Abstract
Motivated by the question of whether and how a state-of-the-art regional chemical transport model (CTM) can facilitate characterization of CO2 spatiotemporal variability and verify CO2 fossil-fuel emissions, we for the first time applied the Community Multiscale Air Quality (CMAQ) model to simulate CO2. This paper presents methods, input data, and initial results for CO2 simulation using CMAQ over the contiguous United States in October 2007. Modeling experiments have been performed to understand the roles of fossil-fuel emissions, biosphere–atmosphere exchange, and meteorology in regulating the spatial distribution of CO2 near the surface over the contiguous United States. Three sets of net ecosystem exchange (NEE) fluxes were used as input to assess the impact of uncertainty of NEE on CO2 concentrations simulated by CMAQ. Observational data from six tall tower sites across the country were used to evaluate model performance. In particular, at the Boulder Atmospheric Observatory (BAO), a tall tower site that receives urban emissions from Denver, CO, the CMAQ model using hourly varying, high-resolution CO2 fossil-fuel emissions from the Vulcan inventory and CarbonTracker optimized NEE reproduced the observed diurnal profile of CO2 reasonably well but with a low bias in the early morning. The spatial distribution of CO2 was found to correlate with NOx, SO2, and CO, because of their similar fossil-fuel emission sources and common transport processes. These initial results from CMAQ demonstrate the potential of using a regional CTM to help interpret CO2 observations and understand CO2 variability in space and time. The ability to simulate a full suite of air pollutants in CMAQ will also facilitate investigations of their use as tracers for CO2 source attribution. This work serves as a proof of concept and the foundation for more comprehensive examinations of CO2 spatiotemporal variability and various uncertainties in the future.
Implications:
Atmospheric CO2 has long been modeled and studied on continental to global scales to understand the global carbon cycle. This work demonstrates the potential of modeling and studying CO2 variability at fine spatiotemporal scales with CMAQ, which has been applied extensively, to study traditionally regulated air pollutants. The abundant observational records of these air pollutants and successful experience in studying and reducing their emissions may be useful for verifying CO2 emissions. Although there remains much more to further investigate, this work opens up a discussion on whether and how to study CO2 as an air pollutant.
Introduction
CO2 emission verification
The increase of atmospheric CO2 concentrations, as the largest human-induced climate forcer, is continuing and accelerating (Canadell et al., Citation2007). Reducing anthropogenic emissions is the most effective way to mitigate the resulting climate change risks. The success of an international collaborative effort in emissions reduction relies upon accurate information of current emissions in each country and their change over time (National Research Council [NRC], Citation2010). Under the United Nations Framework Convention on Climate Change (UNFCCC), all countries are required to report annual anthropogenic emissions and removal of greenhouse gases (GHGs), although developing countries are allowed to report less frequently and in less detail than Annex I (developed) countries. These self-reported national emissions inventories, however, are known to have uncertainties attributable to the incomplete knowledge of the numerous emission sources or inaccurate national and state statistical data (e.g., Andres et al., Citation2012; Guan et al., Citation2012). Emissions verification, which aims at (1) reducing uncertainties in current emissions inventories and (2) monitoring and verifying changes in emissions over time (NRC, 2010), has emerged as an urgent need for decision making by policymakers and business leaders (Marland, Citation2008; Matthias et al., 2010).
From the perspective of the global carbon cycle, our understanding of fossil-fuel emissions is generally believed to be better than that of natural carbon sources and sinks (Canadell et al., Citation2007); there are currently large gaps of knowledge in the biogeochemistry and physics of the natural carbon cycle (Le Quere et al., Citation2009), whereas fossil-fuel emissions are largely constrained by relatively well-documented global fuel consumption data (Boden et al., Citation2011). Nevertheless, our understanding of some important characteristics of fossil-fuel emissions, such as their spatiotemporal variability, remains elusive (Andres et al., Citation2012; Gurney et al., Citation2009). One unique feature of fossil-fuel emissions is their extremely uneven spatial distribution. A striking example pointed out by Marland (Citation2008) shows that even a tiny amount of uncertainty, that is, 0.9% as estimated by two independent sources, in one of the leading emitters like the United States is equivalent to total emissions from a very large group of smaller emitters in the world, that is, 147 countries out of 195 countries analyzed. A refined characterization of fossil-fuel emissions in space and time is necessary for emissions verification, and is also important for better constraining the carbon cycle (Peylin et al., Citation2011; Nassar et al., Citation2013).
A formal approach to the emissions verification problem is inverse modeling, which seeks to improve existing emissions estimates through assimilating information from atmospheric observations (NRC, 2010). In inverse modeling, a “source–receptor” relationship, that is, a relationship between concentrations at a “receptor” site and emissions strength from sources, is first established by modeling CO2 fluxes and atmospheric transport. With such a relationship and observations of CO2 concentrations in the atmosphere, an updated emissions estimate with reduced uncertainties (due to the addition of observational information) can be obtained using various estimation techniques (Tarantola, Citation2005).
Despite the rigor of the theory behind inverse assimilation, inverse modeling of atmospheric CO2 has long been challenged by (1) the sparseness of observational data (e.g., Gurney et al., Citation2002) and (2) inaccurate atmospheric transport modeling (e.g., Prather et al., Citation2008). The majority of CO2 flux-inversion studies have focused on natural (i.e., terrestrial biosphere and oceans) carbon fluxes, which are believed to be much more uncertain than fossil-fuel emissions. In turn, inverting for fossil-fuel emissions to achieve the objective of emissions verification would be challenged by the well-known “signal-to-noise” problem, namely, the strong, highly variable, but quite uncertain interference by the biosphere (NRC, 2010). As such, emissions verification turns out to be a challenging scientific problem of seeking an optimized combination of state-of-the-art observational techniques and modeling capabilities to pinpoint and quantify fossil-fuel emissions signals in the atmosphere. In order to find an effective and practical observational strategy, a growing number of observations from ground sites (e.g., Wunch et al., Citation2009; McKain et al., Citation2012), aircraft (e.g., Mays et al., Citation2009), and satellites (e.g., Kort et al., Citation2012) have been examined for their ability to constrain fossil-fuel emissions. Proxy techniques using isotopologues (e.g., Turnbull et al., Citation2006; Vogel et al., Citation2010; NRC, 2010) and trace-gas species to isolate fossil-fuel CO2 (e.g., Brioude et al., Citation2012; Palmer et al., Citation2006; Turnbull et al., Citation2006; Rivier et al., Citation2006) have also been proposed and investigated. Nevertheless, these previous observational studies were limited to a small number of locales, and thus the larger scale representativeness of their findings is not clear. There is apparently an urgent need for better interpreting these emerging observations and optimizing existing observation networks. The primary motivation of the present study is to answer the question of whether and how a state-of-the-art regional chemical transport model (CTM) could help serve such a need and thereby facilitate emissions verification.
The role of CMAQ—A state-of-the-art regional chemical transport model
Global three-dimensional (3-D) Eulerian CTMs play a pivotal role in global carbon-cycle research. Forward modeling analysis and diagnosis have been applied to understand global CO2 distributions (e.g., Denning et al., 1995) and transport mechanisms (e.g., Miyazaki et al., Citation2008). As a first step in CO2 flux inversion, global CTMs have been routinely applied to calculate the concentration-to-flux response functions at numerous sampling sites around the globe (e.g., Gurney et al., Citation2002). In recent years, a need has emerged for resolving finer scale CO2 transport and variability due to fossil-fuel emissions from urban and point sources in order to refine regional carbon budgets and verify emissions estimates. To this end, a regional CTM that has much higher spatial and temporal resolutions than current global models (often coarser than 1 degree) must be employed.
Compared to global modeling, high-resolution CO2 modeling on regional scales started relatively recently. Previous efforts have demonstrated the feasibility of regional CO2 modeling and shown some promising achievements with a high-resolution regional CTM. For example, Ahmadov et al. (Citation2007) coupled the Weather Research and Forecast Model (WRF) with a diagnostic biospheric model, the Vegetation Photosynthesis and Respiration Model (VPRM), and demonstrated the ability of the coupled model WRF-VPRM to capture the effects of the local land–sea-breeze circulation on CO2 at a coastal site. This circulation involves transport of CO2 respired from vegetation during the night out to sea followed by its return the following day. Other regional modeling studies have identified various factors that can affect CO2 spatiotemporal distributions, such as topography (van der Molen and Dolman, 2002), diurnal variations (Chevillard et al., Citation2002) and spatial heterogeneity of biospheric fluxes (Sarrat et al., Citation2007), covariance of transport and fluxes (Ahmadov et al. Citation2009), and so on. Some key requirements for regional CO2 modeling have also been noted, such as using realistic initial and lateral boundary conditions (Chevillard et al., Citation2002), because of the long lifetime of CO2 in the atmosphere. These regional modeling studies mostly targeted areas where biospheric sources and sinks of CO2 dominate, and issues that are of interest for the emissions verification problem, such as the magnitude and spatial extent of fossil-fuel signals in atmospheric CO2, were not addressed by these studies. Jacobson (Citation2008, Citation2011) has performed high-resolution CO2 modeling on global-through-urban nested domains to investigate the impact of local CO2 domes on O3 and particulate matter (PM) pollution.
Lagrangian particle dispersion models (LPDM) have been widely used in CO2-flux inference (e.g., McKain et al., Citation2012, and references therein) on regional to urban scales, because they can conveniently establish the “source–receptor” relationship needed for flux inversion. In principle, the two modeling approaches, that is, Eulerian and Lagrangian modeling, could be used simultaneously and compliment each other (Pillai et al., Citation2012).
The Community Multiscale Air Quality (CMAQ) model is a widely used regional CTM that was originally developed for atmospheric-chemistry and air-quality research (Byun and Schere, Citation2006). Source attribution of air pollutants has been one of the main applications of CMAQ (e.g., Hu et al., Citation2009; Cohan et al., Citation2005). Capabilities of forward (i.e., decoupled direct method, or DDM) (Dunker, Citation1984; Hakami et al., Citation2004) and adjoint (Hakami et al., Citation2007) sensitivity analysis have also been developed with CMAQ. Although so far there has been no effort of simulating CO2 with CMAQ, the highly modular model structure makes it straightforward to add new chemical species and modify their processes in CMAQ. Adding CO2 into CMAQ while retaining other model species enables simultaneous simulations and examinations of CO2 and a full suite of traditionally regulated air pollutants. Because there is abundant observational information and emissions-reduction experience for those air pollutants, it is of interest to explore their utility for facilitating CO2 source attribution (Brioude et al., Citation2012).
Goal of this work
Atmospheric CO2 has unique characteristics (e.g., long atmospheric lifetime, large background concentration, and strong bidirectional biospheric fluxes) that are distinctly different from other traditionally modeled chemical pollutants. Therefore, it is important to (1) characterize and understand the variability of CO2 on fine spatial and temporal scales and (2) identify and quantify various model uncertainties associated with CO2 fluxes, model transport, and initial and boundary conditions.
This paper serves as a proof-of-concept for using CMAQ to achieve these goals. Because we only present model results for a single month, sweeping conclusions drawn from these results are not possible, considering the significant seasonal variations of CO2. Instead, since this is the first time using CMAQ to simulate CO2, we focus on introducing the methodology, including input data and model experiment design, and try to interpret our results in the context of conventional understanding and findings from previous studies. The modeling framework here will form the foundation for more comprehensive investigations of CO2 spatiotemporal variability and modeling uncertainties in the future, which will be achieved by analyzing model simulations for a longer time span using more observational data.
The sections are organized as follows. In the Methods section, we first explicitly describe the input data used, highlighting the characteristics (e.g., magnitudes, spatial distributions, etc.) of different types of CO2 fluxes. Then we describe the design of model sensitivity experiments and the observational data to which the modeling results are compared. In the Results and Discussion section, we present modeling results from an initial implementation over the contiguous U.S. domain in October 2007. We show the characteristics of spatial patterns of CO2 near the surface simulated by the model, and perform model sensitivity experiments to understand the roles of meteorology, biosphere–atmosphere exchange, and fossil-fuel emissions in shaping the CO2 spatial distribution. A comparison of CO2 concentrations simulated by the model and observed at six tall-tower sites in the National Oceanic and Atmospheric Administration (NOAA) Earth System Research Laboratory (ESRL) network follows, with a focus on one site that is influenced by urban fossil-fuel emissions. Finally, the correlations between model-simulated CO2 and traditionally regulated air pollutants (i.e., CO, NOx, and SO2) are examined, and the implications for inverse modeling are discussed.
Methods
CMAQ configuration and input data for CO2 simulation
For the present study, we used CMAQ Version 5.0 with meteorological inputs from the Weather Research and Forecast (WRF) model (Version 3.1.1). The CMAQ model domain covers the contiguous United States and surrounding regions and has 36-km spatial resolution and 22 vertical layers from the surface to 50 hPa. The base configurations of WRF and CMAQ are listed in Table S1 in the supplemental information. CO2 is added into CMAQ as an inert chemical species, whose concentrations are determined by atmospheric transport (horizontal and vertical advection and diffusion) and four types of fluxes, including (1) bidirectional biosphere–atmosphere exchange, (2) bidirectional ocean–atmosphere exchange, (3) fossil-fuel emissions, and (4) fire emissions. The fossil-fuel and fire emissions fluxes are taken from existing emissions inventories. The atmosphere–biosphere and atmosphere–ocean bidirectional fluxes are from terrestrial biosphere model outputs. These four types of fluxes are prescribed in the model in the same manner as the emissions fluxes for existing chemical species in CMAQ. In principle, the biosphere–atmosphere and ocean–atmosphere bidirectional fluxes can be simulated in an inline mode by two processes, that is, emissions and dry deposition of CO2, as done for ammonia (NH3) and mercury (Hg) in CMAQ (Pleim and Ran, Citation2011). Currently, CO2 does not undergo dry deposition in CMAQ since the negative flux of NEE is an equivalent process. Such capability for modeling CO2 with CMAQ will be used in future efforts. The following subsections describe the input data that were used for CMAQ CO2 simulations in this work.
Biosphere–atmosphere exchange
The bidirectional biosphere–atmosphere exchange of CO2, net ecosystem exchange (NEE), is the net flux between the biosphere and atmosphere due to CO2 uptake during vegetation photosynthesis and CO2 release during respiration. This exchange also includes CO2 releases due to natural and anthropogenic disturbances, such as emissions contributed by fire or conversions in land use (if any). Land-use conversion and fire contributions are specific to the terrestrial biosphere model (TBM) used here (e.g., see detailed comparison of structural differences among TBMs in Huntzinger et al. Citation2013). NEE simulated by current TBMs is still highly uncertain, which could be attributable to variations in inputs to the models (e.g., climate forcing and model parameters) and model structure (e.g., the model’s capability of capturing important processes, such as CO2 fertilization, nitrogen limitation, and disturbances). A major endeavor of carbon-cycle research has involved intercomparison and evaluations of terrestrial-biosphere models (e.g., Huntzinger et al., Citation2012, Citation2013; Schaefer et al., Citation2012; Schwalm et al., Citation2010), and no model is obviously superior to others in all aspects. Efforts using atmospheric CO2 observations to constrain NEE fluxes have increased in recent years. NOAA’s CarbonTracker model (Peters et al., Citation2007) is an example of a data-assimilation system that provides optimized biosphere and ocean CO2 fluxes using in situ CO2 observations from a global observational network.
We used three different sets of NEE fluxes as input to CMAQ, including (1) NEE from the Carnegie–Ames Stanford Approach (CASA), which are used by CarbonTracker-2011 (CT2011, http://carbontracker.noaa.gov) as priors (1˚ × 1˚, 3-hourly), and hereafter denoted by CASA NEE); (2) CT2011 optimized NEE (1˚ × 1˚, 3-hourly, hereafter denoted by CT2011 NEE), which represents an improved estimate over CASA NEE, as the result of imposing observational constraints; and (3) NEE from the Community Land Model (Version 4) with surface and subsurface runoff parameterizations from the Variable Infiltration Capacity (VIC) model (CLM4VIC, the baseline global simulation, i.e., BG1 simulation; Li et al., Citation2011) (0.5˚ × 0.5˚, 3-hourly, hereafter denoted by CLM4VIC NEE) following protocols for the North American Carbon Program (NACP) Multi-Scale Synthesis and Terrestrial Model Intercomparison (MsTMIP) project (http://nacp.ornl.gov/MsTMIP.shtml; Huntzinger et al., Citation2013; Wei et al., Citation2013). More details of the CLM4VIC BG1 simulation are provided in the supplemental information. NEE from CT2011 and CASA do not include fire emissions. CLM4VIC NEE includes fire emissions, but the contribution to NEE in October 2007 is small.
shows the monthly mean NEE fluxes from CT2011, CASA, and CLM4VIC over the model domain for October 2007. During this nongrowing (fall) season, the biosphere acts as a net source of CO2 in terms of total fluxes over the model domain and the contiguous United States, as consistently shown by the three sets of NEE (). A transition from a net source in the north to a net sink in the south can be seen in both CT2011 () and CASA (). The spatial distribution of CLM4VIC NEE is distinctly different. shows a dipole structure in the central and southeastern United States, and the total flux is only less than a half of the former two CASA-derived NEE fluxes. We note that such intermodel discrepancies of NEE observed here are not surprising, in comparison with previous biosphere-model intercomparison studies (e.g., Huntzinger et al., Citation2012; Schaefer et al., Citation2012). Differences between the CLM4VIC and CASA models include, but are not limited to, land cover and land-use history, meteorological input data, and resolution of model biogeophysical and biogeochemical parameterizations and representations. For example, plant phenology is constrained by satellite observed Normalized Difference Vegetation Index (NDVI) in CASA, but is simulated prognostically in CLM4VIC. All these differences could contribute to the differences in spatial patterns and domain total fluxes. Fully understanding the effects of such model discrepancies would require a detailed comparison of the algorithms and input data used in CASA and CLM4VIC, which is outside the scope of this work. Instead, such intermodel differences of NEE from these three representative data sets can be employed as a rough estimate of uncertainties of NEE predicted by current TBMs. The differences of model-simulated atmospheric CO2 concentrations, as a result of using these three different NEE inputs, will provide a rough estimate of the CO2 uncertainty caused by uncertainty in NEE (Huntzinger et al., Citation2011; Messerschmidt et al., Citation2012). We note that although only spatial distributions are shown in , uncertainty of temporal variability of NEE as an important factor has also been taken into account by using these three NEE inputs.
Table 1. CO2 fluxes used in CMAQ simulations
Fossil-fuel, fire, and ocean fluxes
Two fossil-fuel emissions inventories are used in this work. In our standard model configuration (), for model grids within the United States, we use the Vulcan fossil-fuel emissions inventory (Gurney et al., Citation2009). The Vulcan inventory is a well-documented high-resolution (10-km grid spacing, hourly temporal resolution), process driven, and fuel-specific fossil-fuel CO2 emissions inventory compiled for the United States. In the Vulcan inventory, eight emissions sectors are taken into account, that is, aircraft, cement, commercial, industrial, nonroad, on-road, residential, and electricity production. Nonroad, nonpoint, point, and airport emissions activity data are taken from the U.S. Environmental Protection Agency (EPA) National Emissions Inventory (NEI) (for the year of 2002), which is a comprehensive inventory of all criteria air pollutants (CAPs) and hazardous air pollutants (HAPs) across the United States. Data from the EPA Emissions tracking system/continuous emissions monitoring systems (ETS/CEMs) are used for electricity production emissions. National Mobile Inventory Model (NMIM) County Database (NCD) data are used for deriving on-road CO2 emissions. AERO2K data are used for aircraft emissions (Eyers et al., Citation2004). Data for Portland cement are used for deriving CO2 emissions from cement production. More detailed information about the Vulcan inventory can be found by referring to Gurney et al. (Citation2009) and the website of the Vulcan project (http://vulcan.project.asu.edu, accessed January 14, 2013). Since the Vulcan inventory is compiled for the year of 2002 and there is a notable weekday/weekend effect in the data (McKain et al., Citation2012), we shifted the days in Vulcan such that the weekday/weekend patterns match the dates in 2007.
Table 2. List of model sensitivity experiments
For model grids outside the United States, where Vulcan does not have values, we used an emissions inventory for 2007 from the Carbon Dioxide Information Analysis Center (CDIAC), a widely used global emissions inventory (1˚ × 1˚, monthly) in global CO2 modeling (Andres et al., Citation2011). The Vulcan inventory is based on NEI-2002 and is used here to investigate the advantages of using an inventory with high spatial and temporal resolution. In principle, CO2 emissions can be processed together with other pollutants using an emissions processor, for example, the Sparse Matrix Operator Kernel Emissions (SMOKE), based on the U.S. EPA NEI, in future CO2 regional modeling studies.
shows the monthly mean fossil-fuel emissions from Vulcan and CDIAC inventories in the model domain for October 2007. The differences between Vulcan and CDIAC reflect different spatial resolutions, spatial allocation methods, and reference years of the two inventories (2002 for Vulcan and 2007 for CDIAC). The two inventories, although compiled for different years, have similar (within 3%) total emissions in the contiguous United States (), reflecting the small interannual variability of national CO2 emissions in the U.S. in the latest decade (Boden et al., 2012). Another key difference between the two inventories is that Vulcan takes into account temporal variations while CDIAC does not. A comparison of and reveals that NEE shares characteristics with area sources, that is, relatively little spatial variability and small gradients, whereas fossil-fuel emissions are dominated by point sources and show considerable spatial heterogeneity and large gradients. By comparing CO2 simulations using these two emissions inventories against observations, we examined the benefit of using a high-resolution emissions inventory like Vulcan.
Figure 2. Monthly mean fossil-fuel CO2 emissions for October 2007 from (a) Vulcan and (b) CDIAC in the model domain.
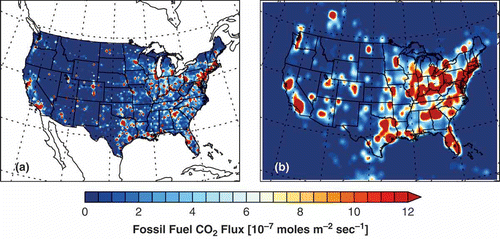
For fire emissions, GFED (0.5˚ × 0.5˚; monthly) inventory (as used in CT2011) is used. Fire emissions are highly variable in space and time, but are of minor importance compared to fossil-fuel emissions and to biosphere–atmosphere exchange for the simulation month (). However, they are likely to be more important during active fire months and regions. Fire emissions are turned off when CLM4VIC NEE is used, as it already includes fire emissions. The effects of fire will be examined in future by using different fire emissions algorithms such as GFEDv3.1 (0.5˚ × 0.5˚; 3-hourly; http://www.globalfiredata.org/Data/index.html, accessed January 14, 2013) and SmartFire.
We used CT2011 optimized estimates for ocean fluxes (1˚ × 1˚; 3-hourly), which are of minor importance compared to the biospheric and fossil-fuel fluxes ().
CO2 net fluxes
It is instructive to examine the net CO2 fluxes as a result of the sum of all four types of fluxes (i.e., fossil fuel, bisospheric, fire, and ocean). The spatial pattern of the CO2 net flux shown in retains features from NEE (regional pattern) and fossil-fuel emissions (scattered hot spots) because of their comparable flux magnitudes in this month ( and ). A number of CO2 emissions hotspots shown in Vulcan () are still clearly seen in , such as the Los Angeles (LA) Basin and San Francisco Bay Area along the West Coast, and the Houston–Galveston–Brazoria area along the Gulf Coast. Many smaller and weaker emission sources in , especially those in the eastern United States., do not appear as clearly because of comparable or larger NEE flux. Neutral (zero) and negative net fluxes are seen in the southwestern United States and the Mexican mainland, with all three sets of NEE, as a result of negative NEE and small fossil-fuel emissions fluxes in these areas. When CLM4VIC NEE is used, negative net fluxes are also observed throughout the central United States and in many areas of the intermountain West.
Figure 3. Sensitivity of monthly mean net CO2 fluxes to choice of NEE, (a) CT2011 for VCT (“our standard model”), (b) CASA for VCS, and (c) Community Land Model 4VIC-BG1 for VCL. Fossil-fuel emissions (Vulcan inside the United States and CDIAC outside), fire emissions (GFED), and ocean fluxes (CT2011) are the same for the three model configurations.
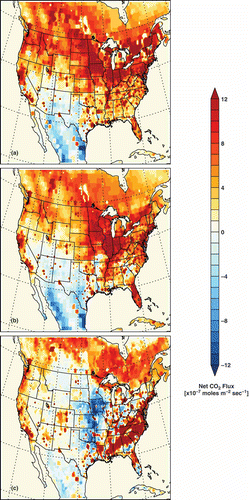
The differences in NEE models, as shown in , lead to different spatial patterns of net fluxes in , , and . Much smaller differences between models are found along the West Coast than in the eastern United States. shows a large net CO2 sink in the central United States and a strong net CO2 source in the southeastern United States with CLM4VIC NEE, which are not seen with NEE from CASA or CT2011. In turn, the fossil-fuel emissions signals in the southeastern United States reflected in the net fluxes with CT2011 () and CASA () NEE are not seen with CLM4VIC (). As shown in later sections, such different net fluxes due to the differences in these three NEE inputs indeed lead to different spatial patterns of CO2 near the surface simulated by CMAQ. The differences of net fluxes in can facilitate understanding the interference by the uncertainty of NEE with the interpretation of CO2 simulations and observations.
Initial and boundary conditions
Given the long atmospheric lifetime of CO2, concentrations simulated by a regional model like CMAQ are expected to be sensitive to model initial conditions (IC) and boundary conditions (BC), as has been shown by previous studies (Chevillard et al., Citation2002). Four-dimensional concentration output from CT2011 using optimized NEE and ocean fluxes at 3˚ × 2˚ and 3-hourly resolution were used as lateral and top BC in CMAQ. To minimize the impact of IC uncertainty, we spun up the model for 10 days. An experiment replacing 3-hourly BC with constant BC profiles shows large impact over the whole domain. The sensitivity of the simulation to IC/BC can be assessed in future work using the DDM-3D technique with CMAQ (Hakami et al., Citation2004).
Model experiments
We performed simultaneous simulations of CO2 and a full suite of default chemical species in CMAQ for October 2007. Two sets of model simulations were conducted. The first set was designed for evaluating the roles of different sources/sinks of CO2 in regulating the CO2 spatial distribution by decomposing CO2 into three components, that is, the background, biosphere, and fossil-fuel components. Specifically, we defined the region of interest to be the contiguous United States. The background component was defined as CO2 concentrations as a result of transport, fire, and ocean fluxes over the whole domain, and fossil-fuel emissions outside the United States. The biosphere (or fossil-fuel) component was defined to be CO2 due to NEE (or fossil-fuel emissions) within the United States domain in this month. A second set of simulations was performed to assess the impact of NEE uncertainty on simulated CO2 concentrations by comparing model results using three different NEE inputs.
Configurations of the seven model runs for these experiments are tabulated in . The key to model notation for simulations 1 to 4 is as follows: A three-letter code is used, in which the first letter refers to the source of fossil fuel input in the United States (V for Vulcan, C for CDIAC). The next two letters refer to the source of NEE input (CT for CT2011, CS for CASA, and CL for Community Land Model [CLM]). These simulations consist of (1) our standard model (VCT) using CT2011 optimized NEE and GFED fire emissions over the entire domain, CDIAC fossil-fuel emissions outside the United States, and Vulcan fossil-fuel emissions within the United States; (2) a CDIAC run (DCT) that differs from VCT by replacing Vulcan emissions with CDIAC emissions within the United States; (3) a CASA run (VCS) that differs from VCT by replacing CT2011 NEE with CASA NEE; (4) a run (VCL) that differs from VCT by replacing CT2011 NEE with NEE from the Community Land Model (v. 4); (5) a background run (BG) that differs from VCT by turning off NEE and fossil-fuel fluxes within the United States; (6) a biosphere run (BIO) that differs from VCT by turning off fossil-fuel emissions within the United States; and (7) a fossil-fuel run (FF) that differs from VCT by turning off NEE in the United States. The biosphere component of the net flux of CO2 in the United States was obtained by subtracting concentrations in BG from BIO, and the fossil-fuel component was obtained by subtracting BG from FF.
CO2 observations from NOAA ESRL tall towers
As a component of the NOAA ESRL global sampling network, CO2 has been continuously measured at a network of six tall-tower sites across the contiguous United States (Andrews et al., Citation2009). These in situ near-surface CO2 data have been extensively used for carbon-cycle research, and are assimilated by CarbonTracker (Peters et al., Citation2007). The majority of the tall-tower sites are located in remote areas with insignificant influences from local fossil-fuel emissions. However, such local fossil-fuel emissions of CO2 are of interest to the emissions-verification problem. CO2 data are available from all six tall-tower sites in the model domain for October 2007. Information (location, elevation, and sampling altitude) of these sites is given in Table S2. Five of the six sites are far from fossil-fuel emissions sources, and thus the observed CO2 variability at these sites is mostly driven by long-range transport and more local biospheric fluxes. The Boulder Atmospheric Observatory (BAO) (40.05˚ N, 105.00˚ W, 300 m above ground, 1584 m elevation) is a unique site that frequently receives local emissions from Denver, CO. For this reason, the data from BAO in this month were not assimilated by CT2011 to avoid an artificial scaling factor applied to a larger region due to the misrepresentation of local emissions impact by the global model TM5. In this work, we compared model-simulated CO2 with observations from the six tall tower sites to evaluate general model performance. In particular, we elaborate on the comparison for BAO to understand the underlying factors driving the observed variability of CO2 at a site that is influenced by fossil-fuel emissions from a city.
Results and Discussions
Spatial distribution of CO2 near the surface over the contiguous United States
From CarbonTracker to CMAQ
shows the monthly mean spatial distributions of CO2 from CT2011 () and two CMAQ simulations, that is, DCT in and VCT in . DCT and CT2011 show similar large-scale patterns in general, but large differences are apparent between the two (as large as 15–20 ppmv), particularly along the western coast of the Mexican mainland. DCT uses CT2011 CO2 outputs as initial and boundary conditions, and is driven by the same set of CO2 fluxes as used by CT2011 (). Therefore, differences between the results from DCT () and CT2011 () can be attributed to the differences of model transport in CMAQ and CT2011, in the following key aspects: (1) assimilated meteorological fields (WRF for CMAQ/DCT versus EWF forecast for TM5/CT2011), (2) model resolution (36 km × 36 km for DCT versus 1˚ × 1˚ or ∼85 km × ∼111 km for CT2011), and (3) transport representations (CMAQ versus TM5). As expected, the higher spatial resolution of DCT allows for resolving fine-scale features that are not seen in CT2011. Furthermore, by replacing the CDIAC inventory in DCT with the Vulcan inventory, which has much higher spatial and temporal resolution, VCT simulates numerous hot spots and stronger spatial heterogeneity of CO2, while retaining the synoptic-scale spatial pattern in DCT. Overall, demonstrates that, compared to CT2011, the much-refined descriptions of transport and emissions in CMAQ allow for more detailed characterization of the spatial distribution of CO2. A spatial map of CO2, as shown in , can facilitate the interpretation of sparse observational data in a regional context. In the next section, we discuss the roles of meteorology, biosphere, and fossil-fuel emissions in shaping the spatial pattern of CO2 simulated by CMAQ in the United States by examining the contributions to CO2 from these components individually.
Decomposition of CO2 into background, biosphere, and fossil-fuel components
shows the background, biosphere, and fossil-fuel components of CO2 simulated by CMAQ, using the model configurations shown in . As can be seen in , a relatively uniform background CO2 of 380–383 ppmv over most places in the country in October 2007 is simulated by the background run in the absence of biospheric and fossil-fuel fluxes in the contiguous United States. However, discernible gradients in CO2 are still seen in the northeastern and western United States. In , the biosphere, as a net source (positive NEE) of CO2 in this month, is responsible for prevalent CO2 enhancement in the United States on top of the background in . Weaker CO2 enhancement is seen in the Southwest because of lower NEE fluxes, and depletion of CO2 (up to more than 4 ppmv) occurs in central Texas and, to a lesser degree, in the Los Angeles (LA) Basin. In comparison, the fossil-fuel component in exhibits a similar spatial pattern to the biosphere component with some marked differences. Numerous domes of CO2 (>16 ppmv) form near large emissions sources (as shown in ). Dispersion of CO2 from these domes and from smaller emissions sources result in an increase of 2–4 ppmv of CO2 superimposed on the background. Comparing and suggests that (1) in areas far away from large fossil-fuel emissions sources, the biosphere component is similar to or even higher than the fossil-fuel component, and (2) the biosphere component in the majority of cities cannot be regarded as negligible, with the possible exception of some urban areas, for example, the LA Basin in October 2007. The decomposition of CO2 into biosphere and fossil-fuel components also facilitates the interpretation of CO2 distribution shown in . For example, clearly shows that NEE is the main contributor to the high CO2 in central Pennsylvania. The biosphere CO2 component is expected to vary significantly with season, however, and thus its contribution to atmospheric CO2 is expected to change with season as well.
Sensitivity of CO2 spatial distribution to uncertainty in NEE
All the results discussed thus far are from simulations using CT2011 NEE as input. In this subsection, we examine the impact of uncertainty of NEE on the spatial patterns of CO2. compares monthly mean spatial distributions of CO2 near the surface simulated by VCT, VCS, and VCL, which use the same fossil-fuel emissions input but different NEE (). A comparison among , , and reveals considerable model discrepancies as a result of differences in NEE inputs, consistent with a recent inverse modeling study using synthetic data at a few tower sites over the contiguous United States (Huntzinger et al. Citation2011). The regional mean concentration of CO2 is lower in VCL () than in the other two models, consistent with its overall lower NEE (). CO2 domes in the southeastern United States shown in VCT () diminish in both VCS and VCL ( and ). In contrast, CO2 concentrations along the West Coast do not vary much with different NEE inputs, implying smaller effects of uncertainty in NEE. Such regionally varied situations imply that conclusions drawn from studies at one locale (e.g., in the LA Basin) need to be reexamined when looking at another locale (e.g., Washington, DC, or Atlanta, GA). The uncertainty of NEE as reflected by intermodel differences has been found to be seasonally dependent (e.g., Huntzinger et al., Citation2011). Therefore, the conclusions drawn here from one month of simulation cannot be seen as representative for all seasons. More comprehensive model comparisons for all seasons using NEE outputs from a larger group of TBMs are needed in the future to better understand the issue of NEE uncertainty and biospheric interference.
Comparison with tall-tower measurements
In this section, we examine the model results against observations at the six NOAA ESRL tall-tower sites listed in Table S2 to evaluate and understand CMAQ-simulated temporal variations of CO2. Figures S1 through S6 show the CO2 time series and mean diurnal profiles from CMAQ and CT2011 simulations, compared to the associated observations at the six sites. CT2011 has perturbed NEE fluxes to match CO2 observations at five of the six sites (except for BAO). In general, CMAQ models using CASA/CT2011-derived NEE fluxes (DCT, VCT, and VCS) show better performance than the model using the Community land Model 4VIC NEE (VCL). Compared to CT2011, the model using CASA-derived NEE (VCS) can simulate better monthly mean concentrations (as suggested by the reduced mean biases) and resolve more high-frequency variability (as suggested by the closer-to-unity ratios of standard deviations) at most of the sites. However, these CMAQ models do not always show higher correlations with observations or lower root-mean-square error (RMSE) than CT2011, suggesting that switching to new transport and fluxes at higher resolution also introduces more model-data mismatches. The mean diurnal profiles in Figures S1–S6 show that almost all the CMAQ models show a low bias compared to observations at night through early morning. Possible reasons include, but are not restricted to, errors in model transport in the boundary layer and emissions temporal profiles.
As mentioned earlier, BAO is unique and of higher interest compared to the other five sites because (1) it receives fresh fossil-fuel emissions from Denver, CO, and (2) the observations were not assimilated by CT2011 in this month. Figure S1 show that all the CMAQ models have improved performance over CT2011 in reproducing the 3-hourly observed CO2 concentrations at BAO. CT2011-simulated CO2 roughly tracks the observed background (lowest observed level) and shows negligible diurnal variability, possibly because of the smoothed local topography with the coarse grid of TM5 (the global model used for CT2011) and diluted emissions in CDIAC near BAO. shows that CMAQ-simulated diurnal profiles are in general stronger than CT2011, but also vary with different NEE and/or fossil-fuel emissions. The standard model (VCT) with CT2011-optimized NEE and Vulcan fossil-fuel emissions shows the best agreement with observation in terms of both 3-hourly and mean diurnal variability, but also has a low bias in early morning, as found for all other CMAQ models at all sites. DCT, which uses the same fluxes as CT2011 but higher resolution meteorology, simulates a slightly stronger diurnal variability than CT2011. Using the hourly varying Vulcan emissions (VCT, VCS, and VCL), which resolve the morning rush-hour emissions peak, helps to capture the observed morning peak around 8:00 a.m. By switching to different NEE inputs, VCS and VCL simulate lower CO2 concentrations in general than VCT. These results suggest that both time-varying emissions and biospheric fluxes are important drivers of the 3-hourly and diurnal variability of CO2 at BAO. The importance of time-varying emissions was recently demonstrated by a recent modeling study, which shows that diurnal and weekly variations of emissions could result in up to 8 ppmv of perturbations of CO2 near the surface (Nassar et al., Citation2013).
Figure 7. Monthly mean diurnal profiles of CO2 in October 2007 observed at Boulder Atmospheric Observatory (BAO) (TOWER) and simulated by CT2011 and CMAQ with different configurations, DCT, VCT, VCS, and VCL.
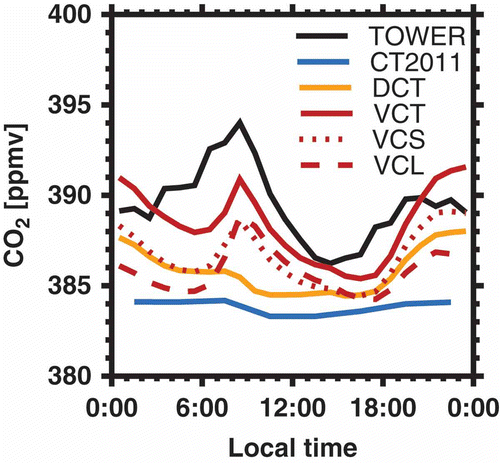
It is very important to understand the causes for the model–data mismatch to guide subsequent inverse modeling. Since inverse modeling essentially seeks to match observations by perturbing selected model fluxes, an incorrect attribution of the model–data mismatch would directly lead to erroneous inversion results (e.g., Ahmadov et al., Citation2007). For instance, attributing the early-morning low bias to errors in fossil-fuel emissions, or NEE, or model transport would lead to drastically different conclusions (i.e., scaling up emissions in the first vs. no scaling in the latter two). Indeed, our model results illustrate that emissions verification is confounded by factors that affect CO2 concentrations simultaneously with emissions, such as transport and biospheric fluxes. Alternatively, fossil-fuel emissions could be isolated using certain tracer or proxy techniques.
Spatial correlations between CO2 and traditionally regulated pollutants
Another question that can be conveniently addressed with CMAQ is the feasibility of using traditionally regulated air pollutants, such as CO, NOx, and SO2, to provide constraints for fossil-fuel CO2 (e.g., Brioude et al., Citation2012). For these traditionally regulated air pollutants, there are abundant long-term ground-based and satellite monitoring data that contain valuable information of historical trends and spatial patterns of emissions. Effective and economical strategies for emissions verification are urgently needed to ensure the success of near-term emissions reductions (McKain et al., Citation2012; NRC, 2010). It is thus worthwhile to consider approaches that (1) take full advantage of currently available observational networks and experiences in air pollutant emissions monitoring and reduction, and (2) combine state-of-the-art atmospheric transport and emissions modeling techniques. It has been shown recently that concurrent measurements of CO2, CO, NOy, and SO2 can be used to derive a “top-down” estimate of CO2 emissions from a city (e.g., Brioude et al., Citation2012). The emissions trend of CO2 over China was recently inferred from satellite NO2 columns (Berezin et al., Citation2013). CO:CO2 correlation slopes from aircraft observations during TRACE-P were used for understanding model–data mismatches and constraining emissions fluxes (Suntharalingam et al., Citation2004).
compares the CMAQ-simulated monthly mean spatial patterns of CO2, SO2, NOx, and CO. Fossil-fuel combustion is the largest source for all four compounds, although emissions factors for their common source sectors are different, and each of them has its unique sources and sinks. In terms of spatial distributions, CO2 correlates better with NOx (R = 0.63) and CO (R = 0.61) than with SO2 (R = 0.38). These correlations of concentrations are slightly better than the correlations of emissions of these compounds (R = 0.43 for CO2:NOx, R = 0.4 for CO2:CO, and R = 0.24 for CO2:SO2), reflecting that, in addition to similar emissions sources, transport processes also contribute to the similar spatial patterns observed in . Using emissions of CO2 and tracer species for different years and regridding the Vulcan inventory to the model resolution might have degraded the correlations between CO2 and the three pollutants. A consistent processing procedure for emissions of CO2 and its tracers is necessary for future studies. CO2 hot spots (e.g., the CO2 hot spot in central Pennsylvania and the broad high-CO2 region in the central and northern United States) arising primarily from biospheric fluxes can be readily identified with the assistance of the three tracers that are mainly emitted in urban areas (with the possible exception of electricity generating units, which can be located in rural areas). A quantitative understanding of such correlations and their utility to CO2 emissions inference needs to be attained by taking into account (1) emissions factors and activities for each individual source (e.g., Palmer et al., Citation2006; Berezin et al., 2012) and (2) model-simulated transport (Wang and Zeng, Citation2004) and model errors (Wang et al., Citation2009). The tracer correlation problem has been studied extensively in the stratosphere (e.g., Michelsen et al., Citation1998; Plumb et al., 2007). Modeling and observations need to be combined to better understand the characteristics of tracer correlations in the troposphere. Correlations on different dimensions, for example, a one-dimensional (1-D) temporal correlation from a single ground site (e.g., Vogel et al., Citation2010), a two-dimensional (2-D) spatial correlation shown in , or a four-dimensional (4-D) spatiotemporal correlation from aircraft measurements (e.g., Brioude et al., Citation2012; Palmer et al., Citation2006), convey different physical meanings and should be examined and used carefully. Incorporating CO2 into a positive matrix factorization (PMF) analysis with multiple tracers (possibly including both gaseous species and PM2.5 components) could also be considered to aid the tracking of CO2 from different sources. Another possible direction is to explore a joint CO2:tracer flux inversion (Wang et al., Citation2009) that makes use of the correlation of model errors between CO2 and a tracer.
Summary
In this work, we have described the motivation and methods for simulating CO2 with CMAQ and have presented initial modeling results for the contiguous United States in October 2007 to examine the potential of using CMAQ to characterize CO2 spatial and temporal variability.
- By decomposing CMAQ-simulated CO2 into background, biosphere, and fossil-fuel components, we have found that biospheric fluxes and fossil-fuel emissions are comparably important in shaping spatial distributions of CO2 near the surface over the contiguous United States during October 2007, with each component showing its unique characteristics.
- By using three different sets of NEE as inputs, we have shown that the uncertainty of NEE estimates has considerable impact on model-simulated atmospheric CO2 concentrations near the surface, a finding that is consistent with previous studies (e.g., Huntzinger et al., Citation2011). While only three sets of NEE inputs from two different TBMs are used here, in future work additional TBM outputs from model intercomparison projects (e.g., the ongoing MsTMIP project) will be used to comprehensively address the issue of NEE uncertainty.
- By comparing the model results with observations from six tall-tower sites in the NOAA ESRL network, we have evaluated the model-simulated 3-hourly and diurnal temporal variability of CO2. In particular, at BAO near Denver, CO, the model using the Vulcan emissions and CT2011 NEE shows the best performance in matching the observed mean diurnal profile, although with a low bias in the early morning. Using different NEE inputs degrades the model–data agreement. More work is needed to better understand the model–data mismatch and inform subsequent inverse modeling.
- The model-simulated spatial pattern of CO2 near the surface shows varying degrees of correlations with NOx, CO, and SO2 as a result of their similar emission sources and common transport processes. Future work will explore the utility of these tracers for constraining fossil-fuel CO2 emissions.
Findings from this work serve as a proof of concept and suggest that a regional CTM like CMAQ has the potential to facilitate interpretation of CO2 observations and emissions verification. Future work will improve CMAQ CO2 simulations in the following aspects: (1) increasing the model spatial resolution to better resolve urban and point sources, (2) processing gridded CO2 emissions using SMOKE, (3) developing inline simulation of bidirectional biospheric fluxes, and (4) comprehensively evaluating the model performance using observations from ground networks, aircrafts, and satellites for all seasons of a year. Other greenhouse gases can also be studied using CMAQ in a manner similar to that shown here.
Funding
The work at Sandia National Laboratories was funded by the Sandia Laboratory Directed Research and Development (LDRD) Program. Sandia is a multiprogram laboratory operated by Sandia Corporation, a Lockheed Martin Company, for the National Nuclear Security Administration under contract DE-AC04-94-AL85000. The WRF output and non-CO2 emission data are products of the Southeastern Modeling, Analysis, and Planning (SEMAP) project (http://www.metro4-sesarm.org; accessed January 14, 2013). The CLM4VIC output is a product of the MsTMIP project. The MsTMIP project has been funded by the National Aeronautics and Space Administration (NASA) under grants NNX11AO08A and NNH10AN68I, and is a contribution of the North American Carbon Program. PNNL is operated for the U.S. Department of Energy (DOE) by Battelle Memorial Institute under contract DE-AC06-76RLO1830.
Supplementary Data
Supplemental data are available for this paper. Go to the publisher’s online edition of the Journal of the Air & Waste Management Association.
Supplemental Material
Download MS Word (1.4 MB)Acknowledgments
The authors are very grateful to Drs. Andrew Jacobson, Anna Michalak, Kevin Schaefer, Bart Van Bloemen Waanders, Habib Najm, Cosmin Safta, and Ling Jin for their extensive comments and suggestions. The information in this article has been subjected to review by the National Center for Environmental Assessment, U.S. Environmental Protection Agency, and approved for publication. The authors thank Arlyn Andrews and the NOAA GMD for making the tower CO2 data public, and thank NOAA ESRL for making the CarbonTracker 2011 results public. CarbonTracker 2011 results were provided by NOAA ESRL, Boulder, CO, from the website at http://carbontracker.noaa.gov. The authors thank Kevin Gurney and the Vulcan project for generating and sharing the Vulcan emission inventory (http://vulcan.project.asu.edu).
Additional information
Notes on contributors
Zhen Liu
Zhen Liu is a postdoctoral appointee at Sandia National Laboratories, Livermore, CA.
Ray P. Bambha
Ray P. Bambha is a staff scientist at Sandia National Laboratories, Livermore, CA.
Joseph P. Pinto
Joseph P. Pinto is a research scientist at U.S. EPA, Research Triangle Park, NC.
Tao Zeng
Tao Zeng is a staff scientist and Jim Boylan is a staff scientist and manager at Georgia Department of Natural Resources, Atlanta, GA.
Maoyi Huang
Maoyi Huang and Chun Zhao are staff scientists at Atmospheric Sciences and Global Change Division, Pacific Northwest National Laboratory, Richland, WA.
Huimin Lei
Huimin Lei is a professor in the Department of Hydraulic Engineering, Tsinghua University, Beijing, China.
Shishi Liu
Shishi Liu is a postdoctoral research associate at Oak Ridge National Laboratory, Oak Ridge, TN.
Christopher R. Schwalm
Christopher R. Schwalm is an assistant professor at School of Earth Sciences and Environmental Sustainability, Northern Arizona University, Flagstaff, AZ.
Yaxing Wei
Shishi Liu and Yaxing Wei are postdoctoral research associates, and Jiafu Mao and Xiaoying Shi are staff scientists at Oak Ridge National Laboratory, Oak Ridge, TN.
Hope A. Michelsen
Hope A. Michelsen is a staff scientist at Sandia National Laboratories, Livermore, CA.
References
- Ahmadov, R., C. Gerbig, R. Kretschmer, S. Köerner, B. Neininger, A.J. Dolman, and C. Sarrat. 2007. Mesoscale covariance of transport and CO2 fluxes: Evidence from observations and simulations using the WRF-VPRM coupled atmosphere-biosphere model. J. Geophys. Res. Atmos. 112:D22. doi:10.1029/2007jd008552
- Ahmadov, R., C. Gerbig, R. Kretschmer, S. Köerner, C. Rödenbeck, P. Bousquet, and M. Ramonet. 2009. Comparing high resolution WRF-VPRM simulations and two global CO2 transport models with coastal tower measurements of CO2. Biogeosciences 6:807–17. doi:10.5194/bg-6-807-2009
- Andres, R.J., J.S. Gregg, L. Losey, G. Marland, and T.A. Boden. 2011. Monthly, global emissions of carbon dioxide from fossil fuel consumption. Tellus B 63:309–27. doi:10.1111/j.1600-0889.2011.00530.x
- Andres, R.J., T.A. Boden, F.M. Bréon, P. Ciais, S. Davis, D. Erickson, J.S. Gregg, A. Jacobson, G. Marland, J. Miller, T. Oda,et al. 2012. A synthesis of carbon dioxide emissions from fossil-fuel combustion. Biogeosciences 9:1845–71. doi:10.5194/bg-9-1845-2012
- Andrews, A.E., J. Kofler, P. S. Bakwin, C. Zhao, and P. Tans. 2009. Carbon dioxide and carbon monoxide dry air mole fractions from the NOAA ESRL Tall Tower Network. 1992–2009. Version: 2011-08-31. Path: http://ftp://ftp.dl.noaa.gov/ccg/towers (accessed January 14, 2012).
- Andrews, A.E., J.D. Kofler, M.E. Trudeau, J.C. Williams, D.H. Neff, K.A. Masarie1, D.Y. Chao, D.R. Kitzis, P.C. Novelli, C.L. Zhao,et al. 2013. CO2, CO and CH4 measurements from the NOAA Earth System Research Laboratory’s Tall Tower Greenhouse Gas Observing Network: Instrumentation, uncertainty analysis and recommendations for future high-accuracy greenhouse gas monitoring efforts. Atmos. Mech. Tech. Discuss. 6:1461–553. doi:10.5194/amtd-6-1461-2013
- Berezin, E.V., I.B. Konovalov, P. Ciais, A. Richter, S. Tao, G. Janssens-Maenhout, M. Beekmann, and E.D. Schulze. 2013. Multiannual changes of CO2 emissions in China: Indirect estimates derived from satellite measurements of tropospheric NO2 columns. Atmos. Chem. Phys. Discuss. 13:255–309. doi:10.5194/acpd-13-255-2013
- Boden, T.A., G. Marland, and R.J. Andres. 2011. Global, Regional, and National Fossil-Fuel CO2 Emissions. Carbon Dioxide Information Analysis Center, Oak Ridge National Laboratory, U.S. Department of Energy, Oak Ridge, TN. doi:10.3334/CDIAC/00001_V2011
- Brioude, J., G. Petron, G.J. Frost, R. Ahmadov, W.M. Angevine, E.Y. Hsie, S.W. Kim, S.H. Lee, S.A. McKeen, M. Trainer,et al. 2012. A new inversion method to calculate emission inventories without a prior at mesoscale: Application to the anthropogenic CO2 emission from Houston, Texas. J. Geophys. Res. Atmos. 117:D05312. doi:10.1029/2011jd016918
- Byun, D., and K.L. Schere. 2006. Review of the governing equations, computational algorithms, and other components of the models-3 Community Multiscale Air Quality (CMAQ) modeling system. Appl. Mech. Rev. 59:51–77. doi:10.1115/1.2128636
- Campbell, J.E., G.R. Carmichael, T. Chai, M. Mena-Carrasco, Y. Tang, D.R. Blake, N.J. Blake, S.A. Vay, G.J. Collatz, I. Baker,et al. 2008. Photosynthetic control of atmospheric carbonyl sulfide during the growing season. Science 322:1085–88. doi:10.1126/science.1164015
- Canadell, J.G., C. Le Quere, M.R. Raupach, C.B. Field, E.T. Buitenhuis, P. Ciais, T.J. Conway, N.P. Gillett, R.A. Houghton, and G. Marland. 2007. Contributions to accelerating atmospheric CO2 growth from economic activity, carbon intensity, and efficiency of natural sinks. Proc. Natl. Acad. Sci. USA 104:18866–70, doi:10.1073/pnas.0702737104
- Chevillard, A., U. Karstens, P. Ciais, S. Lafont, and M. Heimann. 2002. Simulation of atmospheric CO2 over Europe and western Siberia using the regional scale model REMO. Tellus Ser. B Chem. Phys. Meteorol. 54:872–94. doi:10.1034/j.1600–0889.2002.01340.x
- Cohan, D.S., A. Hakami, Y.T. Hu, and A.G. Russell. 2005. Nonlinear response of ozone to emissions: Source apportionment and sensitivity analysis. Environ. Sci.Technol. 39:6739–48. doi:10.1021/es05664m
- Dunker, A.M. 1984. The decoupled direct method for calculating sensitivity coefficients in chemical-kinetics. J. Chem. Phys. 81:2385–93. doi:10.1063/1.447938
- Eyers, C.J., P. Norman, J. Middel, M. Plohr, S. Michot, K. Atkinson, R.A. Christou. 2004. AERO2k Global Aviation Emissions Inventories for 2002 and 2025. Center for Air Transport and the Environment, QINETIQ/04/01113. http://aero-net.info/fileadmin/aeronet_files/links/documents/AERO2K_Global_Aviation_Emissions_Inventories_for_2002_and_2025.pdf
- Guan, D.B., Z. Liu, Y. Geng, S. Lindner, and K. Hubacek. 2012. The gigatonne gap in China’s carbon dioxide inventories. Nat. Climate Change 2:672–75. doi:10.1038/nclimate1560
- Gurney, K.R., R.M. Law, A.S. Denning, P.J. Rayner, D. Baker, P. Bousquet, L. Bruhwiler, Y.-H. Chen, P. Ciais, S. Fan,et al. 2002. Toward robust regional estimates of CO2 sources and sinks using atmospheric transport models. Nature 415:626–30. doi:10.1038/415626a
- Gurney, K.R., D.L. Mendoza, Y.Y. Zhou, M.L. Fischer, C.C. Miller, S. Geethakumar, and S.D. Du Can. 2009. High resolution fossil fuel combustion CO2 emission fluxes for the United States. Environ. Sci. Technol. 43:5535–41. doi:10.1021/es900806c
- Hakami, A., M.T. Odman, and A.G. Russell. 2004. Nonlinearity in atmospheric response: A direct sensitivity analysis approach. J. Geophys. Res. Atmos. 109:D15303. doi:10.1029/2003jd004502
- Hakami, A., D.K. Henze, J.H. Seinfeld, K. Singh, A. Sandu, S. Kim, D.W. Byun, and Q. Li. 2007. The adjoint of CMAQ. Environ. Sci. Technol. 41:7807–17. 10.1021/es070944p.
- Hu, Y., M.T. Odman, and A.G. Russell, 2009. Top-down analysis of the elemental carbon emissions inventory in the United States by inverse modeling using Community Multiscale Air Quality model with decoupled direct method (CMAQ-DDM). J. Geophys. Res. Atmos. 114:D24302. doi:10.1029/2009jd011987
- Huntzinger, D.N., S.M. Gourdji, K.L. Mueller, and A.M. Michalak. 2011. The utility of continuous atmospheric measurements for identifying biospheric CO2 flux variability. J. Geophys. Res. Atmos. 116:D06110. doi:10.1029/2010jd015048
- Huntzinger, D.N., C. Schwalm, A.M. Michalak, K. Schaefer, A.W. King, Y. Wei, A. Jacobson, S. Liu, R.B. Cook, W.M. Post, et al. 2013. The North American Carbon Program (NACP) Multi-scale Synthesis and Terrestrial Model Intercomparison Project (MsTMIP): Part I—Overview and experimental design. J. Geosci. Model Dev. in press.
- Huntzinger, D.N., W.M. Post, Y. Wei, A.M. Michalak, T.O. West, A.R. Jacobson, I.T. Baker, J.M. Chen, K.J. Davis, D.J. Hayes,et al. 2012. North American Carbon Program (NACP) regional interim synthesis: Terrestrial biospheric model intercomparison. Ecol. Model. 232:144–57. doi:10.1016/j.ecolmodel.2012.02.004
- Jacobson, M.Z. 2008. On the causal link between carbon dioxide and air pollution mortality. Geophys. Res. Lett. 35:L03809. doi:10.1029/2007GL031101
- Jacobson, M.Z. 2011. Enhancement of local air pollution by urban CO2 domes. Environ. Sci. Technol. 44:2497–502. doi:10.1021/es903018m
- Kort, E.A., C. Frankenberg, C.E. Miller, and T. Oda. 2012. Space-based observations of megacity carbon dioxide. Geophys. Res. Lett. 39:L17806. doi:10.1029/2012gl052738
- Le Quere, C., M.R. Raupach, J.G. Canadell, G. Marland, L. Bopp, P. Ciais, T.J. Conway, S.C. Doney, R.A. Feely, P. Foster,et al. 2009. Trends in the sources and sinks of carbon dioxide. Nat. Geosci. 2:831–36. doi:10.1038/ngeo689
- Li, H., M. Huang, M. S. Wigmosta, Y. Ke, A.M. Coleman, L.R. Leung, A. Wang, and D.M. Ricciuto. 2011. Evaluating runoff simulations from the Community Land Model 4.0 using observations from flux towers and a mountainous watershed. J. Geophys. Res. 116:D24120. doi:10.1029/2011JD016276
- Marland, G. 2008. Uncertainties in accounting for CO2 from fossil fuels. J. Ind. Ecol. 12:136–39. doi:10.1111/j.1530-9290.2008.00014.x
- Mays, K.L., P.B. Shepson, B.H. Stirm, A. Karion, C. Sweeney, and K.R. Gurney. 2009. Aircraft-based measurements of the carbon footprint of Indianapolis. Environ. Sci. Technol. 43:7816–23. doi:10.1021/es901326b
- McKain, K., S.C. Wofsy, T. Nehrkorn, J. Eluszkiewicz, J.R. Ehleringer, and B.B. Stephens. 2012. Assessment of ground-based atmospheric observations for verification of greenhouse gas emissions from an urban region. Proc. Natl. Acad. Sci. USA 109:8423–28. doi:10.1073/pnas.1116645109
- Messerschmidt, J., J. Notholt, C.M. Roehl, V. Sherlock, B.B. Stephens, S.A. Vay, and S.C. Wofsy. 2012. The imprint of surface fluxes and transport on variations in total column carbon dioxide. Biogeosciences 9:875–91. doi:10.5194/bg-9-875-2012
- Michelsen, H.A., G.L. Manney, M.R. Gunson, C.P. Rinsland, and R. Zander. 1998. Correlations of stratospheric abundances of CH4 and N2O derived from ATMOS measurements. Geophys. Res. Lett. 25:2777–80. doi:10.1029/98gl01977
- Miyazaki, K., P.K. Patra, M. Takigawa, T. Iwasaki, and T. Nakazawa. 2008. Global-scale transport of carbon dioxide in the troposphere. J. Geophys. Res. Atmos. 113:D15301. doi:10.1029/2007jd009557
- Nassar, R., L. Napier-Linton, K.R. Gurney, R.J. Andres, T. Oda, F.R. Vogel, and F. Deng. 2013. Improving the temporal and spatial distribution of CO2 emissions from global fossil fuel emission data sets. J. Geophys. Res. Atmos. 118:917–33. doi:10.1029/2012jd018196
- National Research Council. 2010. Verifying Greenhouse Gas Emissions: Methods to Support International Climate Agreements. Washington, DC: National Academies Press.
- Palmer, P.I., P. Suntharalingam, D.B.A. Jones, D.J. Jacob, D.G. Streets, Q.Y. Fu, S.A. Vay, and G.W. Sachse. 2006. Using CO2:CO correlations to improve inverse analyses of carbon fluxes. J. Geophys. Res. Atmos. 111:D12318. doi:10.1029/2005jd006697
- Peters, W., A.R. Jacobson, C. Sweeney, A.E. Andrews, T.J. Conway, K. Masarie, J.B. Miller, L.M.P. Bruhwiler, G. Petron, A.I. Hirsch,et al. 2007. An atmospheric perspective on North American carbon dioxide exchange: CarbonTracker. Proc. Natl. Acad. Sci. USA 104:18925–30. doi:10.1073/pnas.0708986104
- Peylin, P., S. Houweling, M.C. Krol, U. Karstens, C. Rodenbeck, C. Geels, A. Vermeulen, B. Badawy, C.T. Pieterse, P. Ciais, and M. Heimann. 2011. Importance of fossil fuel emission uncertainties over Europe for CO2 modeling: model intercomparison. Atmos. Chem. Phys. 11:6607–22. doi:10.5194/acp-11-6607-2011
- Pillai, D., C. Gerbig, R. Kretschmer, V. Beck, U. Karstens, B. Neininger, and M. Heimann. 2012. Comparing Lagrangian and Eulerian models for CO2 transport—A step towards Bayesian inverse modeling using WRF/STILT-VPRM. Atmos. Chem. Phys. 12:8979–91. doi:10.5194/acp-12-8979-2012
- Pleim, J., and L. Ran. 2011. Surface flux modeling for air quality applications. Atmosphere 2:271–302. doi:10.3390/atmos2030271
- Prather, M., X. Zhu, S.E. Strahan, S.D. Steenrod, and J.M. Rodriguez. 2008. Quantifying errors in trace species transport modeling. Proc. Natl. Acad. Sci. USA 105:19617–21. doi:10.1073/pnas.0806541106
- Rivier, J., P. Ciais, D.A. Hauglustaine, P. Bakwin, P. Bousquet, P. Peylin, and A. Klonecki. 2006. Evaluation of SF6, C2Cl4, and CO to approximate fossil fuel CO2 in the Northern Hemisphere using a chemical transport model. J. Geophys. Res. 111:D16311. doi:10.1029/2005JD006725
- Sarrat, C., J. Noilhan, P. Lacarrere, S. Donier, C. Lac, J.C. Calvet, A.J. Dolman, C. Gerbig, B. Neininger, P. Ciais, J.D. Paris, F. Boumard, M. Ramonet, and A. Butet. 2007. Atmospheric CO2 modeling at the regional scale: Application to the CarboEurope Regional Experiment. J. Geophys. Res. Atmos. 112:D12. doi:10.1029/2006jd008107
- Schaefer, K., C.R. Schwalm, C. Williams, M.A. Arain, A. Barr, J.M. Chen, K.J. Davis, D. Dimitrov, T.W. Hilton, D.Y. Hollinger,et al. 2012. A model-data comparison of gross primary productivity: Results from the North American Carbon Program site synthesis. J. Geophys. Res. Biogeosci. 117:G03010. doi:10.1029/2012jg001960
- Schwalm, C.R., C.A. Williams, K. Schaefer, R. Anderson, M.A. Arain, I. Baker, et al. 2010. A model-data intercomparison of CO2 exchange across North America: Results from the North American Carbon Program site synthesis. J. Geophys. Res. Biogeosci. 115. doi 10.1029/2009JG001229
- Shim, C.S., Y.H. Wang, H.B. Singh, D.R. Blake, and A.B. Guenther. 2007. Source characteristics of oxygenated volatile organic compounds and hydrogen cyanide. J. Geophys. Res. Atmos. 112:D10305. doi:10.1029/2006jd007543
- Suntharalingam, P., D.J. Jacob, P.I. Palmer, J.A. Logan, R.M. Yantosca, Y.P. Xiao, M.J. Evans, D.G. Streets, S.L. Vay, and G.W. Sachse. 2004. Improved quantification of Chinese carbon fluxes using CO2/CO correlations in Asian outflow. J. Geophys. Res. Atmos. 109:D18S18. doi:10.1029/2003jd004362
- Tarantola, A. 2005. Inverse Problem Theory and Methods for Model Parameter Estimation. Philadelphia, PA: SIAM.
- Turnbull, J.C., J.B. Miller, S.J. Lehman, P.P. Tans, R.J. Sparks, and J. Southon. 2006. Comparison of 14CO2, CO, and SF6 as tracers for recently added fossil fuel CO2 in the atmosphere and implications for biological CO2 exchange. Geophys. Res. Lett. 33:L01817. doi:10.1029/2005GL024213
- van der Molen, M. K., and A. J. Dolman. 2007. Regional carbon fluxes and the effect of topography on the variability of atmospheric CO2. J. Geophys. Res. Atmos. 112:D1. doi:10.1029/2006jd007649
- Vogel, F.R., S. Hammer, A. Steinhof, B. Kromer, and I. Levin. 2010. Implication of weekly and diurnal 14C calibration on hourly estimates of CO-based fossil fuel CO2 at a moderately polluted site in southwestern Germany. Tellus Ser. B Chem. Phys. Meteorol. 62:512–20. doi:10.1111/j.1600-0889.2010.00477.x
- Wang, H., D.J. Jacob, M. Kopacz, D.B.A. Jones, P. Suntharalingam, J.A. Fisher, R. Nassar, S. Pawson, and J.E. Nielsen. 2009. Error correlation between CO2 and CO as constraint for CO2 flux inversions using satellite data. Atmos. Chem. Phys. 9:7313–23.
- Wang, Y.H., and T. Zeng. 2004. On tracer correlations in the troposphere: The case of ethane and propane. J. Geophys. Res. Atmos. 109: D24306. doi:10.1029/2004jd005023
- Wei, Y., S. Liu, D.N. Huntzinger, A.M. Michalak, N. Viovy, W.M. Post, C.R. Schwalm, K. Schaefer, A.R. Jacobson, C. Lu, et al. 2013. The North American Carbon Program Multi-scale Synthesis and Terrestrial Model Intercomparison Project – Part 2: Environmental driver data. Geosci. Model Dev. Discuss. 6:5375–5422. doi:10.5194/gmdd-6-5375-2013
- Wunch, D., P.O. Wennberg, G.C. Toon, G. Keppel-Aleks, and Y.G. Yavin. 2009. Emissions of greenhouse gases from a North American megacity. Geophys. Res. Lett. 36:L15810. doi:10.1029/2009gl039825