Abstract
We report on the analysis of contributions from road traffic emissions to fine particulate matter (PM2.5) concentrations within London for 2008 with the OSCAR Air Quality Assessment System. A spatiotemporal evaluation of the OSCAR system has been conducted with measurements from the London air quality network (LAQN). For the predicted and measured hourly time series of concentrations at 18 sites in London, the medians of correlation, mean absolute error, index of agreement, and factor of two (FAC2) of all stations were 0.80, 4.1 μg/m3, 0.86, and 74%, respectively. Spatial evaluation of modeled and observed annual mean concentrations also showed a fairly good agreement, with all the values falling within the FAC2 range. According to model predictions, the urban increment (including the contributions from urban traffic and other urban sources) was evaluated to be on the average 18%, 33%, 39%, and 43% of the total PM2.5 in suburban environments, in the urban background, near roads, and near busy roads, respectively. However, the highest values of the urban traffic increment can be around 50% of the total PM2.5 concentrations near motorways and major roads. The total concentrations (including regional background, and the contributions from urban traffic and other urban sources) can therefore be almost three times the regional background. The total urban increment close to busy roads was around 7–8 μg/m3, in which the estimated traffic contribution is more than 2 μg/m3. On the average, urban traffic contributes approximately 1 μg/m3 of PM2.5 to the urban background across London. According to modeling, approximately two-thirds of the traffic increment originated from exhaust emissions and most of the rest was due to brake and tire wear.
Implications:
The urban increment and traffic contribution to the total PM2.5 are significant and spatially heterogeneous across London. The highly heterogeneous distribution of PM2.5 hence requires detailed modeling studies to be carried out at high spatial resolution, which can be particularly important for exposure and health impact assessment. This type of information can be used to quantify health impacts resulting from specific sources of PM2.5 such as traffic emissions, to aid city and national decision makers when formulating pollution control strategies.
Introduction
Significant health impacts are associated with traffic-related airborne particulate matter pollution (Pope and Dockery, Citation2006; Grahame and Schlesinger, Citation2010; Rohr and Wyzga, Citation2012). Quantifying the traffic-related contributions to the overall fine particulate matter (PM2.5) concentrations will allow for (i) source category-specific modeling of actual exposures and for (ii) an evaluation of the potential reductions in exposure from actions to reduce the emissions in urban areas. Studies have revealed substantial spatial variability in the relationships between long-term exposure to outdoor air pollution and health, for example, using cohorts across Europe (Eeftens et al., Citation2012). Air quality management assessment or health studies, including the ones based on epidemiological methods, require models to provide concentrations at fine spatiotemporal scale to estimate space–time activity exposure patterns.
To calculate exposure to air pollutants a range of approaches has been employed, including geostatistical interpolation technique (e.g., Singh et al., Citation2011), land use regression models (e.g., Beelen et al., Citation2013), dispersion models (e.g., Kousa et al., Citation2001) and hybrid models (Ashmore et al., 2005). However, when it comes to roadside calculations, Gaussian line source dispersion models have proved to be very useful to calculate the dispersion of pollutants such as PM2.5 from a road. Examples of such applications include GM (Chock, Citation1978), HIWAY-2 (Petersen, Citation1980), CALINE-4 (Benson, Citation1992), GFLSM (Luhar and Patil, Citation1989), ROADWAY (Eskridge and Catalano, Citation1987), OMG (Kono and Ito, Citation1990), OSPM (Berkowicz, Citation2000), CAR-FMI (Kukkonen et al., Citation2001; Harkonen et al., Citation1995), and ADMS (CERC, Citation2006). A detailed review of such models can be found in Holmes and Morawska (Citation2006).
Dispersion models have been used to study the road traffic contribution near the roads. Tiitta et al. (Citation2002) modeled PM2.5 concentrations near a major road in Kuopio, Finland and compared with the data from simultaneous measurements at increasing distance from a road to study dispersion characteristics. They found that traffic emissions caused an increase in concentrations of approximately 30% from the nearest to the largest distances. Kauhaniemi et al. (Citation2008) presented a modeling system to model the dispersion of PM2.5 on an urban scale, combined with a statistical model for estimating the contribution of long-range transported aerosols to the Helsinki urban area. They found that long-range transport (LRT) to the street level PM2.5 varied spatially from 40% in the most trafficked areas to nearly 100% in the outskirts of the area. Yura et al. (Citation2007) have explored the capabilities of CALINE4 to model PM2.5 near roads in suburban (Sacramento, CA) and urban (London, UK) locations.
London is one of the largest cities in Europe, with a population more than 8 million people as per 2011 census (ONS, 2012). It is estimated that 45 billion vehicle-kilometers are traveled on London roads annually, leading to 1.5 kT/yr of PM2.5 emissions (GLA, 2010). A few London-specific modeling studies have been reported in the literature. Beevers et al. (Citation2013) validated the KCLurban dispersion model against annual mean PM2.5 measurements and performed source apportionment modeling at selected sites in London. They reported the regional background to be the largest component contributing to the total PM2.5 concentration; however, the roadside emissions near busy roads could double the concentration.
Within this study we use the OSCAR Air Quality Assessment system to quantify contributions of road traffic to PM2.5 concentrations in London. Previous evaluation of the OSCAR system has been reported by Sokhi et al. (Citation2008) for measurements at Cromwell Road (London) and Vallila (Helsinki, Finland). The first version of the OSCAR system was designed to address only a modest number of road links to enable air quality predictions on a neighborhood scale.
The current, revised version of the OSCAR system is capable of handling the whole road network in major cities and a metropolis such as London. The model also includes new treatments for up-to-date emission standards, and it is straightforward to modify these standards. For example, it is possible to study the impact of various future emission mitigation scenarios, by using assumptions about the changes in fleet composition as per fuel type, vehicle weight, engine size, and new emission standards for newer vehicles. A similar policy-oriented model chain has been presented by Lefebvre et al. (Citation2013); their modeling consisted of an activity-based transport model, a road traffic emission model, a bi-Gaussian atmospheric dispersion model, and a concentration measurement interpolation model.
The first aim of this paper is to present the extended performance evaluation of the OSCAR system by comparing model outputs with extensive PM2.5 measurements across London. Such comprehensive evaluations of urban modeling systems in megacities have previously been very scarce. This study is also the first extensive evaluation of the OSCAR modeling system in a major city. The second aim is to examine the spatial extent of the pollution across London in 2008, both for the total concentrations and for the concentrations originating from urban road transport sources. In particular, we estimate the spatial distribution of the contribution of traffic to total PM2.5 concentrations, and compare it to contributions from other urban sources and regional backgrounds derived from measurement stations.
Methodology
Monitoring sites
The model results have been evaluated at 18 monitoring stations from the London air quality network (LAQN). These include 4 urban background, 12 roadside, and 2 curbside stations. Curbside stations are the sites with sample inlets within 1 m of the curb of a busy road, while roadside stations are sites with sample inlets between 1 m and 5 m of the curbside. Sampling heights for both types of sites are within 2–3 m of the ground. The monitoring stations were selected based on the availability of PM2.5 data for the year 2008. We have also not evaluated the model at suburban and industrial stations, as the main emphasis of this paper is given to road traffic emissions.
The details of the monitoring stations for PM2.5 are shown in . The station classification has been adapted from the London air quality network, and it is slightly different from the classification recommended in the relevant European Union (EU) directives. The visual representations of the location of all stations are shown in .
Table 1. Modeled and observed annual mean PM2.5 concentrations at LAQN sites in London in 2008. This also shows average daily traffic (ADT) and mean speed of the nearest roads for the roadside and kerbside stations
Figure 1. Study domain showing the road and street network, and location of the selected PM2.5 measurement locations in 2008. The geographical extent of the domain is 61 km × 52 km. Central London is located in the center of the domain with a dense road network. The suburban areas are in the outskirts with a relatively more sparse road network.
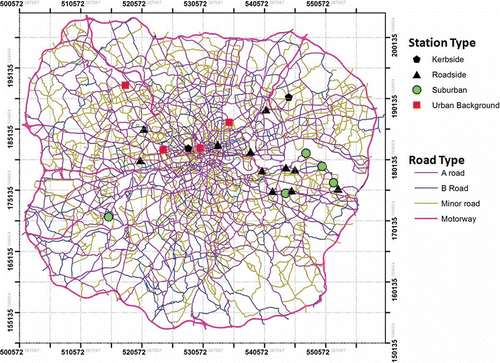
Road traffic data
The road traffic data for London have been obtained from the London Atmospheric Emission Inventory (LAEI; GLA, 2010). depicts the geographical layout of the 63726 road links in London, showing motorways and major and minor roads. Motorways are grade-separated expressways with higher average daily traffic (ADT) and speed. A-roads are the major routes that may vary from motorway standard to narrow local roads but overall with less ADT than motorways. B-roads are local routes interconnecting the road network in London. The unclassified roads that have been included in the study are marked as minor roads. We have included most of such unclassified roads, which have been connected with other major roads in London. There are also other unclassified roads that connect minor roads to residential properties; these have not been considered in this study. However, the average daily traffic of such other unclassified roads is less than 0.1% of that of the included unclassified roads.
Each road link is characterised by the amount of vehicles per day per vehicle category and mean speed. The traffic activity data are disaggregated by vehicle categories such as motorcycles, cars including taxis, buses, light goods vehicles (LGV), and heavy goods vehicles (HGV). The HGVs are further subdivided into articulated HGVs and rigid HGVs categories. The fleet compositions have been further subdivided as per fuel type, vehicle weight, engine size, and emission standards. The segregation of vehicle types into 265 categories has been included in the current OSCAR system and the fractions of disaggregated vehicle categories have been obtained from LAEI.
Model setup details
The OSCAR Air Quality Assessment System (Sokhi et al. Citation2008) has been set up to model traffic-related PM2.5 at high resolution near the whole road network across London for the year 2008. The domain in this study, showing different types of road and street networks, is shown in . The models within the OSCAR system consist of an emission model, a meteorological preprocessing model, and a line source Gaussian dispersion model (CAR-FMI). The OSCAR system calculates emission from line sources to predict hourly concentration at the defined high resolution receptor points placed at varying distance of 10, 40, and 90 m near both sides of the roads and 100 m apart in the outskirts.
The calculated concentrations are then compared with the LAQN measurements. A brief description of the components of the OSCAR system is discussed next. Detailed information can be found in Sokhi et al. (Citation2008).
Emission model
The emission model in the current version of the OSCAR system uses the emission functions and factors based on the COPERT IV (Gkatzoflias et al., Citation2012) and Department for Transport (DfT) emission database as used in the London Atmospheric Emission Inventory. The previous version of the OSCAR system reported by Sokhi et al. (Citation2008) used emission estimates bases on COPERT III (Ntziachristos and Samaras, Citation2000). The basic formula to calculate the total emission from the line source remains same; however, new emission factors have been used in the current calculations. The emission rate Q (μg m−1 s−1) of a line source has been calculated as the product of number of vehicles per hour nj and emission factor qj (g/km) summed over the 265 vehicle categories, as follows:
The emission factors have been calculated as a sixth-order polynomial function of speed,
where q is the emissions in g/km, x is the speed in km/hr, k is the adjustment factor, and a, b, c, d, e, f, and g are polynomial coefficients. The function coefficients have been taken from Boulter et al. (Citation2009).
In addition to exhaust emissions of particulate matter, PM2.5 emissions due to tire and brake wear have also been included from UK National Atmospheric Emission Inventory (NAEI). The resuspension of material deposited on the road surface have not been taken into account; its contribution has been estimated to be smaller than that of tire and brake wear in the conditions of the United Kingdom (Beevers et al., Citation2013). The emission model also does not take into account the congestion of traffic.
All the road links have been treated as a straight line of finite length and the traffic volume of a road during each hour is assumed to be constant. The total emissions from each line source are then segregated using hourly, weekly, and monthly profiles. A common temporal traffic profile has been used for all roads in London.
Meteorological model
A range of hourly parameters is needed, including wind speed, solar radiation, friction, velocity, and Monin–Obukhov length. These are provided by the dedicated OSCAR met preprocessor GAMMA met described by Bualert (Citation2002). The meteorological model employs meteorological data, such as solar radiation, roughness length, and heat flux, to estimate atmospheric stability parameters, including the Monin–Obukhov lengths and mixing heights. Further details of the meteorological model can be found in Bualert (Citation2002). The effects of land use characteristics on parameters such as surface roughness, Bowen ratio, albedo, and anthropogenic heat flux are taken into account in the calculation. The meteorological preprocessor needs six meteorological input parameters: time (month, day, hour), wind speed (m/s or knots), wind direction (degrees), ambient temperature, cloud cover (Okta), and global radiation (W/m2).
Line source dispersion model
The CAR-FMI model (Kukkonen et al., Citation2001; Harkonen et al., Citation1995) is used within the OSCAR system to calculate the dispersion of emissions from the roads. The model computes an hourly time series of the pollutant dispersion from the line source, based on the inputs from the emission model and meteorological model. The dispersion equation for the line source model is based on a semianalytical solution of the Gaussian diffusion equation for a finite line source. The dispersion parameters are modeled as a function of the Monin–Obukhov length, the friction velocity, and the mixing height following the formulation of Gryning et al. (Citation1987). Traffic-originated turbulence is modeled with a semiempirical treatment (Petersen, Citation1980). For this study, we did not use model treatments for processes such as resuspension or removal through wet deposition. The model does not allow for the possible formation of secondary particles in an urban area, due to chemical and physical transformation processes.
This CAR-FMI model has been previously evaluated against the measured data of urban measurement networks in the Helsinki Metropolitan Area and against limited data in London both (i) for gaseous pollutants (e.g., Karppinen et al., Citation2000; Kousa et al., Citation2001) and (ii) for PM2.5 (Kauhaniemi et al., Citation2008; Sokhi et al., Citation2008). The performance of the CAR-FMI model has also been evaluated against the results of a field measurement campaign and against other models (Kukkonen et al., Citation2001; Öttl et al., 2001; Levitin et al., Citation2005).
Regional and urban background concentrations
Regional and urban background levels of PM2.5 were taken from Grice et al. (Citation2009). These contain regional and urban background annual mean concentrations of PM2.5 on a 1-km × 1-km grid resolution in 2008 for the whole United Kingdom. The background concentrations are based on the National Atmospheric Emissions Inventory (Dore et al., 2008). This includes regional and urban emissions from domestic heating, agriculture, combustion, and processes in industries, construction, energy production, quarries, solvents, waste, shipping, and aviation. Also, secondary PM2.5 is included. Urban traffic increments in London were excluded from these background values to avoid double counting.
However, these regional and urban background concentration values have been reported only as annual averages. We have therefore used the measured hourly time series from the urban background station at Camden–Bloomsbury, to allow for the temporal variability. The station of Camden–Bloomsbury was selected as it has been evaluated to be representative for the urban background in Central London by the local authorities. However, the measured temporal variation at Camden–Bloomsbury also includes the influence of urban traffic, although this in principle should be excluded from this temporal variation. This procedure therefore provides only an approximation of the temporal variation of the regional and urban background from other sources, except for urban traffic.
Results and Discussion
Model evaluation
The output of the dispersion model consists of hourly time series of PM2.5 concentrations for 2008. The concentrations were computed in an adjustable grid. The receptor grid intervals ranged from approximately 10 m in the vicinity of the roads to 100 m on the outskirts of the area. The total number of receptor points in the domain is over 200,000. Fine particulate matter (PM2.5) was treated as a tracer; that is, neither chemical reactions nor aerosol processes were included in the calculations.
The results have been analysed as per the DEFRA (Derwent et al., Citation2010) and FAIRMODE recommendations (European Environment Agency [EEA], 2011). The statistical indexes used in this study to evaluate the model performance are correlation coefficient, mean error, mean absolute error (MAE), normalized mean gross error (NMGE) standard deviation, index of agreement (IOA) and within factor of two (FAC2).
For temporal evaluation, hourly modeled time series have been compared with the observed time series. We present the daily mean modeled and observed concentrations at three selected sites in . We have selected a representative site in terms of model performance, for curbside (MY1), roadside (GB6), and urban background (HK4). The agreement of predictions and measurements is fairly good at the roadside and urban background stations, but worse at the curbside station. The maximum concentrations occur in late winter and spring, in both the measured and the predicted results.
Figure 2. Time-series plot of modeled and observed daily mean concentrations at three selected sites in London in 2008.
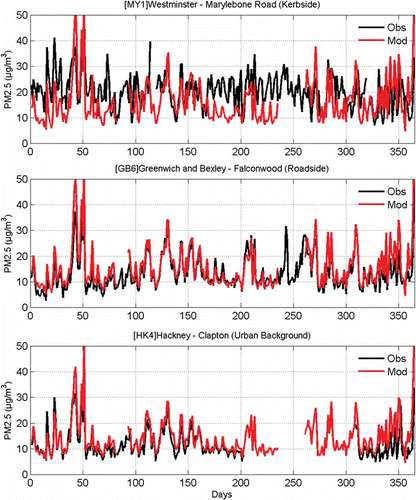
The scatter plots of the hourly modeled and observed concentrations at the same sites are shown in . For the curbside station, the scatter of these points is the largest, and the model underpredicts the measured values. One reason for the model underprediction at this curbsite station is that the emission modeling does not take into account the frequently occurring traffic congestion. Marylebone Road is one of the most densely trafficked streets in London. The emissions of particulate matter can be substantially higher than predicted for severely congested traffic, due to, for example, frequent traffic acceleration and deceleration. Another reason is that dispersion modeling did not taken into account the influence of individual buildings; annual averaged concentrations in street canyons and in the presence of high buildings are therefore underpredicted.
Figure 3. Scatter plot of modeled and observed hourly concentrations at three selected sites. These scatter plots include the counts of the data point to allow clearer interpretation of the results. The dotted lines indicate a factor of 2. The equation of the linear fit, R2, and correlation (ρ) are shown in the top left corner of each panel.
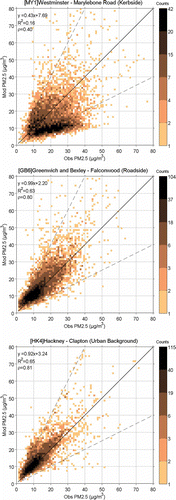
Aggregated statistics for all stations are shown in terms of a box plot in . It can be seen that the modeled time series is highly correlated with observed time series, showing a median correlation of around 0.8 and MAE of 4.1 μg/m3. The median of the index of agreement between modeled and observed hourly concentrations is 0.86 and almost 74% values fall with a factor of two. The overall good agreement of the measured and predicted time series is partly caused by the use of the measured temporal variation (at Camden–Bloomsbury) in the determination of the regional and urban background concentrations. Clearly, another major factor is the modeling of the local traffic contributions, as their temporal variations are more pronounced, compared with those of the urban and regional background contributions.
Figure 4. Box and whisker plot of the model performance evaluated against measured time series at 17 monitoring sites. The line in the middle of each box is the median. The tops and bottoms of each rectangle are the 25th and 75th percentiles. The whiskers (lines extending above and below each box) show the data range. Data beyond the whisker length are marked as outliers shown with + symbol. CORR: correlation, IOA: index of agreement, FAC2: factor of 2.
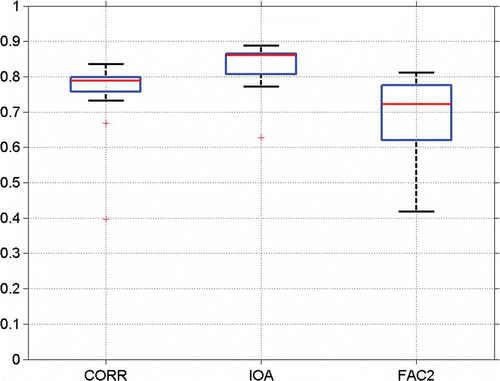
The model performance clearly deteriorates for some of the most densely trafficked sites and for the sites located in central London. At Marylebone Road in central London, the correlation is much lower than for all the other selected stations (0.40).
In order to do the spatial evaluations across different sites in London, the annual mean modeled concentrations have been compared with the annual mean observations. The comparison between modeled and observed annual mean PM2.5 concentrations is shown in . The table also shows the average daily traffic (ADT) and mean speed of the roads in km/h nearest to the roadside and curbside stations in 2008. The calculated correlation between concentrations at the sites near the road and ADT is found to be 0.58.
The scatter plot of the modeled against observed annual mean concentrations at various sites is shown in . The modeled concentrations show a reasonable agreement with the observations; all the values fall within factor of 1.5 from the central line. The aggregated statistics for all 18 stations are shown in . The correlation between modeled and observed annual mean concentrations is 0.52, and the index of agreement is 0.60. The already-mentioned correlation represents the ability of the model to simulate small-scale spatial variation of concentrations in urban area.
Figure 5. The scatter plot of the modeled against observed annual mean concentrations at urban background (circle), roadside (diamonds) and curbside (square) sites. There are 4 urban background, 12 roadside, and 2 curbside stations.
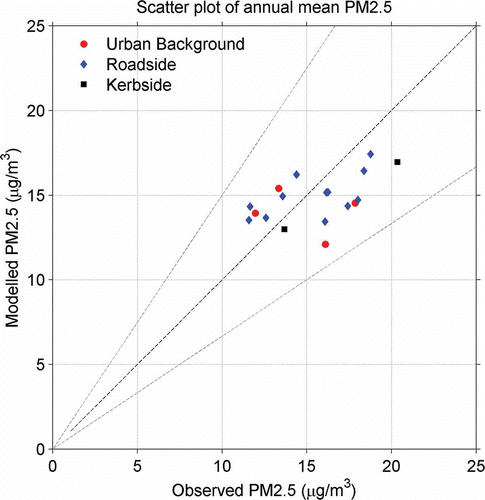
Table 2. Spatial evaluation statistics for annual mean PM2.5 using modeled against LAQN measurements at 18 sites
Variation of roadside PM2.5 concentrations with distance
The variation of mean modeled PM2.5 concentrations resulting from traffic emissions against perpendicular distances is shown in for motorways, major roads (ADT > 30,000 per day), and all other roads in London. The distances in the figure are measured from the center lines of the roads. The traffic contribution of PM2.5 from motorways decreases more rapidly compared to other roads, with modeled PM2.5 concentrations falling by 30% over the first 100 m. In addition to motorways representing the strongest emission source strength, there are commonly very few other roads near the motorways to influence the motorway contribution. Near motorways the traffic increment can be as much as 6 μg/m3, but after about half a kilometer away the traffic increment is reduced to approximately one-third of the maximum value. For major roads, the traffic increment can be as much as 2.5 μg/m3 across the dense road network in London. The contribution of PM2.5 from other (smaller) roads merges quickly with the overall urban traffic increment.
Traffic contribution to the urban increment
shows the box plot of the observed annual mean PM2.5 concentrations at different site types. The so-called busy roads have been defined as having an average daily traffic (ADT) > 30,000 vehicles. The regional contribution, observed at Harwell station, was around 9.8 μg/m3 for the year 2008. The concentration of PM2.5 increases as we go toward the London center due the emissions from urban sources including traffic. The amount of increase in the concentrations to the regional background is defined as urban increment. Moreover, the traffic increment, a part of urban increment, is defined as the contribution coming from the traffic at given location. In this paper we have considered only urban traffic on the road network shown in .
Figure 7. Box and whisker plot of the observed annual mean PM2.5 concentrations at different site types. Each whisker plot contains the variation of annual mean concentrations within a range of sites. The figure therefore also illustrates the total urban increments (i.e., the additional concentrations, compared with the rural background) at various site categories. The line in the middle of each box is the median. The tops and bottoms of each rectangular box are the 25th and 75th percentiles; the distances between the tops and bottoms are the inter-quartile ranges. Busy roads have been defined as the roads with ADT > 30,000 vehicles.
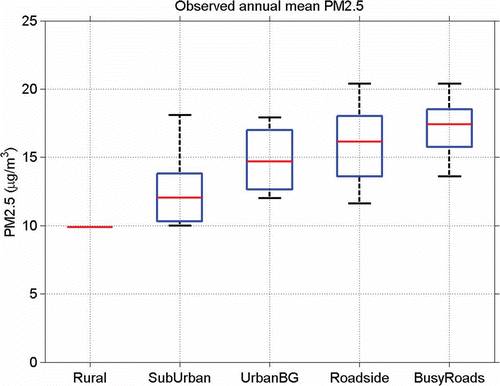
At the suburban station, the median of urban increment in the annual mean PM2.5 is around 2.2 μg/m3, which increases to 4.8 μg/m3 at the urban background location. The medians of the total urban increment at roadside and busy road side sites are found to be 6.3 and 7.5 μg/m3, respectively. However, the total variabilities of all the urban increments (suburban, urban background, roadside, and busy roads) are overlapping with respect to each other. In particular, the measured annual average concentration at Marylebone Road is 20 μg/m3, which is more than double the regional background level. The predicted PM2.5 concentrations could be up to 35 μg/m3 close to motorways, which is more than three times the regional background level. However, less than 0.05% of the population of greater London lives within 100 m of the motorways.
In order to quantify the amount of addition from London traffic sources to the total urban increment, shows a stacked bar plot of the total PM2.5 at different sites where regional background, median traffic, and median urban from other sources increments have been shown separately. It has been estimated that the traffic emissions contribute 0.49 ± 0.12, 1.21 ± 0.58, 1.89 ± 1.09, and 2.48 ± 0.91 μg/m3 at suburban, urban background, roadside, and busy roadside sites, respectively. The variability shown here is the standard deviation of the traffic contribution for the given site type.
Spatial distribution of PM2.5
The spatial distribution of the modeled annual mean PM2.5 concentrations for the year 2008 is shown in . Clearly, the highest concentrations occur near busy roads and motorways, at their junctions, and in the center of London. The modeled annual mean PM2.5 concentration in central London is between 14 and 17 μg/m3, which decreases to around 10–14 μg/m3 in the suburban area. shows the contribution of urban traffic as a percentage of the total PM2.5 concentration. The mean traffic increment of PM2.5 can be as high as 50% near motorways, and from 10 to 20% in the vicinity of the most busy other roads and in the center of London.
Conclusion
Traffic contributions to PM2.5 concentrations in London have been modeled with the OSCAR air quality assessment system at high resolution across London for the year 2008. In order to conduct the analysis of traffic increments to PM2.5 levels, the OSCAR system has gone through a number of improvements, including meteorological preprocessor, traffic emissions processor, and geospatial analysis capabilities. The extended OSCAR system has been evaluated with measurements from 18 LAQN monitoring roadside and background stations across London.
Model performance
The statistical metrics show that the model performs well in terms of capturing the temporal variations of PM2.5. Regarding the agreement of the hourly time series of measured and predicted concentrations, the medians of correlation coefficient, IA and FAC2 were calculated to be 0.80, 0.86, and 74%, respectively. However, the model underestimated the concentrations at the most busy road sites in central London.
The underestimation is partly caused by frequently occurring traffic congestion, when the vehicles are stationary or very slowly moving, as the emission modeling does not currently take into account such conditions. An additional source of uncertainty is the differing street geometries, which have not been addressed in the current analysis. We have also not allowed for the resuspension of particles from road surfaces. These factors may also explain the underestimation of the modeled PM2.5 concentrations near roads.
Dispersion characteristics of PM2.5 near roads
In terms of dispersion properties of PM2.5, the traffic contribution from motorways decreases more rapidly compared to other roads due to higher emissions; commonly there are very few other roads near the motorway to influence the motorway contribution. For major roads, the traffic increment can be as much as 2.5 μg/m3 across the dense road network in London. The contribution of PM2.5 from other roads with less traffic volume is small, and merges very quickly with the overall urban traffic increment. Moreover, a constant level of traffic-related PM2.5 is maintained across the dense road network in London.
Urban increments to PM2.5 concentrations
This study shows that the urban increment including the traffic contribution in London is very significant and the total concentrations can therefore be up to three times the regional background near major roads. As expected, the increment is higher near the busy roads, and lower at urban background and suburban locations. The overall urban increment was evaluated to be 18%, 33%, 39%, and 43% of the total PM2.5 in suburban environments, in the urban background, near roads, and near busy roads, respectively.
The traffic increment has almost a two-thirds contribution coming from exhaust emissions and the remainder is due to brake and tire wear. The spatial distribution of the percentage traffic increment across London shows extensive heterogeneity and expectedly is closely associated with the road network across the city. The mean overall traffic increment of primary PM2.5 can be as high as 50% of the total concentrations near motorways.
Funding
This work was supported by 7th Framework Programme project TRANSPHORM (grant agreement 243406) and the APTA project funded by the Academy of Finland.
Additional information
Notes on contributors
Vikas Singh
Vikas Singh is a research fellow and Ranjeet S. Sokhi is a research professor and director of the Centre of Atmospheric and Instrumentation Research (CAIR), University of Hertfordshire, Hatfield, UK.
Ranjeet S. Sokhi
Vikas Singh is a research fellow and Ranjeet S. Sokhi is a research professor and director of the Centre of Atmospheric and Instrumentation Research (CAIR), University of Hertfordshire, Hatfield, UK.
Jaakko Kukkonen
Jaakko Kukkonen is a research professor at the Finnish Meteorological Institute in Helsinki, Finland.
References
- Ashmore, M. 2005. Quantification of population exposure benefits of reducing roadside and urban background pollution concentrations. Reports of the UK Department of Health Policy Research Programme. http://webarchive.nationalarchives.gov.uk/20130107105354/http://www.dh.gov.uk/prod_consum_dh/groups/dh_digitalassets/@dh/@en/documents/digitalasset/dh_092835.pdf.
- Beelen, R., G. Hoek, D. Vienneau, M. Eeftens, K. Dimakopoulou, X. Pedeli, M.-Y. Tsai, et al. 2013. Development of NO2 and NOx land use regression models for estimating air pollution exposure in 36 study areas in Europe—The ESCAPE Project. Atmospheric Environment 72(June): 10–23. doi:10.1016/j.atmosenv.2013.02.037
- Beevers, S.D., N. Kitwiroon, M.L. Williams, F.J. Kelly, H.R. Anderson, and D.C. Carslaw. 2013. Air pollution dispersion models for human exposure predictions in London. Journal of Exposure Science and Environmental Epidemiology (February 27). doi:10.1038/jes.2013.6 http://www.nature.com/jes/journal/vaop/ncurrent/abs/jes20136a.html.
- Benson, P.E. 1992. A review of the development and application of the CALINE3 and 4 models. Atmos. Environ. Part B. Urban Atmosphere 26 (3): 379–390. doi:10.1016/0957-1272(92)90013-I
- Berkowicz, R. 2000. OSPM—A parameterised street pollution model. Environ. Monit. Assess. 65(1–2): 323–331. doi:10.1023/A:1006448321977
- Boulter, P.G., T.J. Barlow, and I.S. McCrae. 2009. Road vehicle emission factors 2009. Department for Transport. https://www.gov.uk/government/publications/road-vehicle-emission-factors-2009.
- Bualert, S. 2002. Development and application of an advanced Gaussian urban air quality model. University of Hertfordshire, Hatfield, Hertfordshire, UK.
- CERC. 2006. AMDS-Roads, An air quality management system user guide. Cambridge, UK: Cambridge Environmental Research Consultants Ltd.
- Chock, D.P. 1978. A simple line-source model for dispersion near roadways. Atmos. Environ. 12 (4): 823–829. doi:10.1016/0004-6981(78)90019-7
- Derwent, D., A. Fraser, J. Abbott, M. Jenkin, P. Willis, and T. Murrells. 2010. Evaluating the performance of air quality models. Issue 3. DEFRA. http://uk-air.defra.gov.uk/reports/cat05/1006241607_100608_MIP_O_Final_Version.pdf
- Dore, C.J., et al. 2008. UK emissions of air pollutants 1970 to 2006. http://uk-air.defra.gov.uk/reports/cat07/0810291043_NAEI_2006_Report_O_Final_Version(3).pdf
- Eeftens, M., M.-Y. Tsai, C. Ampe, B. Anwander, R. Beelen, T. Bellander, G. Cesaroni, et al. 2012. Spatial variation of PM2.5, PM10, PM2.5 absorbance and PMcoarse concentrations between and within 20 European study areas and the relationship with NO2—Results of the ESCAPE project. Atmos. Environ. 62(December): 303–317. doi:10.1016/j.atmosenv.2012.08.038
- Eskridge, R., and J. Catalano. 1987. ROADWAY—A numerical model for predicting air pollutants near highways—User’s guide. EPA-68-02-4106. Research Triangle Park, NC: U.S. Environmental Protection Agency
- European Environment Agency. 2011. The application of models under the European Union’s Air Quality Directive: A technical reference guide. Technical report no 10/2011. EEA. http://www.eea.europa.eu/publications/fairmode
- Gkatzoflias, D., C. Kouridis, L. Ntziachristos, and Z. Samaras. 2012. COPERT 4, Computer programme to calculate emissions from road transport. User manual. v. 9.0. European Environment Agency. http://www.emisia.com/docs/COPERT4v9_manual.pdf
- GLA. 2010. The London Atmospheric Emissions Inventory 2008. http://data.london.gov.uk/laei-2008
- Grahame, T.J., and R.B. Schlesinger. 2010. Cardiovascular health and particulate vehicular emissions: A critical evaluation of the evidence. Air Quality, Atmosphere and Health 3 (1): 3–27. doi:10.1007/s11869-009-0047-x
- Grice, S., S.L. Cooke, J.R. Stedman, T.J. Bush, K.J. Vincent, M. Hann, J. Abbott, and A.J. Kent. 2009. UK air quality modeling for annual reporting 2007 on ambient air quality assessment under Council Directives 96/62/EC, 1999/30/EC and 2000/69/EC. ED 48208 AEAT/ENV/R/2656, Issue 1. http://laqm.defra.gov.uk/documents/0905061048_dd12007mapsrep_v8.pdf.
- Gryning, S.E., A.A.M. Holtslag, J.S. Irwin, and B. Sivertsen. 1987. Applied dispersion modeling based on meteorological scaling parameters. Atmos. Environ. Part A Gen. Topics 21 (1): 79–89.doi:10.1016/0004-6981(87)90273-3
- Harkonen, J., E. Valkonen, J. Kukkonen, E. Rantakrans, L. Jalkanen, and K. Lahtinen. 1995. Operational dispersion model for predicting pollution from a road. Int. J. Environ. Pollut. 5(4–6): [d]602–610.
- Holmes, N.S., and L. Morawska. 2006. A review of dispersion modeling and its application to the dispersion of particles: An overview of different dispersion models available. Atmos. Environ. 40 (30): 5902–5928. doi:10.1016/j.atmosenv.2006.06.003
- Karppinen, A., J. Kukkonen, T. Elolähde, M. Konttinen, and T. Koskentalo. 2000. A modeling system for predicting urban air pollution: Comparison of model predictions with the data of an urban measurement network in Helsinki. Atmos. Environ. 34 (22): 3735–3743. doi:10.1016/S1352-2310(00)00073-X
- Kauhaniemi, M., A. Karppinen, J. Härkönen, A. Kousa, B. Alaviippola, T. Koskentalo, P. Aarnio, T. Elolähde, and J. Kukkonen. 2008. Evaluation of a modeling system for predicting the concentrations of PM2.5 in an urban area. Atmos. Environ. 42 (19): 4517–4529. doi:10.1016/j.atmosenv.2008.01.071
- Kono, H., and S. Ito. 1990. A comparison of concentration estimates by the OMG VOLUME-SOURCE dispersion model with three line source dispersion models. Atmos. Environ. Part B Urban Atmos. 24 (2): 253–260. doi:10.1016/0957-1272(90)90030-X
- Kousa, A., J. Kukkonen, A. Karppinen, P. Aarnio, and T. Koskentalo. 2001. Statistical and diagnostic evaluation of a new-generation urban dispersion modeling system against an extensive dataset in the Helsinki area. Atmos. Environ. 35 (27): 4617–4628. doi:10.1016/S1352-2310(01)00163-7
- Kukkonen, J., J. Härkönen, J. Walden, A. Karppinen, and K. Lusa. 2001. Evaluation of the CAR-FMI model against measurements near a major road. Atmos. Environ. 35 (5): 949–960. doi:10.1016/S1352-2310(00)00337-X
- Lefebvre, W., B. Degrawe, C. Beckx, M. Vanhulsel, B. Kochan, T. Bellemans, D. Janssens, et al. 2013. Presentation and evaluation of an integrated model chain to respond to traffic- and health-related policy questions. Environ. Model. Software 40(February): 160–170. doi:10.1016/j.envsoft.2012.09.003
- Levitin, J., J. Härkönen, J. Kukkonen, and J. Nikmo. 2005. Evaluation of the CALINE4 and CAR-FMI models against measurements near a major road. Atmos. Environ. 39 (25): 4439–4452. doi:10.1016/j.atmosenv.2005.03.046
- Luhar, A.K., and R.S. Patil. 1989. A general finite line source model for vehicular pollution prediction. Atmos. Environ. 23 (3): 555–562. doi:10.1016/0004-6981(89)90004-8
- Ntziachristos, L., and Z. Samaras. 2000. COPERT III. Computer program to calculate emissions from road transport. methodology and emission factors (version 2.1). 49. European Environment Agency. http://www.eea.europa.eu/publications/Technical_report_No_49
- Oettl, D., J. Kukkonen, R.A. Almbauer, P.J. Sturm, M. Pohjola, and J. Härkönen. 2001. Evaluation of a Gaussian and a Lagrangian model against a roadside data set, with emphasis on low wind speed conditions. Atmos. Environ. 35 (12): 2123–2132. doi:10.1016/S1352-2310(00)00492-1
- ONS. 2012. 2011 Census—Population and household estimates for England and Wales, March 2011. Statistical Bulletin, Office for National Statistics, UK. http://www.ons.gov.uk/ons/dcp171778_270487.pdf
- Petersen, W. 1980. User’s guide for HIWAY2, a highway air pollution model. EPA-600/8-80-018. Research Triangle Park, NC: U.S. Environmental Protection Agency.
- Pope, C.A., and D.W. Dockery. 2006. Health effects of fine particulate air pollution: Lines that connect. J. Air Waste Manage. Assoc. 56 (6): 709–742. doi:10.1080/10473289.2006.10464485
- Rohr, A.C., and R.E. Wyzga. 2012. Attributing health effects to individual particulate matter constituents. Atmos. Environ. 62(December): 130–152. doi:10.1016/j.atmosenv.2012.07.036
- Singh, V., C. Carnevale, G. Finzi, E. Pisoni, and M. Volta. 2011. A cokriging based approach to reconstruct air pollution maps, processing measurement station concentrations and deterministic model simulations. Environ. Model. Software 26 (6): 778–786. doi:10.1016/j.envsoft.2010.11.014
- Sokhi, R.S., H. Mao, S.T.G. Srimath, S. Fan, N. Kitwiroon, L. Luhana, J. Kukkonen, et al. 2008. An integrated multi-model approach for air quality assessment: Development and evaluation of the OSCAR air quality assessment system. Environ. Model. Software 23):(3) 268–281. doi:10.1016/j.envsoft.2007.03.006
- Tiitta, P., T. Raunemaa, J. Tissari, T. Yli-Tuomi, A. Leskinen, J. Kukkonen, J. Härkönen, and A. Karppinen. 2002. Measurements and modeling of PM2.5 concentrations near a major road in Kuopio, Finland. Atmos. Environ. 36 (25): 4057–4068. doi:10.1016/S1352-2310(02)00309-6
- Yura, E.A., T. Kear, and D. Niemeier. 2007. Using CALINE dispersion to assess vehicular PM2.5 emissions. Atmos. Environ. 41 (38): 8747–8757. doi:10.1016/j.atmosenv.2007.07.045