Abstract
NO2 vertical column densities (VCDs) over East Asia in June and December 2007 were simulated by the Community Multi-scale Air Quality (CMAQ) version 4.7.1 using an updated and more elaborate version of the Regional Emission Inventory in Asia (REAS) version 2. The modeling system could reasonably capture observed spatiotemporal changes of NO2 VCDs by satellite sensors, the Global Ozone Monitoring Experiment-2 (GOME-2), the Scanning Imaging Absorption Spectrometer for Atmospheric Cartography (SCIAMACHY), and the Ozone Monitoring Instrument (OMI), even at the coarsest horizontal resolution of 80 km. The CMAQ simulations were performed in a sequence of three horizontal resolutions (80 km, 40 km, and 20 km) for June and December 2007 to investigate the influence of changes of horizontal resolution on the obtained NO2 VCDs. CMAQ-simulated NO2 VCDs generally increased with improvements in resolution from 80 km to 40 km and then to 20 km. Increases in the CMAQ-simulated NO2 VCDs were greater for the change from 80 km to 40 km than for those from 40 km and 20 km, in which the increases of NO2 VCDs due to the improvement of horizontal resolution were approached convergence at the horizontal resolution of approximately 20 km. Conversely, no clear convergences in NO2 VCDs changes were found at near Tokyo and over the East China Sea. The biases of the NO2 VCDs simulated at a resolution of 20 km against the satellite retrievals were ∼36% near Beijing (CHN1) and ∼78% near Shanghai (CHN2) in summer; these errors were found to be comparable to the horizontal resolution-dependent errors, which were 18–25% at CHN1 and 44–58% at CHN2 from 80 km to 40 km. Conversely, the influence of changes of horizontal resolution in winter was relatively less compared to that in summer.
Implications:
NO2 VCDs over East Asia in June and December 2007 were simulated using CMAQ version 4.7.1 and REAS version 2. The modeling system could reasonably capture observed spatiotemporal changes of NO2 VCDs by satellite sensors. The CMAQ simulations were performed in a sequence of three horizontal resolutions, 80, 40, and 20 km, to investigate the influence of changes of horizontal resolution on the obtained NO2 VCDs. The results suggested that the influence of changes of horizontal resolution was larger in summer compared to that in winter. The magnitude of the influence was comparable to the biases of the NO2 VCDs simulated at a resolution of 20 km against the satellite retrievals.
Introduction
Nitrogen oxides (NOx ≡ NO + NO2) emitted from fossil-fuel combustion, biomass burning, soil microbial processes, and lightning play important roles in tropospheric air quality and climate change through their feedback on hydrogen radicals (HOx ≡ OH + HO2 + RO2). Tropospheric NOx is emitted primarily as NO; subsequently, photochemical equilibration with NO2 occurs within a few minutes. The major sinks of tropospheric NOx are as follows: oxidation to HNO3 by reaction of NO2 with OH during daytime; and reaction of NO2 with O3 followed by heterogeneous reactions of NO3 and N2O5 on aerosol particles during nighttime (Dentener and Crutzen, Citation1993). The concentrations of these removal agents depend strongly on NOx concentration, which is controlled by the tropospheric NO2 lifetime; that is, the removal rate of tropospheric NOx depends largely its own concentration. Therefore, in order to represent tropospheric chemistry realistically, a chemical transport model (CTM) must be used to accurately resolve the spatiotemporal distribution of tropospheric NOx concentrations from its source until it reaches background levels.
It is well known that species subject to nonlinear sources or sinks are susceptible to biases in CTMs with coarse resolution. Column NO2 measured in areas with large point sources (e.g., Valin et al., Citation2011a) and ground- and space-based measurements in California metropolitan regions (e.g., Russell et al., 2011) suggest that the NOx removal could occur at scales of 10–20 km, which agrees well with previously simulated results (e.g., Loughner et al., 2007). Wild et al. (Citation2006) adopted a global CTM and suggested that the reduced averaging and greater separation of polluted and clean regions leads to slower removal of NOx at higher spatial resolution. Moreover, they determined that the export of short-lived precursors (such as NOx) by convection is overestimated at coarse resolutions, and that the T106 resolution is still too coarse to resolve regional O3 chemistry. A comparison of simulated NO2 vertical column densities (VCDs) from a regional CTM, the Weather Research and Forecasting (WRF) model coupled with Chemistry (WRF–Chem), and space-based observations over source regions of the United States revealed that a resolution of 4–12 km was sufficient for accurate modeling of nonlinear effects on the NO2 loss rate (Valin et al., Citation2011b).
East Asia is one of the primary pollutant source regions globally. In particular, a significant increase in NO2 VCDs has been detected since the early 1990s over central east China based on satellite measurements such as those from the Global Ozone Monitoring Experiment (GOME) and the Scanning Imaging Absorption Spectrometer for Atmospheric Cartography (SCIAMACHY) (Richter et al., Citation2005; Irie et al., Citation2005; van der A et al., 2006; Uno et al., Citation2007); these results reflect an increase in NOx emissions (e.g., Ohara at al., 2007). NO2 VCDs simulated by a regional CTM, the Community Multiscale Air Quality modeling system (CMAQ), agree reasonably well with the increase detected in satellite retrievals; however, the CMAQ model tended to underestimate the GOME retrievals by a factor of 2–4 over polluted areas such as central east China (Uno et al., Citation2007). Similarly, van Noije et al. (Citation2006) presented a systematic comparison of NO2 VCDs from 17 global CTMs and three state-of-the-art GOME retrievals for the year 2000, and concluded that the CTMs generally underestimated retrievals over industrial regions such as central east China. However, despite investigating from the perspectives of both satellite retrievals and CTMs with emission inventories, van Noije et al. (Citation2006) were unable to clarify the underlying reasons for this discrepancy.
This study focuses on the accuracy of the NO2 VCDs over East Asia. Here, CMAQ-simulated NO2 VCDs derived using the Regional Emission Inventory in Asia (REAS) version 2 (Kurokawa et al., Citation2013) are compared with three satellite-retrieved NO2 VCDs from GOME-2, SCIAMACHY, and the Ozone Monitoring Instrument (OMI). Additionally, the effects of alteration of the model’s horizontal grid resolutions on NO2 VCDs were evaluated for both summer and winter.
Experimental Methods
Model description
The modeling system employed in the present study was CMAQ version 4.7.1 driven by WRF version 3.3 (Skamarock and Klemp, Citation2008). The WRF simulation used the National Centers for Environmental Prediction (NCEP) Final Analysis data (ds083.2) for the year 2007. Detailed model setups are shown in . The vertical layers consisted of 37 sigma-pressure coordinated layers from the surface to 50 hPa, with the first layer at approximately 20 m height. Monthly anthropogenic emissions used over East Asia were obtained from REAS version 2 (Kurokawa et al., Citation2013), which was recently updated and is more elaborate than REAS version 1 (Ohara et al., Citation2007). The spatial distributions of rural, urban, and total populations and the road network were used to allocate country- and subregion-based emissions to grids. The spatial resolution of gridded data from REAS version 2 was 0.25°, which represents a considerable improvement from that of REAS version 1 (0.5°). Population data were updated with the Global Rural–Urban Mapping Project version 1 (GRUMPv1), whose Urban/Rural Extents data set comprises 30″ × 30″ grids, and the Gridded Population of the World version 3 (GPWv3), whose data set comprises 2.5′ × 2.5′ grids (CIESIN et al., Citation2005, Citation2011). Spatial distributions of total population for 2000, 2005, and 2010 were obtained from GPWv3 and interpolated for each year between 2000 and 2008. Using GRUMPv1 data for 2000, the total population data were divided and aggregated to 0.25° × 0.25° urban and rural population data. For road transport emissions, the road network data at 0.5° × 0.5° were divided into 0.25° × 0.25° grids using the updated total population data. Therefore, the procedure for deriving surrogate data to allocate road transport emissions was not fundamentally different to that for REAS version 1. Biomass burning and biogenic emissions were obtained from the Reanalysis of the Tropospheric Chemical Compositions over the past 40 years (RETRO) (EC Framework Programme, Citation2002) and the Model of Emissions of Gases and Aerosols from Nature (MEGAN) version 2.0 (Guenther et al., Citation2012), respectively. illustrates the model domains with NO2 emissions. The horizontal resolutions and grid dimensions were 80, 40, and 20 km and 95 × 75, 110 × 88, and 184 × 132 grids for domain 1 (D1), domain 2 (D2), and domain 3 (D3), respectively. NO2 emission flux from central eastern China (111°E–121°E, 27°N–40°N) was 1.1 kmol km−2 sec−1 for every model domain in June 2007. The highest emissions for the model domains with horizontal resolutions of 80 km (D1), 40 km (D2), 20 km (D3) were 6.1, 12.0, and 25.0 kmol km−2 sec−1 of NO2 emissions, respectively, found near Shanghai. Relatively high emission rates of more than 2.5 kmol km−2 sec−1 occurred in nine grids at megacities such as Shanghai, Beijing, Seoul, and Tokyo on D1. Meanwhile, the numbers of grids including more than 2.5 kmol km−2 sec−1 of NO2 emissions were increased up to 56 grids for D2 and 272 grids for D3. The grid cells were found not just at those megacities but at the other cities in China, Korea, and Japan. The finer horizontal resolutions illustrate in detail the emission distribution pattern.
Table 1. Model setup or WRF and CMAQ
Satellite data
Tropospheric NO2 VCDs from GOME-2, SCIAMACHY, and OMI were used; these are equipped with ultraviolet (UV)/visible sensors that measure sunlight backscattered from the earth’s atmosphere and reflected by the surface, in addition to the direct solar irradiance spectrum. GOME-2 passes over the equator at about 09:30 local time (LT) and achieves global coverage observations every day, with a ground pixel size of 80 × 40 km2 (240 × 40 km2 for the back scan). SCIAMACHY passes over the equator at about 10:00 LT and achieves global coverage observations in 6 days with a spatial resolution of 60 × 30 km2. OMI passes over the equator at around 13:40–13:50 LT. Daily global measurements are achieved by a wide field of view of 114°, in which 60 discrete viewing angles (at a nominal nadir spatial resolution of 13 × 24 km2) are distributed perpendicular to the flight direction. Therefore, while observation specifications are somewhat different among the three sensors, tropospheric NO2 VCD data are retrieved using the same basic algorithms (DOMINO products for OMI and TM4NO2A products for SCIAMACHY and GOME-2) (Boersma et al., 2004, 2007, 2011). Unreliable satellite swath data affected by so-called row anomalies were screened out, following Boersma et al. (2011). The error in the satellite tropospheric NO2 VCD data includes uncertainties in the slant column, the stratospheric column, and the tropospheric air mass factor (AMF) (Boersma et al., 2004), and can be expressed as ±1 × 1015 molecules cm−2 ± 30% for polluted situations. Comparisons were made for the version 2 retrievals under cloud-free conditions, that is, with cloud fractions (CFs) less than 20%. Irie et al. (Citation2012) demonstrated the biases in SCIAMACHY, OMI, and GOME-2 data against the MAX–DOAS observations over China to be −5 ± 14%, −10 ± 14%, and +1 ± 14%, respectively, and suggested that these biases were sufficiently low to allow combination of these satellite data for air quality analysis. Therefore, these satellite data were assumed to be accurate and were used for comparisons with the simulated NO2 VCD data in the present study. However, it should be noted that the bias estimates just described were based on summertime data over China.
Model–Satellite intercomparison
Accumulated hourly NO2 concentrations from the surface to approximately 11 km were used as CMAQ-simulated NO2 VCDs in the present study. The CMAQ-simulated NO2 VCDs in June and December 2007 were compared with three tropospheric NO2 VCDs retrievals from three different satellite sensors: GOME-2, SCIAMACHY, and OMI observed the atmosphere at 09:30 LT, 10:00 LT, and 13:40–13:50 LT, respectively.
To ensure direct comparisons with satellite retrievals described in the following sections, both the CMAQ-simulated and satellite-retrieved NO2 VCDs were averaged across a 1° × 1° grid. Satellite swath data, which covered 80 × 40 km2 (GOME-2), 60 × 30 km2 (SCIAMACHY), and 13 × 24 km2 (OMI), fell into each 1° × 1° grid and were used to estimate monthly mean NO2 VCDs, respectively. The hourly CMAQ-simulated NO2 VCDs averaged for each 1° × 1° grid were interpolated to obtain observed local times for the respective satellites. The monthly mean simulated NO2 VCDs were estimated using only the averaged CMAQ-simulated NO2 VCDs for the times at which the satellite 1° × 1° NO2 VCDs existed.
Spatial distributions of satellite-retrieved NO2 VCDs and CMAQ-simulated NO2 VCDs
– and – exhibit monthly averaged spatial distributions of tropospheric NO2 VCDs according to CMAQ simulations and satellite retrievals at 9:30 LT, 10:00 LT, and 13:40–13:50 LT in June 2007, respectively. This model captured the spatial patterns of satellite-observed NO2 and temporal change reasonably well, owing to a modeled NO2 decrease in the daytime that was detected by all three different satellite sensors: (a) GOME-2, (b) SCIAMACHY, and (c) OMI. The CMAQ simulations reproduced the relatively high levels of NO2 VCDs (i.e., more than 1 × 1016 molecules cm-2) detected by the satellites on grids from the North China Plain to the Yangtze River delta, in the northern parts of eastern China, and in the megacities of Seoul and Tokyo. However, CMAQ tended to slightly overestimate these NO2 VCDs with respect to the satellite retrievals, particularly in the morning. The CMAQ-simulated morning NO2 VCDs were maximums of 3.9 × 1016, 4.7 × 1016, 3.5 × 1016, and 4.6 × 1016 molecules cm-2 in Beijing, Shanghai, Seoul, and Tokyo, respectively, representing overestimation by factors of 1.8, 3.1, 8.8, and 4.2, respectively, in relation to the GOME-2 retrievals. However, the CMAQ-simulated afternoon NO2 VCDs were smaller than those retrieved by OMI, except grids including the highest NO2. Based on the small biases of GOME-2 and OMI (+1 % ± 14 % and −10% ± 14%, respectively) against the MAX–DOAS observations, the discrepancies between simulated and retrieved NO2 VCDs may be due to the adoption of simulation conditions that do not incorporate diurnal changes in emissions. Conversely, it should be noted that the satellite spatial resolutions (e.g., 80 × 40 km2, or 240 × 40 km2 for the back scan for GOME-2 and 60 × 30 km2 for SCIAMACHY data) were insufficient to evaluate point sources in the megacities.
Figure 2. Monthly averaged spatial distributions of NO2 VCDs (units: e16 molecules cm-2) on a 1° × 1° grid based on satellite retrievals including (a) GOME-2, (b) SCIAMACHY, and (c) OMI, and (d)–(f) CMAQ simulations using D1 (80 km) for three different local times (09:30, 10:00, and 13:40–13:50) in June 2007. Absolute biases, calculated as (CMAQ-simulated NO2 VCDs) – (satellite NO2 VCDs), are shown in (g)–(i).
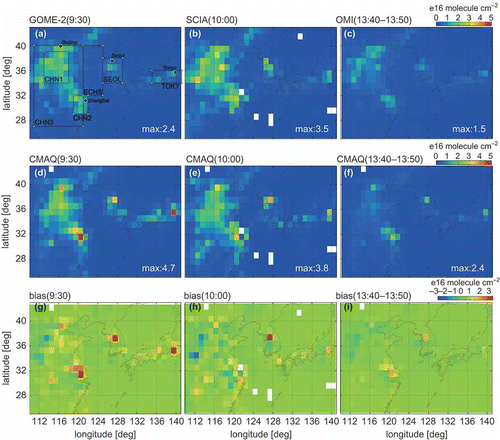
– present absolute biases, that is, (CMAQ-simulated NO2 VCDs) − (satellite NO2 VCDs), for further comparison. The biases relative to GOME-2 NO2 over eastern China and the Korean Peninsula were typically between −1 × 1016 and +1 × 1016 molecules cm-2, except for a few grids near the megacities of Beijing, Shanghai, Seoul, and Tokyo. Over the ocean, CMAQ exhibited negative biases over the Yellow Sea and the Japan Sea, but positive biases over the northwestern Pacific, versus the GOME-2 NO2 VCDs. The positive biases for SCIAMACHY were smaller than those for GOME-2 over land areas. For OMI, the biases were lower than those of the other two retrievals; in fact, they were close to zero, and CMAQ NO2 VCDs agreed well with OMI NO2 VCDs, except for a few overestimated grids near Shanghai and Seoul.
– and – illustrate monthly averaged spatial distributions of NO2 VCDs according to CMAQ simulations and satellite retrievals at 09:30 LT, 10:00 LT, and 13:40–13:50 LT in December 2007. The CMAQ-simulated NO2 VCDs exhibited similar spatial patterns to those retrieved by satellite for each sensor. The simulated NO2 VCDs ranged from 3 × 1016 to 5 × 1016 molecules cm-2, with a maximum of 4.6 × 1016 molecules cm-2 at 09:30 LT. Although the satellite-retrieved NO2 VCDs tended to be highest at 10:00, it should be noted that there were some missing cells over the high concentration area in the latter. The simulated NO2 VCDs were clearly lower than those retrieved by GOME-2 and SCIAMACHY in the morning over the North China Plain. Meanwhile, CMAQ NO2 VCDs were much closer to OMI-retrieved NO2 VCDs in the afternoon than those of the other two observed in the morning. Thus, the simulated daytime NO2 changes were more moderate than those observed.
Figure 3. Monthly averaged spatial distributions of NO2 VCDs (units: e16 molecules cm-2) on a 1° × 1° grid based on satellite retrievals including (a) GOME-2, (b) SCIAMACHY, and (c) OMI, and (d)–(f) CMAQ simulations using D1 (80 km) for three different local times (09:30, 10:00, and 13:40–13:50) in December 2007. Absolute biases, calculated as (CMAQ-simulated NO2 VCDs) – (satellite NO2 VCDs), are shown in rows (g)–(i).
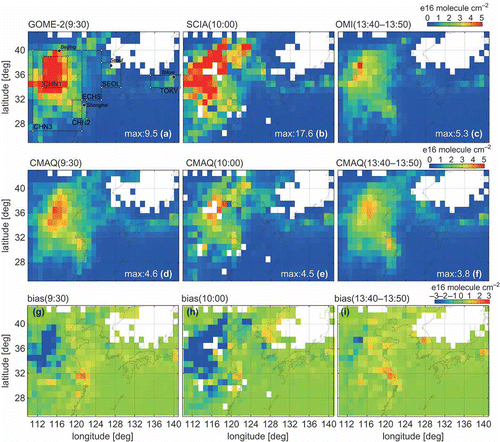
The biases of CMAQ against GOME-2 and SCIAMACHY NO2 VCDs observed in the morning were negative: −3 × 1016 to −6 × 1016 molecules cm-2 and −3 × 1016 to −9 × 1016 molecules cm-2, respectively, over the North China Plain. However, a few grids near Shanghai indicated positive values, as shown in and Meanwhile, the biases relative to OMI were smaller than those for the other two retrievals, typically remaining between −2 × 1016 and +2 × 1016 molecules cm-2 (). The biases of simulated NO2 VCDs against satellite-derived NO2 VCDs in winter were clearly larger than those in summer. As discussed earlier, the biases in these satellite data against the MAX–DOAS observations have been investigated based on summertime data only (Irie et al., Citation2012); therefore, further and more precise validation studies are required to explain the differences in biases between seasons.
Previous studies comparing CMAQ-simulated NO2 VCDs using REAS version 1 and the Asia Pacific Regional Aerosol Characterization Experiment emission inventory (ACE-ASIA) with GOME-retrieved NO2 VCDs have demonstrated that using CMAQ version 4.4 with SAPRC-99/REAS version 1 results in annual underestimation of regional NO2 VCDs by a factor of 2–4. The underestimations were typically observed for central eastern China (Uno et al., Citation2007). Use of CMAQ version 4.3 with a fourth-generation carbon bond mechanism (CBM4) with ACE-ASIA resulted in annual underestimation of NO2 VCDs by ∼57.3% in North China (Han et al., Citation2009). Moreover, a slight overestimation by the CMAQ simulations was detected on a few grids near Shanghai, Seoul, and Busan and in South China. Present results of CMAQ version 4.7.1 with SAPRC-99/REAS version 2 were generally consistent with those of previous studies; however, these CMAQ-simulated NO2 VCDs were clearly closer to the values obtained from GOME-2 than those obtained using CMAQ version 4.4 and REAS version 1 (Uno et al., Citation2007). The major reason for the moderated underestimation was likely an increase in NO2 due to the inclusion of soil NOx in the updated REAS. Another previous study comparing CMAQ-simulated NO2 VCDs using the Intercontinental Chemical Transport Experiment Phase B (INTEX-B) inventory with OMI retrievals (Han et al., Citation2011) demonstrated that the CMAQ-simulated NO2 VCDs were generally larger than those of OMI retrievals over China, particularly for southern parts of China; however, simulated NO2 tended to underestimate OMI retrievals for some grids in North China. and also illustrate that a slight simulated overestimation and slight simulated underestimation were detected in the southern and northern parts of eastern China, respectively.
CMAQ-simulated NO2 VCDs at different horizontal resolutions
To investigate the impact of changes of horizontal resolution on simulated NO2 VCDs, CMAQ-simulated NO2 VCDs at three horizontal resolutions between 20 km and 80 km were compared with satellite-retrieved NO2 VCDs for three diagnostic land areas of China: CHN1 (near Beijing), CHN2 (near Shanghai), and CHN3 (central eastern China). Two diagnostic land areas outside China—SEOL (near Seoul) and TOKY (near Tokyo)—and one diagnostic area over sea (ECHS, over the East China Sea) were also selected, as detailed in .
Table 2. Diagnostic areas
–, –, and – illustrate the evolution of the relationships between satellite-retrieved and CMAQ-simulated NO2 VCDs with changes of horizontal resolution from 80 km (D1) to 40 km (D2) and then to 20 km (D3) at 09:30 LT (GOME-2), 10:00 LT (SCIAMACHY), and 13:40–13:50 LT (OMI), respectively, in June 2007.
Figure 4. Scatterplots between monthly averaged NO2 VCDs of satellite retrievals (GOME-2 in the first row, SCIAMACHY in the second row, and OMI in the third row) and CMAQ simulations at different horizontal resolutions: D1 (80 km) in the first column, D2 (40 km) in the second column, and D3 (20 km) in the third column. Simulations were conducted for six diagnostic areas: CHN1 (near Beijing), CHN2 (near Shanghai), CHN3 (central eastern China), SEOL (near Seoul), TOKY (near Tokyo), and ECHS (over the East China Sea); details of these areas for June 2007 are listed in . Error bars represent the maximum and minimum values of the daily data for the diagnostic areas.
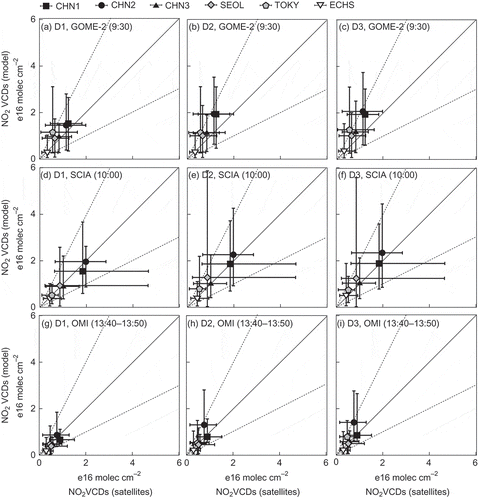
Correlations between the D1 simulated NO2 VCDs and GOME-2, as shown in , indicate that CMAQ-simulated NO2 VCDs were larger than GOME-2-retrieved NO2 VCDs by factors of 1.2 (for CHN3) to 2.0 (for TOKY) for all land diagnostic areas. However, over sea areas (i.e., for ECHS), the D1 simulated NO2 VCDs were 10% smaller than those retrieved. Improvements in horizontal resolution from 80 km to 20 km resulted primarily in nonlinear increases in CMAQ-simulated NO2 VCDs. Consequently, the positive biases against the retrievals were greater at finer horizontal resolutions for the land areas, that is, for CHN1, CHN2, CHN3, SEOL, and TOKY (–). The D1 simulated NO2 VCDs, with resolution of 80 km, exhibited strong similarities to those retrieved by SCIAMACHY, with differences within ±10% (). Negative biases against retrieved NO2 VCDs were found for the diagnostic areas over land in China, where improvements in horizontal resolution resulted in increases and decreases of simulated NO2 VCDs and negative biases, respectively.
For correlations between the D1 simulated NO2 VCDs and OMI NO2 VCDs, as shown in , relatively small differences (i.e., within ±10%) were detected for CHN2 and TOKY, where the improvement of horizontal resolution from 80 km to 20 km resulted in positive biases against the retrieved NO2 VCDs ( and ). For the other areas, the D1 simulated NO2 VCDs were lower than those retrieved by factors of 0.8 (for CHN3) to 0.6 (for ECHS); the improvement of horizontal resolution resulted in increases and decreases in simulated NO2 VCDs and negative biases, respectively.
–, –, and – indicate the evolution of the relationships between satellite-retrieved and CMAQ-simulated NO2 VCDs due to changes of horizontal resolution from 80 km (D1) to 40 km (D2), and then to 20 km (D3) at 09:30 LT (GOME-2), 10:00 LT (SCIAMACHY), and 13:40–13:50 LT (OMI) in winter 2007, respectively.
Figure 5. Scatterplots between monthly averaged NO2 VCDs of satellite retrievals (GOME-2 in the first row, SCIAMACHY in the second row, and OMI in the third row) and CMAQ simulations at different horizontal resolutions: D1 (80 km) in the first column, D2 (40 km) in the second column, and D3 (20 km) in the third column. Simulations were conducted for six diagnostic areas: CHN1 (near Beijing), CHN2 (near Shanghai), CHN3 (central eastern China), SEOL (near Seoul), TOKY (near Tokyo), and ECHS (over the East China Sea); details of these areas for December 2007 are listed in . Error bars represent the maximum and minimum values of the daily data for the diagnostic areas.
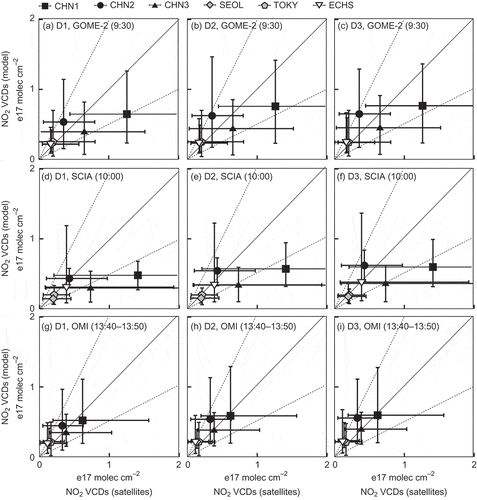
For the correlations between the D1 simulated NO2 VCDs and GOME-2, as shown in , CMAQ-simulated NO2 VCDs were lower than those retrieved by up to a factor of 2 at CHN1 and CHN3 but were 20–50% larger than those retrieved for other areas. The improvement in resolution from D1 to D3 resulted in nonlinear increases in CMAQ-simulated NO2 VCDs, resulting in a decrease in negative bias relative to the retrievals at finer spatial resolutions for CHN1 and CHN3 (–). Conversely, the positive biases at CHN2 increased by up to a factor of 2. The correlations between the D1 simulated NO2 VCDs and the SCIAMACHY retrievals are presented in Similar to the 9:30LT case, the simulated NO2 VCDs were slightly larger than the retrievals at CHN2, where increased CMAQ NO2 VCDs due to the change of horizontal resolution from D1 to D3 resulted in increases in positive biases. For other areas, CMAQ generally underestimated the SCIAMACHY retrievals by 10% (for TOKY) to 70% (for CHN1). Moreover, the negative biases against the retrievals decreased as a result of the increase of the simulated NO2 VCDs due to changes of horizontal resolution. In particular, the D3 CMAQ-simulated NO2 VCDs for SEOL, TOKY, and ECHS were rather close to the associated retrievals. The correlations between the coarsest CMAQ (D1, i.e., 80 km resolution) and OMI are presented in CMAQ underestimated OMI-retrieved NO2 VCDs by 20% at CHN1 and 10% at CHN3. For other areas, CMAQ tended to overestimate by between 30% (for TOKY) and 70% (for ECHS). On par with the morning cases, the changes of horizontal resolution from 80 km to 20 km resulted in increases in the CMAQ-simulated NO2 VCDs. Consequently, the negative biases for CHN1 and CHN3 approached zero for D2 and D3.
compares simulated NO2 VCDs changes between D1 and D2 and between D2 and D3 for each area: CHN1, CHN2, CHN3, SEOL, TOKY, and ECHS. Increases in the CMAQ-simulated NO2 VCDs from D1 to D2 were greater than those between D2 and D3 at CHN1, CHN2, CHN3, and SEOL, and that means the increases of NO2 VCDs due to the improvement of horizontal resolution are approaching convergence at a horizontal resolution of approximately 20 km. Conversely, clear convergences were not found NO2 VCDs changes at TOKY. Moreover, simulated NO2 VCDs over sea areas without emissions decreased owing to changes of the horizontal resolution (from 40 km to 20 km) in winter.
Table 3. Increases in CMAQ simurated NO2 VCDs due to model spatial resolution change (e16 molecules cm–2)
A previous study investigating the effects of model horizontal resolution on NO2 VCDs (Valin et al., Citation2011b) demonstrated that NO2 VCDs increased with increasing resolution and that different horizontal resolutions were necessary to ensure 10% accuracy (with respect to UV/visible instrument data collected in July 2006) for different regions of the United States: 4 km over Four Corners, New Mexico; 24 km over the San Joaquin Valley, California; and 40 km over Los Angeles, CA. Moreover, Valin et al. (Citation2011b) suggested that 4–12 km was sufficient to accurately model nonlinear effects in the NO2 loss rate. The present study suggested that a resolution of 20 km would be sufficient to avoid horizontal resolution-dependent error over land areas including large emission sources, such as eastern China, because increases in the simulated NO2 VCDs began to converge as resolution was decreased from 40 km to 20 km. However, such convergences were not detected for TOKY and ECHS.
For summertime, the biases of D3 simulated NO2 VCDs against the satellite retrievals were +36%, −1%, and −15% at CHN1 and +75%, +30%, and +78% at CHN2 for GOME-2, SCIAMACHY, and OMI, respectively. It should be noted that these errors are comparable to the horizontal resolution-dependent errors, 18–25% at CHN1 and 44–58% at CHN2 from 80 km to 40 km. For wintertime, the horizontal resolution-dependent errors were relatively unimportant (e.g., 11–18% at CHN1 and 24–37% at CHN2) compared to the summertime errors.
Summary
NO2 VCDs over East Asia in June and December 2007 were simulated by CMAQ version 4.7.1 using an updated and more elaborate version of the Regional Emission Inventory in Asia, REAS version 2. The CMAQ-simulated NO2 VCDs were compared with three tropospheric NO2 retrievals from three different satellite sensors (GOME-2, SCIAMACHY, and OMI). CMAQ had good performance in capturing the observed spatiotemporal changes of NO2 VCDs, even at the coarsest resolution (80 km).
Six diagnostic areas were investigated, including CHN1 (near Beijing), CHN2 (near Shanghai), CHN3 (central eastern China), SEOL (near Seoul), TOKY (near Tokyo), and ECHS (over the East China Sea). For these regions, the averaged simulated NO2 VCDs remained primarily within factors of ±2 of those retrieved by satellite. The results achieved using CMAQ version 4.7.1 with SAPRC-99/REAS version 2 were generally consistent with those of previous studies (Uno et al., Citation2007; Han et al., Citation2009; Han et al., Citation2011); however, these NO2 VCDs in the present study were clearly closer to the retrievals than the results determined using CMAQ version 4.4 and REAS version 1 (Uno et al., Citation2007). The moderated underestimation was likely caused primarily by NO2 increases as a result of use of the updated REAS version 2.
The CMAQ simulations were performed in a sequence of three horizontal resolutions (80 km, 40 km, and 20 km) in June and December 2007 to investigate the influence of changes of horizontal resolution on the obtained NO2 VCDs. CMAQ-simulated NO2 VCDs generally increased with improvements in resolution from 80 km to 40 km and then to 20 km; this resulted in larger positive biases but improved the negatively biases between CMAQ simulations and satellite retrievals. However, the finer horizontal resolutions did not necessarily exhibit better agreement with the satellite retrievals than the coarser resolutions in the present study.
Increases in the CMAQ-simulated NO2 VCDs in response to changes of horizontal resolution were greater for the change from 80 km to 40 km than for those from 40 km and 20 km for the four diagnostic land areas including large emission sources in China and Korea (CHN1, CHN2, CHN3, and SEOL), in which the increases of NO2 VCDs due to the improvement of horizontal resolution were close to the convergence at the horizontal resolution of approximately 20 km. Conversely, no clear convergences in NO2 VCD changes were found at near Tokyo (TOKY) and over the sea (ECHS).
The biases of the NO2 VCDs simulated at a resolution of 20 km against the satellite retrievals were ∼36% at CHN1 and ∼78% at CHN2 in summer; these errors were found to be comparable to the horizontal resolution-dependent errors, which were 18–25% at CHN1 and 44–58% at CHN2 from 80 km to 40 km. Conversely, the influence of changes of horizontal resolution in winter, for example, 11–18% at CHN1 and 24–37% at CHN2, were relatively less compared with those in summer.
Funding
This research was supported by the Environment Research and Technology Development Fund (S-7) of the Ministry of the Environment, Japan, and the support program for young and women researchers of the University of Tokyo.
Additional information
Notes on contributors
Kazuyo Yamaji
Kazuyo Yamaji is a scientist and Kohei Ikeda is a postdoctoral researcher at the Research Institute for Global Change, Japan Agency for Marine–Earth Science and Technology, Yokohama, Japan.
Kohei Ikeda
Kazuyo Yamaji is a scientist and Kohei Ikeda is a postdoctoral researcher at the Research Institute for Global Change, Japan Agency for Marine–Earth Science and Technology, Yokohama, Japan.
Hitoshi Irie
Hitoshi Irie is an associate professor at the Center for Environmental Remote Sensing, Chiba University, Chiba, Japan.
Jun–ichi Kurokawa
Jun–ichi Kurokawa is a senior scientist at Asia Center for Air Pollution Research, Niigata, Japan.
Toshimasa Ohara
Toshimasa Ohara is the director of the Center for Regional Environmental Research, National Institute for Environmental Studies, Tsukuba, Japan.
References
- Center for International Earth Science Information Network (CIESIN), Columbia University, United Nations Food and Agriculture Programme (FAO), and Centro Internacional de Agricultura Tropical (CIAT). 2005. Gridded Population of the World, Version 3 (GPWv3), Population Count Grid, Future Estimates, Palisades, NY, NASA Socioeconomic Data and Applications Center (SEDAC). http://sedac.ciesin.columbia.edu/data/set/gpw-v3-population-count-future-estimates (accessed April 15, 2013).
- Center for International Earth Science Information Network (CIESIN), Columbia University, International Food Policy Research Institute (IFPRI), The World Bank, and Centro Internacional de Agricultura Tropical (CIAT). 2011. Global Rural-Urban Mapping Project, Version 1 (GRUMPv1), Population Count Grid, Palisades, NY, NASA Socioeconomic Data and Applications Center (SEDAC). http://sedac.ciesin.columbia.edu/data/set/grump-v1-population-count (accessed April 15, 2013).
- Dentener, F. J., and P. J. Crutzen. 1993. Reaction of N2O5 on tropospheric aerosols: Impact on the global distribution of NOx, O3, and OH, J. Geophys. Res. 98(D4):7149–63. doi:10.1029/92JD02979
- EC Framework Programme, 2002. Reanalysis of the Tropospheric Chemical Compositions over the past 40 years. http://retro.enes.org (accessed April 15, 2013).
- Guenther, A. B., X. C. L. Jiang, Heald, T. Sakulyanontvittaya, T. Duhl, L. K. Emmons, and X. Wang. 2012. The Model of Emissions of Gases and Aerosols from Nature version 2.1 (MEGAN2.1): An extended and updated framework for modeling biogenic emissions. Geosci. Model Dev. 5:1503–60. doi:10.5194/gmdd-5-1503-2012
- Han, K. M., C. H. Song, H. J. Ahn, R. S. Park, J. H. Woo, C. K. Lee, A. Richter, J. P. Burrows, J. Y. Kim, and J. H. Hong. 2009. Investigation of NOx emissions and NOx-related chemistry in East Asia using CMAQ-predicted and GOME-derived NO2 columns. Atmos. Chem. Phys. 9:1017–36. doi:10.5194/acp-9-1017-2009
- Han, K. M., C. K. Lee, J. Lee, J. Kim, and C. H. Song. 2011. A comparison study between model-predicted and OMI-retrieved tropospheric NO2 columns over the Korean peninsula. Atmos. Environ. 45(17):2962–71. doi:10.1016/j.atmosenv.2010.10.016
- Heue, K.-P., T. Wagner, S. P. Broccardo, D. Walter, S. J. Piketh, K. E. Ross, S. Beirle, and U. Platt. 2008. Direct observation of two dimensional trace gas distributions with an airborne Imaging DOAS instrument Atmos. Chem. Phys. 8:6707–17. doi:10.5194/acp-8-6707-2008
- Irie, H., K. Sudo, H. Akimoto, A. Richter, J. P. Burrows, T. Wagner, M. Wenig, S. Beirle, Y. Kondo, V. P. Sinyakov, and F. Goutail. 2005. Evaluation of long-term tropospheric NO2 data obtained by GOME over East Asia in 1996–2002. Geophys. Res. Lett. 32(L11810). doi:10.1029/2005GL022770
- Irie, H., K. F. Boersma, Y. Kanaya, H. Takashima, X. Pan, and Z. F. Wang. 2012. Quantitative bias estimates for tropospheric NO2 columns retrieved from SCIAMACHY, OMI, and GOME-2 using a common standard for East Asia. Atmos. Meas. Technol. 5:2403–11. doi:10.5194/amt-5-2403-2012
- Kurokawa, J.-I., T. Ohara, T. Morikawa, S. Hanayama, J.-M. Greet, T. Fukui, K. Kawashima, and H. Akimoto. 2013. Emissions of air pollutants and greenhouse gases over Asian regions during 2000–2008: Regional Emission inventory in ASia (REAS) version 2. Atmos. Chem. Phys. Discuss. 13: 10049–123. doi:10.5194/acpd-13-10049-2013
- Ohara, T., H. Akimoto, J. -I. Kurokawa, N. Horii, K. Yamaji, X. Yan, and T. Hayasaka. 2007. An Asian emission inventory of anthropogenic emission sources for the period 1980–2020. Atmos. Chem. Phys. 7:4419–44. doi:10.5194/acp-7-4419-2007
- Skamarock, W. C., and J. B. Klemp. 2008. A time-split nonhydrostatic atmospheric model for weather research and forecasting applications. J. Comput. Phys. 227:3465–85. doi:10.1016/j.jcp.2007.01.037
- Richter, A., J. P. Burrows, H. Nüß, C. Granier, and U. Niemeier. 2005. Increase in tropospheric nitrogen dioxide over China observed from space. Nature 437: 129–32. doi:10.1038/nature04092
- Russell, A. R., L. C. Valin, E. J. Bucsela, M. O. Wenig, and R. C. Cohen. 2010. Space-based constraints on spatial and temporal patterns of NOx emissions in California, 2005–2008. Environ. Sci. Technol. 44:3608–15. doi:10.1021/es903451j
- Uno, I., Y. He, T. Ohara, K. Yamaji, J. -I. Kurokawa, M. Katayama, Z. Wang, K. Noguchi, S. Hayashida, A. Richter, and J. P. Burrows. 2007. Systematic analysis of interannual and seasonal variations of model-simulated tropospheric NO2 in Asia and comparison with GOME-satellite data. Atmos. Chem. Phys. 7:1671–81. doi:10.5194/acp-7-1671-2007
- Valin, L. C., A. R. Russell, E. J. Bucsela, J. P. Veefkind, and R. C. Cohen. 2011a. Observation of slant column NO2 using the superzoom mode of AURA-OMI. Atmos. Meas. Technol. 4:1929–35. doi:10.5194/amt-4-1929-2011
- Valin, L. C., A. R. Russell, R. C. Hudman, and R. C. Cohen. 2011b. Effects of model resolution on the interpretation of satellite NO2 observations. Atmos. Chem. Phys. 11:11647–55. doi:10.5194/acp-11-11647-2011
- van Noije, T. P. C., Eskes, H. J., Dentener, F. J., D. S. Stevenson, K. Ellingsen, M. G. Schultz, O. Wild, M. Amann, C. S. Atherton, D. J. Bergmann, et al. 2006. Multi-model ensemble simulations of tropospheric NO2 compared with GOME retrievals for the year 2000. Atmos. Chem. Phys. 6:2943–2979. doi:10.5194/acpd-6-2965-2006
- van der A, R.J.,H. J. Eskes, K. F. Boersma, T. van Noije, M. van Roozendael, I. De Smedt, D. H. M. U. Peters, and E.W. Meijer. 2008. Trends, seasonal variability and dominant NOx source derived from a ten year record of NO2 measured from space, J. Geophys. Res. Atmos. 113(D04302). doi:10.1029/2007JD009021
- Wild, O., and M. J. Prather. 2006. Global tropospheric ozone modeling: quantifying errors due to grid resolution. J. Geophys. Res. Atmos. 111(D11305). doi:10.1029/2005jd006605