Abstract
Several studies indicate that mortality and morbidity can be well correlated to atmospheric aerosol concentrations with aerodynamic diameter less than 2.5 µm (PM2.5). In this work the PM2.5 at Recife city was analyzed as part of a main research project (INAIRA) to evaluate the air pollution impact on human health in six Brazilian metropolitan areas. The average concentration, for 309 samples (24-hr), from June 2007 to July 2008, was 7.3 µg/m³, with an average of 1.1 µg/m³ of black carbon. The elemental concentrations of samples were obtained by x-ray fluorescence. The concentrations were then used for characterizing the aerosol, and also were employed for receptor modelling to identify the major local sources of PM2.5. Positive matrix factorization analysis indicated six main factors, with four being associated to soil dust, vehicles and sea spray, metallurgical activities, and biomass burning, while for a chlorine factor, and others related to S, Ca, Br, and Na, we could make no specific source association. Principal component analysis also indicated six dominant factors, with some specific characteristics. Four factors were associated to soil dust, vehicles, biomass burning, and sea spray, while for the two others, a chlorine- and copper-related factor and a nickel-related factor, it was not possible to do a specific source association. The association of the factors to the likely sources was possible thanks to meteorological analysis and sources information. Each model, although giving similar results, showed factors’ peculiarities, especially for source apportionment. The observed PM2.5 concentration levels were acceptable, notwithstanding the high urbanization of the metropolitan area, probably due to favorable conditions for air pollution dispersion. More than a valuable historical register, these results should be very important for the next analysis, which will correlate health data, PM2.5 levels, and sources contributions in the context of the six studied Brazilian metropolises.
Implications:
The analysis of fine particulate matter (PM2.5) in Recife city, Brazil, gave a significant picture of the local concentration and composition of this pollutant, which exhibits robust associations to adverse human health effects. Data from 1 year of sampling evaluated the seasonal variability and its connections with weather patterns. Source apportionment in this metropolitan area was obtained based in a combination of receptor models: principal component analysis (PCA)/chemical mass balance (CMB) and positive matrix factorization (PMF). These results give guidelines for local air pollution control actions, providing significant information for a health study in the context of establishing a new national air pollution protocol based on Brazilian cities data.
Introduction
Several studies have been done to analyze air pollutants and their effects on human health. The present one takes part of “INAIRA” (the Brazilian acronym for “National Institute of Integrated Environmental Risk Analysis”), a project sponsored by the Ministry of Science and Technology (MCT), National Council of Research (CNPq), and São Paulo Research Foundation (FAPESP), designed to evaluate the automotive sources contribution to the PM2.5 atmospheric concentrations, and its impacts to human health in six Brazilian metropolitan areas: São Paulo, Rio de Janeiro, Belo Horizonte, Curitiba, Porto Alegre, and Recife. The first results, presenting average concentrations for all cities and the characteristics of samplings, were presented in Miranda et al. (Citation2010).
Various studies indicate that mortality and morbidity are well correlated to the PM2.5 concentrations (e.g., Anderson et al., Citation2011; Pope and Dockery, Citation2006; Pope et al., Citation2009). Nevertheless, this type of evaluation is not straightforward, due to several other factors, specifically those related to meteorological variables and quality of living in the urban areas, that could also impart damage to human health. In this collection of metropolises a high amplitude of values is covered for several characteristics, from geographic positioning and climatic dynamic, to economical and social parameters. The study performed for Recife provided elements, for the next steps of the project, to distinguish between air pollution and other factors that could impact human health.
Recife has 1.55 million inhabitants, being the main city of a metropolitan area (RMR) with 3.69 million people, and capital of the state of Pernambuco. Its shoreline has beautiful beaches and the city is crossed by big rivers, like the Beberibe and the Capibaribe with their tributaries, some islands, and several bridge connections, thus giving its nickname of “Brazilian Venice.” Among the other 14 cities of the RMR, the neighboring city of Olinda is recognized by UNESCO as a World Heritage Site, because of its historical and cultural value. Besides those qualifiers, the RMR has an important economical regional role, and due to the natural propitious attributes of the coastal area, it is an important commercial port. Since the European occupation of the region, in the sixteenth century, the sugarcane crops occupied the area’s fertile lands for the sugar industry. In the last decade this intensive farming supplied also the engines that produce ethanol for fueling vehicles. In both cases, the cane thatch burning, preceding harvesting, imparts a significant environmental impact. In the last decade there has been also a regional increase of industrial activities and of the vehicular fleet.
Until recently, just a few studies about the local air pollution, not related to this project, have been done. More than 30 years ago Van Greken et al. (Citation1982) conducted a pioneering and dense work, analyzing the total suspended particulate matter (TSP) and its size distribution. They identified a restricted number of sources (mainly soil dust and sea spray) due to the sampling characteristics and to the limitations of the available analytical tools. Conference communications show a few other studies, like heavy metals identification in the TSP collected in the local environmental control stations during the year of 1991 (Florêncio et al., Citation1997), or the identification of some chemical elements, by neutron activation analysis, in extracts of bromeliads obtained in two local forestry reserves (Santos et al., Citation2011), and a study of the black carbon concentration variability as a function of rainfall for this project (Picolo et al., Citation2010).
The present study evaluated the concentrations of chemical elements, black carbon, and total mass of PM2.5 samples, from June 2007 to July 2008. This database enabled the use of positive matrix factorization (PMF) and principal component analysis (PCA) and enabled us to obtain absolute factors used in a chemical mass balance (CMB) source apportionment (all referenced in the Methodology section). Confronting the results of these methodologies and using meteorological data as well as local sources mapping, the main source of PM2.5 impacting the samples could be identified. A previous analysis for the identification of sources in the context of INAIRA, for six Brazilian capitals, including Recife, was performed and the results were presented in Andrade et al. (Citation2012). The analysis was undertaken with fewer samples and it was not possible to identify the same number of sources.
The Recife city area is characterized as under a regional shoreline meteorological system with favorable conditions for air pollution dispersion, especially considering the sampling point. The measured PM2.5 concentration levels in the sampling station did not exceeded the World Health Organization (WHO) Guidelines (WHO, Citation2005) during the experiment, notwithstanding the high state of regional urbanization. The results obtained in this work represent a valuable historical register, and will be very important for the main project, which has the objective of correlating health data, PM2.5 levels, and sources responsibility, in the context of six important Brazilian metropolises.
Methodology
shows the Recife metropolitan area, including some potential sources with appreciable magnitude and the sampling station, settled in the campus of the Federal University of Pernambuco (UFPE), at 8°2.93’ S; 34°56.77’ W, at an altitude of 15 m amsl. The sampling period was from June 2007 to July 2008, providing 309 samples of 24 hr each.
Figure 1. Map of Recife. UFPE: sampling station of PM2.5 (8°2.93’ S, 34°56.77’ W, and 15 m amsl). INMET, meteorological station of the National Institute of Meteorology; AER,Weather Station—Airport of Guararapes (REDEMET). (A) Metallurgy, (B) chemical industry, (C) landfill, (D) alcohol plant, (E) sugarcane crop, (F) steel mill, (G) thermoplastic, (H) metallurgy, (I) landfill, (J) port, (K) central commerce area, (L) cement plant, (M) industrial area and port. The detail shows a magnification of the area around the sampling site with the road system and port. (Images from Google Earth: Image © 2013 DigitalGlobe – DATA SIO, NOAA, US Navy, NGA, GEBCO, and for detail, Image © 2013 TerraMetrics, Image © 2013 MapLink, Image © 2013 DigitalGlobe.)
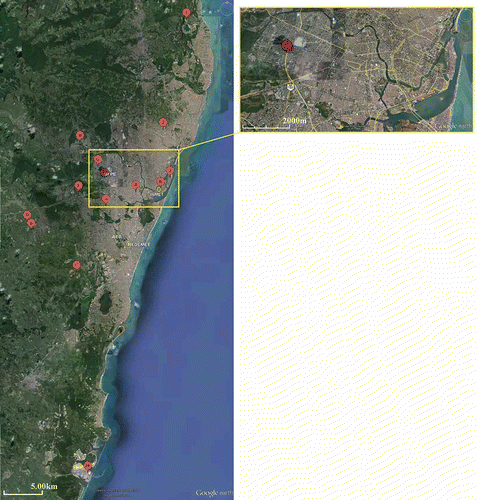
The PM2.5 was collected on 37-mm polycarbonate filters (0.4 μm pore size) using Harvard samplers with a flow rate of 10.0 L/min (Marple and Willeke, Citation1976; Marple et al., Citation1987). The filters were weighted before and after sampling using a balance with a precision of 1 μg, after at least a 24-hr equilibration period inside the weighing room (humidity and temperature controlled: 45 ± 5% relative humidity [RH] and 22 ± 1º C, respectively; Gatti and Kerr, Citation2008), and submitted to a system to eliminate accumulated electrical charges. The volumes to calculate concentrations were given by a volume integrator.
The filters were analysed by energy-dispersive x-ray fluorescence (XRF-ED) for the determination of the concentrations of the elements with atomic number (Z) greater than or equal to 11 (Na). Black carbon (BC) concentrations were obtained by light reflectance. Since this BC measurement depends on the standard used for calibration and on the specific PM composition being analyzed (Baumgardner et al., Citation2012; Quincey et al., Citation2011), these data were corrected using an intercalibration between our reflectometer and thermal/optical transmittance (TOT) equipment (U.S. Environmental Protection Agency [EPA], Citation2012).
Three receptor models were used to identify and estimate the sources impact in the study area: positive matrix factorization (PMF), principal components analysis (PCA) with the determination of absolute factors, and chemical mass balance (CMB).
PMF (Paatero, Citation1997) enables the obtaining of a set of p factors, searching the best solution for the equations relating the data base matrix x, with the factor profiles f and the amount of mass g the factor contributed in each sample:
In this methodology, the determination of uij is an important issue, since each data xij is inversely weighed by this value, impacting the g and f determination. Polissar et al. (Citation1998) used the analytical uncertainty plus LD/3 (1/3 of the limit of detection [LD]) as the total uncertainty of each element, reducing the weight of the elements that were harder to detect. Nevertheless, the uncertainty for analytical methods like XRF-ED has an LD bias because of the background subtraction when integrating the characteristic x-ray peak for each element. Therefore, we opted for not increasing the LD weights beyond this limit. Otherwise, the uij used were a combination (square root of the squared values sum) of the uncertainties due to the analytical method and the general 8% uncertainty associated to the PM2.5 sampling process itself (using the result for paired sampling obtained for Harvard samplers by CitationDionisio et al. [2010]). Doing so, there is a reduction in the range of weights values for the elements more precisely determined by the analytical method, smoothing their role in the f and g determination. Finally, a few missing values, for a few elements [Mg (7.4%), Al (2.3%), Cl (1.6%), S and K (0.65%), Na and Ca (0.32%)], were filled with LD/2 (half the limit of detection), considering equally probable any concentration value between 0 and LD, and adopting (5/6)LD as their analytical method uncertainty (Polissar et al. Citation1998).
The concentration matrix (missing values filled) was also used for PCA, a well-known methodology (e.g., Johnson and Winchern, Citation1982) that seeks the least number (p) of factors (Fk) explaining well the common variance of a database x, in general normalized by its average (μi) and standard deviation (σii); therefore:
The factors were rotated to maximize the variance of its squared loadings (Varimax), given the rotated loadings rlij, used also to calculate fij, the absolute factors profile (Keiding, Citation1986):
CMB is a well-established receptor model, used to evaluate sources participation in the collected samples, assuming that
The fij must be known and the numerical solution is obtained by weighted least squares. With the U.S. EPA software CMB 8.2 (Coulter, Citation2004), we evaluated the source apportionment in the collected PM2.5, using either the sources identified with the absolute factors obtained by PCA, or source profiles from the literature (SPECIATE, for instance, from U.S. EPA [2011]).
Results and Discussion
shows the mass concentrations obtained for PM2.5 during the sampling period. The average concentration for the sampling period was 7.4 μg/m³. Averaging the concentrations month by month (from July 1, 2007, to June 30, 2008), the annual monthly average concentration was 7.3 μg/m³, and no daily concentration exceeds 25 μg/m³, following the WHO guidelines for this pollutant (WHO, Citation2005). Notwithstanding the high state of urbanization of the metropolitan area, the PM2.5 concentrations were acceptable for this period in the sampling station. This most likely is due to the general circulation in the local and to the regional shoreline meteorological system, both favorable to air pollution dispersion.
Figure 2. Daily concentration of PM2.5 for the sampling period. The continuous and the dashed lines show the WHO guidelines for PM2.5 (annual concentrations of 10 μg/m³, and daily concentrations not exceeding 25 μg/m³ in more than 1% of the samples).
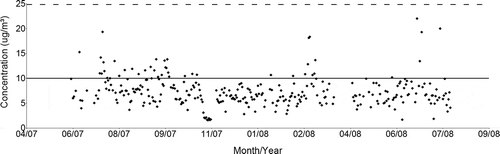
PM2.5 and BC concentrations were reported for this Recife database in previous works (Andrade et al., Citation2012; Miranda et al. Citation2010), registering values higher than that shown here (Table 1) for BC: 1.9 μg/m³ for a global average, or 1.3 μg/m³ and 2.7 μg/m³ for BC in summer and winter periods, respectively. There is a small difference in the number of samples selected, since in this work we are taking only those simultaneously analyzed by XRF and reflectance. However, the main difference was in the methodology adopted after those publications. We used an intercalibration with TOT, as described in the Methodology section, while the previous work calibrated the reflectometer with standard filters coated with a reference BC.
The BC concentrations are presented in . A seasonal trend can be observed in and for the total mass and BC, respectively. The Recife region has two climatic seasons: summer or dry (October to March), and winter or rainy (April to September). The higher concentration levels occurred during the winter period. Although such a result is often expected, it was not obvious for that region, close to the Equator and with the rainy period, propitious to PM removal, occurring during the winter. and show the rainfall distribution during sampling periods, during winter and summer, respectively, making it clear that the winter has a higher precipitation level than summer, and that the last period had 2.6 times more dry days than the first. Otherwise, the wind speed distribution for winter () indicates lower intensities than in summer (), with 8 times more hours of calm winds, which could explain the higher concentration levels in the winter. The decrease in the wind intensity reduces the air pollution dispersion, while the higher insolation in summer has an opposite action, due to the increase of wind speed, of turbulence, and of the mixing layer height. From the wind direction point of view, an opposite behavior would be expected. The prevalent wind direction is from the south to east quarter for both seasons ( and ). Nevertheless, there is an increase of more easterly wind frequencies in summer, probably due to the intensification of the solar radiation and the corresponding intensification of the regional sea breeze circulation pattern (from the east). Easterly wind intensifies the air pollution transport from the downtown zone, an important source of PM, to the sampling point (). Anyway, the prevailing effect observed was the lowering of PM2.5 concentrations during summer.
Figure 3. Daily concentration of black carbon, for the sampling period. The continuous line shows the mean.
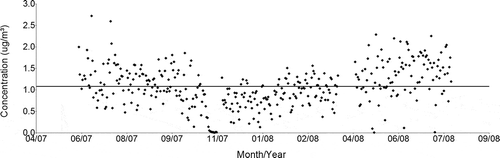
Figure 4. Rainfall amount distribution during the sampling period [database from INMET meteorological station at Recife, INMET (Citation2007)]: (a) winter; (b) summer. The black column indicates days without precipitation.
![Figure 4. Rainfall amount distribution during the sampling period [database from INMET meteorological station at Recife, INMET (Citation2007)]: (a) winter; (b) summer. The black column indicates days without precipitation.](/cms/asset/465ccb1e-d0a8-4a3c-97db-4785b368552c/uawm_a_854282_f0004_b.gif)
Figure 5. Wind intensity distribution [database from REDEMET meteorological station at Recife (REDEMET, Citation2012)]: (a) winter; (b) summer. The black column indicates hours with calm winds (speed < 0.5 m/s).
![Figure 5. Wind intensity distribution [database from REDEMET meteorological station at Recife (REDEMET, Citation2012)]: (a) winter; (b) summer. The black column indicates hours with calm winds (speed < 0.5 m/s).](/cms/asset/2cde8089-eb1d-4148-9fbb-705a56256b57/uawm_a_854282_f0005_b.gif)
Figure 6 Wind direction frequency [database from REDEMET meteorological station at Recife (REDEMET, Citation2012)]: (a) winter; (b) summer.
![Figure 6 Wind direction frequency [database from REDEMET meteorological station at Recife (REDEMET, Citation2012)]: (a) winter; (b) summer.](/cms/asset/82e0cb4e-6d6c-421a-a3db-deeb0900eee7/uawm_a_854282_f0006_b.gif)
Average concentrations, and respective standard deviations, of elements, black carbon, and total mass for the 309 samples are presented in . Winter (rainy) and summer (dry) periods are highlighted.
Table 1. Average concentrations and standard deviation for chemical elements, BC, and total MP2,5 (309 samples)
Analyzing the database by PMF, six factors were identified and associated to regional sources (): (1) metallurgy, (2) Cl sources, (3) Na/S/Ca/Br source, (4) soil dust, (5) biomass burning, and (6) vehicles and sea spray.
Table 2. PMF analysis indicating the percentage of each element in the extracted six factors, with the predominant elements on each factor boldfaced
In are presented the rotated loadings (VARIMAX method) obtained for the six factors extracted by PCA analysis, using the same database. In are presented the absolute factors obtained using the PCA loadings calculated as presented in eq 5. The elements are grouped following the intensity of the loadings for each factor (the high loading values in each factor are bold marked). In the last line below the factor number is the percentage of database variance explained by each factor. The six factors explained 78.5% of the total database variance. The source association for each factor is also indicated in the table, and followed the connection between the chemical elements in the sources and the high loaded element in the factor. Therefore, the association of the first factor with soil dust is straightforward, in the same way as the fifth is related to the sea spray. The second factor was associated to vehicles emission mainly due to the presence of Pb (and also BC); in Brazil leaded gasoline has been banned for a long time, but in this case Pb is a geological residue in the petroleum or contamination in the production process (Gioia et al., Citation2010). K in the third factor indicates the association with biomass burning. We are not secure about the four and six factors’ association, but they could be attributed specially to industrial activity. An integrated source discussion will be done after the CMB results.
Table 3. Main rotated factors loadings, with the six factors explaining 78.5% of the total database variance
Table 4. Absolute factors (fraction of the total mass)
Equation 6 may be solved using several source arrangements to obtain a local source apportionment. However, a mathematically adequate blend of sources is not necessarily compatible with the local observed reality. PMF and PCA may show what should be expected, and PCA does that by grouping the correlated elements. But the selections provided by PCA and PMF are sensitive, among others, to the defined number of factors, or to the elements turned on or off, and, in the case of PMF, to the uncertainty set up. An integrated use of these models, with their peculiarities, besides the knowledge of local environmental characteristics (sources, wind transport and other meteorological factors, for instance), could provide a more balanced evaluation of the local sources and respective impacts.
groups the source apportionment results for PMF and PCA/CMB in the average concentrations (general, winter, and summer). We did not have local sources profiles to be used in CMB. We tried to use vehicular profiles recently obtained inside tunnels at, or around, São Paulo city in Brazil (not published yet) and U.S. EPA profiles organized by SPECIATE (U.S. EPA, Citation2011): sea spray, biomass burning, steel mill, and cement plant (there is one factory 22 km NNE of the sampling point, and another 37 km SSW). From the several combinations possible, the best results come when considering only the five sources obtained by absolute PCA (), and only the sea spray component was not extracted because of its anticorrelation with total mass concentration.
Table 5. PCA/CMB and PMF estimative of the contribution of factors/sources for average concentrations, for all samples, winter period samples, and summer period samples
The sources profiles extracted by receptor models express the transformations of the aerosol in its trajectory from the emission until the receptor. But they also show some sources blending, depending on wind transport and the sampling station positioning, and yet, long sampling period, like a 24-hr cycle, averages sources specificities that otherwise could help sources’ discrimination. It is not surprising, therefore, that PMF mixed vehicles and sea spray, since the most frequent wind, from the east-southeast, brings together the urban and the sea particles. The model attributed 40 to 54% of the sampled mass to that source, being the summer value higher than winter one. This result is consistent with the easterly deviation of the wind, transporting more of the harbor and vehicular emissions from downtown and from a highway placed beside the UFPE campus. These values seem to be more adequate than the CMB/PCA (9 to 20%), considering the dimension of the local vehicular fleet (400,000 units, being 17,000 heavy duty vehicles). The higher value obtained by PMF for this source probably is not due to the adjunct sea spray, present prevalently in the coarse PM. Both methods identified a source highly loaded with Cl, probably because it comes from more than one source. There are industries of plastics, sea spray, and biomass burning, for instance, and the sugar and alcohol production. This factor is lower in the summer, and one possible explanation is connected to Cl depletion in salt-containing particles. The intensification of solar radiation during the summer can increase the production of sulfuric and nitric acid due to photochemical reactions. Both acids could react with Cl from NaCl-containing particles, forming gaseous HCl and reducing the Cl concentration in the PM2.5 (McInnes, Citation1994). The biomass burning source, characterized by K and Br (and some correlation with BC), could have expressive participation of the sugarcane thatch burning, before harvesting, and wood burning for cooking. This factor was well defined and apportioned an expressive mass amount in both models, although for summer the PMF estimated much less mass than PCA/CMB. The source soil dust also was well defined in both models, and in this case no severe discrepancy was observed in the seasonal values. The increase of soil dust fraction during summer is coherent with the rain reduction and higher wind speed, facilitating dust resuspension in this period. The Ni source discriminated by PCA/CMB may be connected to metallurgical activities in the area ().
Taking the 20 samples with higher concentrations, only 4 days were in the dry period (summer), and for 3 of them the dominant source was soil dust, being related to reported intense NW to N winds (a rare wind direction in the region). In the 16 other days, in the wintertime, soil dust had a reduced participation and the impact was fractionated among the other sources, and in the more intense concentrations the prevalent wind came from the south.
It is important to point out some differences between the receptor modeling results shown here and those obtained in a previous work dealing with results of the six Brazilian capitals studied in the main project INAIRA (Andrade et al., Citation2012). That study considered a global view of the six capitals, and approximately half of the database had been submitted to XRF analysis (148 samples in the case of Recife). The present work went deeply into the study of Recife, analyzing all samples with an increased LD for the elements with Z < 14 (reanalyzing the first 148 samples to avoid any analytical disruption), using an intercalibration for BC, and articulated absolute PCA/CMB with PMF to improve the sources identification analysis, considering, otherwise, more extensively the meteorological parameters. The first work identified that the average PM2.5 source apportionment gave 8% for light vehicles, 8% for crustal, 29% for heavy-duty vehicles, and 24% for sea spray. We could observe that crustal was very similar, and if we sum all contribution from vehicles, this result do not diverge substantially from that obtained by PMF analysis performed here. The use of the double number of samples and better LD for elements with low Z provided the inclusion of Na, Mg, and Al, not used before. Therefore, in the present work it was possible to increase the number of factors and to distinguish, also, biomass burning and industrial contributions, although the sea spray could not be separated from vehicles.
Conclusion
The use of PCA/CMB and PMF receptor models provided a study of the impact of the principal sources acting in the area of Recife, considering the seasonal peculiarities. The models showed some difference in the results, but for soil dust and biomass burning they were similar (a participation between 5 to 17% and between 18 to 47%, respectively). PCA/CMB estimated a participation of 9 to 20% of vehicles to the PM2.5, less realistic than PMF (40 to 54%), although it mixed sea spray in this factor, while PCA/CMB did not. Both models showed a factor highly identified with chlorine, which could come from more than one source, and other factors with different structures that could be related to metallurgical activities.
The PM2.5 concentration levels in the sampling site, during the period of this experiment, remained within the WHO guidelines, notwithstanding the high state of urbanization of the metropolitan area. Probably this could be related to the general wind circulation in the local, associated with the regional shoreline meteorological system, given favorable conditions for air pollution dispersion. The rainy period (winter) showed higher concentration levels, probably due to the worsening of the atmospheric conditions for dispersion. Those results represent a valuable register of the PM2.5 in the region of Recife, as well as its dynamics associated with local climate, and should be very important for the next analysis, which will correlate health data, PM2.5 levels, and sources responsibility, in the context of INAIRA, the comprehensive project where six Brazilian Capitals are being studied.
Acknowledgment
The authors are grateful to the following research institutions for the sampling infrastructure and technical support: the Department of Anatomy, Center for Biological Sciences, Universidade Federal de Pernambuco (UFPE, Federal University of Pernambuco) and Instituto Nacional de Meteorologia (INMET, National Meteorology Institute).-
Funding
This work was supported by the Hewlett Foundation, CNPq (Conselho Nacional de Pesquisa), and FAPESP (Fundação de Amparo à Pesquisa do Estado de São Paulo).
Additional information
Notes on contributors
Luis H.M. dos Santos
Luís H.M. dos Santos is an undergraduate student and Thiago G. Veríssimo is a graduate student of the Institute of Physics, University of São Paulo, owning a bursary to perform air pollution studies.
Américo A.F.S. Kerr
Américo A.F.S. Kerr is a professor ofPhysics of Air Pollution at the Department of Applied Physics, Institute of Physics, University of São Paulo.
Thiago G. Veríssimo
Luís H.M. dos Santos is an undergraduate student and Thiago G. Veríssimo is a graduate student of the Institute of Physics, University of São Paulo, owning a bursary to perform air pollution studies.
Maria de Fatima Andrade
Maria de Fatima Andrade is a professor of Air Pollution Meteorology and Adalgiza Fornaro is professor of Atmospheric Chemistry at the Department of Atmospheric Sciences, Institute of Astronomy, Geophysics and Atmospheric Sciences, University of São Paulo, coordinator of the Laboratory of Atmospheric Process (LAPAt).
Regina Maura de Miranda
Regina Maura de Miranda is a professor at the School of Arts, Sciences and Humanities, University of São Paulo.
Adalgiza Fornaro
Maria de Fatima Andrade is a professor of Air Pollution Meteorology and Adalgiza Fornaro is professor of Atmospheric Chemistry at the Department of Atmospheric Sciences, Institute of Astronomy, Geophysics and Atmospheric Sciences, University of São Paulo, coordinator of the Laboratory of Atmospheric Process (LAPAt).
Paulo Saldiva
Paulo Saldiva is full professor of the Department of Pathology, Faculty of Medicine, University of São Paulo, coordinator of the Laboratory of Experimental Atmospheric Pollution (LAPAE). All authors, except Dr. Paulo Saldiva, are members of the LAPAt.
References
- Anderson, J.O., J.G. Thundiyil, and A. Stolbach. 2011. Clearing the air: A review of the effects of particulate matter air pollution on human health. J. Med. Toxicol. 8: 166–75. doi:10.1007/s13181-011-0203-1
- Andrade, M. de F., R.M. de Miranda, A. Fornaro, A. Kerr, B. Oyama, P.A. de Andre, and P. Saldiva. 2012. Vehicle emissions and PM2.5 mass concentrations in six Brazilian cities. Air Qual. Atmos. Health. doi:10.1007/s11869-010-0104-5
- Baumgardner, D., O. Popovicheva, J. Allan, V. Bernardoni, J. Cao, F. Cavalli, J. Cozic, E. Diapouli, K. Eleftheriadis, P.J. Genberg, C. Gonzalez, M. Gysel, A. John, T.W. Kirchstetter, T.A.J. Kuhlbusch, M. Laborde, D. Lack, T. Müller, R. Niessner, A. Petzold, A. Piazzalunga, J.P. Putaud, J. Schwarz, P. Sheridan, R. Subramanian, E. Swietlicki, G. Valli, R. Vecchi, and M. Viana. 2012. Soot reference materials for instrument calibration and intercomparisons: A workshop summary with recommendations. Atmos. Measure. Techniques Discuss. 5: 2315–62. doi:10.5194/amtd-5-2315-2012
- Dionisio, K., R. Arku, A. Hughes, J. Vallarino, H. Carmichael, J. Spengler, S. Agyei-Mensah, and M. Ezzati. 2010. Air pollution in Accra neighborhoods: Spatial, socioeconomic, and temporal patterns. Environ. Sci. Technol. 44: 2270–76. doi:10.1021/es903276s
- Coulter, C.T 2004. EPA-CMB8.2 Users Manual. EPA-452/R-04-011. U.S. Environmental Protection Agency. http://www.epa.gov/ttn/scram/models/receptor/EPA-CMB82Manual.pdf (accessed November 20, 2012).
- Florêncio, L., M. do C. Tavares, E.S. de Lima, M.T. Kato, R.A. Silva, M.B. Barbosa, and L.S. do Espírito Santo. 1997. Caracterização preliminar da concentração de metais pesados nas partículas totais em suspensão na região metropolitana do Recife. Paper presented to the 19th Brazilian Congress of Sanitary and Environmental Engineering, Foz do Iguaçu, Paraná, Brazil, September, 14–19.
- Gatti, M., and A.A.F.S. Kerr. 2008. Avaliação da influência de cargas eletrostáticas e humidade na gravimetria de filtros de policarbonato. Paper presented at the XV CBMET Brazilian Congress of Meteorology, São Paulo, SP, Brazil, August 24–29.
- Gioia, S.M.C.L., M. Babinski, D.J. Weiss, and A.A.F.S. Kerr. 2010. Insights into the dynamics and sources of atmospheric lead and particulate matter in São Paulo, Brazil, from high temporal resolution sampling. Atmos. Res. 98: 478–85. doi:10.1016/j.atmosres.2010.08.016
- INMET. 2007. Instituto Nacional de Meteorologia, Brazil. http://www.inmet.gov.br/portal
- Johnson, R.A., and D.W. Winchern. 1982. Applied Multivariate Statistical Analysis. Englewood Cliffs, NJ: Prentice Hall.
- Keiding, K., F.P. Jensen, and N.Z. Heidam. 1986. Absolute modelling of urban aerosol elemental composition by factor analysis. Anal. Chim. Acta 181: 79–85. doi:10.1016/S0003-2670(00)85222-8
- Marple, V.A., and K. Willeke. 1976. Impactors design. Atmos. Environ. 10: 891–96.
- Marple, V.A., K.L. Rubow, W. Turner, and J.D. Spengler. 1987. Low flow rate sharp cut impactors for indoor air sampling: Design and calibration. J. Air Pollut. Control Assoc. 37: 1303–7.
- McInnes, L.M., D.S. Covert, P.K. Quinn, and M.S. Germani. 1994. Measurements of chloride depletion and sulfur enrichment in individual sea-salt particles collected from the remote marine boundary layer. J. Geophys. Res. 99: 8257–68. doi:10.1029/93JD03453
- Miranda, R.M., M. de F. Andrade, A. Fornaro., R. Astolfo, P.A. de Andre, and P. Saldiva. 2010. Urban air pollution: A representative survey of PM2.5 mass concentrations in six Brazilian cities. Air Qual. Atmos. Health. doi:10.1007/s11869-010-0124-1
- Norris, G., R. Vedantham, K. Wade, S. Brown, J. Prouty, and C. Foley. 2008. EPA Positive Matrix Factorization (PMF) 3.0, Fundamentals & User Guide. Washington, DC: U.S. Environmental Protection Agency, Office of Research and Development.
- Paatero, P. 1997. Least squares formulation of robust non-negative factor analysis. Chemometrics Intelligent Lab. Systems 37: 23–35.
- Picolo, M.F., R.M. de Miranda, M. de F. Andrade, and A. Fornaro. 2010. Variabilidade da concentração em massa e Black Carbon de MP2,5 da cidade de Recife, efeitos da pluviometria (remoção). Paper presented at the Brazilian Congress of Meteorology, Belém, Pará, Brazil, September 13–17.
- Polissar, A.V., P.K. Hopke, P. Paatero, W.C. Malm, and J.F. Sisler. 1998. Atmospheric aerosol over Alaska 2. Elemental composition and sources. J. Geophys Res (Atmos.) 103 (15):19045–57.
- Pope, A.C. III, and D.W. Dockery. 2006. Health effects of fine particulate air pollution: Lines that connect. J. Air Waste Manage. Assoc. 56 (6):709–42. doi:10.1080/10473289.2006.10464485
- Pope, A.C. III, M. Ezzati, and D.W. Dockery. 2009. Fine-particulate air pollution and life expectancy in the United States. N. Engl. J. Med. 360: 376–86. doi:10.1056/NEJMsa0805646
- Quincey, P., D. Butterfield, D. Green, and G.W. Fuller. 2011. Black smoke and black carbon: Further investigation of the relationship between these ambient air metrics. Atmos. Environ. 45: 3528–34. doi:10.1016/j.atmosenv.2011.04.009
- REDEMET. 2012. Rede de Meteorologia do comando da Aeronáutica, Brazil, 2012. http://www.redemet.aer.mil.br
- Santos,Thiago, O., C.A. Silva Filho, M. Saiki, F.A. Genezini, E.J. França, C.A. Hazin, and E. Valentim. 2011. Chemical element composition of atmospheric bromeliads for active biomonitoring of the sugarcane cultivation impacts. Paper presented at the International Nuclear Atlantic Conference, Belo Horizonte, Minas Gerais, Brazil, October, 24–28.
- U.S. Environmental Protection Agency. 2011. Speciation database development documentation. http://cfpub.epa.gov/si/speciate (accessed November 20, 2012).
- U.S. Environmental Protection Agency. 2012. Standard operating procedure (SOP) for the analysis of organic and elemental carbon (OC/EC) using the Sunset Laboratory semi-continuous carbon aerosol analyzer. Office of Air Quality Planning and Standards. http://www.epa.gov/ttnamti1/files/ambient/pm25/spec/Sunset_SOP_draft030212.pdf
- Van Grieken, R., L.V. Dack, C.C. Dantas, W.M. de Amorim, and W. Maenhaut. 1982. Elemental constituents of atmospheric aerosols in Recife, north-east Brazil. Environ. Pollut. (Ser. B) 4: 143–63. doi:10.1016/0143-148X(82)90025-8
- World Health Organization. 2005. Air quality guidelines for particulate matter, ozone, nitrogen dioxide and sulfur dioxide. http://www.who.int/phe/health_topics/outdoorair/outdoorair_aqg/en/index.html (accessed August 15, 2011).