suita, Japan.
Hiroshi Hayami
Hiroshi Hayami is a senior research scientist at the Environmental Science Research Laboratory, the Central Research Institute of Electric Power Industry, Abiko, Japan.
Satoru Chatani
Satoru Chatani is a researcher at the Toyota Central R&D Labs., Inc., Nagakute, Japan.
Yu Morino
Yu Morino is a researcher at the Center for Regional Environmental Research, the National Institute for Environmental Studies, Tsukuba, Japan.
Yasuaki Mori
Yasuaki Mori is a chief engineer at the Japan Weather Association, Tokyo, Japan.
Tazuko Morikawa
Tazuko Morikawa is a researcher at the Energy and Environment Research Division, the Japan Automobile Research Institute, Tsukuba, Japan.
Kazuyo Yamaji
Kazuyo Yamaji is a scientist at the Research Institute for Global Change, the Japan Agency for Marine-Earth Science and Technology, Yokohama, Japan.
Toshimasa Ohara
Toshimasa Ohara is the director of the Center for Regional Environmental Research, the National Institute for Environmental Studies, Tsukuba, Japan.
References
- Binkowski, F.S, S. Arunachalam, Z. Adelman, and J. Pinto. 2007. Examining photolysis rates with a prototype on-line photolysis module in CMAQ. J. Appl. Meteor. Clim. 46:1252–56. doi:10.1175/JAM2531.1
- Bond, T.C., D.G. Streets, K.F. Yarber, S.M. Nelson, J.-H. Woo, and Z. Klimont. 2004. A technology-based global inventory of black and organic carbon emissions from combustion. J. Geophys. Res. 109:D14203. doi:10.1029/2003JD003697
- Byun, D.W., and K.L. Schere. 2006. Review of the governing equations, computational algorithms, and other components of the Models-3 Community Multiscale Air Quality (CMAQ) modelling system. Appl. Mech. Rev. 59:51–77. doi:10.1115/1.2128636
- Carter, W.P.L. 2000. Documentation of the SAPRC-99 chemical mechanism for VOC reactivity assessment. Final Report to California Air Resources Board, Contract No. 92-329, and (in part) 95-308. http://www.cert.ucr.edu/~carter/absts.htm#saprc99
- Chang, J.S., R.A. Brost, I.S.A. Isaksen, S. Madronich, P. Middleton, W.R. Stockwell, and C.J. Walcek. 1987. A three-dimensional Eulerian acid deposition model: Physical concepts and formulation. J. Geophy. Res. 92:14681–700. doi:10.1029/JD092iD12p14681
- Chatani, S., T. Morikawa, S. Nakatsuka, S. Matsunaga, and H. Minoura. 2011. Development of a framework for a high-resolution, three-dimensional regional air quality simulation and its application to predicting future air quality over Japan. Atmos. Environ. 45:1383–93. doi:10.1016/j.atmosenv.2010.12.036
- Chatani, S., Y. Morino, H. Shimadera, H. Hayami, Y. Mori, K. Sasaki, , M. Kajino, , T. Yokoi, , T. Morikawa, and T. Ohara. 2014. Multi-model analyses of dominant factors influencing elemental carbon in Tokyo Metropolitan Area of Japan. Aerosol Air Qual. Res. 14:396–405. doi:10.4209/aaqr.2013.02.0035
- Colella, P., and P.L. Woodward. 1984. The piecewise parabolic method (PPM) for gasdynamical simulations. J. Comput. Phys. 54:174–201. doi:10.1016/0021-9991(84)90143-8
- Davis, J.M., P.V. Bhave, and K.M. Foley. 2008. Parameterization of N2O5 reaction probabilities on the surface of particles containing ammonium, sulfate, and nitrate. Atmos. Chem. Phys. 8:5295–311. doi:10.5194/acp-8-5295-2008
- Dentener, F. J., and P. J. Crutzen. 1993. Reaction of N2O5 on tropospheric aerosols: Impact on the global distributions of NOX, O3, and OH. J. Geophys. Res. 98:7149–63. doi:10.1029/92JD02979
- Dudhia, J. 1989. Numerical study of convection observed during the Winter Monsoon Experiment using a mesoscale two-dimensional model. J. Atmos. Sci. 46:3077–107. doi:10.1175/1520-0469(1989)046<3077:NSOCOD>2.0.CO;2
- Emmons, L.K., S. Walters, P.G. Hess, J.-F. Lamarque, G.G. Pfister, D. Fillmore, C. Granier, A. Guenther, D. Kinnison, T. Laepple, J. Orlando, X. Tie, G. Tyndall, C. Wiedinmyer, S. L. Baughcum, and S. Kloster. 2010. Description and evaluation of the Model for Ozone and Related chemical Tracers, version 4 (MOZART-4). Geosci. Model Dev. 3:43–67. doi:10.5194/gmd-3-43-2010
- European Environment Agency. 2009. EMEP/EEA air pollutant emission inventory guidebook—2009. http://www.eea.europa.eu/publications/emep-eea-emission-inventory-guidebook-2009 (accessed November 24, 2012)
- Evans, M.J., and D.J. Jacob. 2005. Impact of new laboratory studies of N2O5 hydrolysis on global model budgets of tropospheric nitrogen oxides, ozone and OH. Geophys. Res. Lett. 32:L09813. doi:10.1029/2005GL022469
- Guenther, A., T. Karl, P. Harley, C. Wiedinmyer, P.I. Palmer, and C. Geron. 2006. Estimates of global terrestrial isoprene emissions using MEGAN (Model of Emissions of Gases and Aerosols from Nature). Atmos. Chem. Phys. 6:3181–210. doi:10.5194/acp-6-3181-2006
- Hasegawa, S., S. Yonemochi, K. Kumagai, N. Yamaguchi, H. Hagino, K. Sekiguchi, A. Iijima, H. Shimadera, and H. Hayami. 2012. Simultaneous high-time resolution observations of PM2.5 components in Kanto area in summer and winter. Proceedings of the 29th Symposium on Aerosol Science & Technology, I06 ( in Japanese).
- Hertel O., R. Berkowicz, J. Christensen, and O. Hov. 1993. Test of two numerical schemes for use in atmospheric transport-chemistry models. Atmos. Environ. 27A:2591–611. doi:10.1016/0960-1686(93)90032-T
- Hong, S.-Y., J. Dudhia, and S.-H. Chen. 2004. A revised approach to ice microphysical processes for the bulk parameterization of clouds and precipitation. Monthly Weather Rev. 132:103–20. doi:10.1175/1520-0493(2004)132<0103:ARATIM>2.0.CO;2
- Kain, J.S. 2004. The Kain–Fritsch convective parameterization. An update. J. Appl. Meteorol. 43:170–81. doi:10.1175/1520-0450(2004)043<0170:TKCPAU>2.0.CO;2
- Kannari, A., Y. Tonooka, T. Baba, and K. Murano. 2007. Development of multiple-species 1 km × 1 km resolution hourly basis emissions inventory for Japan. Atmos. Environ. 41:3428–39. doi:10.1016/j.atmosenv.2006.12.015
- Kim, Y.-P., J.H. Seinfeld, and P. Saxena. 1993. Atmospheric gas–aerosol equilibrium I. Thermodynamic model. Aerosol Sci. Technol. 19:157–81. doi:10.1080/02786829308959628
- Ministry of the Environment. 2012. Report on monitoring of fine particulate matter. http://www.env.go.jp/air/osen/jokyo_h22/pm25full.pdf (accessed November 24, 2012) (in Japanese).
- Minoura, H., K. Takahashi, J.C. Chow, and J.G. Watson. 2006. Multi-year trend in fine and coarse particle mass, carbon, and ions in downtown Tokyo, Japan. Atmos. Environ. 40:2478–87. doi:10.1016/j.atmosenv.2005.12.029
- Mlawer, E.J., S.J. Taubman, P.D. Brown, M.J. Iacono, and S.A. Clough. 1997. Radiative transfer for inhomogeneous atmospheres: RRTM, a validated correlated-k model for the longwave. J. Geophys. Res. 102:16663–82. doi:10.1029/97JD00237
- Morino, Y., S. Chatani, H. Hayami, K. Sasaki, Y. Mori, T. Morikawa, T. Ohara, S. Hasegawa, and S. Kobayashi. 2010. Inter-comparison of chemical transport models and evaluation of model performance for O3 and PM2.5 prediction—Case study in the Kanto area in summer 2007. J. Jpn. Soc. Atmos. Environ. 45:212–26 (in Japanese).
- Nansai, K., N. Suzuki, K. Tanabe, S. Kobayashi, and Y. Moriguchi. 2004. Design of Georeference-Based Emission Activity Modeling System (G-BEAMS) for Japanese emission inventory management. Proc., 13th International Emission Inventory Conference, 1–11, in Clearwater, Florida.
- National Institute for Environmental Studies. 2012. National Greenhouse Gas Inventory Report of JAPAN. CGER-I104-2012. http://www.cger.nies.go.jp/publications/report/i104 (accessed November 24, 2012)
- Nawahda, A., K. Yamashita, T. Ohara, J. Kurokawa, and K. Yamaji. 2012. Evaluation of premature mortality caused by exposure to PM2.5 and ozone in East Asia: 2000, 2005, 2020. Water, Air Soil Pollut. 223:3445–59. doi:10.1007/s11270-012-1123-7
- Nenes, A., S.N. Pandis, and C. Pilinis. 1998. ISORROPIA: A new thermodynamic equilibrium model for multiphase multicomponent inorganic aerosols. Aquat. Geochem. 4:123–52. doi:10.1023/A:1009604003981
- Neuman, J.A., D.D. Parrish, T.B. Ryerson, C.A. Brock, C. Wiedinmyer, G.J. Frost, J.S. Holloway, and F.C. Fehsenfeld. 2004. Nitric acid loss rates measured in power plant plumes. J. Geophys. Res. 109:D23304. doi:10.1029/2004JD005092
- Ohara, T., H. Akimoto, J. Kurokawa, N. Horii, K. Yamaji, X. Yan, and T. Hayasaka. 2007. An Asian emission inventory of anthropogenic emission sources for the period 1980–2020. Atmos. Chem. Phys. 7:4419–44. doi:10.5194/acp-7-4419-2007
- Pinder, R.W., P.J. Adams, A.N. Pandis, and A.B. Gilliland. 2006. Temporally-resolved ammonia emission inventories: Current estimates, evaluation tools, and measurement needs. J. Geophys. Res. 111:D16310. doi:10.1029/2005JD006603
- Pleim, J.E. 2007. A combined local and non-local closure model for the atmospheric boundary layer. Part 1: Model description and testing. J. Appl. Meteor. Clim. 46:1383–95. doi:10.1175/JAM2539.1
- Pleim, J.E., and J.S. Chang. 1992. A non-local closure model in the convective boundary layer. Atmos. Environ. 26A:965–81. doi:10.1016/0960-1686(92)90028-J
- Pleim, J.E., A. Xiu, P.L. Finkelstein, and T.L. Otte. 2001. A coupled land-surface and dry deposition model and comparison to field measurements of surface heat, moisture, and ozone fluxes. Water Air Soil Pollut. Focus 1:243–52. doi:10.1023/A:1013123725860
- Riemer, N., H. Vogel, B. Vogel, B. Schell, I. Ackermann, C. Kessler, and H. Hass. 2003. Impact of the heterogeneous hydrolysis of N2O5 on chemistry and nitrate aerosol formation in the lower troposphere under photosmog conditions. J. Geophys. Res. 108:4144. doi:10.1029/2002JD002436
- Sandu, A., J.G. Verwer, J.G., Blom, E.J. Spee, G.R. Carmichael, and F.A. Potra. 1997. Benchmarking stiff ODE solvers for atmospheric chemistry problems. II: Rosenbrock solvers. Atmos. Environ. 31:3459–72. doi:10.1016/S1352-2310(97)83212-8
- Shimadera, H., A. Kondo, A. Kaga, K.L. Shrestha, and Y. Inoue. 2009. Contribution of transboundary air pollution to ionic concentrations in fog in the Kinki Region of Japan. Atmos. Environ. 43:5894–907. doi:10.1016/j.atmosenv.2009.08.022
- Shimadera, H., H. Hayami, Y. Morino, T. Ohara, S. Chatani, S. Hasegawa, and N. Kaneyasu. 2013. Analysis of summer-time atmospheric transport of fine particulate matter in Northeast Asia. Asia-Pacific J. Atmos. Sci. 49: 347–360. doi:10.1007/s13143-013-0033-y
- Skamarock, W.C., and J.B. Klemp. 2008. A time-split nonhydrostatic atmospheric model for weather research and forecasting applications. J. Comput. Phys. 227:3465–85. doi:10.1016/j.jcp.2007.01.037
- Streets, D.G., T.C. Bond, G.R. Carmichael, S.D. Fernandes, Q. Fu, D. He, Z. Klimont, S.M. Nelson, N.Y. Tsai, M.Q. Wang, J.H. Woo, and K.F. Yarber. 2003. An inventory of gaseous and primary aerosol emissions in Asia in the year 2000. J. Geophys. Res. 108:8809. doi:10.1029/2002JD003093
- Ueda, K., H. Nitta, M. Ono, and A. Takeuchi. 2009. Estimating mortality effects of fine particulate matter in Japan: A comparison of time-series and case-crossover analyses. J. Air Waste Manage. 59:1212–18. doi:10.3155/1047-3289.59.10.1212
- Willmott, C.J. 1981. On the validation of models. Phys. Geogr. 2:184–94.
- Xiu, A., and J.E. Pleim. 2001. Development of a land surface model part I: Application in a mesoscale meteorology model. J. Appl. Meteor. 40:192–209. doi:10.1175/1520-0450(2001)040<0192:DOALSM>2.0.CO;2
- Zhang, L., J.R. Brook, and R. Vet. 2003. A revised parameterization for gaseous dry deposition in air-quality models. Atmos. Chem. Phys. 3:2067–82. doi:10.5194/acp-3-2067-2003
- Zhang, Q., D.G. Streets, G.R. Carmichael, K. He, H. Huo, A. Kannari, Z. Klimont, I. Park, S. Reddy, J.S. Fu, D. Chen, L. Duan, Y. Lei, L. Wang, and Z. Yao. 2009. Asian emissions in 2006 for the NASA INTEX-B mission. Atmos. Chem. Phys. 9:5131–53. doi:10.5194/acp-9-5131-2009