Abstract
Visibility is a good indicator of air quality because it reflects the combined influences of atmospheric pollutants and synoptic processes. Trends in visibility and relationships with various factors in Chengdu and Chongqing, two megacities in southwest China, were analyzed using daily data from National Climatic Data Center and the Air Pollution Index (API) of the Ministry of Environmental Protection of China. Average annual visibility during the period of 1973–2010 was 8.1 ± 3.9 in Chengdu and 6.2 ± 4.3 km in Chongqing. PM10 dominates the reported primary pollutants in both cities, although concentrations have decreased from a high of 127.9 and 150 µg m3 before 2005 to 100.4 and 93.5 µg m−3 in Chengdu and Chongqing, respectively. Low average visibility and extremely high levels of PM10 were observed in winter, whereas relative humidity had irregular and weak seasonal variations. Visibility in both cities has deteriorated in comparison to the 1960s and 1970s, mostly due to the elevation of optical depth caused by anthropogenic pollution.
Correlations and principal component analysis (PCA) were undertaken to determine the key factors affecting visibility. Visibility was only moderately correlated with PM10. In Chengdu, visibility displayed weak correlations with various factors, whereas visibility in Chongqing was most strongly related to relative humidity due to the atmospheric particulates in the region containing more hygroscopic components. PCA results further confirmed that high relative humidity and low wind speed increased the occurrence of low visibility events under high PM10 concentrations. Temperature and pressure, as indicators of weather systems, also played important roles in affecting visibility. Mathematical models of visibility prediction indicated that wind speed had the largest coefficients among all meteorological factors, and reductions in PM10 concentration only led to minor improvements in visibility.
Long-term data indicates that visibility in Chengdu and Chongqing has been lower than 10 km since the 1970s, and the poor visibility primarily results from anthropogenic pollution. Although PM10 concentrations have decreased consistently to around 100 μg m−3, trends of visibility have shown no improvement but much fluctuation. Correlation and principal component analysis demonstrate that low visibility in Chengdu is influenced by high relative humidity, while in Chongqing the degrading visibility is related with high relative humidity and pressure and low wind speed under a stable weather system. The results are important to understand the widespread haze event in the two megacities of southwest China.
Supplemental Materials: Supplemental materials are available for this paper. Go to the publisher's online edition of the Journal of the Air & Waste Management Association.
Introduction
Visibility, defined as the maximum distance at which a black object can be recognized against the horizon (CitationHorvath, 1981), is routinely recorded at meteorological stations where pollutant concentrations may be unavailable (CitationLee, 1990). Globally, long-term trends in visibility are considered to be a good indicator of air pollution because visibility is greatly influenced by light extinction from atmospheric particles (CitationDoyle and Dorling, 2002; CitationSchichtel et al., 2001; CitationSloane, 1982a, 1982b, Citation1984; CitationTsai, 2005; CitationWatson, 2002). Various studies have identified large-scale visibility degradation and associated serious particulate pollution in rapidly developing regions of China (CitationChe et al., 2007; CitationChe et al., 2009; Qu et al., 2009; CitationWang et al., 2009), including the Beijing–Tianjin–Hebei area (CitationZhao et al., 2011), Yangtze River Delta (CitationGao et al., 2011; CitationHuang et al., 2008), and Pearl River Delta (CitationWu et al., 2010).
The 30-year (1981–2010) average visibility distribution in China based on data from the U.S. National Climatic Data Center (NCDC) clearly demonstrates two notable haze regions: the Yangtze River Delta and the Sichuan Basin (red-colored regions in ). Located in the southwest of the country, the Sichuan Basin is one of the most populous regions in China (about 100 million people) and is recognized as a serious haze region with visibility lower than 10 km since the 1970s. From 1953 to 1997, the annual extinction coefficient averaged over the Sichuan Basin increased by 0.015 km−1 per decade (CitationQian and Giorgi, 2000). The two megacities of Chengdu and Chongqing, which are both located in the Sichuan Basin (Cheng-Yu Area) (), have recorded the lowest visibilities among all the available meteorological stations in China. Since the West Development Project of the central government in 2000, the gross domestic products (GDPs) of Chengdu and Chongqing, along with other cities in the basin, have increased by an annual rate of more than 10%, while the consumption of coal increased drastically from 8.9 × 107 to 2.34 × 108 tonnes of standard coal equivalent (CitationChina Energy Statistical Yearbook, 1986–2010; CitationChen and Xie, 2012). The rapid urbanization and industrialization in the basin have raised concerns regarding environmental protection and visibility improvement. To develop ways to improve visibility, the mechanisms linking visibility to multiple meteorological and pollution variables must be better understood.
Figure 1. (a) Spatial distribution of 30-yr average visibility in China (Sichuan and Chongqing are the regions within the gray lines). (b) Location and elevation of the Sichuan Basin, Chengdu and Chongqing (color figure available online).
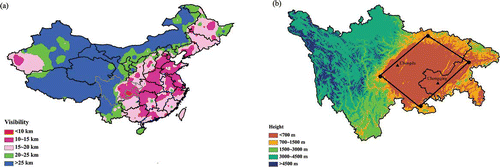
Previous studies have confirmed that visibility is related to fine particles within PM10, especially particles with a diameter less than 1 μm (CitationWatson, 2002). The total light extinction generated by atmospheric particles can be apportioned to the major components of particles, including sulfate, nitrate, organic carbon (OC), black carbon (BC), soil, and the coarse mass fraction (PM10 minus PM2.5) (CitationMalm et al., 1994). In addition, meteorological factors, especially relative humidity (RH), also influence visibility greatly. As the RH increases, hygroscopic particles progressively absorb more water, leading to increased scattering cross sections and proportionately reduced visibility. For example, the scattering cross section of ammonium sulfate could be enhanced by a factor of five or more above that of dry particles by RH above 90% (CitationMalm et al., 1994; CitationMalm and Day, 2001). However, few studies have focused on the Sichuan Basin owing to its particular location, less developed economy, and little emphasis on emission reduction. There are no continuous measurements of PM2.5, and PM10 concentrations retrieved from the Air Pollution Index (API) are so far the best available data set reflecting long-term changes in local air quality. In this study, we investigate trends in visibility in Chengdu and Chongqing, as well as their relationship with meteorological parameters including relative humidity, wind speed, and air temperature, using the daily visibility data provided by the National Climatic Data Center (NCDC). To explain the influence of atmospheric particulates, we used data for PM10 because of the lack of PM2.5 data. The results are expected to be useful for understanding the extremely low visibility and widespread haze pollution in the Sichuan Basin of southwest China, where the two megacities of Chengdu and Chongqing are located.
Data and Method
Visibility and meteorological data
Daily average meteorological data for Chengdu and Chongqing were collected from the website of the U.S. NCDC and were used to perform a visibility trend analysis from 1973 to 2010. Data prior to 1973 were not included as the data set was incomplete. The NCDC is a respected authority that develops global data sets on weather and climate information. The meteorological data set has been subjected to extensive automated quality control to correctly decode as much synoptic data as possible and to eliminate many of the random errors found in the original data. The observation sites in Chengdu and Chongqing are synoptic weather stations managed by the China Meteorological Administration and were located at 30°42′ N, 104°1′ E and 29°35′ N, 106°28′ E, respectively. In the summary of daily data, 18 surface meteorological parameters including mean temperature, mean dewpoint, mean visibility, and precipitation amount were derived from a minimum of four observations per day. The visibility data were measured by professionally trained staff using predefined reference objects at known distances. Visibility data were collected at least eight times per day and recorded in kilometers with an uncertainty of 0.1 km. Relative humidity (RH) was calculated via , using the dry bulb temperature (T, °C) and the dewpoint temperature (Td, °C) (CitationLinsley et al., 1988).
Trend analysis of visibility
Trend analysis of visibility is to reveal the anthropogenic influences on air quality, and then any natural effects on air need to be unfolded from the data (CitationSloane, 1982a). However, the interaction between air quality and meteorology is complex and much remains to be done in this area. Many previous studies have screened out the low-visibility observations made in the presence of dust, mist, precipitation, fog, and high RH (RH > 90%) (CitationChe et al., 2007; CitationSloane, 1982b), which are unrepresentative of air pollution status. In this study, daily visibility data that had missing codes, precipitation, and/or high RH (>90%) were excluded to unfold the influences of meteorological factors and emphasize anthropogenic effects. The data screen was only used in the trend analysis of visibility, but not in the following analysis to explore the relationships between visibility and various influencing factors. The average percentages of visibility data excluded due to precipitation and high RH for Chengdu and Chongqing were 23.5% and 30.6%, respectively. Detailed information about the total and the removed observations during 1973–2010 is shown in the Supplementary Material. Although the reliability of the human-made visibility observations has been supported in isolated tests, the autocorrelated nature of the data has caused problems in trend analysis. Both cumulative percentile and ridit analysis, which are free of the assumption about the independence or distribution of the data (CitationSloane, 1982a), were used for trend extraction. In addition, the days of very good (>19 km) and low (<10 km) visibility per year, which are good indicators of the long-range transport and haze situation (CitationGomez and Smith, 1987), respectively, were also analyzed for Chengdu and Chongqing.
The Nth cumulative percentile is the visibility that is equaled or exceeded N percent of the time, and the trend of a particular percentile reflects changes in the visibility level (CitationSloane, 1982a, 1982b). The commonly used 50th percentile was chosen to represent the visibility to be equaled or exceeded 50% of the time or the “median” visibility.
Ridit is an indication of the probability that a visibility observation in a given period is better than a reference visibility distribution. CitationCraig and Faulkenberry (1979) and CitationSloane (1982a) have provided detailed descriptions of the application of ridit analysis to visibility trends, and these works were consulted in this study. Ridit is defined by Equationeq 1:
Herein, both Chengdu and Chongqing have their own reference distributions, defined as the visibility records observed during the entire period (1973–2010) at each site. Annual ridits for an individual year were calculated by a comparison of that year's visibility distribution with the reference distribution. The ridit for each year was estimated by partitioning the intervals according to target availability and representing the distributions by histograms through Equationeq 2, where and
are the relative frequencies of the ith subinterval for each distribution. Five subdivisions of visibility were chosen, 0–5 km, 6–10 km, 11–15 km, 16–20 km, and >21 km. For each year distribution, ni
was the number of observations in the visibility subdivisions and n was the total number of observations, and
equaled ni/n. The term
was defined as being analogous to
but for distribution R. For each site, the trend of mean ridits represents the detailed changes in visibility. Ridit values >0.5 indicate a better visibility when compared to the entire period, and vice versa for values <0.5.
API and PM10 pollution
The API was introduced in 1997 by the former State Environmental Protection Agency of China to provide a quantitative indicator of the overall air pollution situation with respect to PM10, SO2 and NO2 levels in each city. The index ranges from 0 to 500 and is divided into five categories. API values in the range of 0–50 and 51–100 indicate clean and good air quality, respectively, whereas values of 101–200, 201–300, and 301–500 denote low-, mid-, and high-level pollution, respectively ().
Table 1. Air quality category and the upper limit of daily average concentrations for each pollutant corresponding to different API classifications
Table 2a. Summary of visibility, meteorological factors, API, and PM10 pollution in Chengdu
To obtain APIs, the daily average concentrations of PM10, SO2, and NO2 were first calculated from the continuous data of all monitoring stations dispersed in different regions of the city. Then a subpollution index (sPI) for each pollutant was induced by linear interpolation of the average concentration between the grading limits for each air quality classification in using Equationeq 3 (CitationQu et al., 2010):
The pollutant with the maximum sPI was defined as the primary pollutant, and its sPI was reported as the API for the city and day ( Equationeq 4):
The daily APIs of Chengdu and Chongqing from June 2000 to December 2011, comprised of 4215 and 4213 observations, respectively, were from the data center of the Ministry of Environmental Protection (MEP) of China (http://datacenter.mep.gov.cn/main/index.action?indexType=air). During the study period, PM10 was the primary pollutant on 84.3% and 90.9% of the total days in Chengdu and Chongqing, respectively. Daily PM10 concentrations were then retrieved from the APIs when PM10 was reported as the primary pollutant using Equationeq 5, where C, APIhigh , APIlow , Chigh , and Clow have the same denotations as Equationeq 3. Inspection and comparison demonstrate that PM10 most often has the highest API among PM10, SO2, and NO2 when the API is less than 50 and no primary pollutant is reported (CitationQu et al., 2010). As APIs are most often a reflection of the PM10 loadings, for the days with no primary pollutant reported, we assumed that PM10 was the primary pollutant and also deduced the PM10 concentrations according to Equationeq 3.
Relationship between visibility and its affecting factors
Relationship analysis is an important tool for exploring the hidden information behind the voluminous records of visibility, meteorological factors, and air pollutants. Two methods, correlation analysis and principal component analysis (PCA), were used to identify how various factors directly or indirectly affected visibility in Chengdu and Chongqing. As there are multiple factors affecting visibility and they often intercorrelate with each other, the information obtained is sometimes ambiguous. PCA is a useful data reduction exercise that produces linear combinations of the multiple variables and reduces the original data matrix to a factor pattern with fewer components, which accounts for as much of the total variance as possible (CitationBuhr et al., 1992; CitationTsai, 2005; CitationDeng et al., 2011). In each of the extracted principal components (PCs), the loading coefficients between the PCs and the original variables represent the relative influence of each parameter in determining that component. Loading coefficients larger than or equal to 0.5 are considered to be influential for identifying the significant PCs. On one hand, the PCA results simplify the possible models describing the data set; on the other, the PCs with large loading coefficients of visibility also shed light on the factors that contribute to visibility impairment in pollution episodes. By performing varimax-rotated PCA analysis on time-matched data of meteorological factors and PM10 concentrations from 2000 to 2010, the major factors that correlated with visibility were identified. Based on the relationships explored by correlation and PCA analysis, multivariate linear regression was employed to derive the optimum models for visibility prediction.
Results and Discussion
General statistics of visibility, meteorological factors, and PM10 pollution in Chengdu and Chongqing
Table 2 presents the overall statistics for daily average visibility, temperature, relative humidity, wind speed, and pressure in Chengdu and Chongqing from 1973 to 2010. The daily visibility of Chengdu and Chongqing during the 38-yr period ranged from 0.2 to 36.2 and from 0.5 to 37.5 km, with averages of 8.1 and 6.2 km, respectively. The two cities had similar average RH values of around 80% (78.6% for Chengdu and 78.5% for Chongqing) and similar average wind speeds (1.3 m/s for Chengdu and 1.5 m/s for Chongqing). The average visibilities and wind speeds of Chengdu and Chongqing are much lower than those reported for other large cities worldwide (CitationTsai, 2005; CitationYang et al., 2011; CitationDeng et al., 2011), whereas the RH was much higher, indicating a rather poor visual situation and possible meteorological reasons for that situation. The mean temperature and pressure were 289.8 K and 1015.6 hPa for Chengdu and 291.3 K and 1014.0 hPa for Chongqing, respectively.
depicts the cumulative distribution functions of visibility in Chengdu and Chongqing. Most visual ranges in Chengdu and Chongqing were lower than 15 km (97.3% and 97.8%), with 73.3% and 88.1% lower than 10 km, respectively, further highlighting the poor visual situation. The frequency of visibilities lower than 5 km was 54.6% in Chongqing, contributing significantly to the low average visibility of 6.2 km. In Chengdu, 49.0% of visibility readings were in the range of 5–10 km.
The temporal trends of PM10 in Chengdu and Chongqing are shown in There were only 8.4% and 8.6% occurrences of “clean” air in Chengdu and Chongqing, respectively, with no primary pollutant reported. Furthermore, 13.9% and 26.2% of the days in Chengdu and Chongqing had APIs higher than 100, respectively, which do not meet the National Grade II Ambient Air Quality Standard (NAAQS-GII). In both cities, PM10 dominated the reported primary pollutants, with the occasional exception of SO2, and therefore the derived PM10 concentrations from the API were considered to be representative enough of local particulate pollution.
Figure 3. Percentage occurrence of days with PM10 as the primary pollutant and trends of PM10 concentrations in Chengdu and Chongqing.
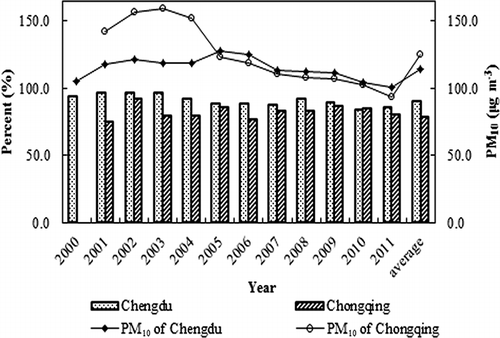
In Chengdu, the occurrence of PM10 as a primary pollutant decreased from a high of 96.7% in 2001, and stabilized at around 85% in the two years prior to this study. Concurrently, PM10 levels have also decreased from a high of 127.9 μg m−3 in 2005 to 100.4 μg m−3 in 2011. There were still 28.8% of days on which PM10 concentrations exceed the NAAQS-GII of 150 μg m−3 as a daily average. In Chongqing, the occurrence of PM10 as a primary pollutant varied between 74.5% and 91.8% before 2005 and then decreased to 79.9% in 2011. The PM10 concentrations decreased substantially from more than 150 μg m−3 before 2005 to 93.5 μg m−3 in 2011. On a national scale, PM10 concentrations in Chengdu and Chongqing, with 10-year averages of 114.6 and 124.9 μg m−3, respectively, are similar to levels in many cities of northern China that are influenced by sand and dust (about 96.6–144.7 μg m−3), but remain much higher than levels in other cities in southern China (about 49.9–74.9 μg m−3) (CitationQu et al., 2010). During some heavy pollution episodes, the PM10 concentrations can reach an incredible level of more than 300 μg m−3, of which more than 50% is PM2.5 and is an order of magnitude higher than in regions in the eastern United States (CitationYang et al., 2011).
Seasonal cycles of visibility and its relevant factors
Seasonal variations of visibility and its relevant factors in Chengdu and Chongqing were also investigated, and the results are shown in Visibility in both cities exhibited seasonal cycles, but the exact pattern varied. The highest monthly average visibilities in Chengdu and Chongqing were recorded in June (10.0 km) and July (9.5 km), respectively. In Chengdu, the seasonal average visibility was 9.2 km in spring (March–May), 9.6 km in summer (June–August), 7.8 km in autumn (September–November), and 5.9 km in winter (December–February). In Chongqing, the highest visibility was also recorded in summer (8.6 km) and an extremely low visibility of 3.9 km was observed in winter. and show that monthly average concentrations of PM10 were in the range of 89.8–165.9 μg m−3 and were inversely correlated with visibility. The concentration of PM10 was relatively higher from January to April and from November to December, whereas it was lower from May to October. The worst visibility in winter resulted from the combinations of high particulate concentrations and unfavorable meteorological conditions. Stagnant weather systems with low wind speeds, high pressure, strong inversions, and low mixing heights reduced the effective diffusion of pollutants and degraded visibility to a low level. The best visibility in summer occurred during the reverse situation, that is, an active convection of the mixing layer and abundant precipitation for particle scavenging (CitationQu et al., 2010).
Figure 4. Monthly variations of visibility, PM10, and meteorological factors in Chengdu (a, b) and Chongqing (c, d).
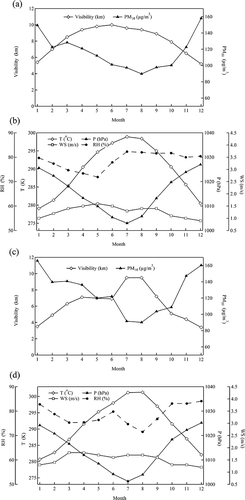
Both Chengdu and Chongqing are in a region of calm winds. Monthly average wind speeds are mostly within the range of 1–2 m/s, with the largest speed of 1.7 m/s in summer and the smallest value around 1.0 m/s in winter. Although the variations are weak, well positive correlations between visibility and wind speed are observed. and indicate that RH generates irregular and weak seasonal variations. High RH was observed throughout the year in both cities, and was attributable to frequent precipitation in summer and the high occurrence of fogs in autumn and winter. In Chengdu, except for the slightly lower RH in spring (73.3%), RH was very similar in the other three seasons (80.7%, 81.1%, and 79.1% in summer, autumn, and winter). Chongqing had higher RH of 81.0% and 81.9% in autumn and winter, respectively, and relatively low RH of 75.7% and 75.3% in spring and summer, respectively. It is expected that the high RH contributes significantly to the low visibility in Chengdu and Chongqing, especially in winter when extremely high particle levels occur. For example, in December in Chongqing with an average PM10 concentration of 160.3 μg m−3 and an average RH of 84.1%, the average visibility was degraded to 3.4 km. Both temperature and pressure changed significantly in the different months. The temperature is highest in July and August during summer and lowest in January during winter, whereas the opposite pattern is true for changes in pressure.
Long-term trends of visibility over Chengdu and Chongqing
The trends of the 50th cumulative percentile of visibility and ridit values are shown in The trends of the ridit plots closely follow the variations in visibility. The ridit plot for Chengdu shows an initial degrading period from 1973 to 1982. In 1983, the ridit value increased from 0.45 to 0.55 and an improved visibility was sustained through to 1996. From 1983 to 1996, the ridits were all >0.5, except for a value of 0.49 in 1985, indicating a better visibility compared to the entire period. After 1996, there was another degradation period in Chengdu, with a minor improvement in recent years. In contrast, a persistent decreasing trend has been observed in Chongqing with an unexpected high value in 2001. The best visibility period in Chengdu occurred during 1980s and 1990s, while best visibility of Chongqing appeared in 1970s and early 1980s, when most of the ridit values are larger than 0.5. Significant reductions of very good visibility (>19 km) days and increases of low visibility (<10 km) days were observed in Chengdu and Chongqing (). During the 1970s, the two sites had about 10 days per year with visibility higher than 19 km. After 1995, visibility higher than 19 km nearly ceased in these two rapidly developing megacities, whereas low visibility events occurred more frequently, reaching around 280 and 210 days per year, respectively.
Figure 5. Trends of the 50th cumulative percentile of visibility and ridit values in (a) Chengdu and (b) Chongqing.
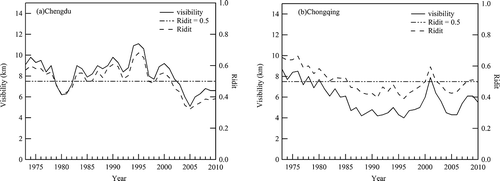
Figure 6. Days with visibility >19 km per year and days with visibility <10 km per year in Chengdu and Chongqing.
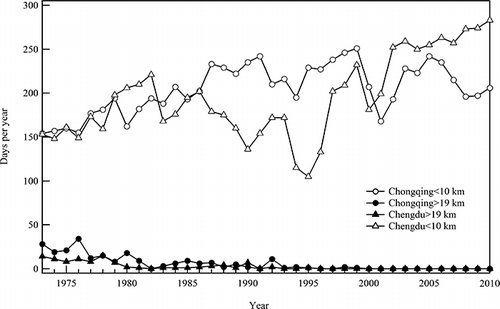
Generally speaking, despite of the fluctuations, visibility levels in recent years were lower than those in the 1970s. The deterioration of visibility and the increase in haze days in recent years have been more prevalent when compared with the 1960s (). In the 1960s, China was a largely agricultural country with no industry and lower population. This could be considered to be a period of contrasting visibility with less anthropogenic pollution. During the 2006 to 2010 period, not only did the 5-year average visibility of Chengdu (6.2 km) reduce to half of that in the 1961 to 1964 (12.3 km), but the number of haze days also doubled (from 104 to 270 days/yr) (). A similar situation also happened in Chongqing. The decreasing visibility was mostly attributable to an elevation in the aerosol optical depth (CitationChameides et al., 1999), given the relatively stable climatology within the period (Supplementary Figure). The large-scale economic growth in China during the 1970s and 1980s led directly to the visibility degradation in the two cities, and SO2 and PM10 were the most representative pollutants in that period. From the late 1980s, a regional economy gradually formed in the Sichuan Basin, resulting in intensive pollutant emissions in Chengdu and Chongqing, as well as in many of the surrounding medium-sized cities. At the same time, more stringent measures aimed at controlling acid rain and SO2 were implemented, including reductions on coal consumption in urban area and requirements for flue-gas desulfurization at power plants. This explains why such variation and a temporary visibility recovery were observed in the last two decades. The improvement in Chengdu during the 1980s and 1990s also seemed to be more significant than in Chongqing, which may probably result from the more developed heavy industry and persistent high sulfur (>3.5%) coal consumed in Chongqing.
Figure 7. (a) Comparison of visibility between 1960s and 2000s in Chengdu and Chongqing. (b) Comparison of haze days between 1960s and 2000s in Chengdu and Chongqing.
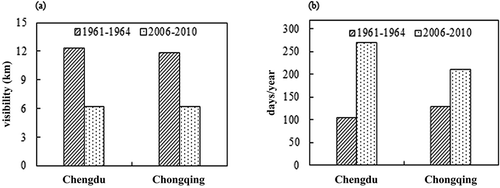
During the 2000s, the air pollution situation aggravated in both Chengdu and Chongqing, because of unprecedented levels of economic growth, boom of vehicular population, and the development of large-scale industries and services (). While both cities are still heavily dependent upon coal for energy and the pollution from coal combustion is not totally solved, complex air pollution characterized by increased atmospheric oxidation capacity and enhanced formation of secondary particles has also become more common in the Sichuan Basin. Visibility has been preserved at a low level and little improvement has been observed, despite the reduction in PM10 concentrations in Chengdu and Chongqing during 2000s. The major light extinction components, including sulfate, nitrate, and OC, are mostly concentrated in the PM2.5 fraction. Thus, visibility degradation is more directly related to levels of fine atmospheric particles than to the coarse ones. In recent years, large increases in vehicle population in Chengdu and Chongqing have resulted in the sharp increase of NOx and VOCs, which lead to the formation of ozone and the oxidation of secondary fine aerosols. It is possible that the reduction in PM10 concentrations may have been offset by the continuous increase in PM2.5/PM10 ratios and possible increases of PM2.5 concentrations. Another possible reason for the continued degradation of visibility lies in the nonlinear relationship between aerosol concentrations and visibility (CitationSong et al., 2003; CitationDeng et al., 2008). When PM concentrations are high, the improvement or worsening of visibility becomes insensitive to a minor reduction or increase in PM levels. More comprehensive sensitivity analysis is needed for targeted a targeted visibility improvement in each city.
Relationship between visibility and its affecting factors
summarizes the correlation matrix of visibility, various meteorological factors, time-matched API values, and PM10 from 2000 to 2010. Correlation coefficients that are significant at the 99% confidence level are shown in boldface. In both Chengdu and Chongqing, all the parameters studied are significantly related to visibility, highlighting the complex physical and chemical mechanisms that affect the visual range in the atmosphere (CitationWatson, 2002). In Chengdu, visibility was only weakly correlated with all the parameters studied, as the absolute values of coefficients only varied between 0.344 and 0.433. In contrast, visibility in Chongqing had the strongest correlation with RH (r = –0.608). Visibility is inversely proportional to light extinction, 90% of which caused by atmospheric particles (CitationLiu et al., 2006). However, weak negative correlations were observed between PM10 concentrations and visibility in both cities. The poor relationship, which may result from changes in the size distribution of the fine and coarse modes or the relative mix of the fine and coarse modes in PM10 measurements and the associated effects of RH on hygroscopic components (CitationDeng et al., 2011; CitationMui et al., 2009), also suggests that a reduction in the coarse fraction of PM10 will not improve the visibility impairment in Chengdu and Chongqing.
Table 2b. Summary of visibility, meteorological factors, API, and PM10 pollution in Chongqing
Visibility was positively related to wind speed and temperature, but negatively related to RH and pressure. Both and demonstrate that there is a good reverse correlation between visibility and RH, with episodes with visibility lower than 5 km mostly occurring when the RH >60%. The stronger correlation between visibility and RH in Chongqing indicates that there are more hygroscopic components such as sulfate and nitrate in the fine aerosols of that location.
Figure 9. Scatter plots of visibility vs (a) RH and (b) wind speed in Chongqing (color figure available online).
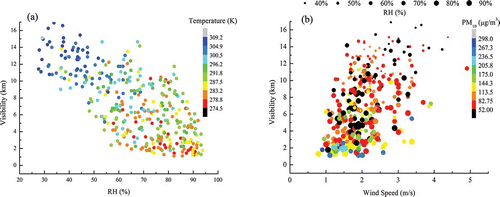
Figure 10. Scatter plots of visibility vs (a) RH and (b) wind speed in Chengdu (color figure available online).
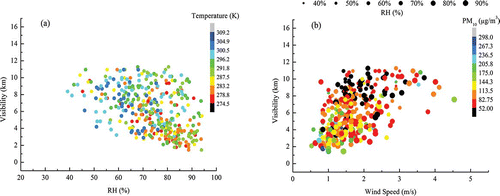
In most cases, a larger wind speed suggests more efficient diffusion conditions and dilutes PM10 and other pollutants to low concentrations. High wind speeds are usually associated with improved visual situation. When the wind speed was larger than 3 m/s, visibility is mostly above 10 km, determined by RH and PM10 concentrations ( and ). Nevertheless, winds from certain directions may enhance regional air pollution transport and increase visibility deterioration, and high wind speeds rarely appear in Chongqing. Hence, correlation between visibility and wind speed is rather weak (r = 0.396 in Chengdu and r = 0.389 in Chongqing).
Many previous studies have concluded that the correlations of visibility with pressure and temperature are weak and sometimes ambiguous (CitationDeng et al., 2011). In our study, the results of the correlation analysis suggest that visibility increases with temperature and decreases with increasing pressure in Chengdu and Chongqing. This is possibly related to the dominant weather systems of southwest China in the different seasons. In summer, warm fronts with high temperature, low pressure and much precipitation favors high visibility, whereas in winter the stable air under cold, high-pressure systems often leads to extremely low visibility. Both temperature and pressure are important indicators of the weather system and are strongly correlated with each other (r = –0.876 and r = –0.878 in Chengdu and Chongqing, respectively).
Principal component analysis
As reflected from the preceding section, visibility is directly or indirectly affected by some interactional factors and the interactions are extremely complex, and it is important to further identify the major factors that control the visibility variations using PCA. Results of the varimax-rotated PCA are presented in . In Chengdu, four PCs that explain more than 80% of the total variance were extracted. The PC1 for Chengdu, which explains the maximum variance of 38.9% and had a strong loading of 0.962 from PM10, represents low visibility under high PM concentrations (). The PC2 with 22.2% explained variance had large loading from RH, indicating the effect of aerosol hygroscopicity on visibility. The relative loadings of visibility in PC1 and PC2 were 0.558 and 0.548, respectively, indicating that low visibility primarily resulted from humid air and high PM10 concentrations. The remaining variance was mainly divided among PC3 (17.6%) and PC4 (14.8%), with major components of wind speed (PC3) and temperature (PC4).
Table 3a. Correlation matrix of visibility (V), temperature (T), wind speed (WS), relative humidity (RH), pressure (P), PM10, and API (2000–2010) in Chengdu
Different from Chengdu, only three PCs were extracted in Chongqing. PC1, accounting for 47.2% of the explained variance, had large loadings from temperature, RH, and pressure. This component mainly reflected the visibility-degrading effect of stable weather system with low temperature, high pressure, and high humidity on visibility. In PC2 with 17.5% of the explained variance, visibility had the largest loading of 0.751 among all the PCs, along with wind speed and RH, again verifying the importance of meteorological factors in influencing visibility. From , it can be seen that weather with an RH higher than 70% and a wind speed lower than 2 m/s is common in Chongqing, and under these condition low visibility will occur even if PM10 is lower than 50 μg m−3. PC3 has a strong positive loading from PM10 (0.946) but a very weak loading of visibility (0.207), suggesting that coarse particulate pollution is not the sole cause of low visibility in Chongqing.
Models for visibility prediction in Chengdu and Chongqing
To predict visibility under different circumstances, empirical models were developed using multivariate regression methods. The models for Chengdu and Chongqing during the period of 2000 to 2010, which relate visibility to meteorological factors and PM10 pollution, are shown in , where Vis is visibility in km, T is temperature in ºC, WS is wind speed in m s−1, and RH is relative humidity (%). In both models, the natural logarithm of the PM10 concentration (ln[PM10]) in μg m−3 is used due to the nonlinear relationship between aerosol and visibility (CitationMui et al., 2009; CitationSong et al., 2003) .
Table 3b. Correlation matrix of visibility (V), temperature (T), wind speed (WS), relative humidity (RH), pressure (P), PM10, and API (2000–2010) in Chongqing
Table 4a. Factor loadings for visibility, meteorological factors, and PM10 pollutants in Chengdu
Table 4b. Factor loadings for visibility, meteorological factors, and PM10 pollutants in Chongqing
Table 5. Empirical models of visibility in Chengdu and Chongqing
In the model for Chengdu (), the pressure parameter was not included because of missing data after 2004. Wind speed has a greater influence on visibility than temperature and relative humidity do. In both cities, visibility has the largest coefficient in the model and seems to be the dominant factor for visibility, since it is the only proxy we have for anthropogenic pollution. However, a 50% decrease in PM10 concentrations will only improve visibility by 1.7 and 1.5 km in Chengdu and Chongqing, respectively, further indicating the weak correlation between PM10 and visibility.
To verify the practicability of each model, the observed visibility during January, April, July, and October in 2011 were compared with the calculated visibility from each model (). The correlation coefficients between the observed and calculated values were 0.777 and 0.871 for Chengdu and Chongqing, respectively, and the correlations were significant under a 99% confidence level using a t-test. Data for the relevant pollutants of visibility were not regularly monitored or easily accessed in this study. For simplification, the hygroscopic growth of inorganics was also ignored in the model. Therefore, the statistical models, including only PM10 and meteorological factors, do not feature as perfect calculations for visibility prediction. Moreover, the model prediction may only be useful over short time periods, with relatively stable emission sources and insignificant changes in PM composition.
Summary
To develop a better understanding of the low visibility situation in Chengdu and Chongqing in southwest China, long-term trends of visibility and various meteorological parameters from 1973 to 2010, as well as their interactions with PM10 concentrations, were analyzed using several statistical methods for trend and correlation analysis. During the whole study period, visibility in Chengdu and Chongqing remained at low levels with averages of 8.2 ± 3.9 and 6.2 ± 4.3 km, respectively. Seasonal variations, characterized by high visibility in summer and low visibility in winter, were clearly due to the combined impact of meteorological conditions and air pollution. RH generated irregular and weak variations, and high RH (average around 80%) was observed throughout the year in both cities. The current visibility levels in Chengdu and Chongqing were significantly degraded when compared with those in the 1960s and 1970s, and visibility fluctuations during the 1980s and 1990s were due to the impacts of stringent emission control measure on levels of air pollution. During the 2000s, PM10 levels in both cities consistently fell to around 100 μg m−3, but visibility levels show no sign of improvement. It is expected that the rapid economic growth and drastic increase in vehicular population in last decade have exacerbated the complex air pollution and resulted in an increase in the secondary components of PM2.5.
The results of the correlation analysis suggest that complex chemical and synoptic mechanisms are involved in visual range formation, as visibility is significantly correlated with all the meteorological and air quality factors in both Chengdu and Chongqing. In particular a strong relationship between visibility and RH was observed in Chongqing, indicating more hygroscopic components in the particles in that location. PCA results further confirmed that the low visibility in Chengdu primarily resulted from high RH and PM10 concentrations, while in Chongqing the degraded visibility was largely explained by the high RH and pressure and low wind speed under stable weather systems. Empirical models for visibility prediction demonstrated that visibility improvements were most sensitive to reductions in PM10 concentrations. One limitation of this study is that only daily visibility data and PM10 concentrations were used for analysis. In order to obtain a better understanding of the factors influencing visibility, more data about specific pollutants such as the components of PM2.5, SO2, and NOx and more information on emission sources are required. The regression coefficients between observed and calculated visibility will be significantly improved when more data for PM2.5 and other gaseous pollutants are included.
Our results not only highlight the importance of meteorological factors on visibility reductions in Chengdu and Chongqing, but also confirm the essential role of air pollution in degrading visibility given the relatively stable climatology within the study period. The results are important for this special region with hilly topography, high RH, and ineffective dilution conditions. It is estimated that rapid economic development in the two cities will continue for several decades as the demand for urbanization and higher living standards intensifies. However, until now, no effective measures aimed at controlling PM2.5 or improving visibility have been implemented. The findings of this study may also be used to promote local efforts in visibility improvement.
Supplementary Material
Download MS Word (377.6 KB)Acknowledgment
The authors appreciate the financial support from the Major State Basic Research Development Program of China (973 Program) (number 2010CB955608), the Research of Characteristics and Controlling Measures of Atmospheric Haze in Cheng-Yu Area (number 201009001), and the Air Pollution Index provided by the Ministry of Environmental Protection in China. The authors also thank the Energy Foundation (U.S.) for the scholarship sponsored to this study and the National Climatic Data Center (U.S.) for the visibility data.
References
- Buhr , M.P. , Trainer , M. , Parrish , D.D. , Sievers , R.E. and Fahsenfeld , F.C. 1992 . Assessment of pollutant emission inventories by principal component analysis of ambient air measurements . Geophys. Res. Lett. , 19 : 1009 – 12 . doi: 10.1029/92GL01020
- Chameides , W. L , Yu , H. , Liu , S.C. , Bergin , M. , Zhou , X. , Mearns , L. , Wang , G. , Kiang , C.S. , Saylor , R.D. , Luo , C. , Huang , Y. , Steiner , A. and Giorgi , F. 1999 . Case study of the effects of atmospheric aerosols and regional haze on agriculture: An opportunity to enhance crop yields in China through emission controls? . Proc. Natl. Acad. Sci. USA , 96 : 13626 – 33 . doi: 10.1073/pnas.96.24.13626
- Che , H.Z. , Zhang , X.Y. , Li , Y. , Zhou , Z.J. and Qu , J.J. 2007 . Horizontal visibility trends in China 1981–2005 . Geophys. Res. Lett. , 34 : L24706 – 10 . doi: 10.1029/2007GL031450
- Che , H.Z. , Zhang , X.Y. , Li , Y. , Zhou , Z.J. , Qu , J.J. and Hao , X.J. 2009 . Haze trends over the capital cities in 31 provinces in China, 1981–2005 . Theor. Appl. Climatol. , 97 : 235 – 42 . doi: 10.1007/s00704-008-0059-8
- Chen , Y. and Xie , S.D. 2012 . Temporal and spatial visibility trends in the Sichuan Basin, China, 1973 to 2010 . Atmos. Res. , 112 : 25 – 34 . doi: 10.1016/j.atmosres.2012.04.009
- China Energy Statistical Yearbook , 1986 – 2010 . Beijing : China Statistical Press .
- Craig , C.D. and Faulkenberry , G.D. 1979 . The application of ridit analysis to detect trends in visibility . Atmos. Environ. , 13 : 1617 – 22 . doi: 10.1016/0004-6981(79)90319-6
- Data Center of Ministry of Environmental Protection, China. 2012. Air Pollution Index http://datacenter.mep.gov.cn/main/index.action?indexType= air (http://datacenter.mep.gov.cn/main/index.action?indexType= air) (Accessed: April 2012 ).
- Deng , X.J. , Tie , X.X. , Wu , D. , Zhou , X.J. , Bi , X.Y. , Tan , H.B. , Li , F. and Jiang , C.L. 2008 . Long-term trend of visibility and its characterizations in the Pearl River Delta (PRD) region, China . Atmos. Environ. , 42 : 1424 – 35 . doi: 10.1016/j.atmosenv.2007.11.025
- Deng , J.J. , Wang , T.J. , Jiang , Z.Q. , Xie , M. , Zhang , R.J. , Huang , X.X. and Zhu , J.L. 2011 . Characterization of visibility and its affecting factors over Nanjing, China . Atmos. Res. , 101 : 681 – 91 . doi: 10.1016/j.atmosres.2011.04.016
- Doyle , M. and Dorling , S. 2002 . Visibility trends in the UK 1950–1997 . Atmos. Environ. , 36 : 3161 – 72 . doi: 10.1016/S1352-2310(02)00248-0
- Gao , L.N. , Jia , G.S. , Zhang , R.J. , Che , H.C. , Fu , C.B. , Wang , T.J. , Zhang , M.G. , Jiang , H. and Yan , P. 2011 . Visual range trends in the Yangtze River Delta region of China, 1981–2005 . J. Air Waste Manage. Assoc. , 61 : 843 – 49 . doi: 10.3155/1047–3289.61.8.843
- Gomez , B. and Smith , C.G. 1987 . Visibility at Oxford 1926–1985 . Weather , 42 : 98 – 106 . doi: 10.1002/j.1477-8696.1987.tb06937.x
- Horvath , H. 1981 . Atmospheric visibility . Atmos. Environ. , 15 : 1785 – 96 . doi: 10.1016/0004-6981(81)90214-6
- Huang , J. , Wu , D. , Huang , M.H. , Li , F. , Bi , X.Y. , Tan , H.B. and Deng , X.J. 2008 . Visibility variations in the Pearl River Delta of China during the period of 1954–2004 . J. Appl. Meteorol. Sci. , 19 : 61 – 70 . in Chinese with English abstract
- Lee , D.O. 1990 . The influence of wind direction, circulation type and air pollution emissions on summer visibility trends in southern England . Atmos. Environ , 24A : 195 – 201 . doi: 10.1016/0960-1686(90)90456-W
- Linsley , R.K. , Kohler , M.A. and Paulhus , J.L.H. 1988 . Hydrology for Engineers , New York , NY : McGraw-Hill .
- Liu , X.G. , Zhang , Y.H. , Zeng , L.M. , Yang , H. and Liu , Y. 2006 . Research on the influential factor of visibility reduction in Guangzhou . Climat. Environ. Res. , 11 : 733 – 38 . in Chinese with English abstract
- Malm , W.C. and Day , D.E. 2001 . Estimates of aerosol species scattering characteristics as a function of relative humidity . Atmos. Environ. , 35 : 2845 – 60 . doi: 10.1016/S1352-2310(01)00077-2
- Malm , W.C. , Sisler , J.F. , Huffman , D. , Eldred , R.A. and Cahill , T.A. 1994 . Spatial and seasonal trends in particle concentration and optical extinction in the United States . J. Geophys. Res. , 99 : 1347 – 70 . doi: 10.1029/93JD02916
- Mui , K.W. , Wong , L.T. and Chung , L.Y. 2009 . Mathematical models for accurate prediction of atmospheric visibility with particular reference to the seasonal and environmental patterns in Hong Kong . Environ. Monit. Assess. , 158 : 333 – 41 . doi: 10.1007/s10661-008-0587-9
- National Climatic Data Center (U.S.). 2013. Climate data online http://www7.ncdc.noaa.gov/CDO/cdoselect.cmd?datasetabbv=GSOD&countryabbv=CH &georegionabbv=&resolution=40 (http://www7.ncdc.noaa.gov/CDO/cdoselect.cmd?datasetabbv=GSOD&countryabbv=CH &georegionabbv=&resolution=40) (Accessed: January 5, 2013 ).
- Qian , Y. and Giorgi , F. 2000 . Regional climatic effects of anthropogenic aerosols? The case of southwestern China . Geophys. Res. Lett. , 27 : 3521 – 24 . doi: 10.1046/j.1435-6935.2003.00070.x
- Qu , W.J. , Arimoto , R. , Zhang , X.Y. , Zhao , C.H. , Wang , Y.Q. , Sheng , L.F. and Fu , G. 2010 . Spatial distribution and interannual variation of surface PM10 concentrations over eighty-six Chinese cities . Atmos. Chem. Phys. , 10 : 5641 – 62 . doi: 10.5194/acp-10-5641-2010
- Schichtel , B.A. , Husar , R.B. , Falke , S.R. and Wilson , W.E. 2001 . Haze trends over the United States, 1980–1995 . Atmos. Environ. , 35 : 5205 – 10 . doi: 10.1016/S1352-2310(01)00317-X
- Sloane , C.S. 1982a . Visibility trends—I. Methods of analysis . Atmos. Environ. , 16 : 41 – 51 . doi: 10.1016/0004-6981(82)90312-2
- Sloane , C.S. 1982b . Visibility trends—II. Mideastern United States 1948–1978 . Atmos. Environ. , 16 : 2309 – 21 . doi: 10.1016/0004-6981(82)90117-2
- Sloane , C.S. 1984 . Meteorological adjusted air quality trends: visibility . Atmos. Environ. , 18 : 1217 – 29 . doi: 10.1016/0004-6981(84)90153-7
- Song , Y. , Tang , X.Y. , Fang , C. , Zhang , Y.H. , Hu , M. , Zeng , L.M. , Li , C.C. , Mao , J.T. and Bergin , M. 2003 . Relationship between the visibility degradation and particle pollution in Beijing . Acta Sci. Circums. , 23 : 468 – 71 . in Chinese with English abstract)
- Tsai , Y.I. 2005 . Atmospheric visibility trends in an urban area in Taiwan, 1961–2003 . Atmos. Environ. , 39 : 5555 – 67 . doi: 10.1016/j.atmosenv.2005.06.012
- Wang , K.C. , Dickinson , R.E. and Liang , S.L. 2009 . Clear sky visibility has decreased over land globally from 1973 to 2007 . Science , 323 : 1467 – 70 . doi: 10.1126/science.1167549
- Watson , J.G. 2002 . Visibility: Science and regulation . J. Air Waste Manage. Assoc. , 52 : 628 – 713 . doi: 10.1080/10473289.2002.10470813
- Wu , D. , Wu , X.J. , Li , F. , Tan , H.B. , Chen , J. , Cao , Z.Q. , Sun , X. , Chen , H.H. and Li , H.Y. 2010 . Temporal and spatial variation of haze during 1951–2005 in Chinese mainland . Acta Meteorol. Sin. , 68 : 680 – 88 . in Chinese with English abstract)
- Yang , F. , Tan , J. , Zhao , Q. , Du , Z. , He , K. , Ma , Y. , Duan , F. , Chen , G. and Zhao , Q. 2011 . Characteristics of PM2.5 speciation in representative megacities and across China . Atmos. Chem. Phys. , 11 : 5207 – 19 . doi: 10.5194/acp-11-5207-2011
- Zhao , P.S. , Zhang , X.L. , Xu , X.F. and Zhao , X.J. 2011 . Long-term visibility trends and characteristics in the region of Beijing, Tianjin, and Hebei China . Atmos. Res. , 101 : 711 – 18 . doi: 10.1016/j.atmosres.2011.04.019