Abstract
This study presents an evaluation of summertime ozone concentrations over North America (NA) and Europe (EU) using the database generated from Phase 1 of the Air Quality Model Evaluation International Initiative (AQMEII). The analysis focuses on identifying temporal and spatial features that can be used to stratify operational model evaluation metrics and to test the extent to which the various modeling systems can replicate the features seen in the observations. Using a synoptic map typing approach, it is demonstrated that model performance varies with meteorological conditions associated with specific synoptic-scale flow patterns over both eastern NA and EU. For example, the root mean square error of simulated daily maximum 8-hr ozone was twice as high when cloud fractions were high compared with when cloud fractions were low over eastern NA. Furthermore, results show that over both NA and EU the regional models participating in AQMEII were able to better reproduce the observed variance in ambient ozone levels than the global model used to specify chemical boundary conditions, although the variance simulated by almost all regional models is still less that the observed variance on all spatiotemporal scales. In addition, all modeling systems showed poor correlations with observed fluctuations on the intraday time scale over both NA and EU. Furthermore, a methodology is introduced to distinguish between locally influenced and regionally representative sites for the purpose of model evaluation. Results reveal that all models have worse model performance at locally influenced sites. Overall, the analyses presented in this paper show how observed temporal and spatial information can be used to stratify operational model performance statistics and to test the modeling systems’ ability to replicate observed temporal and spatial features, especially at scales the modeling systems are designed to capture.
Implications:
The analyses presented in this paper demonstrate how observed temporal and spatial information can be used to stratify operational model performance and to test the modeling systems’ ability to replicate observed temporal and spatial features. Decisions for the improvement of regional air quality models should be based on the information derived from only regionally representative sites.
Introduction
In both North America and Europe, regional-scale air quality modeling systems are being used to help guide emission control policies aimed at meeting and maintaining the relevant air quality standards and to address issues related to the transport of air pollution across state and national boundaries. However, despite the fact that the science issues facing the regional-scale modeling communities on both continents are similar, there has been only limited collaboration on defining and applying a comprehensive approach for model evaluation aimed at critically assessing the strengths and limitations of the models being used in a policy setting. Recognizing this gap, scientists from both continents launched the Air Quality Model Evaluation International Initiative (AQMEII) as a long-term forum to monitor the state-of-the-science in regional-scale air quality models and model evaluation methodologies (Rao et al., Citation2011). The objective of AQMEII is to help build a coordinated international effort on regional air quality model evaluation methodologies using the framework defined in Dennis et al. (Citation2010). This framework identifies operational, diagnostic, dynamic, and probabilistic types of model evaluation. As described in Dennis et al. (Citation2010), operational evaluation is aimed at determining whether model estimates are in agreement with the observations in an overall sense; diagnostic evaluation focuses on process-oriented analyses, dynamic evaluation assesses the ability of the air quality model to simulate changes in air quality stemming from changes in source emissions and/or meteorology, and probabilistic evaluation attempts to assess the confidence that can be placed in model predictions. In its first phase, AQMEII organized annual model simulations that were conducted for the year 2006 over both continents using specified input data sets. Outputs from these simulations were used to conduct a number of operational and probabilistic model evaluation analyses (Galmarini et al., Citation2012b).
Recognizing the uniqueness of the data set generated in AQMEII Phase 1, Galmarini and Rao (Citation2011) called for the community to utilize these data to develop new approaches for performing model evaluation. This study follows this call and aims at introducing and applying techniques that can highlight common aspects as well as differences between the performances of different modeling systems. Specifically, the proposed techniques aim at identifying temporal and spatial subsets of data that can be used to discern the associations between meteorological conditions and air quality model performance and to better quantify model performance by relying on the observational data that are representative of the scales that air quality models were designed to capture. Given the enormity of the AQMEII Phase 1 database, any individual analysis is necessarily limited in scope, and in this paper, we focus on summertime ozone concentrations to illustrate and apply the proposed model evaluation approaches.
Database
This study utilized observations and model predictions of daily maximum 8-hr average (DM8A) ozone concentrations for May 1 to September 30, 2006, over North America (NA) and Europe (EU). Both observations and corresponding model predictions were extracted from the AQMEII database of the ENSEMBLE system (Galmarini et al., Citation2004a, Citation2004b, Citation2012a). lists the modeling systems utilized in this study. As part of the AQMEII activity, the modeling systems listed in have been extensively evaluated against available observations, both in terms of single model performance (Appel et al., Citation2012; Brandt et al., Citation2012; Ferreira et al., Citation2012; Forkel et al., Citation2012; Gilliam et al., Citation2012; Nopmongcol et al., Citation2012; Pirovano et al., Citation2012; Sartelet et al., Citation2012) and in terms of multimodel ensemble performance (Solazzo et al., Citation2012a, Citation2012b; Vautard et al., Citation2012). Most modeling systems used the common set of emissions and boundary condition prepared for AQMEII as described in Pouliot et al. (Citation2012) and Schere et al. (Citation2012), respectively. The horizontal grid spacing of the individual simulations ranged from 12 to 50 km.
Table 1. List of modeling systems analyzed in this study
Rather than performing diagnostic model evaluation and identifying causes behind model-to-model differences, the goal of this paper is to introduce several model evaluation approaches aimed at establishing which space and time scales the models participating in AQMEII Phase 1 are better at simulating and for which ones they exhibit lower skill. Thus, all model results are presented anonymously in all figures and tables. In these figures and tables, “NA1” through “NA8” refer to the eight NA models listed in , and “EU1” to “EU11” refer to the 11 EU models. The ordering of the models is consistent between the tables and figures but is different from the order in which the individual models are identified in to preserve anonymity.
Methods and Results
Use of map typing to stratify operational model evaluation
To investigate the associations between atmospheric circulation patterns (i.e., prevailing meteorological conditions) and DM8A ozone concentrations, we categorized atmospheric circulation patterns through a map typing approach based on daily gridded mean sea level pressure (MSLP). For NA, we used daily MSLP fields obtained from the National Center for Atmospheric Research/Department of Energy (NCAR/DOE) Reanalysis 2 (Kanamitsu et al., Citation2002). For EU, we used daily MSLP fields obtained from the European Centre for Medium-Range Weather Forecasts (ECMWF) interim ERA reanalysis data set (Dee et al., Citation2011). The map typing approach used here was introduced by Lund (Citation1963) and Kirchhofer (Citation1973), and details on its implementation can be found in Yarnal (Citation1993), McKendry et al. (Citation1995), and Hegarty et al. (Citation2007). This approach has been used in several previous studies to represent ozone climatology in the northeastern United States (Hegarty et al., Citation2007; Hogrefe et al., Citation2004). As described in Yarnal (Citation1993), McKendry et al. (Citation1995), and Hegarty et al. (Citation2007), the amount of intrapattern variability as well as the total number of identified patterns are controlled by two parameters, i.e., the critical correlation coefficient and the minimum group size. After performing several initial trials aimed at maintaining pattern separation as well as keeping the number of patterns to a manageable size, in the current study the critical correlation coefficient was set to 0.5 for all map typing domains and the minimum group size was set to 4 days for NA and 7 days for EU. Using a minimum group size of 4 days for the EU domain would have resulted in several additional relatively infrequent patterns that did not add value to the current analysis. For the NA domain, map typing was performed separately for the western part (west of −100°W) and the eastern part (east of −100°W) of the domain due to the differences in prevailing meteorological conditions; separating the EU domain was not deemed necessary for map typing purposes. Application of the map typing methodology using these parameters resulted in the selection of six representative patterns for the eastern NA domain, four for the western NA domain, and six for the EU domain. Figures S1–S3 in Supplemental Materials show maps of the representative MSLP patterns along with their specific dates for each of the analysis domains. presents the frequency of occurrence of each of the patterns as well as the percentage of unassigned days for all three map typing domains.
Table 2. Frequency of occurrence of the different synoptic patterns for the E. NA, W. NA, and EU map typing domains
The representative circulation patterns identified through the MSLP map typing procedure represent specific synoptic-scale situations affecting both meteorological and air quality variables. To elucidate these conditions, we calculated composite maps of temperature, winds, cloudiness, short-wave radiation, and ozone concentrations by averaging these variables over all days that were associated with a given pattern. Meteorological fields were obtained from the reanalysis data sets described above. To eliminate the confounding influence of latitudinal gradients on the temperature and short-wave radiation, the seasonal mean values for these variables at each grid point were subtracted from the daily values to create daily anomalies prior to calculating the composite maps. The resulting composite maps for each pattern for each of the three analysis domain are shown in Figures S4–S19 in Supplemental Materials. Although it is beyond the scope of this study to provide a detailed discussion of the meteorological features of each of these patterns, these maps are provided for reference purposes, and the subsequent discussions of model performance under different synoptic regimes will refer to some of the features depicted in these composite maps.
To quantify the dependence of model performance on synoptic conditions, we calculated the mean bias error (MBE) and root mean square error (RMSE) of the DM8A ozone concentrations for each model for each synoptic regime for the following eight analysis subregions in the modeling domain: Northeast (NE), Midwest (MW), Southeast (SE), Northwest (NW), California (CA), Southwest (SW), northern Europe (N. EU), southern Europe (S. EU). The first three of these analysis subregions are located in the eastern NA map typing domain, the next three in the western NA map typing domain, and the last two in the Europe map typing domain. contains a definition of each of these analysis domains. Segregating the analysis within each map typing region into smaller areas is necessary because a given synoptic regime influences different parts of the map typing domain differently. For each analysis subregion, we also computed “all pattern” MBE and RMSE values by combining data from all days assigned to any pattern.
Table 3. Definition of analysis regions for stratifying operational model performance based on synoptic patterns
–d present results of this analysis for the NE and N. EU domains. Within each analysis domain, model MBE and RMSE were calculated at each site for the May–September analysis time period; the box-and-whisker diagrams in -d depict the distribution of these metrics across all sites within these analysis domains. For the NE region, –b show that all eight model simulations tend to have the lowest absolute MBE and lowest RMSE for pattern number 6, whereas they tend to have larger MBE (mostly negative) and larger RMSE for synoptic patterns 1, 4, and 5. Consultation of the composite maps in Supplemental Materials (Figures S4–S9) indicates that pattern 6 is characterized by a high-pressure system centered over the midwestern United States. Winds over the NE region (as defined in ) for this pressure pattern were northerly except northern New England, Quebec, Nova Scotia, and New Brunswick where westerly winds prevailed. In addition, over the NE region this pressure pattern is associated with slightly above normal temperatures, clear skies, and below-average DM8A ozone concentrations. Patterns 4 and 5 are characterized by a high-pressure system situated off the New England coast and low pressure to the west and south. The center of the low-pressure system is situated over Florida and Georgia for pattern 4 and over Michigan and Ontario for pattern 5. Over the NE region, both patterns are associated with easterly to southerly winds, high cloud fractions, below-average temperature and short-wave radiation, and below-average DM8A ozone concentrations. Pattern 1 is characterized by a high-pressure system centered over the Bahamas and a low-pressure system centered over Ontario. Over the NE analysis domain, this pattern is associated with above-average temperatures, southwesterly winds, relatively low cloud fractions, and above-average DM8A ozone concentrations.
Figure 1. Box-and-whisker plots of RMSE and MBE for NE and N. EU domains for each model and each pattern. The all-pattern results are also shown for each model. Boxes-and-whiskers represent the distribution of RMSE and MBE across the monitoring sites in the analysis domains. On either side of the box, the whiskers extend to the most extreme data point or 1.5 times the interquartile range (i.e., the difference between the 25th and 75th percentiles), whichever is less.
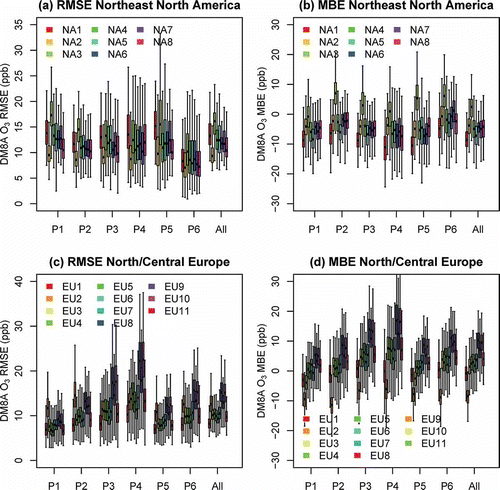
For the N. EU region, –d show that most of the 11 model simulations tend to have the smallest absolute MBE and RMSE for patterns 1 and 5 and the largest absolute MBE (mostly positive) and RMSE for pattern 4. Consultation of the composite maps in Supplemental Materials (Figures S14–S19) shows that pattern 1 is characterized by a high-pressure system centered over western France and northern Spain and a low-pressure system centered over Finland and Sweden, whereas pattern 5 is characterized by a high-pressure system centered west of Ireland and a low-pressure system centered over western Russia. For the N. EU analysis domain, these patterns 1 and 5 are associated with mostly westerly and northerly winds, below-average temperatures and short-wave radiation, high cloud cover, and below-average DM8A ozone concentrations. Pattern 4 is characterized by a low-pressure pattern located northwest of Ireland and high pressure over most of Europe. For the N. EU analysis domain, this pattern is associated with mostly easterly and southerly winds, above-average temperatures and short-wave radiation, low cloud cover, and above-average DM8A ozone concentrations.
Because the variability in the illustrative examples depicted in –d is dominated by between-model differences for any given pattern, it can be difficult to discern the between-pattern differences for any given model. Therefore, we performed analysis of variance (ANOVA) to determine whether, for any given model, the differences in mean MBE/RMSE between different patterns were statistically significant. The results of this analysis show that for any given model, differences between the pattern specific MBE/RMSE and the all-pattern MBE/RMSE were statistically significant at the 95% level for at least one pattern in both the NE and N. EU analysis domains. For most models, especially over the N. EU analysis domain, differences between the metrics for a given pattern and the all-pattern metrics were significant at the 95% level for at least three patterns and often five or all six patterns. In other words, for any given model, the use of the synoptic classification approach can help to distinguish significant differences in model performance.
The results shown in –d illustrate the use of map typing to stratify operational model evaluation over two of the eight analysis domains. Summary results for all eight analysis domains are shown in . For display in these tables, RMSE and MBE values for a given synoptic pattern were calculated separately for each model at each monitoring site within the analysis domain, the median value across all sites was then calculated for each model, and finally the results were averaged over all models. The last column shows the range of the model-average RMSE across all synoptic patterns to illustrate the impact of different synoptic patterns on model performance. For the three analysis regions within the eastern NA map typing domain (NE, MW, and SE; ), the MW region shows the largest range of model performance, both across synoptic patterns and across different models. In the SE region, the impact of different synoptic regimes on RMSE and MBE is least pronounced. In all three analysis regions, pattern 6 is characterized by a low average RMSE and absolute MBE. The pattern with the largest average RMSE is pattern 1 in the NE, pattern 5 in the MW, and pattern 3 in the SE.
Table 4. (a) RMSE and (b) MBE of DM8A ozone for each synoptic pattern for the three analysis regions in the eastern NA map typing domain
Table 5. (a) RMSE and (b) MBE of DM8A ozone for each synoptic pattern for the three analysis regions in the western NA map typing domain
Table 6. (a) RMSE and (b) MBE of DM8A ozone for each synoptic pattern for the two analysis regions in the EU map typing domain
The three analysis regions within the western NA map typing domain (NW, CA, and SW; ) generally show less impact of different synoptic patterns on model performance compared with the regions within the eastern NA map typing domain. In particular, in the SW region there is only a difference of 0.7 ppb (1.2 ppb) between the synoptic patterns with the lowest and highest average RMSE (MBE). This indicates that synoptic influences play a relatively small role in governing summertime ozone air quality in this region, consistent with Figures S10–S13 (Supplemental Materials) that show relatively small DM8A ozone anomalies for the four different synoptic patterns in the SW region. In addition, a unique feature of the SW analysis domain is that the model-average MBE is positive for all patterns; future analyses may be aimed at investigating the reasons for this behavior. For the NW region, pattern 1 is associated with the lowest average RMSE, whereas pattern 2 is associated with the largest average RMSE. For CA, pattern 4 is associated with the lowest average RMSE, whereas pattern 2 again is associated with the largest average RMSE.
For the two analysis regions in the EU map typing domain (N. EU and S. EU), different synoptic patterns cause a wider spread in both average RMSE and MBE in the N. EU than the S. EU region (). For both N. EU and S. EU, the lowest RMSE is associated with pattern 1 that is characterized by below-average temperature and solar radiation, above-average cloud fraction, strong westerly winds, and below-average observed DM8A ozone concentrations over much of Europe. For N. EU, the highest RMSE is associated with pattern 4, whereas the highest RMSE is associated with pattern 3 for S. EU. Both patterns are characterized by above-average observed DM8A ozone concentrations in these respective regions (Figures S14–S19, Supplemental Materials).
In an attempt to better link the underlying meteorological characteristics of each pattern with air quality model performance, we plotted the DM8A ozone RMSE and MBE for a given pattern at each site against the meteorological parameters for this pattern at the same site. For easy display, we then created bins of ranges of the different meteorological parameters and computed the average model performance metric (RMSE or MBE) for all values (sites/patterns) within this bin. The results for the eastern NA map typing domain are provided in , and the results for the EU map typing domain are shown in . Analysis was also performed for the western NA map typing domain, but results showed little dependence of model performance on meteorological parameters associated with the different synoptic patterns, consistent with the discussion of above. Therefore, no results are shown for the western NA map typing domain.
Figure 2. RMSE of DM8A ozone for different models over the eastern NA map typing domain as a function of (a) pattern average cloud fraction, (b) pattern short-wave radiation anomalies, (c) pattern 2-m temperature anomalies, and (d) pattern average wind speed.
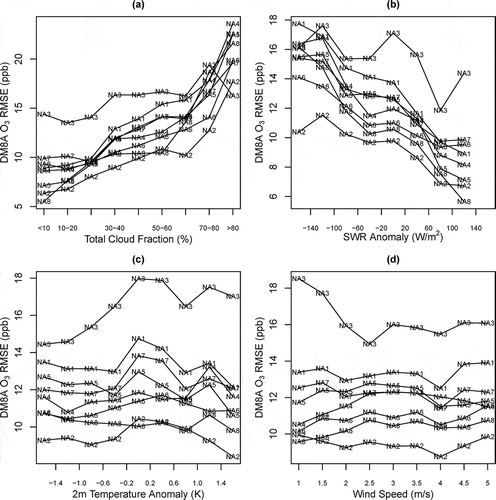
For NA, reveal that the performance of all modeling systems participating in this international model intercomparison study is strongly linked with cloud cover and short-wave radiation anomalies, whereas there was little dependence on temperature anomalies and wind speed. In particular, all modeling systems showed a large positive MBE and a large RMSE when the cloud fraction was high and the short-wave solar radiation anomalies were negative. Conversely, RMSE was lowest and the MBE was close to zero for most models when the cloud fraction was low and the short-wave solar radiation anomalies were positive. Note that the results shown in include stations in all three analysis regions of the eastern NA map typing domain; separate analysis (not shown here) revealed that performing this analysis separately for each analysis region had only a minor impact on the results.
The behavior in the EU map typing domain () is different from that in the eastern NA map typing domain. Almost all modeling systems showed deteriorating performance (larger RMSE and more negative MBE) with increasingly positive short-wave radiation anomalies. In terms of cloud fraction, the model performance was generally the worst for intermediate cloud conditions (cloud fractions 30–50%), which were characterized by the largest RMSE and most negative MBE. Further analysis showed slight differences in this behavior between N. EU and S. EU, with modeling systems generally showing a more monotonous decrease in RMSE with increasing cloud cover in N. EU while showing a maximum RMSE at intermediate cloud fractions in S. EU. In further contrast to eastern NA, model performance for EU also showed an association with both temperature anomalies and wind speeds, with lower temperatures and higher winds generally leading to better model performance for DM8A ozone and higher temperature and lower winds generally leading to worse model performance. The contrast in model behavior between the eastern NA and EU domains will be the subject of future diagnostic model evaluation studies. Potentially contributing factors include differences in the types of prevalent cloud types (stratiform vs. convective) over both domains and systematic differences in the agreement between modeled emissions and actual emissions that would confound the interpretation of model performance solely in terms of meteorological forcing.
In summary, the results presented in this section demonstrate that meteorological conditions associated with specific synoptic patterns had a distinct impact on model performance over both the eastern NA and EU map typing domains for almost all modeling systems participating in AQMEII. For example, RMSE of DM8A ozone typically was twice as high when cloud fractions were high than when cloud fractions were low in eastern NA. Results also showed map typing to be more useful to discriminate model behavior in some regions than others, depending on atmospheric dynamics and the relative effects of synoptic-scale versus local forcings on pollutant concentrations.
Observed and modeled temporal features
The above analysis used observed synoptic conditions to stratify operational model performance and to determine associations between different meteorological parameters and model performance for DM8A ozone. A related question is how well the different modeling systems performed in replicating observed forcings on the synoptic as well as other time scales. To this end, we applied the framework of scale analysis described in Rao et al. (Citation1997) and Hogrefe et al. (Citation2000) to observed and simulated hourly time series of ozone. As a starting point, –b depict the power spectra of observed and modeled ozone over both NA and EU. The spectra were calculated separately at each site and then averaged over all sites to highlight the key features. These figures show two curves with model-predicted spectra: the “regional models” curve was calculated by averaging over the spectra calculated for each of the individual models listed in , whereas the “GEMS” curve represents the power spectrum estimated from the coarse-grid global fields that were used to derive boundary conditions for many of the regional AQMEII simulations (Schere et al., Citation2012). The global fields were obtained from the Global and Regional Earth-system Monitoring Using Satellite and In-Situ Data (GEMS) project. Note that these global fields were only available every 3 hr, thus the “GEMS” spectrum starts at a larger period than the observed and the regional model spectra calculated from hourly data. These figures indicate that over both NA and EU, the average regional model spectrum is closer to that of the observations than the “GEMS” spectrum for all periods, indicating that the regional models were able to better simulate some of the variance that was missing from the global fields. Moreover, –b also show that over both continents the regional models underestimate the observed variability for fluctuations with periods lasting to about 2 days, though less so than the global fields. For longer periods, there is relatively close agreement between the observed and average regional model spectra over NA, whereas over EU the observed variability continues to be underestimated by the regional models, though to a lesser extent than for the higher frequencies.
Figure 6. Power spectra of observations, regional model simulations, and global model simulations used as boundary conditions for most regional model simulations. The power spectra were calculated separately at each site and then averaged over all sites. (a) NA, (b) EU.
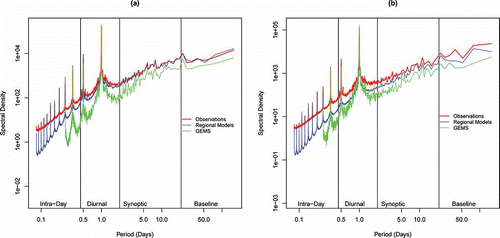
To further investigate the modeling systems’ ability to reproduce observed variance on different time scales, show the observed and modeled variances for each model and each of the four frequency bands defined in Hogrefe et al. (Citation2000) and delineated in . As discussed in Hogrefe et al. (Citation2000), the intraday component represents fast-acting, local-level processes, the diurnal component is dominated by the 24-hr periodicity, the synoptic component contains fluctuations related to changing weather patterns, and the baseline component reflects the low-frequency part of the signal representing temporal fluctuations that are largely determined by boundary conditions and seasonal variations in emissions and photochemical activity. The variances in were derived by summing the spectral densities shown in for each of the four frequency bands; this calculation was performed separately at each site and results were then averaged spatially for display. The results confirm the “regional model” results from the discussion above that was based on averaging all power spectra calculated from individual models. These analyses also show that the largest component of the observed and modeled variances occurs on the diurnal scale, followed by the synoptic, baseline, and intraday scales, both over NA and EU. The modeling systems generally capture this time scale dependence of the variance.
In addition, also show substantial model-to-model differences. For example, the variances of the baseline components differ by more than a factor of 2 between the different model simulations. Several features are noteworthy in displaying results for EU. First, the observed and simulated variances on the diurnal and synoptic scales tend to be lower than those over NA despite the fact that the mean ozone levels are similar over both continents (not shown). A potential explanation for this behavior may be that a greater portion of monitors in the EU domain are affected by nearby water bodies, which would tend to suppress diurnal and synoptic forcings. Second, model-to-model variability is more pronounced in EU than in NA, especially on the diurnal and baseline scales. And finally, and confirm that almost all models underestimate the observed variance on all scales, including the synoptic and baseline scales, although the agreement between observed and modeled variances for these two scales is better over NA than EU. The most notable exception to the general underestimation of observed component variances is the behavior of model “NA3,” which shows overestimation especially for the diurnal component. Separate analysis showed that the treatment of vertical mixing processes in this simulation likely played a major role in causing these differences.
Although the analysis above measures the modeling systems’ ability to reproduce the observed overall variability on different time scales, it does not provide information on how well the modeling systems captured the temporal evolution of the observed component time series. Therefore, we calculated the correlation coefficients between the observed and modeled component time series on the intraday, diurnal, synoptic, and baseline time scales for each model and at each site. The component time series were estimated with the Kolmogorov-Zurbenko (KZ) filter as described in Hogrefe et al. (Citation2000). –b depict this information as box-and-whisker plots for both NA and EU. These plots illustrate that all modeling systems had correlations of 0.3 or less with the observed intraday component over both NA and EU. The correlations tend to be highest for the diurnal component because of the inherent 24-hr cycle present in both observations and model predictions. The correlations are also high for the baseline component over both continents, with median values of 0.6 or higher. For the synoptic component, the correlations tend to be higher over NA than EU. In addition, model-to-model differences in the correlation coefficients tend to be most pronounced for the synoptic and baseline components over both continents, indicating that these two components play a vital role in determining overall model skill in terms of capturing observed fluctuations in pollutant concentrations.
Figure 9. Box-and-whisker plots of correlation coefficients between observations and model simulations for different temporal components. (a) NA, (b) EU. On either side of the box, the whiskers extend to the most extreme data point or 1.5 times the interquartile range (i.e., the difference between the 25th and 75th percentiles), whichever is less.
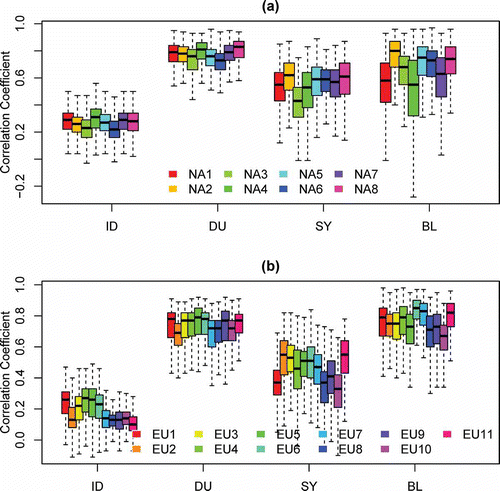
Use of spatial representativeness analysis to stratify operational model evaluation
When applied to continental-scale domains such as those used in AQMEII, the modeling systems should be expected to replicate observed regional-scale phenomena. On the other hand, such simulations should not be expected to reproduce more local-scale phenomena. Thus, for model evaluation it is often of interest to separate monitors sited to measure regional-scale phenomena from those influenced by local-scale phenomena (Solazzo et al., Citation2012b). Such separation can be accomplished through a variety of means, e.g., a careful review of the network design plan and site metadata that may contain information about land use, site location, nearby sources, etc. However, such an approach can be very resource-intensive for large monitoring data sets such as the ones used in AQMEII and the approach can also be hampered by the varying completeness and quality of the site metadata, in particular when observations are assembled from a variety of sources located in different jurisdictions. Moreover, using metadata as grouping criterion is only an indirect way of distinguishing between stations that are influenced by processes on different scales. An alternate approach would be to rely on an analysis of the spatial variability present in the observational data set to subdivide this data set into regionally representative sites and locally influenced sites for the purpose of model evaluation. The rationale for this approach is that if certain sites behave very differently from surrounding sites, they may not be representative of the scales the regional model simulations were designed to capture and should be treated separately for operational evaluation.
To illustrate this alternate approach, at each site in a given domain (NA or EU) we first calculated the root mean square difference (RMSD) between the May–September 2006 time series of the DM8A ozone concentrations at that site and the corresponding time series at all other monitoring locations and also noted the distance between each pair of sites. Next, these RMSD values were binned into different distance-between-station intervals and an average RMSD value was calculated for each distance bin. Finally, this analysis was repeated for each station in the analysis domain so that for each distance bin, there was a distribution of average RMSD values, with the sample size of the distribution being equal to the number of stations. After reviewing the outcome of this step, we defined “regionally representative sites” as those sites for which the RMSD at a separation distance of 50 km is at or below the 75th percentile of all RMSD values in that distance bin. In other words, this definition implies that 75% of all sites are considered to be “regionally representative,” whereas the remaining 25% are considered to be “locally influenced.” In reality, this separation is likely a continuum rather than a constant value, but a fixed threshold definition was chosen here for illustration purposes. For both the NA and EU domains, this threshold corresponds to a 50 km RMSD value of roughly 10 ppb.
–b list the RMSE and correlation coefficients for all modeling systems grouped by regionally representative versus locally influenced sites for both NA and EU. Over NA, the average RMSE across all models is 12.1 ppb at regionally representative sites and 15.0 ppb (i.e., roughly 25% higher) at locally influenced sites. The average correlation coefficient at the regionally representative sites is 0.69 versus 0.61 at locally influenced sites. Moreover, the results also show a greater model-to-model spread of performance metrics at regionally representative versus locally influenced sites; this spread is 7.0 ppb for RMSE and 0.2 for the correlation coefficient at the regionally representative sites versus 5.2 ppb for RMSE and 0.14 for the correlation coefficient at the locally influenced sites. In other words, focusing the analysis on sites influenced by scales the modeling systems were designed to capture yields a better ability to distinguish performance between models because the comparison is less influenced by phenomena the regional models are not designed to simulate. shows that results for the EU are quite similar. Here, the average RMSE across all models is 11.0 ppb at regionally representative sites and 12.8 ppb (i.e., roughly 15% higher) at locally influenced sites. The average correlation coefficient at the regionally representative sites is 0.72 versus 0.61 at locally influenced sites. And as was the case for the NA domain, results for the EU domain also show a greater model-to-model spread of performance metrics at regionally representative versus locally influenced sites, again indicating that regionally representative sites provide a better data set for evaluating these types of models.
Table 7. RMSE and correlation coefficient at regionally representative and locally influenced sites over (a) NA and (b) EU
To contrast the results obtained by grouping stations based on their similarity to neighboring stations as proposed in this analysis with the more traditional approach of grouping stations based on station metadata such as land use, –b present model performance results when stations were grouped as urban, suburban, or rural based on available station metadata. These results show that there are only minor differences in model performance between the three land-use types, both for NA (RMSE differences of 1.2 ppb and correlation coefficient differences of 0.01) and EU (RMSE differences of 0.3 and correlation coefficient differences of 0.01), indicating that station grouping based on station metadata information is not the best approach to select stations best suitable for evaluating regional-scale modeling systems.
Table 8. RMSE and correlation coefficient at rural, suburban, and urban sites over (a) NA and (b) EU
These results illustrate that including sites influenced by local-scale phenomena for the evaluation of regional-scale modeling systems such as those analyzed in AQMEII increases the estimated RMSE, confounding the use of this metric as a measure of regional model error to be reduced through improvements to model inputs or model physics/chemistry, or numerical modeling. On the other hand, if model development and/or model applications are explicitly focused on smaller scales, such locally influenced sites can provide a valuable subset to quantify the improvements in model performance achieved through such model development. As noted above, there are a number of ways to address the issue of spatial representativeness for operational model evaluation, the specific observation-based selection criterion for “regionally representative” sites used here should only be viewed as an illustrative example and starting point for further analysis. It is likely that such analyses of the spatial representativeness of the observational data available for model evaluation need to be performed separately for each metric and pollutant of interest.
Observed and modeled spatial features
In the analysis above, observed spatial features were used to stratify the operational model evaluation. In this section, we explore how well the different modeling systems reproduced observed spatial structures. The metric used for this analysis is the e-folding distance of synoptic-scale ozone fluctuations that has been described and applied in previous model evaluation studies (Gilliland et al., Citation2008; Godowitch et al., Citation2008). As discussed in these studies, the e-folding distance is a measure of how well the time series of the synoptic-scale ozone component at a given location correlates with the synoptic-scale ozone component at a different location. Typical e-folding distances for summertime ozone over the northeastern United States range between 400 and 600 km, with shorter distances indicating that local processes modify synoptic-scale ozone fluctuations to a greater degree compared with locations with longer e-folding distances.
–d present box-and-whisker plots of e-folding distances calculated from observations and model predictions for both NA and EU using two separate sets of sites, i.e., the locally influenced versus regionally representative sites defined in the previous section. The e-folding distances were calculated separately at each site; the box-and-whisker plots represent the distributions across all sites. The results reveal that the e-folding distances are generally shorter over EU than NA in both observations and model predictions for both types of sites. In addition, the e-folding distances are generally also shorter at locally influenced versus regionally representative sites, corroborating the argument that this metric can be used to measure the relative influence of local versus regional effects. The results of ANOVA showed that over NA, the mean e-folding distances were different at the 95% confidence level between the two types of sites for observations and all model simulations. For the EU domain, the difference in mean e-folding distances between the two types of sites was significant at the 95% level for the observations and 8 of the 11 models.
Figure 10. Box-and-whisker plots of observed and simulated e-folding distances at regionally representative and locally influenced sites as defined in the text. (a) Locally influenced sites, NA; (b) regionally representative sites, NA; (c) locally influenced sites, EU; (d) regionally representative sites, EU. On either side of the box, the whiskers extend to the most extreme data point or 1.5 times the interquartile range (i.e., the difference between the 25th and 75th percentiles), whichever is less. The dashed line represents the median observed e-folding distance.
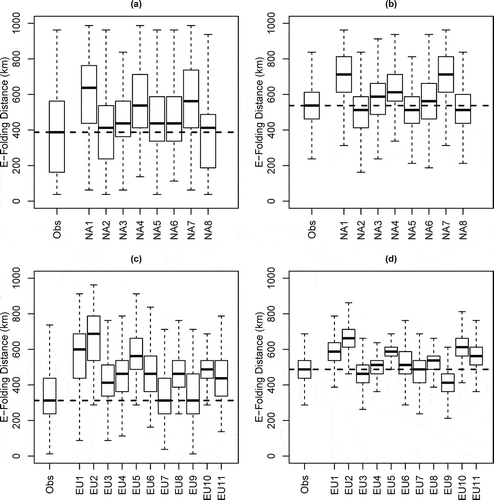
All modeling systems overestimate the observed e-folding distances over both NA and EU at locally influenced sites, consistent with the notion that the regional-scale modeling systems applied in AQMEII are not designed to fully represent local-scale effects. On the other hand, the model performance at regionally representative sites is more varied: although most modeling systems overestimate the observed e-folding distances at these sites over both NA and EU, several models show a slight underestimation. Because the modeling systems applied in AQMEII should be expected to represent the phenomena at regionally representative sites, future work should be directed at identifying and correcting the reasons for the discrepancies seen between observed and modeled e-folding distances at these sites.
Summary
This study presented a space-time evaluation of summertime DM8A ozone concentrations over North America and Europe using the database generated during Phase 1 of AQMEII (Galmarini and Rao, Citation2011). The evaluation focused on identifying temporal and spatial features that could be used to stratify operational model evaluation and to test to which extent the various modeling systems could replicate such features present in the observations. Using map typing to perform temporal stratification of model performance, it was demonstrated that the meteorological conditions associated with specific synoptic patterns had a distinct impact on model performance over both eastern NA and EU for almost all modeling systems participating in AQMEII.
In terms of observed and modeled variances on different time scales, the results show that over both NA and EU the regional models participating in AQMEII were able to better simulate the observed variance than the global model used to derive chemical boundary conditions. However, almost all regional models still underestimated the observed variance on all scales. All modeling systems showed poor correlations with observed fluctuations on the intraday time scale over both NA and EU. Model-to-model differences in the correlation coefficients with the observed component time series tend to be most pronounced for the synoptic and baseline components over both continents, indicating that these two components play a key role in determining overall model skill in terms of capturing observed fluctuations in pollutant concentrations.
Using observed root mean square differences of the DM8A ozone between different sites as a criterion, we introduced a methodology to distinguish between locally influenced and regionally representative sites for the purpose of model performance evaluation. The results show that all of the regional-scale modeling systems analyzed in this study have worse performance at locally influenced sites. In other words, inclusion of sites influenced by local-scale phenomena for the evaluation of regional-scale modeling systems increases the estimated RMSE, confounding the use of this metric as a measure of model error that should be reduced through improvements to model inputs or the modeling system itself. On the other hand, the locally influenced sites identified by this method could serve a good reference data set for evaluating model performance in future studies aimed at evaluating and improving model performance at small scales.
In summary, the analyses presented in this paper demonstrated how observed temporal and spatial information can be used to stratify operational model performance and to test the modeling systems’ ability to replicate observed temporal and spatial features, especially at scales the modeling systems are designed to capture. Furthermore, decisions for the improvement of regional air quality models should be based on the information derived from only regionally representative sites.
Supplemental Data
Supplemental data are available for this paper. Go to the publisher’s online edition of the Journal of the Air & Waste Management Association for additional results from the map typing analysis described in this paper.
Supplemental Material
Download PDF (3.2 MB)Acknowledgments
The authors gratefully acknowledge the contribution of various groups to the first Air Quality Model Evaluation International Initiative (AQMEII) activity. The modeling simulations analyzed in this study were performed by IPSL, CEA/CNRS/UVSQ, France; Aarhus University, Denmark; University of Aveiro, Portugal; Helmholtz-Zentrum Geesthacht, Germany; U.S. Environmental Protection Agency, USA; Environ International Corporation, USA; Environment Canada, Canada; CEREA, France; Leibniz Institute for Tropospheric Research, Germany; Finnish Meteorological Institute, Finland; Meteorological and Hydrological Service, Croatia; TNO, The Netherlands; University of Herfordshire, United Kingdom; IMK-IFU, Germany; and National Oceanic and Atmospheric Administration, USA. The following agencies have prepared the data sets used in this study: U.S. Environmental Protection Agency (North American emissions processing and gridded meteorology); U.S. Environmental Protection Agency, Environment Canada, Mexican Secretariat of the Environment and Natural Resources (Secretaría de Medio Ambiente y Recursos Naturales, SEMARNAT), and National Institute of Ecology (Instituto Nacional de Ecología, INE) (North American national emissions inventories); TNO (European emissions processing); Laboratoire des Sciences du Climat et de l’Environnement, IPSL, CEA/CNRS/UVSQ (gridded meteorology for Europe); and ECMWF/GEMS project and Météo-France/CNRM-GAME (chemical boundary conditions). Ambient North American ozone concentration measurements were extracted from Environment Canada’s National Atmospheric Chemistry Database (NAtChem) database and provided by several U.S. and Canadian agencies (AQS, CASTNet, and NAPS networks); for European air quality data the following data centers were used: EMEP European Environment Agency/European Topic Center on Air and Climate Change/AirBase provided European ozone data. The Finish Meteorological Institute provided biomass burning emission data for Europe. Joint Research Center Ispra/Institute for Environment and Sustainability provided its ENSEMBLE system for model output harmonization and analyses and evaluation. The authors also thank OAA/OAR/ESRL PSD, Boulder, Colorado, USA, for providing the NCEP Reanalysis 2 data via their Web site at http://www.esrl.noaa.gov/psd/. Finally, the authors thank ECMWF for providing the ERA interim reanalysis fields via their Web site at http://data-portal.ecmwf.int/data/d/interim_full_daily.
The views expressed here are those of the authors and do not necessarily reflect the views and policies of the U.S. Environmental Protection Agency (EPA) or any other organization participating in the AQMEII project. This paper has been subjected to EPA review and approved for publication.
Additional information
Notes on contributors
C. Hogrefe
C. Hogrefe, S. Roselle, and R. Mathur are with the Atmospheric Modeling and Analysis Division in the National Exposure Research Laboratory, Office of Research and Development, U.S. Environmental Protection Agency, Research Triangle Park, NC.
S.T. Rao
S.T. Rao is an adjunct professor at the Department of Marine, Earth and Atmospheric Sciences, North Carolina State University, Raleigh, NC. He previously was the director of the Atmospheric Modeling and Analysis Division in the National Exposure Research Laboratory, Office of Research and Development, U.S. Environmental Protection Agency, Research Triangle Park, NC.
S. Galmarini
S. Galmarini is with the European Commission Joint Research Centre in Ispra, Italy.
References
- Appel, K.W., C. Chemel, S.J. Roselle, X.V. Francis, R.-M. Hu, R.S. Sokhi, S.T. Rao, and S. Galmarini. 2012. Examination of the Community Multiscale Air Quality (CMAQ) model performance over the North American and European domains. Atmos. Environ. 53:142–155. doi:10.1016/j.atmosenv.2011.11.016
- Brandt, J., J.D. Silver, L.M. Frohn, C. Geels, A. Gross, A.B. Hansen, K.M. Hansen, G.B. Hedegaard, C.A. Skjoth, H. Villadsen, A. Zare, and J.H. Christensen. 2012. An integrated model study for Europe and North America using the Danish Eulerian Hemispheric Model with focus on intercontinental transport of air pollution. Atmos. Environ. 53:156–176. doi:10.1016/j.atmosenv.2012.01.011
- Dee, D. P., S. M. Uppala, A. J. Simmons, P. Berrisford, P. Poli, S. Kobayashi, U. Andrae, M. A. Balmaseda, G. Balsamo, P. Bauer,et al. 2011. The ERA-Interim reanalysis: Configuration and performance of the data assimilation system. Q. J. R. Meteorol. Soc. 137:553–597. doi:10.1002/qj.828
- Dennis, R., T. Fox, M. Fuentes, A. Gilliland, S. Hanna, C. Hogrefe, J. Irwin, S. T. Rao, R. Scheffe, K. Schere, D. Steyn, and A. Venkatram. 2010. A framework for evaluating regional-scale numerical photochemical modeling systems. Environ. Fluid Mech. (Dordr.) 10:471–489. doi:10.1007/s10652-009-9163–2
- Ferreira, J., A. Rodriguez, A. Monteiro, A. I. Miranda, M. Dios, J. A. Souto, G. Yarwood, U. Nopmongcol, and C. Borrego. 2012. Air quality simulations for North America-MM5-CAMx modelling performance for main gaseous pollutants. Atmos. Environ. 53:212–224. doi:10.1016/j.atmosenv.2011.10.020
- Forkel, R., J. Werhahn, A.B. Hansen, S. McKeen, S. Peckham, G. Grell, and P. Suppan. 2012. Effect of aerosol-radiation feedback on regional air quality—A case study with WRF/Chem. Atmos. Environ. 53:202–211. doi:10.1016/j.atmosenv.2011.10.009
- Galmarini, S., R. Bianconi, R. Addis, S. Andronopoulos, P. Astrup, J. C. Bartzis, R. Bellasio, R. Buckley, H. Champion, M. Chino, et al. 2004a. Ensemble dispersion forecasting—Part II: Application and evaluation. Atmos. Environ. 38:4619–4632. doi:10.1016/j.atmosenv.2004.05.031
- Galmarini, S., R. Bianconi, W. Appel, E. Solazzo, S. Mosca, P. Grossi, M. Moran, K. Schere, and S. T. Rao. 2012a. ENSEMBLE and AMET: Two systems and approaches to a harmonized, simplified and efficient facility for air quality models development and evaluation. Atmos. Environ. 53:51–59. doi:10.1016/j.atmosenv.2011.08.076
- Galmarini, S., R. Bianconi, W. Klug, T. Mikkelsen, R. Addis, S. Andronopoulos, P. Astrup, A. Baklanov, J. Bartniki, J. C. Bartzis, et al. 2004b. Ensemble dispersion forecasting—Part I: Concept, approach and indicators. Atmos. Environ. 38:4607–4617. doi:10.1016/j.atmosenv.2004.05.030
- Galmarini, S., and S.T. Rao. 2011. The AQMEII two-continent Regional Air Quality Model evaluation study: Fueling ideas with unprecedented data. Atmos. Environ. 45:2464–2464. doi:10.1016/j.atmosenv.2011.03.025
- Galmarini, S., S. T. Rao, and D. G. Steyn. 2012b. AQMEII: An international initiative for the evaluation of regional-scale air quality models—Phase 1 preface. Atmos. Environ. 53:1–3. doi:10.1016/j.atmosenv.2012.03.001
- Gilliam, R.C., J.M. Godowitch, and S.T. Rao. 2012. Improving the horizontal transport in the lower troposphere with four dimensional data assimilation. Atmos. Environ. 53:186–201. doi:10.1016/j.atmosenv.2011.10.064
- Gilliland, A.B., C. Hogrefe, R.W. Pinder, J.M. Godowitch, K.. Foley, and S.T. Rao. 2008. Dynamic evaluation of regional air quality models: Assessing changes in O-3 stemming from changes in emissions and meteorology. Atmos. Environ. 42:5110–5123. doi:10.1016/j.atmosenv.2008.02.018
- Godowitch, J. M., C. Hogrefe, and S. T. Rao. 2008. Diagnostic analyses of a regional air quality model: Changes in modeled processes affecting ozone and chemical-transport indicators from NO(x) point source emission reductions. J. Geophys. Res. Atmos. 113(D19):D19303. doi:10.1029/2007JD009537
- Hegarty, J., H. Mao, and R. Talbot. 2007. Synoptic controls on summertime surface ozone in the northeastern United States. J. Geophys. Res. Atmos. 112(D14):D14306. doi:10.1029/2006JD008170
- Hogrefe, C., J. Biswas, B. Lynn, K. Civerolo, J. Y. Ku, J. Rosenthal, C. Rosenzweig, R. Goldberg, and P. L. Kinney. 2004. Simulating regional-scale ozone climatology over the eastern United States: Model evaluation results. Atmos. Environ. 38:2627–2638. doi:10.1016/j.atmosenv.2004.02.033
- Hogrefe, C., S. T. Rao, I. G. Zurbenko, and P. S. Porter. 2000. Interpreting the information in ozone observations and model predictions relevant to regulatory policies in the Eastern United States. Bull. Am. Meteorol. Soc. 81:2083–2106. doi:10.1175/1520-0477(2000)081<2083:ITIIOO>2.3.CO;2
- Kanamitsu, M., W. Ebisuzaki, J. Woollen, S. K. Yang, J. J. Hnilo, M. Fiorino, and G. L. Potter. 2002. NCEP-DOE AMIP-II reanalysis (R-2). Bull. Am. Meteorol. Soc. 83:1631–1643. doi:10.1175/BAMS-83-11-1631(2002)083<1631:NAR>2.3.CO;2
- Kirchhofer, W. 1973. Classification of European 500 mb Patterns. Geneva: Arbeitsbericht der Schweizerischen Meteorologischen Zentralanstalt.
- Lund, I. A. 1963. Map-pattern classification by statistical methods. J. Appl. Meteorol. 2:56–65. doi:10.1175/1520-0450(1963)002<0056:MPCBSM>2.0.CO;2
- McKendry, I. G., D. G. Steyn, and G. McBean. 1995. Validation of synoptic circulation patterns simulated by the Canadian climate centre general circulation model for western North America—Research note. Atmos. Ocean 33:809–825. doi:10.1080/07055900.1995.9649554
- Nopmongcol, U., B. Koo, E. Tai, J. Jung, P. Piyachaturawat, C. Emery, G. Yarwood, G. Pirovano, C. Mitsakou, and G. Kallos. 2012. Modeling Europe with CAMx for the Air Quality Model Evaluation International Initiative (AQMEII). Atmos. Environ. 53:177–185. doi:10.1016/j.atmosenv.2011.11.023
- Pirovano, G., A. Balzarini, B. Bessagnet, C. Emery, G. Kallos, F. Meleux, C. Mitsakou, U. Nopmongcol, G. M. Riva, and G. Yarwood. 2012. Investigating impacts of chemistry and transport model formulation on model performance at European scale. Atmos. Environ. 53:93–109. doi:10.1016/j.atmosenv.2011.12.052
- Pouliot, G., T. Pierce, H.D. van der Gon, M. Schaap, M. Moran, and U. Nopmongcol. 2012. Comparing emission inventories and model-ready emission datasets between Europe and North America for the AQMEII project. Atmos. Environ. 53:4–14. doi:10.1016/j.atmosenv.2011.12.041
- Rao, S.T., S. Galmarini, and K. Puckett. 2011. Air Quality Model Evaluation International Initiative (AQMEII): Advancing the state of the science in regional photochemical modeling and its applications. Bull. Am. Meteorol. Soc. 92:23–30. doi:10.1175/2010BAMS3069.1
- Rao, S. T., I. G. Zurbenko, R. Neagu, P. S. Porter, J. Y. Ku, and R. F. Henry. 1997. Space and time scales in ambient ozone data. Bull. Am. Meteorol. Soc. 78:2153–2166. doi:10.1175/1520-0477(1997)078<2153:SATSIA>2.0.CO;2
- Sartelet, K.N., F. Couvidat, C. Seigneur, and Y. Roustan. 2012. Impact of biogenic emissions on air quality over Europe and North America. Atmos. Environ. 53:131–141. doi:10.1016/j.atmosenv.2011.10.046
- Schere, K., J. Flemming, R. Vautard, C. Chemel, A. Colette, C. Hogrefe, B. Bessagnet, F. Meleux, R. Mathur, S. Roselle, R.-M. Hu, R.S. Sokhi, S. T. Rao, and S. Galmarini. 2012. Trace gas/aerosol boundary concentrations and their impacts on continental-scale AQMEII modeling domains. Atmos. Environ. 53:38–50. doi:10.1016/j.atmosenv.2011.09.043
- Solazzo, E., R. Bianconi, G. Pirovano, V. Matthias, R. Vautard, M.D. Moran, K.W. Appel, B. Bessagnet, J. Brandt, J.H. Christensen, et al. 2012a. Operational model evaluation for particulate matter in Europe and North America in the context of AQMEII. Atmos. Environ. 53:75–92. doi:10.1016/j.atmosenv.2012.02.045
- Solazzo, E., R. Bianconi, R. Vautard, K.W. Appel, M.D. Moran, C. Hogrefe, B. Bessagnet, J. Brandt, J.H. Christensen, C. Chemel, et al. 2012b. Model evaluation and ensemble modelling of surface-level ozone in Europe and North America in the context of AQMEII. Atmos. Environ. 53:60–74. doi:10.1016/j.atmosenv.2012.01.003
- Vautard, R., M.D. Moran, E. Solazzo, R.C. Gilliam, V. Matthias, R. Bianconi, C. Chemel, J. Ferreira, B. Geyer, A.B. Hansen, A. Jericevic, M. Prank, A. Segers, J.D. Silver, J. Werhahn, R. Wolke, S.T. Rao, and S. Galmarini. 2012. Evaluation of the meteorological forcing used for the Air Quality Model Evaluation International Initiative (AQMEII) air quality simulations. Atmos. Environ. 53:15–37. doi:10.1016/j.atmosenv.2011.10.065
- Yarnal, B.M. 1993. Synoptic Climatology in Environmental Analysis: A Primer. Boca Raton, FL: Belhaven Press.