Abstract
Representative profiles for particulate matter particles less than or equal to 2.5 µm (PM2.5) are developed from the Kansas City Light-Duty Vehicle Emissions Study for use in the U.S. Environmental Protection Agency (EPA) vehicle emission model, the Motor Vehicle Emission Simulator (MOVES), and for inclusion in the EPA SPECIATE database for speciation profiles. The profiles are compatible with the inputs of current photochemical air quality models, including the Community Multiscale Air Quality Aerosol Module Version 6 (AE6). The composition of light-duty gasoline PM2.5 emissions differs significantly between cold start and hot stabilized running emissions, and between older and newer vehicles, reflecting both impacts of aging/deterioration and changes in vehicle technology. Fleet-average PM2.5 profiles are estimated for cold start and hot stabilized running emission processes. Fleet-average profiles are calculated to include emissions from deteriorated high-emitting vehicles that are expected to continue to contribute disproportionately to the fleet-wide PM2.5 emissions into the future. The profiles are calculated using a weighted average of the PM2.5 composition according to the contribution of PM2.5 emissions from each class of vehicles in the on-road gasoline fleet in the Kansas City Metropolitan Statistical Area. The paper introduces methods to exclude insignificant measurements, correct for organic carbon positive artifact, and control for contamination from the testing infrastructure in developing speciation profiles. The uncertainty of the PM2.5 species fraction in each profile is quantified using sampling survey analysis methods. The primary use of the profiles is to develop PM2.5 emissions inventories for the United States, but the profiles may also be used in source apportionment, atmospheric modeling, and exposure assessment, and as a basis for light-duty gasoline emission profiles for countries with limited data.
Implications:
PM2.5 speciation profiles were developed from a large sample of light-duty gasoline vehicles tested in the Kansas City area. Separate PM2.5 profiles represent cold start and hot stabilized running emission processes to distinguish important differences in chemical composition. Statistical analysis was used to construct profiles that represent PM2.5 emissions from the U.S. vehicle fleet based on vehicles tested from the 2005 calendar year Kansas City metropolitan area. The profiles have been incorporated into the EPA MOVES emissions model, as well as the EPA SPECIATE database, to improve emission inventories and provide the PM2.5 chemical characterization needed by CMAQv5.0 for atmospheric chemistry modeling.
Background
Composition of PM2.5 from light-duty gasoline vehicle exhaust
Particulate matter (PM) exhaust from light-duty gasoline vehicles (LDGVs) is a complex mixture composed of elemental carbon (EC), organic carbon (OC), and inorganic elements and ions. Exhaust measurements of particulate mass with aerodynamic diameter less than 2.5 μm (PM2.5) capture the vast majority of particulate mass emitted from light-duty gasoline vehicles (CitationRobert et al., 2007).
Thermal-optical measurements quantify elemental carbon (EC) that forms from fuel pyrolysis during combustion. Quantifying the EC component of gasoline particulate matter is important for understanding the health risks and the climate-forcing potential of LDGV emissions (EPA, 2012). Additionally, the EC fraction is an important source indicator in source-apportionment studies (CitationLough et al., 2007; CitationFujita et al., 2007). Differences in the elemental carbon measurement can have a large impact on the apportionment of emissions between diesel and gasoline engines (CitationFujita et al., 2007).
In motor vehicle exhaust, the organic carbon (OC) refers to carbon bound with other noncarbon elements in compounds that form liquid and solid aerosols. For our purposes, organic carbon particulate is operationally defined from thermal-optical methods. We refer to organic matter (OM) as the mass of OC and the mass of the associated elements bound to the organic carbon (oxygen, hydrogen, nitrogen, and other noncarbon elements). For light-duty vehicle gasoline exhaust, previous researchers have adjusted OC emission measurements to obtain OM measurements using the following factors: 1.2 (CitationZielinska et al., 2004; CitationSchauer et al., 2008), 1.25 (CitationReff et al., 2009), 1.4 (CitationLough et al., 2005; CitationRobert et al., 2007), and 1.55 (CitationCheung et al., 2009). Primary OM emissions occur directly from the tail pipe, and include important air toxics such as polycyclic aromatic hydrocarbons (PAHs). Secondary organic aerosol forms from volatile compounds reacting and condensing in the atmosphere (EPA, 2012). Historically, organic matter has been considered the dominant component of primary PM emissions from light-duty gasoline vehicles (CitationZielinska et al., 2004; CitationFujita et al., 2007; CitationLough et al., 2007).
Particulate matter from LDGVs also includes inorganic components, including metals and other elements that contribute significantly to both the mass and the toxicity of PM (CitationCheung et al., 2010). Light-duty gasoline tail-pipe exhaust is a significant contributor of magnesium, calcium, lead, zinc, molybdenum, and platinum in the roadside environment (CitationSchauer et al., 2006). Similarly, CitationCho et al. (2009) and CitationHays et al. (2011) reported that near-road PM2.5 samples are enriched in metals associated with vehicle emissions, including copper and zinc. Identifying the contribution of each species to LDGV particulate matter is important for understanding the toxicity of the emissions, as well as for estimating the contribution of LDGV exhaust to ambient particulate matter pollution.
Modeling requirements
The Motor Vehicle Emission Simulator (MOVES) is the vehicle emission model developed by the U.S. Environmental Protection Agency (EPA) Office of Transportation and Air Quality to estimate emission rates and emission inventories from on-highway vehicles. MOVES has a range of policy uses, including developing mobile source emission inventories for state implementation plans as required by the Clean Air Act, and developing project-level emission inventories from proposed transportation projects. MOVES is used in conjunction with SMOKE (Sparse Matrix Operator Kernel Emissions) to provide model-ready emission inventories to be used in the Community Multiscale Air Quality System (CMAQ) photochemical air quality model. The EPA uses the MOVES-SMOKE-CMAQ modeling system to evaluate regional and nationwide air quality impacts.
Previous versions of MOVES (2010b, and earlier) estimated PM2.5 emission inventories, but the model outputs were not aligned with the PM2.5 model species used by CMAQ. Significant postprocessing of MOVES PM2.5 outputs was needed to provide PM2.5 emissions inventories that could be transformed by SMOKE into model-ready inputs of speciated PM2.5 for CMAQ. The upcoming version of MOVES is designed to produce all PM2.5 species required by CMAQv5.0, which uses the CMAQ Aerosol Module, version 6, or “AE6.” AE6 includes 18 PM2.5 species as outlined in (CitationSimon, 2012). These additional PM2.5 species produced by MOVES are beneficial to air quality agencies and researchers who use different air quality models, as they are compatible with previous versions of CMAQ and with the Comprehensive Air Quality Model with Extensions (CAMx, 2011) as shown in . The PM2.5 profiles used by MOVES and CMAQ are stored in the EPA SPECIATE database, which is a publicly available repository for PM and VOC speciation profiles (CitationHsu et al., 2006).
Table 1. PM2.5 species included in CMAQv5.0 (Aerosol Module 6), CMAQv4.7.1 (Aerosol Module 5), and CAMx5.4
Data: Kansas City Light-Duty Vehicle Emission Study
The Kansas City Light-Duty Vehicle Emission Study (KCVES) was conducted to improve PM emission inventories from light-duty gasoline vehicles (CitationFulper et al., 2010; EPA, 2008). The KCVES provided information on ambient temperature effects on emissions (CitationNam et al., 2010) and PM emission rates of smoking vehicles (CitationNam et al., 2008), and it is the major source of light-duty gasoline PM rates in the current MOVES model (EPA, 2011). Several follow-up analyses on the KCVES were conducted to evaluate the effect of sampling temperature (CitationFujita et al., 2006b) and operating mode on organic speciation (CitationHerrington et al., 2012), chemical analysis of the lubrication oil (CitationFujita et al., 2006a), and the contribution of lubricating oil to PM emissions (CitationSonntag et al., 2012).
The KCVES collected PM2.5 measurements from a statistically representative sample of LDGVs in the Kansas City Metropolitan Statistical Area (KCMSA). The study was conducted in the summer of 2004 (Phase 1) and winter of 2004/2005 (Phase 2). A stratified sampling design was implemented to test a greater percentage of older, high-emitting vehicles compared to the amount of newer, low-emitting vehicles in the U.S. fleet. The LDGVs were grouped into eight strata classified by two vehicle types and four model year groups, as shown in . The percentages of LDGVs in each strata in the KCMSA are provided in , as well as the calculated contribution to annual vehicle miles traveled (VMT). Four hundred and ninety-two vehicles were tested across two rounds of the study (42 vehicles were tested in both the summer and winter round). More than 555 vehicle tests were conducted when counting repeat vehicle tests within and across seasons. A subset of 98 vehicles was selected for detailed chemical characterization and is referred as the speciation subset in . All vehicles were tested on a three-bag LA-92 driving cycle, also known as the California Unified Cycle. The LA-92 included a cold start (Phase 1), hot stabilized running (Phase 2), and a hot start (Phase 3), covering 9.8 miles representing arterial and freeway driving in Los Angeles, CA. The vehicles were purposely tested at ambient temperatures in a garage with open bay doors. The temperature for the testing facility ranged from 58°F to 96°F in the summer round (average of 76°F) and from 12°F to 74°F (average of 43°F) in the winter round (CitationFulper et al., 2010).
Table 2. Vehicle sample size in the Kansas City Light Duty Vehicle Emissions Study
The emissions measurements made on the KCVES vehicles are grouped into three levels of detail as outlined in . PM2.5 measurement data collected at all three levels are used in the profile developed in this paper. At Level 1, each of the 496 vehicles was tested for criteria pollutant emissions—hydrocarbons (HC), carbon monoxide (CO), carbon dioxide (CO2), oxides of nitrogen (NOx)—and both filter-based PM and indirect measurements of PM. Particulate matter emissions from each phase of the LA-92 were collected on 47-mm Teflo Teflon membrane filters from exhaust extracted from the dilution tunnel and through a PM2.5 cyclone. Included among the indirect PM instruments is a photoacoustic sampler (DRI, Reno, NV) which measured light-absorbing carbon reported as black carbon (BC), providing a comparable measure to elemental carbon for each vehicle test in the full sample. Details on the DRI photoacoustic sampler are provided in Table S1 in the supporting information.
Table 3. Levels of PM characterization conducted on vehicles tested in Kansas City
As summarized in , at level 2 the 98 vehicles selected for chemical analysis received additional phase-specific measurements of elemental carbon, organic carbon, and metals. One vehicle (1979 Buick LeSabre) was tested in both the summer and winter, yielding 49 and 50 vehicles tested in summer and winter, respectively. EC and OC were measured on quartz-fiber filters for each phase of the LA-92 (Phase 1, Phase 2, and Phase 3, corresponding to Bag 1, Bag 2, and Bag 3), using the thermal optical reflectance (TOR) method with the IMPROVE (Interagency Monitoring of Protected Visual Environments) procedures (IMPROVE-TOR) (CitationChow et al., 1993). Forty elements were analyzed using x-ray fluorescence (XRF) on the Teflon filters measured on each phase of LA-92.
At Level 3, the vehicle tests measured in Level 2 were composited for measurements of organic compounds and select elements and ions. The composite measurements were not sampled on the same media, but were extracted from filters measured in each bag of the LA-92. Seven elements (Pb, Hg, As, Cr, Cu, Zn, and Mn) were measured by inductively coupled plasma–mass spectrometry (ICP-MS) from the composited Teflon-filter extraction. The elements measured by ICP-MS were previously measured by XRF (Level 2), but ICP-MS has lower detection limits than XRF. Sulfate and nitrate ions were measured with ion chromatography (IC) from the composited extracts from each half of the quartz-fiber filters sampled. Quantification of ammonium and other cations was not budgeted in the study.
In the case of the 1990 and newer model year vehicles, sample was composited from several vehicles (one to five) before chemical analysis. The composting across vehicle tests reduced the 102 individual vehicle tests selected in Level 2 to 52 composite chemical samples. The compositing occurred across several vehicle tests for the newer vehicles in order to assure enough particulate to conduct organic speciation by gas chromatography and mass spectrometry (GC/MS). The semivolatile organic compounds (SVOC) were sampled using a separate sampling train collected with Teflon-impregnated glass fiber (TIGF) filters and XAD cartridge resin, results from which are reported in EPA (2008).
Comparable composite emission rates for PM2.5 gravimetric mass, EC/OC, and XRF elements was calculated from the individual phase-specific and vehicle-specific measurements, with propagation of uncertainty as described in EPA (2008). Additional details of the vehicle emission testing, sample design, instrument setup, and testing protocol are described in CitationFulper et al. (2010) and EPA (2008).
Profile Development
Data preparation
To develop PM2.5 speciation profiles from the KCVES, the total PM2.5 mass must be reconstructed from the individual particulate species. Several quality control steps are conducted to assure the validity of the individual species measurements: (1) identification of measurements above the detection limit and uncertainty of the analytical measurement; (2) evaluation of the data for inconsistencies or measurement problems; and (3) comparison of the mass balance of individual species to the gravimetric filter mass.
Identification of Significant Measurements
The significance of each PM2.5 species is evaluated using the composite emission rates for each compound measured in the KCVES (EPA, 2008). The field-blank corrected measurements and their associated measurement uncertainties were calculated in the KCVES report (EPA, 2008) for each composite sample using the minimum detection limit, the precision of the analyte measurement, the concentration of the analyte measurement, and the standard deviation of field-blank measurements. All field blanks were used except the outliers identified from comparisons to the dilution tunnel and exhaust measurements. The uncertainty of the ICP-MS and IC measurements is the uncertainty of the single field-blank corrected composite measurements. For the IMPROVE-TOR and XRF measurements, the uncertainty measurements of the composite sample is calculated by propagating the uncertainty from the individual filter measurements from each phase of the LA-92 drive cycle and from each vehicle test. The steps and equations used to calculate the composite measurement uncertainties are provided in the KCVES report (EPA, 2008).
In this work, we conducted a statistical test to determine if the measured compounds were significantly above the dilution tunnel blank measurements. First, we calculated the dilution-tunnel corrected composite values and propagated the uncertainty of the composite measurements with the standard deviation of the dilution-tunnel blank measurements taken in each season. Six composite dilution tunnel blanks were calculated from each round of the study from dilution tunnel blank tests made almost on a day-by-day basis (EPA 2008). Second, we counted the occurances of measurements made above the dilution-tunnel corrected uncertainty for the EC, OC, SO4, NO3, 49 XRF, and 7 ICP-MS elements measured in all 52 composite samples. Third, we calculated the probability of observing the counted occurences due to the variability of the measurement alone using the binomial distribution. The binomial distribution is used because the measurement uncertainty calculated in the KCVES report (EPA, 2008) varies with each composite sample result. Results are summarized in for each compound measured in the composite sample with the corresponding number of measurements that were made above the dilution-tunnel corrected uncertainties, and the probability of observing that number of occurances due to the measurement uncertainty alone (referred to as type I error).
Table 4. Significance test results of species measurements from the KCVES program
For example, titanium was measured above the dilution-tunnel corrected measurement uncertainties on 12 of the 52 composite samples. Assuming the measurement uncertainty is normally distributed, the probability of observing 12 or more measurements above the uncertainty is equal to 0.06. Titanium (Ti) and manganese (Mn) could be considered significant depending on the acceptable type I error and which correction is used (dilution-tunnel corrected, or field blank only). Magnesium (Mg), zirconium (Zr), and mercury-ICP (Hg-ICP) each had only nine composite measurements above the uncertainty of the dilution-tunnel corrected values. These and the elements with fewer measurements above the uncertainty are considered insignificant using reasonable type I error values. identifies the species according to significant and insignificant based on a type I error of 0.10. Only the elements that are deemed significant (titanium and above) are included in the PM2.5 speciation profile. Additional details on dilution tunnel-correction and the statistical test are included in the supporting information.
Inorganic species
The PM2.5 profile is not intended to be an exhaustive list of elements and ions that are emitted in the particle phase, but is intended to capture the species that contribute significantly to the total PM2.5 mass and/or are required by CMAQv5.0. As stated, 10 of the 12 additional PM2.5 species required by CMAQv5.0 are elements. Elements and ions are included in the PM2.5 profile from the KCVES if they are valid measurements. Other sources are used to impute element and ion measurements if no valid measurements from KCVES are available and they are required by CMAQv5.0 AE6 and/or for achieving mass balance.
Several elements known to be emitted in light-duty gasoline PM2.5 are not included in the profile, including trace elements in the catalytic converter washcoat (cesium, rhodium, palladium, platinum, lanthanium), which deteriorate and are emitted in light-duty exhaust (CitationVan Der Schoot et al., 2001). These elements were either not measured or were not detected at significant levels in the KCVES. The excluded elements are emitted in such small concentrations that they do not impact the mass balance of filter mass, nor are they required by CMAQv5.0 AE6. The arsenic measurements are also excluded from the species profile. Although the arsenic ICP-MS measurements were deemed significant, the measurements exhibited strong inconsistencies across the study. The differences in the XRF and ICP-MS measurements for arsenic were an order of magnitude, and more than half of the arsenic ICP-MS values in the winter round were negative. Arsenic is not required by CMAQv5.0 AE6 and values are not imputed from other studies.
In most cases the elements that were measured by both XRF and ICP-MS were within the statistical variability of each other. Because XRF measurements were conducted at the bag level, the XRF measurements are used in the reconstructed PM2.5 profile for chromium, zinc, lead, and copper. In the case of manganese, the ICP-MS and XRF measurements were highly correlated, but a significant difference is detected. The bag-level XRF measurements of Mn were thus scaled to the ICP-MS measurements for use in the PM2.5 profile. Sodium (Na) and magnesium (Mg) measured by XRF are deemed qualitative measurements because they emit weak x-rays due to their small size (David Campbell, personal correspondence). Additionally, the XRF measurements of magnesium were not significantly above the uncertainty of the dilution-tunnel measurements. No measurements of sodium or magnesium were made by the ICP-MS in the KCVES. To provide quantitative emission rates for sodium and magnesium, values are imputed from measurements made using ICP-MS from 53 light-duty gasoline vehicles in the South Coast Air Quality Management District in California (CitationSchauer et al., 2006; CitationLough et al., 2007). The major source of sodium and magnesium is believed to be the engine oil since both of these were measured at significant levels in the used oil from a subsample of vehicles tested in the KCVES. As such, the emissions of sodium and magnesium are imputed using the ratio of sodium and magnesium to zinc (1:15 and 1:4.1, respectively). Zinc is selected because it is also a component of lubricating oil, and because the ratio of Zn to PM2.5 is roughly equal between the summer testing of KCVES and the profile used in the CitationSchauer et al. (2006) study.
Silicon was the most abundant element measured in KCVES (EPA, 2008). The catalytic converter washcoats, substrates, and mats are composed of materials containing silicon (including silica, alumina silicate, vermiculite, and cordierite) (CitationHeck et al., 2002). Erosion of these materials in the exhaust system (CitationWatson and Dietz, 2009) could lead to comparable emissions of both silicon and aluminum in the exhaust (CitationSchauer et al., 2002). However, in KCVES, the silicon emissions were much higher than the aluminum emission rates. CitationSchauer et al. (2002) reported silicon emission rates from nine catalyst vehicles ranging from 1981 to 1994 model years that were one order magnitude smaller than in KCVES. No silicon was detected in the used lubricating oil in the KCVES (CitationFujita et al., 2006a), indicating that the large silicon emission rates were not coming from the engine or the emission control system.
Upon further review, we concluded that silicone rubber in the KCVES sampling system contaminated the particulate measurements with silicon and other organic material. In the KCVES sampling system, silicone rubber boots were used as a coupler between the exhaust tail pipe of the recruited vehicles and a stainless steel exhaust transfer line. In a number of the KCVES tests, several inches of the silicon rubber boots were directly exposed to the hot exhaust. Silicone polymer is known to volatize and breakdown when exposed to high temperatures and can be oxidized from lubricating oil (CitationKeller, 1986). CitationCadle et al. (1999) suspected that silicone couplers may have contributed to particulate measurement artifacts in a past LDGV emission study, and CitationMaricq et al. (1999) confirmed the silicone couplers can contribute to high concentrations of ultrafine particles measured under high exhaust temperatures.
The silicone contribution to the measured PM2.5 mass is estimated using the elemental silicon measurements. The silicone polymer contains silicon, oxygen, carbon, and hydrogen, which contribute to the measured particulate and organic carbon mass; however, the chemical structure of the volatized silicone material measured on the measurement filters is unknown. To estimate the contribution of volatized silicone relative to the elemental silicon measurements, we used a regression model developed in an earlier analysis (CitationSonntag et al., 2012) to estimate the relative contribution of silicon to the measured PM2.5 emissions. The coefficient of silicon was statistically significant for the linear regression of pre-1990 vehicles, with a mean value of 4.075 with 95% confidence intervals of (2.47 to 5.67). Thus, the actual contribution of the volatilized silicone to the particulate matter measurements is estimated to be 4.075 times the measured elemental silicon. The silicon coefficient for predicting PM2.5 for newer vehicles (1990–2004) is 3.84 (Table S4), which is not statistically significant but is quite similar to the coefficient derived for the pre-1990 vehicles. Using the 4.075 silicone mass ratio and the measured elemental silicon from the speciated subset vehicles in KCVES, the volatized silicone contribution to measured PM2.5 is estimated for each sample, as shown later in and .
Figure 1. Comparison of mass-balanced corrected organic matter emission rates with measured organic carbon emission rates.
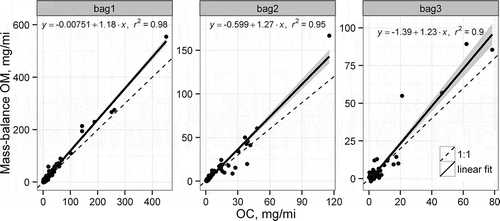
Elemental silicon values are model species in CMAQv5.0 AE6. Because the measured elemental silicon is contaminated by the silicone rubber housing, the silicon emission rates for the profile are substituted with values equal to the KCVES aluminum emission rates for both the cold start and Bag 2 emissions. Aluminium is used due to its similar sources in the emission control system and similar measured emission rates reported from previous LDGV studies not using silicon couplers in the sampling system (CitationSchauer et al., 2002)
The sulfate ion (SO4) and elemental sulfur (S) emission rates for the composite samples are compared in Figure S5 as well as in EPA (2008). The two measurements are highly correlated (r2 = 0.97), and the IC measured sulfate accounts for 81% of the elemental sulfur mass measured by XRF. Lack of mass balance could be due to both (1) measurement uncertainties, including different capture efficiencies of sulfur using the different media (Teflon and quartz) and differences in the XRF and IC measurement methods, and/or (2) particulate sulfur existing in different molecular states such as zinc dithiophosphate (a lubricant additive). Trace amounts of sulfur-containing PAHs were measured in the study (benzonaphthothiophene and dibenzothiophene), which emitted typically less than 0.5% of the sulfur measured by XRF. To estimate the amount of sulfate at the vehicle and bag level, the composite SO4 measurements are apportioned using the XRF S measurements and the SO4 to S ratio from each composite sample. No estimate of nonsulfate particulate sulfur is included in the reconstructed mass.
Nitrate is measured at the composite level, and is apportioned for individual vehicles and each bag of the LA-92 using the nitrate/PM2.5 ratio from each composite test and the PM2.5 measurements from each vehicle and bag. Ammonium concentrations were estimated in the absence of measurement data. CitationChueng et al. (2009) estimated ammonium emissions on gasoline vehicles 12–20 times higher than from diesel vehicles. Previous test measurements of ammonium emissions from light-duty gasoline PM have found ammonium concentrations in stoichiometric equilibrium with measurements of sulfate and nitrate, in the form of ammonium sulfate and ammonium nitrate (CitationFujita et al., 2007, CitationSchauer et al., 2006, 2008). The source of the filter-measured ammonium in these studies may be the reaction of the sulfate ion and ammonia gas in the filters (CitationSchauer, 2008). Because filter mass is used as the reference for estimating the particulate matter composition in this study, ammonium is imputed assuming the sulfate and nitrate is emitted as ammonium sulfate and ammonium nitrate.
Metal-bound oxygen
In comparing the element measurements to the filter mass, we accounted for the metal-bound oxygen by using the oxide state of each metal shown in . Calcium, zinc, and magnesium are assumed to be in the form of phosphates and sulfates, which is consistent with chemical analyses of light-duty gasoline particles (CitationSodeman et al., 2005), light-duty catalytic converters (CitationO’Neill et al., 2006), and diesel particulate ash (CitationSappock, 2009). In , calcium, zinc, and magnesium are not listed in oxide states, because the oxygen is associated with the phosphate and sulfates. Common oxide states used for other elements are taken from CitationReff et al. (2009), including averages where several common oxide states are assumed. Chlorine and bromine are not assumed to be bound to oxygen.
Table 5. Oxide states assumed for calculation of metal-bound oxygen
Elemental carbon
IMPROVE-TOR elemental carbon measurements were made on each of the vehicles tested in the speciation sample. Because all vehicles had BC measurements with the photoacoustic instrument, this subset of vehicles is used to compare the TOR-EC and photoacoustic BC measurements (EPA, 2008). For conventional diesel exhaust, the two instruments provide similar magnitude of emissions (CitationMoosmüller et al., 2001). In the KCVES, the two instruments provided close to 1:1 agreement for the heavily loaded bag 1 samples, but exhibited poor correlation for the lightly loaded bag 2 and bag 3 samples (Figures S7–S9). The difference in the BC and TOR-EC emission rates on the lightly loaded PM samples is attributed to the adsorption of organic compounds on the quartz filters (EPA, 2008).
The BC measurements from the photoacoustic instrument are used in the PM2.5 profile instead of elemental carbon since these were less affected by positive artifact at low emission levels and were measured on all 496 vehicles. In the final PM2.5 profile, the BC measurements reported from the photoacoustic instrument are used to estimate the CMAQv5.0 AE6 definition of EC (, shown later). The BC are not adjusted to represent EC because of the close 1:1 agreement observed in the bag 1 samples (Figure S7).
Organic matter
To account for positive measurement artifact of the organic carbon and the noncarbon organic material, the OM emission rates can be estimated using the following equation:
A backup quartz-fiber filter placed in series behind the primary filter can be used to estimate the positive organic artifact (b in eq 1). However, a backup quartz-fiber filter was only measured on a subset of 10 vehicles sampled during the winter phase of the KCVES (CitationFujita et al., 2006b). Additionally, using a backup filter correction assumes that the adsorption of the vapors is equal on both the primary and secondary filters and can be problematic for low-emitting vehicles where the OC measurements can be as large on the backup filter as on the primary filters (CitationFujita et al., 2006b). Also, because the volatilized silicone rubber from the sampling system likely contributed to the primary OC measurements, a backup filter would not account for the silicone measurement artifact.
Rather than using backup filter correction of the OC measurements, we used a mass-balance approach to estimate the OM emission rates. This approach enables the artifact correction and silicone contamination correction to be estimated from data collected on each test. Mass balance approaches have been used to estimate atmospheric organic carbon concentrations (CitationFrank, 2006; CitationEl-Zanan et al., 2009) and were previously used by the EPA in the postprocessing of MOVES on-highway PM2.5 emission inventories for air quality modeling (CitationStrum, 2011). The organic matter emissions were estimated for each test by the difference of the measured total mass and the individual components, as shown in eq 2:
The OM emission rates estimated from the mass balance approach are compared to the measured organic carbon emission rates in . Simple linear regression models are fit to the data. In each case, the intercept is negative, indicating a positive artifact in the measurements as proposed in eq 1. The slope of the regression ranges from 1.19 to 1.24, which corresponds well with the range of OM to OC ratios reported in the Background section.
Figure 3. Average PM2.5 fraction of each measured species for cold start emissions by model-year group, accompanying 95% confidence intervals.
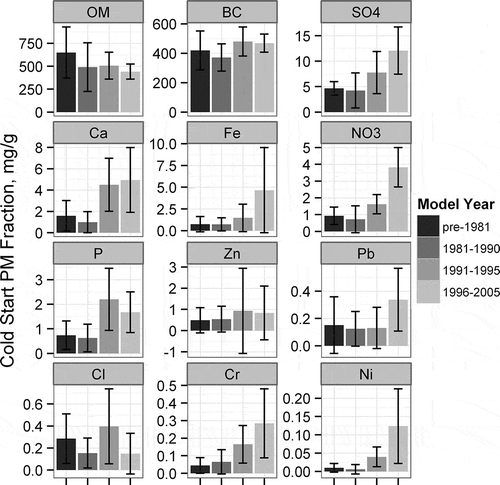
As shown in , the mass-balance approach occasionally yields either negative estimates of OM or OM estimates that are much larger than the original OC measurements. Nineteen of the 270 estimated OM values are negative. Seven of the negative OM estimates resulted from the photoacoustic measurements being larger than the elemental carbon or gravimetric filter mass measurements. The photoacoustic measurements are used exclusively rather than the IMPROVE-EC values in the final PM speciation profile. However, in cases where (1) the reconstructed PM mass is greater than the measured filter mass and (2) the photoacoustic measurements are larger than the TOR-EC measurements, the TOR-EC measurements are used in eq 2 to estimate positive OM emission rates. The remaining 12 negative OM values were imputed with zero values. To avoid OM rates being biased high from the mass-balance approach, the OM estimated values are capped at twice the measured OC emission rates, which impacted 23 of the 270 initially estimated OM values. A factor of 2 is selected because it is the largest OM/OC ratio reported in a range of combustion sources in CitationReff et al. (2009).
The estimated ratio of estimated OM to measured OC by bag of the LA-92 and model year group is displayed in . In general, the OM/OC ratio is greater than 1, which is expected due to the additional mass of the noncarbon atoms in the organic matter. The estimated ratio is less than one for some model year groups in Bag 3, indicating that much of the organic carbon measured in Bag 3 is considered a positive artifact as defined in this paper. Contribution of silicone to the measured OC could also be another reason many of the ratios in are less than 1.2, especially for the older vehicles.
Table 6. Mass-balance OM compared to measured OC
Profile analysis
Average PM2.5 profiles are estimated for cold start and hot stabilized running processes using the bag-specific PM2.5 reconstructed data. Running emissions in MOVES are defined as emissions from the vehicle when the engine block is warm and the operating catalyst temperature is reached, which we refer to as hot stabilized running. We use data from Bag 2 of the LA-92 cycle for the hot stabilized running speciation profile. Cold start emissions are typically defined as the incremental emissions produced from the initial cold start before the engine (and catalyst) have fully warmed. The PM2.5 emission rates for cold start in MOVES are based on the difference of Bag 1 and Bag 3 (warm start) emissions, which have identical speed traces. The cold start PM2.5 emission profile is similarly estimated by subtracting Bag 3 emissions from Bag 1 emissions for each of the measured species from the mass-balanced profiles. In modeling the cold start as Bag 1 – Bag 3, we assume that (1) all of the incremental cold start emissions are captured by Bag 1, and (2) the warm start is a minor contributor in comparison to the Bag 3 running emissions. In reality, a significant temperature dependence is observed in Bag 2 PM2.5 emissions (Figure S18), meaning that the LA-92 Bag 1 is likely too short a cycle to fully warm the vehicles to a stable hot stabilized running condition. The persistent temperature effect on the Bag 2 is considered in the development of the PM2.5 profile.
We developed the PM2.5 profiles using the largest sample size of measurements available. The BC fraction of PM2.5 is estimated using data from the full test program (496 vehicles) because photoacoustic and gravimetric filter measurements were made for each test. The data set includes 42 vehicles that were tested in both the summer and winter. For statistical analysis, the 42 vehicles are treated as separate vehicles in the summer and winter because they compose a small fraction of the total tests and because the large temperature impacts on emissions are anticipated to dominate inter-vehicle variability. For estimating BC/ PM2.5, tests are excluded that did not have detectable BC or PM2.5 emissions. For estimating the hot stabilized running emission rates on Bag 2, the sample size is reduced from 538 to 517 tests. For cold starts, additional tests are excluded when the BC or PM2.5 emissions on Bag 3 is greater than on Bag 1, which reduced the data set to 473 tests (Table S2).
The speciation sample (102 tests) is used to create the speciation profile for the composition of the organic matter, elements, and ions. Ninety-nine of the 102 speciation tests on Bag 2 have valid PM2.5, EC, and OC measurements for development of the hot stabilized running profile (Table S3). For cold starts, only 66 out of 102 speciation tests are deemed valid where roughly one-third of the samples are excluded due to negligible PM2.5, EC, and OC readings on calculated differenced values (Bag 1 – Bag 3). Due to the large number of excluded samples, the Bag 1 PM2.5 emissions of the excluded cold start tests were compared with all of the valid bag 1 PM2.5 emissions in Figure S2. No systematic bias is observed in the reduced or excluded PM2.5 Bag 1 emision rates, suggested that the reduced sample of the cold start emissions used for the speciation profile is still representative of the larger sample. Speciation factors are estimated for each of the species required by CMAQv5.0 and additional elements measured at significant levels (phosphorous, chromium, nickel, copper, zinc, bromine, and molybdenum).
Cold start emission profile
The reconstructed start PM2.5 measurements are initially averaged according to the eight vehicle strata and seasons in , and are used to evaluate the impact of season, vehicle type and model year group on the PM2.5 composition. As shown, the cold start profile is dominated by BC and OM emissions, with a very small inorganic contribution. The BC and PM2.5 cold start emission rates are much larger in the winter than the summer. In the full vehicle sample (473 vehicle tests), the PM2.5 emissions are larger in winter in each stratum (Figure S10), and the BC emissions are significantly larger in all but the pre-1981 truck strata (Figure S11). The BC/PM2.5 ratio remains relatively consistent across seasons using data from the full sample (Figure S12), suggesting that the BC and PM2.5 cold start emissions increase at the same rate at colder temperatures.
The overall emissions of OM, elements, and sulfate emissions also increase significantly at cold temperatures. However, the PM2.5 fraction of organic matter, sum of elements, and sulfate emissions does not vary significantly between seasons for each of the strata (Figures S14–S16). CitationHerrington et al. (2012) found that the PM2.5 proportions of key particulate organic classes (hopanes, steranes, and polycyclic aromatic hydrocarbons) were relatively invariant to changes in season in a subsample of 18 vehicles tested in both seasons in the KCVES. Both analyses suggests that while cold start LDGV PM2.5 emissions are very sensitive to temperature, the relative composition of the cold start PM2.5 emissions is consistent across temperatures. For the final cold start emission profile, the measurements taken in the summer and the winter are equally weighted.
shows that there are no strong differences in the composition between cars and trucks. This is supported by the BC/PM2.5 fraction between cars and trucks shown in Figure S12. graphs the cold start PM2.5 fraction of each measured species averaged by model year groups, to isolate the model-year impact on PM2.5 speciation. Overall, all PM2.5 species decrease with new model year vehicles (Figure S17), but important differences are noted in the proportion of each species. The BC fraction appears to increase slightly for the 1990 and later model year groups (Figure S11), but is difficult to determine due to the variability in the data. The data are pooled to two larger model year groups: pre-1991 and the 1991–2005 model year groups (Figure S13), where the increase in BC/PM2.5 is shown to clearly be significant at the 95% confidence level. Such an increase in the BC/PM2.5 fraction is consistent with previous research (CitationRobert et al., 2007). The fraction of OM decreases as the overall PM2.5 is reduced in the newer model year groups. The trend is reversed for the inorganics, where the relative fraction of sulfate and most elements increases with decreases in PM2.5. Only elements that were measured at significant levels in the cold starts are included in the cold start profile, which leaves 7 of the 14 elements identified as significant in the composite profile (Ca, Fe, P, Zn, Pb, Cr, Ni).
Hot stabilized running emission profile
plots the reconstructed emission rates averaged by season and strata for the PM2.5 hot stabilized running emissions. An increase in PM2.5 emissions in the winter sample is observed in the mean PM2.5 emissions of the newest model year vehicles (1996–2005) in , but no consistent trend is observed for the older model year vehicles. The variability of the PM2.5 emissions across season is not surprising, given the small sample size of vehicles, and differences in the vehicles tested in the summer and winter rounds. The wintertime increase in PM2.5 emissions for the newest vehicles appears to occur in the BC and OM fractions. The hot stabilized running BC emissions are evaluated in the supporting information on the full data set vehicles (Figures S19 and S20). While BC emissions increase in the winter, there is no discernible season/temperature trend on the BC/PM2.5 fraction.
The large contribution of volatized silicone is especially evident in the PM2.5 composition of the Bag 2 emissions. The trucks have a higher contribution, which is expected due to higher exhaust temperatures and larger exhaust tail pipes, which expose more silicone rubber to the hot exhaust. The summer samples have a larger contribution of silicone. Besides the silicone contribution, the composition of the remaining PM2.5 is similar between cars and trucks for each model-year group. The only significant difference noted between the speciation profiles for cars and trucks is for the pre-1981 model-year group. The pre-1981 cars had a relatively large BC fraction, and the trucks have a large sulfate fraction, which could be due to higher prevalence of oxidation catalysts in pre-1981 trucks. However, this difference could also be attributed to the small sample size of the oldest model-year group, and large variation of emissions from the oldest and most deteriorated LDGVs, which is expected based on previous studies (CitationLough et al., 2007; CitationLough and Schauer, 2007).
To create a speciation profile for emissions modeling for hot stabilized running emissions, the summer and winter measurements are equally weighted, and the silicone contribution to PM is removed. The average hot stabilized running PM composition is significantly different according to model year groups as shown in . The fractions of the major components—BC, OM, and SO4—are relatively unchanged between model-year groups, while the fraction of metals and other minor elements significantly increases with newer model-year vehicles. Each of the 14 elements significant in the composite profile is also significant in the hot stabilized running profile.
Final PM2.5 speciation profiles for light-duty gasoline vehicles
We considered the level of aggregation when constructing PM speciation profiles for emission modeling in MOVES. Although the data show model-year differences in the PM composition, we propose using a fleet-average PM profile to capture the prevalence of high emitters within all model year-groups, and to avoid overfitting model year trends. Malfunctioning high-emitting vehicles are known to contribute a significant share of in-use PM emissions from light-duty vehicles (CitationRobert et al., 2007; CitationCarroll et al., 2011; CitationLough and Schauer, 2007; CitationCadle et al., 1999). High-emitting gasoline emissions have a highly variable PM composition, due to failed emission control systems, excessive oil consumption, and poor fuel control. Previous analysis of the KCVES suggested that the speciation subsample (102 tests) provides a reasonable estimate of the total PM mass compared to the full sample (522 tests), but the speciation sample underestimated the high emitting vehicles in the newer model-year groups (CitationSonntag et al., 2012). Other test programs have confirmed that high-emitting gasoline vehicles also occur in modern vehicles, such as 1990s-era vehicles with electronic fuel injection (CitationRobert et al., 2007; CitationCarroll et al., 2011). The speciation sample size was deemed too limited to accurately capture the impact of deteriorated vehicles within each model-year group. Using all the data in a fleet-average approach is deemed more appropriate to characterize representative PM2.5 composition from in-use light-duty gasoline vehicles.
The fleet-average PM speciation profiles are calculated using seasonal, vehicle miles traveled (VMT), and PM mass weighting. The PM profile is calculated using the ratio of the mean emissions of each PM2.5 species, also referred to by others as a mass-normalized emission profile (CitationSchauer et al., 2006). The vehicle tests from each season are equally weighted, and the vehicle tests are averaged according to the calculated contribution to annual VMT in the Kansas City MSA (). The PM species are calculated using the Ratio statement in SAS 9.2 Proc SurveyMeans, which calculates standard errors for the ratios using Taylor series linearization. By using VMT and mass weighting, the profile scales the contribution of older and higher emitting vehicles up according to their high PM emissions, but also scales their contribution down based on their relatively small contribution of VMT.
The OC, elements, and ion emission factors are harmonized with the BC speciation factor to create a single PM speciation profile. First, the BC ratios computed from the full sample are used, because they are based on the largest number of vehicles. Then the speciation factors calculated from the speciation subsample are used to speciate the “non-BC” fraction from the full sample. This caused a 6.17% increase in the non-BC species for cold start emissions and a 0.11% increase in hot stabilized running emissions due to differences in the mean BC fractions measured from the full samples and the speciation samples. The resulting PM speciation factors for the hot stabilized running and cold start emissions are located in . The OC fraction is back-calculated from the OM fraction assuming a 1.2 organic matter correction. “CMAQ5.0 AE6 unspeciated” is composed of unspeciated filter mass, and species not reported by CMAQ 5.0 AE6 (primarily composed of metal-bound oxygen, and two measured metals not reported by CMAQ: zinc and phosphorous). The 95% confidence intervals are based on the original samples as shown in . Confidence intervals are also provided for the imputed values but should be considered semiqualitative compared to the measured species.
Table 7. PM2.5 profile for cold start and hot stabilized running emissions, with weighted average using vehicle miles traveled (VMT)
Summary
Important differences between cold start and hot stabilized running emissions are captured in the developed PM profiles. Cold start PM emissions are dominated by BC emissions from incomplete fuel combustion. BC emissions account for 44% of the cold start emissions, but only 14% of the hot stabilized running emission profile. The fraction of OC is relatively constant between the start and running emissions, accounting for 43% of the cold start and 56% of the hot stabilized running emissions. The estimated OC fraction is smaller than previous LDGV test programs (CitationSchauer et al., 2006; CitationZielinska et al., 2004), which may be due to (1) the positive OC artifact correction accounted for in this study and (2) this profile being weighted heavily toward newer vehicles, which have lower fractions of OC in the total PM emissions.
Important differences between the cold start and hot stabilized running PM2.5 profiles are evident in the inorganic fractions. The sum of measured elements constitutes 5.6% of the hot stabilized running emission profile, but only 1.0% of the cold start emission profile. Many of the elements that were significant in the LA-92 composite cycles were not significantly different from zero in the cold start emission profile. The dominant elements in the hot stabilized running emission profile are engine and exhaust system wear components (iron, aluminum) and lubricating oil additives (calcium, phosphorous, zinc). Similarly, the inorganic ion fraction is considerably higher in the hot stabilized running emissions (10.3%) compared to the cold start emissions (1.6%).
By incorporating separate PM profiles for cold start and hot stabilized running LDGV emissions, important differences in the composition of primary PM2.5 emissions can be modeled. The LDGV start emission rates are very sensitive to ambient temperature in the MOVES model, and essentially double with every 15°F drop in ambient temperature, based on previous analysis (CitationNam et al., 2010), whereas MOVES running emissions are less sensitive to ambient temperature. With separate profiles for start and running operating conditions, different PM composition can be modeled for regions with different cold start and hot stabilized running emission contributions. For example, PM emission inventories in cold regions can model an increased fraction of fuel-derived BC emissions due to a larger contribution from PM start emissions.
The developed profiles provide an important update on PM characterization from a large sample of in-use LDGVs as of 2005 for use in emission modeling. The speciation profile is VMT weighted to be representative of the U.S. on-road vehicle fleet, as represented by the vehicles in the Kansas City Metropolitan Area. The same data could be reweighted to represent vehicle fleets in other areas such as developing countries, where test data are limited but the fleet technology and age distribution can be estimated.
Additional speciation data are needed for estimating the impacts of changing fuels and technologies. For the next generation of vehicles, the composition of PM is expected to become increasingly dominated by BC emissions from both low-emitting port-fuel injected vehicles (CitationRobert et al., 2007; CitationKleeman et al., 2008; CitationAyala et al., 2011; Robinson et al., 2013) and gasoline direct injection vehicles (CitationAndersson, 2009; CitationStorey et al., 2012; CitationForestieri et al., 2013). Additional light-duty gasoline PM profiles should be added to MOVES and SPECIATE as such data becomes available.
Supplemental Data
Supplemental data are available for this paper. Go to the publisher’s online edition of the Journal of the Air & Waste Management Association. Supporting information includes details on quality assurance, details on the statistical test used to identify significant measurements, additional information on quality assurance of element and ion measurements, analysis of the silicone contamination issue, and supporting figures used in developing the PM speciation profile.
Supplementary_Material.docx
Download MS Word (1.3 MB)Acknowledgment
This work was made possible by the contributions of many individuals and organizations. Contributors at the EPA include Chad Bailey, Joseph Somers, Madeline Strum, Richard Snow, James Faircloth, John Koupal, Edward K. Nam, and Megan Beardsley. Eric Fujita and David Campbell at Desert Research Institute (DRI) provided addition information on the chemical measurements made in KCVES. Sandeep Kishan and Scott Fincher at Eastern Research Group (ERG) provided assistance in obtaining data collected from the KCVES. The KCVES was organized and implemented by ERG, and its subcontractors, DRI, BKI, NuStats, Sensors, and ESP, under the direction of Sandeep Kishan.
Funding
Funding for PM speciation profile development work reported in this paper was provided through the American Association for the Advancement of Science (AAAS) fellowship under Cooperative Agreement X3-83459201 awarded by the EPA to the AAAS, and through the Oak Ridge Institute for Science and Education (ORISE) program funded by the EPA Office of Transportation and Air Quality.
Additional information
Notes on contributors
Darrell B. Sonntag
Darrell B. Sonntag and Carl R. Fulper are engineers, and Catherine A. Yanca is an environmental scientist at the National Vehicle Fuel Emissions Laboratory in Ann Arbor, MI.
Richard W. Baldauf
Richard W. Baldauf is an engineer with concurrent positions at the National Vehicle Fuel Emissions Laboratory in Ann Arbor, MI, and the National Risk Management Research Laboratory in Research Triangle Park, NC.
References
- Andersson, J., M. Keenan, and K. Akerman. 2009. GDI particles—Legislation, current levels and control. RD. 09/99801.1.Presented at the 2009 Cambridge Particle Meeting, March 16.
- Ayala, A., S. Zhang, P. Rieger, M. Kamboures, Y. Yu, J. Sandoval, S. Hu, J. Collins, O. Chang, and W. McMahon. 2011. Prospects for low PM and low climate impacts in future advanced clean cars for California. Presented at the 21st CRC Real World Emissions Workshop, March 20–23.
- Carroll, J.N., I.A. Khalek, L.R. Smith, E. Fujita, and B. Zielinska. 2011. Collaborative lubricating oil study on emissions. October. NREL/SR-5400-52668. http://www.nrel.gov/docs/fy12osti/52668.pdf.
- Cadle, S.H., P.A. Mulawa, E.C. Hunsanger, K. Nelson, R.A. Ragazzi, R. Barrett, G.L. Gallagher, D.R. Lawson, K.T. Knapp, and R. Snow. 1999. Composition of light-duty motor vehicle exhaust particulate matter in the Denver, Colorado area. Environ. Sci. Technol. 33:2328–39. doi:10.1021/es9810843
- Cheung, K.L., L. Ntziachristos, T. Tzamkiozis, J.J. Schauer, Z. Samaras, K.F. Moore, and C. Sioutas. 2010. Emissions of particulate trace elements, metals and organic species from gasoline, diesel, and biodiesel passenger vehicles and their relation to oxidative potential. Aerosol Sci. Technol. 44:500–13. doi:10.1080/02786821003758294
- Cheung, K.L., A. Polidori, L. Ntziachristos, T. Tzamkiozis, Z. Samaras, F.R. Cassee, M. Gerlofs, and C. Sioutas. 2009. Chemical characteristics and oxidative potential of particulate matter emissions from gasoline, diesel, and biodiesel cars. Environ. Sci. Technol. 43(16): 6334–40. doi:10.1021/es900819t
- Cho S.-H., H. Tong, J.K. McGee, R.W. Baldauf, Q.T. Krantz, and M.I. Gilmour. 2009. Comparative toxicity of size-fractionated airborne particulate matter collected at different distances from an urban highway. Environ. Health Perspect. 117(11): 1682–89.
- Chow, J.C., J.G. Watson, L.C. Pritchett, W.R. Pierson, C.A. Frazier, and R.G. Purcell. 1993. The DRI Thermal/Optical Reflectance carbon analysis system: Description, evaluation and applications in U.S. air quality studies. Atmos. Environ. 27A:1185–201. doi:10.1016/0960-1686(93)90245-T
- CAMx v5.40 User’s Guide. 2011. Chemistry Mechanisms. September. http://www.camx.com/camx/files/b6/b65abed0-a7d4-498b-8698-b2cf5c23cbfd.pdf
- El-Zanan, H.S, B. Zielinska, L.R. Mazzoleni, and D.A. Hansen. 2009. Analytical determination of the aerosol organic mass-to-organic carbon ratio. J. Air Waste Manage Assoc. 59(1): 58–69. doi:10.3155/1047-3289.59.1.58
- Forestieri, S., S. Collier, T. Kuwayama, Q. Zhang, M.J. Kleeman, and C.D. Cappa. 2013. Real-time black carbon emission factor measurements from light duty vehicles. Environ. Sci. Technol. 47: 13104–13112. doi:10.1021/es401415a.
- Fujita, E.M., D.E. Campbell, and B. Zielinska, 2006a. Chemical analysis of lubrication oil samples from a study to characterize exhaust emissions from light-duty gasoline vehicles in the Kansas City Metropolitan Area. Final report submitted to the National Renewable Energy Laboratory, NREL contract no. ACI-5-55528-01, Golden, CO, and the Coordinating Research Council, CRC E-69A, Alpharetta, GA. December 31. http://www.crcao.com/reports/recentstudies2007/E-69a/E-69a_Final_Report.pdf.
- Fujita, E.M., D.E. Campbell, B. Zielinska, and J.C. Chow. 2006b. Kansas City gasoline vehicle characterization study—Effect of sampling temperature on chemical speciation. ERG NO.; 3491.00.001.001. NREL subcontract number ACI-5-55500-01. NREL project ES04-2. May 31.
- Fujita, E.M., B. Zielinska, D.E. Campbell, W.P. Arnott, J.C. Sagebiel, L. Mazzoleni, J.C. Chow, P.A. Gabele, W. Crews, R. Snow, N.N. Clark, W.S. Wayne, and D.R. Lawson. 2007. Variations in speciated emissions from spark-ignition and compression-ignition motor vehicles in California’s South Coast Air Basin. J. Air Waste Manage. Assoc. 57:705–720. doi:10.3155/1047-3289.57.6.705
- Fulper, C.R., S. Kishan, R.W. Baldauf, M. Sabisch, J. Warila, E.M. Fujita, C. Scarbro, W.S. Crews, R. Snow, P. Gabele, R. Santos, E. Tierney, and B. Cantrell. 2010. Methods of characterizing the distribution of exhaust emissions from light-duty, gasoline-powered motor vehicles in the U.S. fleet. J. Air Waste Manage. Assoc. 60:1376–87. doi:10.3155/1047-3289.60.11.1376
- Frank, N. 2006. SANDWICH material balance approach for PM2.5 data analysis. Presented at the 2006 National Air Monitoring Conference, Las Vegas, NV, November 6–9.
- Hays, M.D., S. Cho, R.W. Baldauf, J.J. Schauer, and M. Shafer. 2011. Particle size distributions of metal and non-metal elements in an urban near-highway environment. Atmos. Environ. 45(4):925–34. doi:10.1016/j.atmosenv.2010.11.010
- Heck, R.M., R.J. Farrauto, and S.T. Gulati. 2002. Catalytic Air Pollution Control. New York, NY: John Wiley & Sons.
- Herrington, J.S., M.D. Hays, B.J. George, and R.W. Baldauf. 2012. The effects of operating conditions on semivolatile organic compounds emitted from light-duty, gasoline-powered motor vehicles, Atmos. Environ. 54:53–59. doi:10.1016/j.atmosenv.2012.02.043
- Hsu, Y., R. Strait, S. Roe, and D. Holoman. 2006. SPECIATE 4.0, Speciation database development documentation. Technical report EPA/600/R-06/161. http://www.epa.gov/ttn/chief/software/speciate/speciate4/documentation/speciatedoc_1206.pdf
- Keller, R.W. 1986. Effects of engine oils on silicone oil seal material. SAE Technical Paper Series 860494. doi:10.4271/860494
- Kleeman, M.J., S.G. Riddle, M.A. Robert, and C.A. Jakober. 2008. Lubricating oil and fuel contributions to particulate matter emission from light-duty gasoline and heavy-duty diesel vehicles. Environ. Sci. Technol. 42:235–42. doi:10.1021/es071054c
- Lough, G.C., J.J. Schauer, J. Park, M.M. Shafer, J.T. DeMinter, and J.P. Weinstein. 2005. Emissions of metals associated with motor vehicle roadways. Environ. Sci. Technol. 39(3): 826–36. doi:10.1021/es048715f
- Lough, G.C., C.C. Christenson, J.J. Schauer, J. Tortorelli, E. Bean, D.R. Lawson, N.N. Clark, and P.A. Gabele, 2007. Development of molecular marker source profiles for emissions from on-road gasoline and diesel vehicle fleets. J. Air Waste Manage. Assoc. 57:1190–99. doi:10.3155/1047-3289.57.10.1190
- Lough, G.C., and J.J. Schauer. 2007. Sensitivity of source apportionment of urban particulate matter to uncertainty in motor vehicle emissions profile. J. Air Waste Manage. Assoc. 57:1200–13. doi:10.3155/1047-3289.57.10.1200
- Maricq, M.M., R.E. Chase, and D.H. Podsiadlik. 1999. Vehicle exhaust particle size distributions: A comparison of tailpipe and dilution tunnel measurements. SAE Paper 1999-01-1461. doi:10.4271/1999-01-1461
- Maricq, M.M., J.J. Scente, M.J. Loos, and R. Vogt. 2011. Motor vehicle PM emissions measurement at LEV III levels. SAE Int. J. Engines 4(1): 597–609. doi:10.4271/2011-01-0623
- May, A.A., N.T. Nguyen, A.A. Presto, T.D. Gordon, E.M. Lipsky, M. Karve, A. Gutierrez, W.H. Robertson, M. Zhang, C. Brandow, et al. 2014. Gas- and particle-phase primary emissions from in-use, on-road gasoline and diesel vehicles. Atmos Environ. 33:247–260.
- Moosmüller, H., W.P. Arnott, C.F. Roger, J.L. Bowen, J.A. Gillies, W.R. Pierson, J.F. Collins, T.D. Durbin, and J.M. Norbeck. 2001. Time-resolved characterization of diesel particulate emissions. 2. Instruments for elemental and organic carbon measurements. Environ. Sci. Technol. 35:1935–42. doi:10.1021/es0015242
- Nam, E., C. Fulper, J. Warila, J. Somers, H. Michaels, R. W. Baldauf, R. Rykowski, and C. Scarbro. 2008. Analysis of Particulate matter emissions from light-duty gasoline vehicles in Kansas City. EPA420-R-08-010. http://www.epa.gov/otaq/emission-factors-research/420r08010.pdf
- Nam, E., S. Kishan, R. W. Bauldauf, C. R. Fulper, M. Sabisch, and J. Warila. 2010. Temperature effects on particulate matter emissions from light-duty, gasoline-powered motor vehicles. Environ. Sci. Technol. 44:4672–77. doi:10.1021/es100219q
- NHTSA. 2006. Vehicle Survivability and Travel Mileage Schedules. DOT HS 809 952, January 2006. http://www-nrd.nhtsa.dot.gov/Pubs/809952.PDF
- Noll, J., and M.E. Birch. 2008. Effects of sampling artifacts on occupational samples of diesel particulate matter. Environ. Sci. Technol. 42:5223–28.doi:10.1021/es702883k
- O’Neill, A., D. Uy, and M. Jagner. 2006. Characterization of phosphates found in vehicle-aged exhaust gas catalysts: A Raman study. SAE Technical Paper 2006-01-0410. Presented at SAE 2006 World Congress & Exhibition, April 3, Detroit, MI. doi:10.4271/2006-01-0410
- Robert, M.A., S. VanBergen, M.J. Kleeman, and C.A. Jakober. 2007. Size and composition distributions of particulate matter emissions: Part 1—Light-duty gasoline vehicles. J. Air Waste Manage. Assoc. 57:1414–28. doi:10.3155/1047-3289.57.12.1414
- Reff, A., P.V. Bhave, H. Simon, T.G. Pace, G.A. Pouliot, J.D. Mobley, and M. Houyoux. 2009. Emissions inventory of PM2.5 trace elements across the United States. Environ. Sci. Technol. 43(15): 5790–96. doi:10.1021/es802930x
- Sappock, A. 2009. The nature of lubricant-derived ash-related emissions and their impact on diesel aftertreatment system performance. Cambridge, MA: Massachusetts Institute of Technology.
- Schauer, J.J., G.C. Lough, M.M. Shafer, W.F. Christensen. M.F. Arndt, J.T. DeMinter, and J.-S. Park. 2006. Characterization of metals emitted from motor vehicles. Health Effects Institute Research Report Number 133. http://pubs.healtheffects.org
- Schauer, J.J., M.J. Kleeman, G.R. Cass, and B.R.T. Simoneit. 2002. Measurement of emissions from air pollution sources. 5. C1–C32 organic compounds from gasoline-powered motor vehicles. Environ. Sci. Technol. 36:1169–80. doi:10.1021/es0108077
- Schauer, J.J., C.G. Christensen, D.B. Kittelson, J.P. Johnson, and W.F. Watts. 2008. Impact of ambient temperatures and driving conditions on the chemical composition of particulate matter emissions from non-smoking gasoline-powered motor vehicles. Aerosol Sci. Technol. 42(3): 210–23. doi:10.1080/02786820801958742
- Simon, H. 2012. CMAQv5.0 PMother speciation. November 15. Community Multiscale Air Quality (CMAQ) Model Wiki. http://www.airqualitymodeling.org/cmaqwiki/index.php?title=CMAQv5.0_PMother_speciation
- Sodeman, D.A., S.M. Toner, and K.A. Prather. 2005. Determination of single particle mass spectral signatures from light-duty vehicle emissions. Environ. Sci. Technol. 39(12): 4569–80. doi:10.1021/es0489947
- Sonntag, D.B., C.R. Bailey, C.R. Fulper, and R.W. Baldauf. 2012. Contribution of lubricating oil to particulate matter emissions from light-duty gasoline vehicles in Kansas City. Environ. Sci. Technol. 46(7): 4191–99. doi:10.1021/es203747f
- Strum, M. 2011. Interim approach to develop CMAQ PM2.5. species from partially-speciated MOVES2010 Exhaust PM2.5. Appendix D: Memo Describing the Differences in MOVES speciated PM and CMAQ PM. 2007v5 Emissions Platform TSD Appendices. http://epa.gov/ttn/chief/emch/2007v5/2007v5_TSD_Appendices_14dec12.pdf
- Storey, J., T. Barone, J. Thomas, and S. Huff. 2012. Exhaust particle characterization for lean and stoichiometric DI vehicles operating on ethanol–gasoline blends. SAE Technical Paper 2012-01-0437. Presented at the SAE 2012 World Congress & Exhibition, April 24, Detroit, MI. doi:10.4271/2012-01-0437
- U.S. Environmental Protection Agency. 2008. Kansas City PM characterization study. Final report. EPA420-R-08-009. Ann Arbor, MI: Assessment and Standards Division Office of Transportation and Air Quality U.S. Environmental Protection Agency. EPA contract no. GS 10F-0036K, October 27, revised April 2008 by EPA staff. http://www.epa.gov/oms/emission-factors-research/420r08009.pdf.
- U.S. Environmental Protection Agency. 2011. Development of emission rates for light-duty vehicles in the Motor Vehicle Emissions Simulator (MOVES2010). Final report. EPA-420-R-11-011. August. http://www.epa.gov/otaq/models/moves/documents/420r11011.pdf
- U.S. Environmental Protection Agency. 2012. Black carbon report to Congress. EPA-450/R-12-001. http://www.epa.gov/blackcarbon/2012report/fullreport.pdf.
- Van der Schoot, M., S. Bhargava, D. Akolekar, K. Föger, et al. 2001. Deterioration of automotive catalytic converters: Physical catalyst characterization. SAE Technical Paper 2001-01-3691. doi:10.4271/2001-01-3691
- Watson, M., and P. Dietz. 2009. A novel technology for the inhibition of ceramic fiber erosion and promotion of substrate retention in catalytic converters. SAE Technical Paper 2009-01-0975. Presented at SAE World Congress & Exhibition, April 20, Detroit, MI.
- Zielinska, B., J. Sagebiel, J.D. McDonald, K. Whitney, and D.R. Lawson. 2004. Emission rates and comparative chemical composition from selected in-use diesel and gasoline fueled vehicles. J. Air Waste Manage. Assoc. 54:1138–50. doi:10.1080/10473289.2004.10470973