Abstract
Fine particulate matter (PM2.5) samples were simultaneously collected on Teflon and quartz filters between February 2010 and February 2011 at an urban monitoring site (CAMS2) in Dhaka, Bangladesh. The samples were collected using AirMetrics MiniVol samplers. The samples on Teflon filters were analyzed for their elemental composition by PIXE and PESA. Particulate carbon on quartz filters was analyzed using the IMPROVE thermal optical reflectance (TOR) method that divides carbon into four organic carbons (OC), pyrolized organic carbon (OP), and three elemental carbon (EC) fractions. The data were analyzed by positive matrix factorization using the PMF2 program. Initially, only total OC and total EC were included in the analysis and five sources, including road dust, sea salt and Zn, soil dust, motor vehicles, and brick kilns, were obtained. In the second analysis, the eight carbon fractions (OC1, OC2, OC3, OC4, OP, EC1, EC2, EC3) were included in order to ascertain whether additional source information could be extracted from the data. In this case, it is possible to identify more sources than with only total OC and EC. The motor vehicle source was separated into gasoline and diesel emissions and a fugitive Pb source was identified. Brick kilns contribute 7.9 μg/m3 and 6.0 μg/m3 of OC and EC, respectively, to the fine particulate matter based on the two results. From the estimated mass extinction coefficients and the apportioned source contributions, soil dust, brick kiln, diesel, gasoline, and the Pb sources were found to contribute most strongly to visibility degradation, particularly in the winter.
Fine particle concentrations in Dhaka, Bangladesh, are very high and cause significant degradation of urban visibility. This work shows that using carbon fraction data from the IMPROVE OC/EC protocol provides improved source apportionment. Soil dust, brick kiln, diesel, gasoline, and the Pb sources contribute strongly to haze, particularly in the winter.
Introduction
Atmospheric particles are major carriers of black carbon (BC), sulfur, nitrogen, and trace metals, as well as crustal elements, and cause changes in climate, fertilization of the oceans and land, acidification of lakes, and health effects to humans. Particle detection over land was studied by the POLDER research group (CitationDeuze et al., 2001). The semiquantitative absorbing aerosol index derived from the TOMS ozone sensor provided useful information about the spatial and temporal pattern of dust and smoke events over the ocean as well as over land (CitationHerman et al., 1997). The global pattern of seasonal horizontal extinction coefficient over the land has been studied (CitationHusar et al., 2000). However, there is still little information available on light extinction related to specific chemical components or apportioned source contributions in the ambient aerosol, especially in the case of Bangladesh, which each year experiences significant haze problems during the winter months.
Dhaka is one of the world's megacities with some of the worst air quality (CitationGurjar et al., 2008), resulting in the likelihood of substantial health impacts (CitationGurjar et al., 2010). There have been studies of the particulate matter concentrations and compositions for over a decade (CitationSalam et al., 2003: CitationBiswas et al., 2003; CitationBegum et al., 2004, 2005, Citation2010, 2013). Over the past 12 years, there was an initial decrease in PM2.5 concentrations up to 2003. There was then an increase from 2004 to 2006 to annual average values of approximately 35 μg/m3 that have stayed relatively constant since 2006 (CitationBegum et al., 2013). Many of these studies have included receptor modeling to identify the major source types contributing to the observed PM. These analyses have been hampered by limited measurements of carbonaceous species in which only black carbon (BC) was measured using reflectometry. During this study period, a similar set of sources, including motor vehicles, brick kilns, biomass burning, and small industrial sources, has been identified using receptor modeling. Based on these studies, the particulate pollution is likely to have resulted from both locally emitted and transported material.
In order to better resolve the sources of fine particulate matter, a special study was performed that measured the concentrations of organic (OC) and elemental (EC) carbon in PM2.5 samples over the course of 1 year (CitationBegum et al., 2013). This paper presents the separation of PM2.5 sources using PMF modeling in two ways. In the first model, only total OC and EC data were included in the elemental data. Second, the fractions of OC and EC data were included in the compositional data set to further apportion the sources of fine PM. The resolved source contributions were then combined with visibility data from a nearby meteorological observatory to determine the particle sources that contributed to the local aerosol extinction coefficient.
Materials and Methods
Site description and measurement period
The samples were collected at an urban site at Dhaka, Bangladesh. PM sampling was performed using Air Metrics MiniVol samplers (CitationBaldauf et al., 2001) for collecting PM2.5 samples from the Farm Gate continuous air monitoring station (CAMS 2) site () in Dhaka. The Farm Gate site is a traffic hot spot site (latitude: 23.76° N; longitude: 90.39° E) due to the proximity of several major roadways intersecting and large numbers of vehicles plying through the area (CitationBegum et al., 2005). The site is in the mixed area (i.e., commercial and semi-industrial area). The Tejgaon industrial area is very near to the site.
At the Farm Gate site, the samplers were placed on the flat roof of the guardhouse of Bangladesh Agricultural Research Council (BARC). This location is known as CAMS 2 site started in 2009 and operated by the Department of Environment (DoE). The MiniVols were programmed to sample at 5 lpm through PM2.5 particle size separator (impactor) and then through 2-μm-pore Teflon and quartz filters, respectively, in the two samplers. The inlets of the samplers were 45 cm apart from each other. The actual flow rate should be 5 lpm at ambient conditions for proper size fractionation. To ensure a constant flow of 5 lpm through the size separator at different air temperatures and ambient pressures, the sampler flow rates were adjusted for the ambient conditions at the sampling site. The MiniVol sampler was positioned with the intake upward and located in an unobstructed area at least 30 cm from any obstacle to air flow, and the sampler inlet was placed at a height of 10 m above ground level for the Farm Gate area. The intake manifold at the Farm Gate location is located about 5 m away from a major road.
The sampling protocol was every third day starting from February 8, 2010, and sampling continued until February 28, 2011. All samples were collected over 24 hr. The clean, conditioned filters were loaded in their respective filter holder assembly at the CAMS conditioning room and were brought to the sampling site in a separate, clean polyethylene bag on each sampling day. After sampling, the filter holder assemblies (keeping the exposed filters inside) were brought into the conditioning room of the Atomic Energy Centre Dhaka (AECD) directly from the sampling site for conditioning and the PM mass measurement. Care was taken in transporting the exposed filter holder assemblies so that there would be no PM loss. The loaded quartz filters were kept in a freezer at –20°C.
PM mass, BC, and carbon fraction analysis
PM mass values were measured in the Chemistry Lab of the Atomic Energy Centre, Dhaka (AECD). The PM2.5 mass concentrations were determined by weighing the filters before and after exposure using a microbalance (CitationBegum et al., 2006a). The filters were equilibrated for 24 hr at a constant humidity of 50% and constant temperature (22°C) in the balance room before each weighing. A Po-210 (alpha emitter) electrostatic charge eliminator was used to remove the static charge accumulated on the Teflon filters before each weighing. The difference in weights for each filter was calculated and the mass concentrations for each PM2.5 sample were determined.
The PM2.5 samples collected on Teflon filters were analyzed with an EEL-type Smoke Stain Reflectometer (Diffusion Systems, Ltd) to measure BC. Secondary standards of known black carbon concentrations were used to calibrate the reflectometer (CitationBiswas et al., 2003). The concentrations are defined based on the amount of reflected light that is absorbed by the filter sample and an assumed mass absorption coefficient. It is related to the concentration of light-absorbing carbon through standards of carbon with known areal density. Iron (Fe) has a moderate light absorption coefficient and can have some limited influence on the BC value measured by reflectance. The uncertainty associated with the BC measurement is rather high (4–9%), and therefore, the influence of variation in Fe concentration on BC measurement has been neglected.
Carbonaceous materials collected on the PM2.5 quartz filters were analyzed via the IMPROVE thermal optical reflectance (TOR) protocol (CitationChow et al., 1993; CitationChow et al., 2007) for eight temperature-resolved carbon fractions (Atmoslytic model DRI-2100A). This protocol provides concentrations of carbon in four organic carbon fractions (OC1, OC2, OC3, OC4), three elemental carbon fractions (EC1, EC2, EC3), and pyrolyzed carbon (OP). The total OC is the sum of the fractions of OC1, OC2, OC3, OC4, and OP. The total EC is obtained as the sum of (EC1 – OP) + EC2 + EC3. The EC and BC values are very well correlated for samples collected at this site (CitationSalako et al., 2012).
Multielemental analysis
Multielemental analyses of the air particulate samples were made using ion beam analysis (IBA) at the Institute of Geological and Nuclear Science (IGNS), New Zealand. The x-ray spectra obtained from IBA measurements were analyzed using the computer code GUPIX (CitationMaxwell et al., 1989; CitationMaxwell et al., 1995). Elemental analyses from H to Pb were performed on the Teflon filters using IBA (CitationCohen et al., 1996). The method is described in detail elsewhere (CitationLandsberger and Creachman, 1998). Among 25 elements determined including H for each fraction of 126 samples, 10 elements, namely, P, Sc, V, Ni, Ga, Ge, As, Se, Rb, and Sr, had more than 80% of the values missing or below the detection limits and were eliminated from the analysis. H was converted to OMH, which represents organic carbon containing hydrogen, using OMH =11([H] – 0.25 × [S]). The regression slope and correlation coefficient between OMH and OC where OC was determined using carbon analyzer is slope = 1.01, r 2 = 0.77, respectively. Data on the concentrations of 14 elements (namely, Na, Al, Si, S, Cl, K, Ca, Ti, Cr, Fe, Cu, Zn, Br, and Pb), black carbon, organic carbon, and mass were available for data analysis. After having complete analysis of samples, with the elemental data together with mass, OC, and BC, there is an attempt to observe the data quality by the reconstructed mass (RCM) analysis. The data quality of the available elemental concentration together with mass, OC, and BC was tested by reconstructed mass (RCM) analysis through comparing the computed RCM values with the gravimetric weight of the filters, where the least-squares fit to the data was RCM = 0.67 × Weight with an R 2 = 0.92 in case of fine mass.
Meteorological and visibility data
In Bangladesh, the climate is characterized by high temperatures and high humidity most of the year and distinctly marked seasonal variations in precipitation. Based on the meteorological conditions (CitationSalam et al., 2003), the year can be divided into four seasons: pre-monsoon (March–May), monsoon (June–September), post-monsoon (October–November), and winter (December–February). The dispersion of PM strongly depends on the wind speed and direction (CitationBegum et al., 2008). Meteorological data such as wind speed, wind direction, rainfall, humidity, temperature, and visibility data according to sampling dates were collected from the nearby meteorological station, which is located about 2 km north of the sampling site.The Bangladesh Meteorological Department is the authorized government organization for all meteorological activities in the country. It maintains a network of surface and upper air observatories, radar and satellite stations, agro-meteorological observatories, geomagnetic and seismological observatories, and meteorological telecommunication systems. The Meteorological Department collects meteorological data every 3 hr.
The visual range was estimated by observation at the meteorological station every 3 hr from a vantage point 2 m above the ground. The observational procedures are specified in the guidelines issued by the CitationWorld Meteorological Organization (WMO, 2008). The extinction coefficient, bext, was derived from each surface visual range observation using the Koschmieder relationship (CitationKoschmieder, 1926). The targets are typically small and at quantized distances from the observer. These conditions will result in a lower reported visual range. The Koschmieder constant, K, was taken to be 1.9 (CitationHusar et al., 2000). This value was chose to reflect that the targets are not black so that the contrast is lower than ideal. The resulting values were then averaged to provide 24-hr values that will be analyzed with the PM2.5 data and source apportionment results.
Positive matrix factorization modeling
Positive matrix factorization (PMF) is a source-receptor model that solves the mass balance equation:
An important feature of PMF2 is FPEAK, which was used to control rotations. By setting the value of FPEAK, the routine is forced to subtract the F factors from each other, yielding more physically realistic solution (CitationPaatero et al., 2002). An additional approach called G-space plotting for PMF models (CitationPaatero et al., 2005) was used to explore the rotational ambiguity. The G-space plotting helps to identify the edges that show if the factors are correlated with one another. Rotations can then be controlled by FPEAK until an appropriate distribution of the edges is achieved. In the present work, FPEAK was set at 0.1 based on the examination of the profiles and the G-space profiles.
A major limitation of the PMF2 software is the very limited estimation of the uncertainties and thus it is not possible to estimate errors in the PMF analysis. For the determination of number of factors in PMF, the primary consideration was to obtain a good fit of the model to the original data. The theoretical Q-value should be approximately equal to the number of degree of freedom, or approximately equal to the number of entries in the data array, provided that correct values of sij have been used. It is helpful to examine the distributions of scaled residuals (eij/sij ). In a well-fit model, the residuals eij and the error estimates sij should be about equal and the ratios eij/sij should lie in the range within ±2 (CitationPaatero and Tapper, 1994). Often there is no reliable information on sij and the presence of outliers (even in the robust mode) complicates the analysis. The explained variation, EV (CitationCrawford et al., 2005), summarizes how important each factor element is in explaining the amount of each element. Values range from 0 to 1 so the EV value shows which factors are providing most of the given species to the observed concentrations.
Conditional probability function (CPF)
To analyze point source impacts from various wind directions, the conditional probability function (CPF) (CitationKim et al., 2003) was calculated using source contribution estimates from PMF, coupled with wind direction values measured on site. To minimize the effect of atmospheric dilution, daily fractional mass contribution from each source relative to the total of all sources was used rather than using the absolute source contributions. The same daily fractional contribution was assigned to each hour of a given day to match to the hourly wind data. Specifically, the CPF is defined as
Results and Discussion
The time series of fine PM fraction (FPM) mass, black carbon (BC), organic carbon (OC), and extinction coefficients (bext) are presented in . provides the average chemical species concentrations with their standard deviations for this study.
Table 1. Summary of fine particulate mass, fractions of OC and EC, and elemental concentrations (ng/m3) used for PMF modeling (2010–2011)
Figure 2. Variation of PM fraction (FPM), black carbon (BC), organic carbon (OC), and bext during the study period.
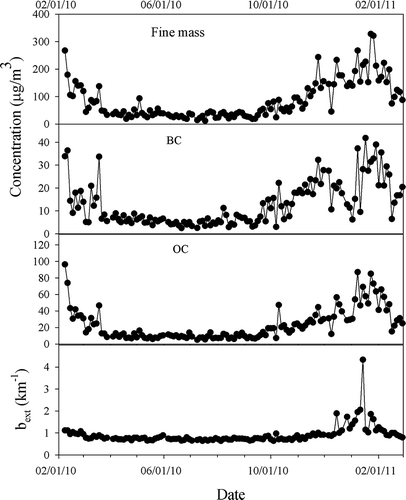
Using the data with total OC and EC, the PMF modeling resolved only five sources. Using the OC and EC fraction data, seven sources were obtained. The average source contributions derived from the PMF modeling are presented in . The PMF solution was evaluated by comparing the predicted mass of fine fractions (sum of the contributions from resolved sources) with measured mass concentrations. The regression slopes and coefficients are slope = 0.95, r 2 =0.93 for total OC and EC data and slope = 0.88, r 2 = 0.91for the OC and EC fraction data.
Table 2. Average source contributions derived from the PMF modeling
Results using total OC and EC
Using total OC and EC concentrations, five sources were resolved by the PMF2 modeling. The identified source profiles are road dust, sea salt and Zn source, soil dust, motor vehicle, and brick kiln and are presented in . The bars show the concentrations of the species in each profile and the points show the explained variation.
Figures 4 and 5 present the time series of the estimated daily contribution for each source and the CPF plots showing the relationships of source contributions with the wind direction, respectively. The road dust source profile is characterized by high OC with respect to EC, Mg, Al, Si, S, K, Ca, Ti, Fe, Ni, and Zn (). The contribution of Ni and S may come from heavy-duty diesel vehicles that operate in the evening between 10 p.m. and 6 a.m. There are many shopping complexes and hospital near the monitoring site. The road adjacent to this site is always busy. The monitor is influenced by the source located in the northwest and southwest direction () and the major roadways connecting Dhaka to the northern part of Bangladesh is in the northwest direction. There are also main roads in the southwest direction that are connected to the city center (CitationBegum et al., 2005). From the examination of the time of year (), it is seen that the concentrations of this source are somewhat depressed during the monsoon quarter compared to the other three when there is very little precipitation. This source has no weekday or weekend effect.
Figure 4. Time-series plots of source contributions for fine particulate matter using total OC and EC.
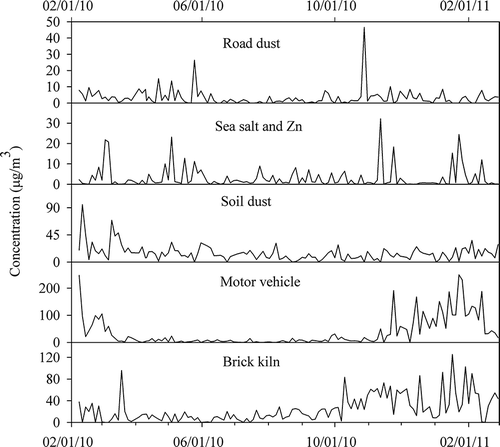
The second factor contains the signature of both sea salt combined with a Zn source. The source profile includes high Na, Cl, Zn, and Pb. The site was influenced by sources located in southwesterly and southeasterly directions (CitationBegum et al., 2005) (). This source has largest influence during May to June. There are also a few peaks in November and January when the wind is from the south and the Bay of Bengal (CitationBegum et al., 2005).
The soil dust factor contains high Al, Si, K, Ca, Fe, S, OC, and EC, and has more influence by northwesterly and southwesterly wind. This source has higher contribution during winter compared to any other season. From G space plotting, it has been found that the factors soil dust and motor vehicle are correlated and are influenced by the same direction. This correlation of these basically collocated sources causes the soil dust factor to be admixed with OC, EC, and S.
The fourth factor is motor vehicle and has a signature of high OC, EC, and S, together with the road dust profile. In Bangladesh, there is no effective mechanism for removal of surface road dust. This road dust is resuspended by the vehicular movement, given relatively constant heavy traffic. This factor shows high contributions during winter and is influenced by northwesterly wind. A large intercity bus station that provides connections between northern Bangladesh and Dhaka stands in this direction. Although buses have been converted into compressed natural gas (CNG) engines, heavy-duty diesel vehicles still operate on these long-distance routes.
The fifth factor is brick kiln and has signature species of OC, EC, S, K, Pb, and other crustal elements. This source shows a high contribution during the winter season and is influenced by northwesterly wind. There are large clusters of brick kilns on the northwestern side of Dhaka. They are mainly operated in the winter each year, since during the monsoon season the area is often flooded. Coal and some wood are used as fuel in those kilns. A few kilns are operated on gas but their numbers are very limited.
PMF2 results using fractions of OC and EC
Adding the OC and EC fraction concentration to the data set allowed seven sources to be resolved. The identified source profiles are road dust, sea salt and Zn source, soil dust, brick kiln, gasoline, diesel, and fugitive Pb sources (). and present the time-series plots of the estimated daily contribution from each of the sources and the relationship of source contribution with wind direction, respectively. The road dust source profile is characterized by OC2, OC3, OC4, and OP with respect to EC1, Mg, Al, Si, S, K, Ca, Ti, Fe, Cu, and Zn (). This source is found to be related to winds from the northwesterly and southwesterly directions (). From time-series plot of contributions (), it is seen that this source has high values in the winter.
Figure 7. Time-series plots of source contributions for fine particulate matter using fractions of OC and EC.
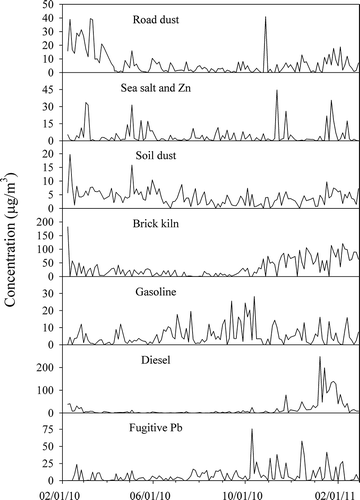
The second factor contains a signature of both sea salt and a Zn source. The source is mixed with high Na, Cl, Zn, and Pb and also contains some crustal elements. The site is again influenced by winds from the southwest and southeast similar to what was observed in the analysis of the reduced data set (). The source has higher contributions during May to June when transport from the marine environment is more common. There were also a few peaks in November and January when the wind came from the south.
The soil dust factor (factor 3) contains high Al, Si, K, Ca, Fe, S, and other dust species and is most highly influenced by northeasterly and southwesterly wind. This source has high contributions during the winter and pre-monsoon quarters. This period is typically one with little or no precipitation, and thus, soil and road dust are extensively aerosolized.
The fourth factor is brick kiln and has a signature of OC1, OC2, OC3, OC4, OP, EC1, EC2, S, and K, together with crustal elements. This factor shows high contributions during winter and is influenced by northwesterly wind as noted previously.
The fifth factor is gasoline and has signature species of OC2, EC1, Fe, and Zn together with some dust species. This factor shows a high contribution during the post-monsoon and winter quarters and is influenced by northwesterly wind. This factor would include both cars and motorbikes. The three-wheel taxis in Dhaka all are fueled by CNG (CitationBegum et al., 2006b).
The sixth factor is diesel and has signature high OC1 and S together with crustal elements. This factor shows high contribution during winter and is influenced by northwesterly wind and has a seasonal variation peaking in winter. The large intercity bus station stands on the northwest direction, which keeps a connection for the northern part of Bangladesh to the capital city, Dhaka. Traffic in Dhaka is very heavy and most driving is stop-and-go. Diesel fuel in Bangladesh contains about 3000 ppm sulfur. Heavy-duty diesel vehicles mostly use this fuel. However, they can only be used in Dhaka from 10 p.m. to 6 a.m.
The seventh factor is fugitive Pb source and has signature of Pb, K, Na, OC2, OC3, OC4, OP, and EC1. There are many Pb smelters in the southeast part of Dhaka. Pb is also used in the galvanizing factories to increase the reflective properties (CitationKrepski, 1985).
Source influences on visibility
The relationships between the coefficient of light extinction, bext, and the source contributions obtained from the PMF analyses of the PM2.5 data were explored. It was found that the mean extinction coefficient was highest during winter, December, January, and February () and lowest during the monsoon season, June to September.
Table 3. Means and standard deviations of visibility, and extinction coefficient in different season during study period
The extinction coefficients derived from the visual range observations can be related to the sources obtained from the fine particulate matter compositional data. Both forward and backward stepwise multiple linear regression analyses were performed between the bext and the source contributions from the carbon fraction data set (road dust, sea salt and Zn, soil dust, brick kiln, gasoline, diesel, and Pb). The regression model was set to have no intercept. The best solutions included five factors (): soil dust, brick kilns, gasoline, diesel, and the lead source. The adjusted squared multiple correlation coefficient equaled 0.816, showing that most of the variance in visibility could be explained by these fine PM sources.
Table 4. Results of the stepwise multiple linear regression analyses
Conclusion
It is possible to identify more sources using the data including the OC and EC fractions compared to only using total OC and EC. The motor vehicle source was separated into gasoline and diesel emissions and a fugitive Pb source was identified. Brick kilns contributed 7.9 μg/m3 and 6.0 μg/m3, respectively, to the fine particulate matter based on the two results. It has been found that the extinction coefficient per unit mass in monsoon season is higher than in winter (). From the multiple linear regression analyses between the bext and the carbon fraction PMF source apportionments (road dust, sea salt and Zn, soil dust, brick kiln, gasoline, diesel and Pb), soil dust, brick kiln, diesel, gasoline, and the Pb sources contributed most strongly to visibility degradation, particularly in the winter.
Acknowledgment
The authors are grateful to the CASE Project, DoE, for permitting the collection of these samples. They are also grateful to the IGNS, Lower Hutt, New Zealand, for analyzing the APM samples.
References
- Baldauf , R.W. , Lane , D.D. and Marotz , G.A. 2001 . Ambient air quality monitoring network design for assessing human health impacts from exposures to airborne contaminants . Environ. Monitoring Assess. , 66 : 63 – 76 . doi: 10.1023/A:1026428214799
- Begum , B.A. , Kim , E. , Biswas , S.K. and Hopke , P.K. 2004 . Investigation of sources of atmospheric aerosol at urban and semi-urban areas in Bangladesh . Atmos. Environ. , 38 : 3025 – 3038 . doi: 10.1023/A:1026428214799
- Begum , B.A. , Biswas , S.K. , Kim , E. , Hopke , P.K. and Khaliquzzaman , M. 2005 . Investigation of sources of atmospheric aerosol at a hot spot area in Dhaka, Bangladesh . J. Air Waste Manage. Assoc. , 55 : 227 – 240 . doi: 10.1023/A:1026428214799
- Begum , B.A. , Akhter , S. , Sarker , L. and Biswas , S.K. 2006a . Gravimetric analysis of air filters and quality assurance in weighing . Nucl. Sci. Appl. , 15 : 36 – 41 . doi: 10.1023/A:1026428214799
- Begum , B.A. , Biswas , S.K. and Hopke , P.K. 2006b . Impact of banning of two-stroke engines on airborne particulate matter concentrations in Dhaka, Bangladesh . J. Air Waste Manage. Assoc. , 56 : 85 – 89 . doi: 10.1023/A:1026428214799
- Begum , B.A. , Biswas , S.K. and Hopke , P.K. 2008 . Assessment of trends and present ambient concentrations of PM2.2 and PM10 in Dhaka . Air Qual. Atmos. Health , 1 : 125 – 133 . doi: 10.1023/A:1026428214799
- Begum , B.A. , Biswas , S.K. , Markwitz , A. and Hopke , P.K. 2010 . Identification of sources of fine and coarse particulate matter in Dhaka, Bangladesh . Aerosol Air Qual. Res. , 10 : 345 – 353 . doi: 10.1023/A:1026428214799
- Begum , B.A. , Hopke , P.K. and Markwitz , A. 2013 . Air pollution by fine particulate matter in Bangladesh . Atmos. Pollut. Res. , 4 : 75 – 86 . doi: 10.1023/A:1026428214799
- Biswas , S.K. , Tarafdar , S.A. , Islam , A. , Khaliquzzaman , M. , Tervahattu , H. and Kupiainen , K.J. 2003 . Impact of unleaded gasoline introduction on the concentration of lead in the air of Dhaka, Bangladesh . J. Air Waste Manage. Assoc. , 53 : 1355 – 1362 . doi: 10.1023/A:1026428214799
- Chow , J.C. , Watson , J.G. , Prichett , L.C. , Pierson , W.R. , Frazier , C.A. and Purcell , R.G. 1993 . The DRI thermal/optical reflectance carbon analysis system: Description, evaluation and applications in U.S. air quality studies . Atmos. Environ. , 27 : 1185 – 1201 . doi: 10.1023/A:1026428214799
- Chow , J.C. , Watson , J.G. , Chen , L.W.A. , Chang , M.C.O. , Robinson , N.F. , Trimble , D. and Kohl , S. 2007 . The IMPROVE_A temperature protocol for thermal/optical carbon analysis: Maintaining consistency with a long-term database . J. Air Waste Manage. Assoc. , 57 : 1023 1014 doi: 10.1023/A:1026428214799
- Cohen , D.D. , Graham , M. , Bailey , M. and Kondepudi , R. 1996 . Elemental analysis by PIXE and other IBA techniques and their application to source fingerprinting of atmospheric fine particle pollution . Nucl. Instrum. Methods (B). , 109 : 218 – 226 . doi: 10.1023/A:1026428214799
- Crawford , J. , Cohen , D. , Dyer , L. and Zahorowski , W. 2005 . “ Receptor modelling with PMF2 and ME2 using aerosol data from Hong Kong ” . In Australian Nuclear Science and Technology Organization, Lucas Heights New South Wales Report no. ANSTO/E-756, May doi: 10.1023/A:1026428214799
- Deuze , J.L. , Breon , F.M. , Devaux , C. , Goloub , P. , Herman , M. , Lafrance , B. , Maignan , F. , Marchand , A. , Nadal , F. , Perry , G. and Tanre , D. 2001 . Remote sensing of aerosols over land surface from POLDER-ADEOS1 polarized measurements . J. Geophys. Res. , 106 : 4913 – 4926 . doi: 10.1023/A:1026428214799
- Gurjar , B.R. , Butler , T.M. , Lawrence , M.G. and Lelieveld , J. 2008 . Evaluation of emissions and air quality in megacities . Atmos. Environ. , 42 : 1593 – 1606 . doi: 10.1023/A:1026428214799
- Gurjar , B.R. , Jain , A. , Sharma , A. , Agarwal , A. , Gupta , P. , Nagpure , A.S. and Lelieveld , J. 2010 . Human health risks in megacities due to air pollution . Atmos. Environ. , 44 : 4606 – 4613 . doi: 10.1023/A:1026428214799
- Herman , J.R. , Bhartia , P.K. , Torres , O. , Hsu , C. , Seftor , C. and Celarier , E. 1997 . Global distribution of UV-absorbing aerosols from Nimbus 7/TOMS data . J. Geophys. Res. , 102 : 16911 – 16922 . doi: 10.1023/A:1026428214799
- Husar , R.B. , Husar , J.D. and Martin , L. 2000 . Distribution of continental surface aerosol extinction based on visual range data . Atmos. Environ. , 34 : 5067 – 5078 . doi: 10.1023/A:1026428214799
- Kim , E. , Hopke , P.K. and Edgerton , E.S. 2003 . Source identification of Atlanta aerosol by positive matrix factorization . J. Air Waste Manage. Assoc. , 53 : 731 – 739 . doi: 10.1023/A:1026428214799
- Koschmieder , H. 1926 . Theorie der horizontalen Sichtweite . Beitr. Phys. Atmos. , 12 : 33 – 55 . doi: 10.1023/A:1026428214799
- Krepski , R.P. 1985 . “ The influence of lead in after-fabrication hot dip galvanizing ” . In In Proceedings of the 14th International Galvanizing Conference (Intergalva’85) Munich , , Germany June 9–14, 1985, pp. 6/6–6/12. London: Zinc Development Association. doi: 10.1023/A:1026428214799
- Landsberger , S. and Creatchmam , M. 1998 . Elemental analysis of airborne particles , Boca Raton , FL : CRC Press . doi: 10.1023/A:1026428214799
- Maxwell , J.A. , Campbell , J.L. and Teesdale , W.J. 1989 . The Guelph PIXE software package . Nucl. Instrum. Methods (B) , 43 : 218 – 230 . doi: 10.1023/A:1026428214799
- Maxwell , J.A. , Teesdale , W.J. and Campbell , J.L. 1995 . The Guelph PIXE software package II . Nucl. Instrum. Methods (B) , 95 : 407 – 421 . doi: 10.1023/A:1026428214799
- Paatero , P. and Tapper , U. 1993 . Analysis of different methods of factor analysis as least square fit problem . Chem. Intel. Lab. Syst. , 18 : 183 – 194 . doi: 10.1023/A:1026428214799
- Paatero , P. and Tapper , U. 1994 . Positive matrix factorization: A non-negative factor model with optimal utilization of error estimates data values . Environmetrics , 5 : 111 – 126 . doi: 10.1023/A:1026428214799
- Paatero , P. 1997 . Least squares formulation of robust non-negative factor analysis . Chem. Intel. Lab. Syst. , 37 : 23 – 35 . doi: 10.1023/A:1026428214799
- Paatero , P. , Hopke , P.K. , Song , X.-H. and Ramadan , Z. 2002 . Understanding and controlling rotations in factor analytic models . Chem. Intel. Lab. Syst. , 60 : 253 – 264 . doi: 10.1023/A:1026428214799
- Paatero , P. , Hopke , P.K. , Begum , B.A. and Biswas , S.K. 2005 . A graphical diagnostic method for assessing the rotation in factor analytical models of atmospheric pollution . Atmos. Environ. , 39 : 193 – 201 . doi: 10.1023/A:1026428214799
- Salam , A. , Bauer , H. , Kassin , K. , Ullah , S.M. and Puxbaum , H. 2003 . Aerosol chemical characteristics of a mega-city in Southeast Asia (Dhaka, Bangladesh) . Atmos. Environ. , 37 : 2517 – 2528 . doi: 10.1023/A:1026428214799
- Salako , G.O. , Hopke , P.K. , Cohen , D.D. , Begum , B.A. , Biswas , S.K. , Pandit , G.G. , Chung , Y.S. , Abd Rahman , S. , Hamzah , M.S. , Davy , P. , Markwitz , A. , Shagjjamba , D. , Lodoysamba , S. , Wimolwattanapun , W. and Bunprapob , S. 2012 . Exploring the variation between EC and BC in a variety of locations . Aerosol Air Qual. Res. , 12 : 1 – 7 . doi: 10.1023/A:1026428214799
- World Meteorological Organization . 1996 . Guide to meteorological instruments and methods of observation , 7th WMO-No. 8, Geneva, Switzerland. http://www.wmo.int/pages/prog/gcos/documents/gruanmanuals/CIMO/CIMO_Guide-7th_Edition-2008.pdf doi: 10.1023/A:1026428214799