Abstract
Animal feeding operations (AFOs) produce particulate matter (PM) and gaseous pollutants. Investigation of the chemical composition of PM2.5 inside and in the local vicinity of AFOs can help to understand the impact of the AFO emissions on ambient secondary PM formation. This study was conducted on a commercial egg production farm in North Carolina. Samples of PM2.5 were collected from five stations, with one located in an egg production house and the other four located in the vicinity of the farm along four wind directions. The major ions of NH4+, Na+, K+, SO42−, Cl−, and NO3− were analyzed using ion chromatography (IC). In the house, the mostly abundant ions were SO42−, Cl−, and K+. At ambient stations, SO42−, and NH4+ were the two most abundant ions. In the house, NH4+, SO42−, and NO3− accounted for only 10% of the PM2.5 mass; at ambient locations, NH4+, SO42−, and NO3− accounted for 36–41% of the PM2.5 mass. In the house, NH4+ had small seasonal variations indicating that gas-phase NH3 was not the only major force driving its gas–particle partitioning. At the ambient stations, NH4+ had the highest concentrations in summer. In the house, K+, Na+, and Cl− were highly correlated with each other. In ambient locations, SO42− and NH4+ had a strong correlation, whereas in the house, SO42− and NH4+ had a very weak correlation. Ambient temperature and solar radiation were positively correlated with NH4+ and SO42−. This study suggests that secondary PM formation inside the animal house was not an important source of PM2.5. In the vicinity, NH3 emissions had greater impact on PM2.5 formation.
Implications
The chemical composition of PM2.5 inside and in the local vicinity of AFOs showed the impact of the AFO emissions on ambient secondary PM2.5 formation, and the fate and transport of air pollutants associated with AFOs. The results may help to manage in-house animal facility air quality, and to develop regional air quality control strategies and policies, especially in animal agriculture-concentrated areas.
Introduction
Animal feeding operations (AFOs) raise animals in confinement areas. While AFOs provide food supplies for meat, egg, and dairy products, they also pose substantial threats to water, air quality, and public health because of the amount of animal wastes and air pollutants generated from AFOs (National Research Council [NRC], 2003). The National Research Council (Citation2003) reported that current air emission factors for AFOs were incomplete and don’t allow the regulatory agencies to determine compliance status with U.S. Environmental Protection Agency (EPA) regulations. Consequently, under EPA supervision, the National Air Emissions Monitoring Study (NAEMS) was conducted to assess emissions from different livestock operations across different regions in the United States. The baseline emission data collected by the NAEMS have been used by the EPA to develop new emission assessment methods and regulations to properly regulate AFOs (Purdue University, Citation2006; Li et al., Citation2013; Wang-Li et al., Citation2013a; Wang-Li et al., Citation2013b).
As a criteria pollutant, PM2.5 can originate from anthropogenic and natural sources or can form through atmospheric reactions (Hinds, Citation1998). Geological materials, organic matter, elemental carbon, sulfate (SO42−), nitrate (NO3−), and ammonium (NH4+) are six major components accounting for the majority of PM2.5 mass (EPA, 1997). The water-soluble ions (SO42−, NO3−, NH4+, etc.) in many areas account for a major fraction of PM2.5 mass (Kerminen et al., Citation2001). PM2.5 from AFOs can have strong impact on air quality in animal production areas (Yang et al., Citation2011). Martin et al. (Citation2008) studied particle composition and size distribution in and around a swine operation, and found downwind ions concentrations increased significantly. Lammel et al. (Citation2004) found that livestock farms in southern Germany were sources for both primary and secondary PM.
The study of ambient PM and co-pollutants’ patterns and characterization in rural AFOs-intensive areas is important to assess AFOs adverse environmental impact and resulting health effects. In this study, a field investigation was conducted to characterize the major ions of PM2.5 inside and in the immediate vicinity of a large commercial egg production farm. This knowledge provides a foundation to assess the role of AFOs on ambient air quality in agriculture-intensive rural areas, which is absent in the EPA national ambient air quality monitoring networks.
Methodology
Field PM2.5 sampling stations
Sampling of PM2.5 was conducted on a commercial egg production farm (also known as a layer farm) in North Carolina. The layout of the farm and the five PM sampling locations (four surrounding stations and one in-house station) are shown in . This farm had four high-rise tunnel ventilated houses (1–4), two high-rise cross-ventilated houses (5 and 6), three shallow-pit naturally ventilated houses (92, 102, and 103), an egg processing plant, and two wastewater treatment lagoons. Houses 1–4 were 177 m long and 18 m wide, housing approximate 103,000 birds in a low-density condition (400 cm2/hen). Houses 5 and 6 were 177 m long and 21 m wide, housing approximate 132,000 birds in a low-density condition (400 cm2/hen). Manure accumulation in high-rise houses was 365 days and was stored in the first floor. Genetic W36 laying hens stayed on the second floors of the high-rise houses for 644 days with average weight of 1.5 kg. House 4 was selected for PM sampling for this study. The in-house sampling station was located 4 m upstream of the first floor middle fan in house 4. This fan was the primary exhaust fan (also known as minimum ventilation fan) and it ran all the time.
Figure 1. The layer farm layout and the locations of PM sampling stations (not to scale). ST1 = PM sampling station 1; ST2 = PM sampling station 2; ST3 = PM sampling station 3; ST4 = PM sampling station 4; ST5 = PM sampling station 5. The distance marked at each station is relative to ST1 (Wang-Li et al., Citation2013a).
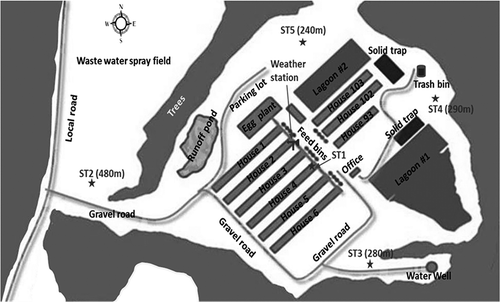
Ambient PM sampling was designed to quantify possible PM fate and transport in the vicinity of the layer farm. Although the annual prevailing winds were from the southwest, four ambient PM sampling stations (ST2–ST5 in ) were placed around the farm in four different directions. Accessibility, security, safety, power availability, and sufficient operation space were main criteria used to determine these ambient sampling locations. Moreover, these ambient stations were located in typical agriculture intensive rural area. The only major highway was 12.7 km away to the southeast of the farm. Around the farm, there were no large point sources, and the only nearest coal-fired power station was 20 km away to the southeast with a capacity of 115 MW.
A 10-m weather tower was used to collect meteorological data. The tower was located between houses 2 and 3 at the east end. Solar radiation, wind speed and direction, ambient temperature, and relative humidity (RH) were continuously monitored with temporal frequency in 1 min. Time-averaged transport winds were defined as the average wind speed and direction of all winds within the sampling periods (Li et al., Citation2011). The U component (zonal) and the V component (meridional) of the wind at each minute were calculated as
Field PM2.5 sample collection
Partisol (model 2300) chemical speciation samplers were used for simultaneously taking 24-hr PM2.5 samples at five sampling stations for four seasons from December 2008 to December 2009, and in total 1012 filter samples were collected. This type of sampler was equipped with four filter cartridges and a flow control system. In this study, only three cartridges with different filters (nylon, Teflon, and quartz) were used to collect PM2.5 samples. The nylon filter was used for ion analyses, the Teflon filter was used for trace element and mass analyses, and the quartz filter was used for organic and element carbon analyses, respectively. Each cartridge contains a sharp-cut PM2.5 impactor operating at a flow rate of 10 L/min (nylon and quartz) or 16.7 L/min (Teflon). In the cartridge containing the nylon filter for ion analysis, one (at ST2-5) or three honeycomb denuders (at ST1) coated with 1% citric acid in ethanol were used at upstream of the filter to remove gaseous NH3. The internal surface area of the denuder was 508 cm2. The denuder collection efficiency was close to 99.9% at low NH3 concentrations and short sampling times, and its capacity was about 1.2 mg NH3 ((Li and Wang, 2012). The absorbed NH3 concentrations of the cleaned solutions were analyzed using ion chromatography (Metrohm Ltd, Switzerland). Commercially purchased nylon filters were washed and dried prior to sampling to minimize background sodium and other common ions. Since nylon filters have high efficiency of taking up gaseous nitric and hydrochloric acids (Anlauf et al., Citation1985; Sturges and Harrison, Citation1989; Masia et al., Citation1994; Mehlmann and Warneck, Citation1995), measurements of nitrate and chloride from nylon filter samples were considered to be the total nitrate and chloride. Also due to the fact that the vapor pressure of H2SO4 is very low and it is most likely in a condensed phase (Markovic et al., Citation2011), measurements of aerosol SO42− of nylon filter samples were considered to be a good proxy for total H2SO4.
PM2.5 sample analysis
The PM2.5 sample analyses were conducted by the collaborators at RTI International in Research Triangle Park, NC. The analytical methods were based on standard operation procedures (SOPs) for the EPA National PM2.5 Chemical Speciation Network (EPA, 2009). All filters were stored in a 4°C container and delivered to the RTI lab on the sampling day. Nylon filters were extracted for anion and cations analysis. First the nylon filters were placed in polypropylene centrifuge tubes with 25.0 mL deionized water in each tube. Then the batch of tubes was ultrasonicated for 60 min (bath temperature was less than 27ºC), followed by mechanical shaking overnight in a cold room (<4ºC). Finally, the extracts were filtered through nylon membranes (0.45 μm pore diameter) and transferred to vials for chemical analysis by ion chromatography (IC). In this process, NH4+, Na+, and K+ were analyzed by IC with a chemical suppressor, and the eluent was 11 mmol/L sulfuric acid (H2SO4) at a flow rate of 1 mL/min. SO42−, Cl−, and NO3− were analyzed by IC with a chemical suppressor, and the eluent was 0.3 mmol/L NaHCO3/2.7 mmol/L Na2CO3 at a flow rate of 1.0 mL/min.
Data validation and analysis
In order to detect and correct (or remove) inaccurate data from field measurements, the data set was validated in following steps. Before sampling, computer-generated bar-code labels were used for each of the filters, which allowed unambiguous identification of each filter and the analytical data derived from that filter. Cross-checks by date, by site locations, and between filter types were conducted to ensure consistency. Invalid or questionable filters due to inadequate flow rates and other field-related problems were recorded and removed before filter analysis. The trimmed standard deviation (SD) and trimmed mean, which are obtained by taking SD and mean of the 5% to 95% range, were applied to decide some potential outliers (Cody, Citation2008). When a data point was beyond three trimmed standard deviations, a laboratory investigation was conducted to seek an assignable cause. If no root cause was determined, and a retest was justified, the potential outlier was recorded for future evaluation.
Data analysis for this study was conducted using SAS/STAT software, version 9.2 (SAS Institute Inc., Cary, NC). The analysis of variance (ANOVA) procedure was applied to perform analysis of variance and Tukey’s studentized range test was performed to test on all means.
Results and Discussion
Data validation
shows the boxplots (also known as box-and-whisker plots) of mass concentrations for NH4+ with outliers and without outliers. The plots for Na+, K+, SO42−, Cl−, and NO3− had similar patterns and are not shown here. As shown in , some potential outliers are located far away from the central of the group data values. If the observations were more than 3SD, then these suspicious observations were checked from field sampling, transportation, laboratory analysis, and data input. The root cause was normally filter contamination and analysis process errors.
Figure 2. Comparison of NH4+ concentration: with outliers vs. validated. The bottom and the top of the box are the 25th and 75th percentiles, the line near the middle of the box is the 50th percentile (median), and the circle is the mean. The whiskers represent the lowest datum with 1.5 interquartile ranges (IQR) of the lower quartile, and the highest datum with 1.5 IQR of the upper quartile. The small circle data points that are not included between the whiskers are potential outliers.
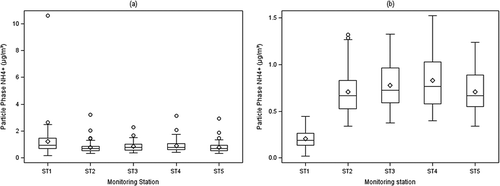
For comparison, another robust method devised by advocates of exploratory data analysis (EDA) was applied to look for outliers (Cody, Citation2008). An outlier was defined if the data point was above or below the whiskers. The lower whisker represents the lowest datum with 1.5 interquartile ranges (IQR) of the lower quartile, and the upper whisker represents the highest datum with 1.5 IQR of the upper quartile. This method is called a robust method because it is independent of the distribution of the data values. As shown in , the method based on trimmed SD and mean accomplished results very similar to those of the EDA method. There were only few points above the upper whisker (Q3 + 1.5IQR) and no points were below the lower whisker (Q1 – 1.5IQR).
Annual average ions concentrations
The total nitrate and chloride partitioning between gas and particle phases in ST2–ST5, and the particle-phase NH4+ uncertainty due to denuder breakthrough in ST1, were simulated by the equilibrium model ISORROPIA (Li et al., Citation2014). The descriptive statistics of ion concentrations of all PM2.5 samples taken from five stations across four seasons are presented in . The descriptive statistics include numbers of observations (n), mean, SD, median, minimum (min), and maximum (max). At ST1, the mostly abundant ions were SO42−, Cl−, and K+, and they were most likely from chicken feed additive and manure, which were consistent with the Yang et al. (Citation2011) results from layer hen facilities. However, at ST2–ST5, SO42−, and NH4+ were the two most abundant ions, and their concentrations were significantly higher than for Na+, K+, and Cl−. For comparison of the mean ion concentrations among five sampling stations, the ANOVA of Tukey’s studentized range (HSD) test results are also shown in . ST1 NH4+ concentrations were significantly lower than ambient NH4+, which indicates that most of PM2.5 at ST1 were primary PM generated from feed, hen bodies, and manure (Li et al., Citation2013). Limited acid compounds in the particle phase resulted in less NH3 partitioning to the particle phase and as a consequence low NH4+ concentrations at ST1. The range of ambient NH4+ concentrations in this study was higher than the range of concentrations (0.13-0.36 μg/m3) measured at seven Clean Air Status and Trends Network (CASTNet) sites in North Carolina in 2009, which may be because the seven CASTNet sites were not located in animal agriculture-intensive areas. Also these sites had different air transport, meteorological conditions, and other precursor conditions (CASTNet, Citation2012). As observed, ST1 (Tukey’s group A) had significantly higher Na+, K+, SO42−, and Cl− concentrations than all other stations (Tukey’s group B), which may be because of feed additive contributions to PM.
Table 1. Annual average concentrations of ions (NH4+, Na+, K+, SO42−, Cl−, and NO3−) at ST1–ST5
The ion (NH4+, Na+, K+, SO42−, Cl−, and NO3−) mass percentages (total major ions mass/total PM2.5 mass) at ST1–ST5 are 10%, 36%, 40%, 41%, and 38%, respectively. The very low ion mass percentages in ST1 indicate a considerable part of PM2.5 mass was organic instead of inorganic and possibly originated from feed and animal bodies (Li et al., Citation2013). Inside the animal houses, gas-phase NH3 had relatively high concentrations (several to 100 ppm), but acidic gases (NOx, SOx) were unlikely to be in high concentrations to react with NH3 to form secondary inorganic aerosols. Moreover, the short residence time (ranged from 1.2 to 11 min) of ventilation air in the house was likely another reason for limited inorganic ammonium aerosol formation. The mass percentage of ions were similar at the 4 ambient stations, and the estimates of the sum contribution of SO42−, NO3−, and NH4+ to total PM2.5 mass were slightly lower than for previous studies at 36 stations located mostly in the western United States and 4 four sites (Rubidoux, CA, Phoenix, AZ, Philadelphia, PA, and Research Triangle Park, NC) (Malm et al., Citation1994; Tolocka et al., Citation2001). In the ambient PM sampling stations, there were no major industrial sources (the nearest coal-fired power station with capacity of 115 MW was 20 km away in southeast) and highway traffic sources (one major highway was 12.7 km away in southeast). The relative low-level precursor gases (NOx and SO2) as emissions from local sources might be one of the reasons for the relatively low inorganic aerosol portion. High geological material and organic matter in agriculture areas could also result in a relatively low ions mass portion.
Seasonal variations
For analysis of seasonal variability, the period of study was grouped into four astronomical seasons (spring: March 20–June 19, summer: June 20–September 19, autumn: September 20–December 20, and winter: December 21–March 19) (Li et al., Citation2011). Since the ion means of four ambient stations were not significantly different, these four ambient stations were combined together to increase sample size. shows boxplots of concentrations of NH4+, Na+, K+, SO42−, NO3−, and Cl− of PM2.5 samples at ST1 and ambient stations (the average of ST2–ST5). At ST1, summer and autumn NH4+ concentrations were slightly higher than in spring and winter, but not statistically significant at P = 0.05 level. Inside the layer house, NH3 concentrations had a very strong seasonal variation, resulting from interaction of NH3 generation from poultry manure and the house ventilation. Winter had much higher in-house NH3 concentrations than summer (Wang-Li et al., 2013). The small seasonal variation in NH4+ indicates that gas-phase NH3 was not the only major force driving its gas–particle partitioning. For Na+, K+, and Cl− at ST1, cold seasons (spring/winter) had significantly higher concentrations than warm seasons (autumn/summer), which were due to high ventilation rates in warm seasons and Na+, K+, and Cl− concentrations were diluted. The in-house concentrations of NO3− and SO42− did not show strong seasonal variations in this study. For the ambient stations, NH4+ had the highest concentrations in summer, which was similar to gas-phase NH3 (Li et al., Citation2014) and the seasonal patterns of NH4+ observed by other researchers in other agricultural-intensive sites (Walker et al., Citation2000; Robarge et al., Citation2002). Ambient NO3− had the highest concentration in winter, because the lower temperature and stable meteorology were in favor of the formation of ammonium nitrate (Park et al., Citation2005; Mariani and de Mello, Citation2007). Ambient K+ had higher concentrations in cold seasons than that in warm seasons, which may be due to the seasonal variation of wood burning in residence areas in the region. Wood (biomass) burning is a primary source of K+ (Chow, Citation1995; Lanz et al., Citation2008). The higher ambient SO42− was found in summer and spring, which may be due to the increased photochemical activity (Husain and Dutkiewicz, Citation1990).
Correlation analysis and molar ratio of NH4+/SO42−
The correlation coefficients between anions and cations of PM2.5 in house (ST1) and ambient stations (ST2-ST5) are shown in . In the house, K+, Na+, and Cl− were highly correlated with each other. These correlations indicate a possible common source for them, and they probably came from animal feed, as they were normally added as animal feed amendment. The relative weak correlations between NH4+ and SO42− /Cl−/NO3− at ST1 indicate that they had different sources or formation processes. In addition to atmospheric H2SO4, HNO3, and HCl, SO42−, NO3−, and Cl− at ST1 may come from feeds or feces. However, at ambient stations (ST2–ST5), SO42− and NH4+ were highly correlated; also, NH4+ and NO3− showed strong correlation, which indicates some formation of secondary inorganic aerosols in the atmosphere or a consequence of air transport. There was a strong correlation between Na+ and Cl−, and no significant correlation between K+ and Cl−. The strong correlation between Na+ and Cl− indicated they both might come from feed additives, or a consequence of marine aerosol transport (Atlantic Ocean, 240 km away). However, K+ is a tracer of biomass burning and it is not directly connected with Cl− (Chow, Citation1995; Lanz et al., Citation2008).
2. Pearson correlation coefficients between anions and cations of PM2.5 taken at in-house station (ST1) and all ambient stations (ST2–ST5)
Ambient PM2.5 ammonium was mostly contributed from NH4NO3, (NH4)2SO4, NH4HSO4, (NH4)3H(SO4)2, and NH4Cl. To estimate the NH4+ associated with SO42−, the molar ratios of (NH4+)s/SO42− were calculated by subtracting NH4+ from NO3− and Cl− (Walker et al., Citation2006). shows the distribution of the (NH4+)s/SO42− molar ratios at ambient stations with a mean in 1.75. Given the relatively high concentration of NH3 around the egg production facility, if gas-phase NH3 was in stable equilibrium with sulfate aerosol, then the ratio should be close to 2. The low (NH4+)s/SO42− molar ratios were also observed by other studies in other agriculture areas (Baek and Aneja, Citation2004; Walker et al., Citation2006). Wexler and Seinfeld (Citation1990) found that ammonium salts in gas–aerosol phases were not always in stable equilibrium, especially under low aerosol loading and cooler conditions. Quinn et al. (Citation1992) reported that the times needed for NH3 aerosol to reach stable equilibrium vary from 0.3 to 7 hr. The average wind speed at this site was 1.52 m/sec with SD = 0.97 m/sec. The time scale of establishment of local NH3-aerosol stable equilibrium might exceed the time scale of air parcel transport with sufficient NH3. This hypothesis was tested by examining the (NH4+)s/SO42− molar ratios under different wind ranges. The (NH4+)s/SO42− molar ratios were 1.83 and 1.73 for wind speed ≤0.70 m/sec and >0.70 m/sec, respectively, which indicated the time scale likely had an impact on the local NH3–aerosol equilibrium. Another possible reason was wind-blown fugitive dust containing sulfate minerals.
Meteorological parameters
Ambient temperature, relative humidity (RH), the U component (zonal) and the V component (meridional) of the wind, and solar radiation were key parameters affecting the origin of particles. The means of temperature, RH, the U component and the V component of the wind, and solar radiation were 16.9ºC, 67%, 0.43 m/sec, –0.71 m/sec, and 146 w/m2, respectively. The correlation between ions and these parameters is presented in . The results show that the meteorological parameters of temperature and solar radiation were positively correlated with NH4+ and SO42−. The temperature and solar radiation could enhance formation of secondary inorganic ammonium sulfate. The relationships between ions and U component of wind indicated that the U component of wind had a positive potential role to enhance the levels of NH4+, Na, SO42−, and Cl−. The negative correlation of temperature with NO3− could be attributed to thermal decomposition of NH4NO3 (Galindo et al., Citation2008).
3. Spearman correlation coefficients among ions and meteorological parameters
Conclusion
This study investigated the major ions of PM2.5 emitted from a commercial layer farm and in its vicinity in North Carolina. In the house, the mostly abundant ions were SO42−, Cl−, and K+, and they were most likely from hen feed additive. At ambient stations, SO42−, and NH4+ were the two most abundant ions, and their concentrations were significantly higher than for Na+, K+, and Cl−. The total ion mass percentages (i.e., total mass of NH4+, Na+, K+, SO42−, Cl, and K+ over PM2.5 mass) at five sampling stations (i.e., ST1-ST5) are 10%, 36%, 40%, 41%, and 38%, respectively. In the house, summer and autumn NH4+ concentrations were slightly higher than for spring and winter, but not statistically significant at the P = 0.05 level. The small seasonal variation in NH4+ indicates that gas-phase NH3 was not the only major force driving its gas–particle partitioning. For the ambient stations, NH4+ had the highest concentrations in summer. In the house, K+, Na+, and Cl− were highly correlated with each other. At ambient stations, SO42− and NH4+ were highly correlated, and NH4+ and NO3− also showed strong correlation, which indicates formation of secondary inorganic aerosol in atmosphere. The mean molar ratio of (NH4+)s/SO42− at ambient stations was less than 2, which indicates gas-phase NH3 was not 100% in equilibrium with sulfate to form (NH4)2SO4 due to the short equilibrium time. At ambient stations, meteorological parameters showed strong impact on the origin of particles. Overall, knowledge gained from this study, with additional consideration of NH3 concentrations and emissions, will lead to better understanding of PM2.5 source and formation, fate and transport, and their atmospheric dynamics.
Acknowledgment
Dr. John T. Walker from EPA reviewed the article and provided insight technical advice and inputs. The authors thankfully acknowledge his peer review.
Funding
This study was supported in part by an NSF-CAREER Award (CBET-0954673) and a National Research Initiative Competitive Grant, 2008-35112-18757, from the U.S. Department of Agriculture (USDA) Cooperative State Research, Education, and Extension Service Air Quality Program. The measurements of wind parameters were conducted under the National Air Emission Monitoring Study (NAEMS) Southeast Layer Operation, funded by the American Egg Board. The authors acknowledge the generous support and collaboration of the egg producer.
Additional information
Notes on contributors
Qian-Feng Li
Qian-Feng Li is a research associate in the Department of Animal Science, Biosystems and Agricultural Engineering, Michigan State University, East Lansing.
Lingjuan Wang-Li
Lingjuan Wang-Li and Sanjay B. Shah are associate professors in the Department of Biological and Agricultural Engineering, North Carolina State University, Raleigh, NC.
Zifei Liu
Zifei Liu is an assistant professor in the Department of Biological and Agricultural Engineering, Kansas State University, Manhattan, KS.
R.K.M. Jayanty
R.K.M. Jayanty is a senior fellow at RTI International, Research Triangle Park, NC.
Sanjay B. Shah
Lingjuan Wang-Li and Sanjay B. Shah are associate professors in the Department of Biological and Agricultural Engineering, North Carolina State University, Raleigh, NC.
Peter Bloomfield
Peter Bloomfield is a professor in the Department of Statistics, North Carolina State University, Raleigh, NC.
References
- Anlauf, K.G., P. Fellin, H.A. Wiebe, H.I. Schiff, G.I. Mackay, R.S. Braman, and R. Gilbert. 1985. A comparison of three methods for measurement of atmospheric nitric acid and aerosol nitrate and ammonium. Atmos. Environ. 19(2): 325–33. doi:10.1016/0004-6981(85)90100-3
- Baek, B.H., and V.P. Aneja. 2004. Measurement and analysis of the relationship between ammonia, acid gases, and fine particles in eastern North Carolina. J. Air Waste Manage. Assoc. 54(5): 623–33. doi:10.1080/10473289.2004.10470933
- CASTNet. 2012. The clean air status and trends network. http://epa.gov/castnet/javaweb/docs/annual_report_2009.pdf ( accessed March 2, 2011).
- Chow, J.C. 1995. Critical review: Measurement methods to determine compliance of ambient air quality standards for the suspended particles. J. Air Waste Manage. Assoc. 45:320–85. doi:10.1080/10473289.1995.10467369
- Cody, R. 2008. Cody’s data cleaning techniques using SAS, 2nd ed. Cary, NC: SAS Institute, Inc.
- Galindo, N., J. F. Nicolás, E. Yubero, S. Caballero, C. Pastor, and J. Crespo. 2008. Factors affecting levels of aerosol sulfate and nitrate on the western Mediterranean coast. Atmos. Res. 88(3–4):305–13. doi:10.1016/j.atmosres.2007.11.024
- Hinds, W.C. 1998. Aerosol technology: Properties, behavior, and measurement of airborne particles. New York, NY: John Wiley & Sons.
- Husain, L., and V.A. Dutkiewicz. 1990. A long-term (1975–1988) study of atmospheric SO42−: Regional contributions and concentration trends. Atmos. Environ. A Gen. Topics 24(5): 1175–87. doi:1016/0960-1686(90)90082-X
- Kerminen, V.M., R. Hillamo, K. Teinila, T. Pakkanen, I. Allegrini, and R. Sparapani. 2001. Ion balances of size-resolved tropospheric aerosol samples: implications for the acidity and atmospheric processing of aerosols. Atmos. Environ. 35(31): 5255–65. doi:10.1016/S1352-2310(01)00345-4
- Lammel, G., F. Schneider, E. Brüggemann, T. Gnauk, A. Röhrl, and P. Wieser. 2004. Aerosols emitted from a livestock farm in southern Germany. Water Air Soil Pollut. 154:313–30. doi:10.1023/B:WATE.0000022962.65942.4b
- Lanz, V.A., M.R. Alfarra, U. Baltensperger, B. Buchmann, C. Hueglin, S. Szidat, M.N. Wehrli, L. Wacker, S. Weimer, A. Caseiro, H. Puxbaum, and A.S. Prevot. 2008. Source attribution of submicron organic aerosols during wintertime inversions by advanced factor analysis of aerosol mass spectra. Environ. Sci. Technol. 42(1): 214–20. doi:10.1021/es0707207
- Li, Q.-F., L. Wang-Li, S.B. Shah, R.K.M. Jayanty, and P. Bloomfield. 2011. Fine particulate matter in a high-rise layer house and its vicinity. Trans. ASABE 54(6): 2299–310.
- Li, Q.-F., L. Wang-Li, K. Wang, L. Chai, E.L. Cortun, I. Kilic, B.W. Bogan, J.-Q. Ni, and A.J. Heber. 2013. National air emissions monitoring study’s Southeast layer site: Part II. particulate matter. Trans. ASABE. 56(3): 1173–84.
- Li, Q.F., L. Wang-Li, R.K.M. Jayanty, and S.B. Shah. 2013 Organic and elemental carbon in atmospheric fine particulate matter in an animal agriculture intensive area in North Carolina: Estimation of secondary organic carbon concentrations. Open J. Air Pollut. 2:7–18. doi:10.4236/ojap.2013.21002
- Li, Q.F., L. Wang-Li, S.B. Shah, R.K. Jayanty, and P. Bloomfield. 2014. Ammonia concentrations and modeling of inorganic particulate matter in the vicinity of an egg production facility in southeastern USA. Environ. Sci. Pollut. Res. Int. 21(6): 4675–85. doi:10.1007/s11356-013-2417-z
- Malm, W.C., J.F. Sisler, D. Huffman, R.A. Eldred, and T.A. Cahill. 1994. Spatial and seasonal trends in particle concentration and optical extinction in the United States. J. Geophys. Res. Atmos. 99(D1): 1347–70. doi:10.1029/93JD02916
- Mariani, R.L., and W.Z. de Mello. 2007. PM2.5-10, PM2.5 and associated water-soluble inorganic species at a coastal urban site in the metropolitan region of Rio de Janeiro. Atmos. Environ. 41(13): 2887–92. doi:10.1016/j.atmosenv.2006.12.009
- Markovic, M.Z., K.L. Hayden, J.G. Murphy, P.A. Makar, R.A. Ellis, R.Y.W. Chang, J.G. Slowik, C. Mihele, and J. Brook. 2011. The effect of meteorological and chemical factors on the agreement between observations and predictions of fine aerosol composition in southwestern Ontario during BAQS-Met. Atmos. Chem. Phys. 11(7): 3195–210. doi:10.5194/acp-11-3195-2011
- Martin, R.S., P.J. Silva, K. Moore, M. Erupe, and V.S. Doshi. 2008. Particle composition and size distributions in and around a deep-pit swine operation, Ames, IA. J. Atmos. Chem. 59(2): 135–50. doi:10.1007/s10874-008-9097-y
- Masia, P., V. Dipalo, and M. Possanzini. 1994. Uptake of ammonia by nylon filters in filter pack systems. Atmos. Environ. 28(2): 365–66. doi:10.1016/1352-2310(94)90112-0
- Mehlmann, A., and P. Warneck. 1995. Atmospheric gaseous HNO3 particulate nitrate, and aerosol-size distributions of major ionic species at a rural site in western Germany. Atmos. Environ. 29(17): 2359–73. doi:10.1016/1352-2310(95)00056-5
- National Research Council. 2003. Air emissions from animal feeding operations: Current knowledge, future needs. Washington DC: National Academies Press.
- Park, S.S., J.M. Ondov, D. Harrison, and N.P. Nair. 2005. Seasonal and shorter-term variations in particulate atmospheric nitrate in Baltimore. Atmos. Environ. 39(11): 2011–20. doi:10.1016/j.atmosenv.2004.12.032
- Purdue University. 2006. National air emissions monitoring study. https://engineering.purdue.edu/˜odor/NAEMS/index.htm ( accessed June 18, 2014).
- Quinn, P.K., W.E. Asher, and R.J. Charlson. 1992. Equilibria of the marine multiphase ammonia system. J. Atmos. Chem. 14(1–4): 11–30. doi:10.1007/BF00115219
- Robarge, W.P., J.T. Walker, R.B. McCulloch, and G. Murray. 2002. Atmospheric concentrations of ammonia and ammonium at an agricultural site in the southeast United States. Atmos. Environ. 36(10): 1661–74. doi:10.1016/S1352-2310(02)00171-1
- Sturges, W.T., and R.M. Harrison. 1989. The use of nylon filters to collect HCl—Efficiencies, interferences and ambient concentrations. Atmos. Environ. 23(9): 1987–96. doi:10.1016/0004-6981(89)90525-8
- Tolocka, M.P., P.A. Solomon, W. Mitchell, G.A. Norris, D.B. Gemmill, R.W. Wiener, R.W. Vanderpool, J.B. Homolya, and J. Rice. 2001. East versus West in the US: Chemical characteristics of PM2.5 during the winter of 1999. Aerosol Sci. Technol. 34(1): 88–96. doi:10.1080/027868201300082067
- U.S. Environmental Protection Agency. 1997. Guidance for network design and optimum site exposure for PM2.5 and PM10. Research Triangle Park, NC. http://www.epa.gov/ttn/amtic/files/ambient/pm25/network/r-99-022.pdf ( accessed December 24, 2010).
- U.S. Environmental Protection Agency. 2009. PM 2.5 and chemical speciation: Laboratory standard operating procedures. Research Triangle Park, NC. http://www.epa.gov/ttn/amtic/specsop.html ( accessed December 7, 2010).
- Walker, J., D. Nelson, and V.P. Aneja. 2000. Trends in ammonium concentration in precipitation and atmospheric ammonia emissions at a coastal plain site in North Carolina, USA. Environ. Sci. Technol. 34(17): 3527–34. doi:10.1021/es990921d
- Walker, J.T., W.P. Robarge, A. Shendrikar, and H. Kimball. 2006. Inorganic PM2.5 at a US agricultural site. Environ. Pollut. 139(2): 258–71. doi:10.1016/j.envpol.2005.05.019
- Wang-Li, L., Q.-F. Li, K. Wang, B.W. Bogan, J.-Q. Ni, E.L. Cortus, and A.J. Heber. 2013a. National Air Emissions Monitoring Study’s Southeast Layer Site: Part I. Site characteristics and monitoring methodology. Trans. ASABE 56(3): 1157–71.
- Wang-Li, L., Q.F. Li, L. Chai, E.L. Cortus, K. Wang, I. Kilic, B.W. Bogan, J.Q. Ni, and A.J. Heber. 2013b. The National Air Emissions Monitoring Study’s Southeast Layer Site: Part III. Ammonia concentrations and emissions. Trans. ASABE 56(3): 1185–97.
- Wexler, A.S., and J.H. Seinfeld. 1990. The distribution of ammonium-salts among a size and composition dispersed aerosol. Atmos. Environ. Part A Gen. Topics 24(5): 1231–46. doi:10.1016/0960-1686(90)90088-5
- Yang, X.F., X.L. Wang, Y.H. Zhang, J.M. Lee, J.W. Su, and R.S. Gates. 2011. Characterization of trace elements and ions in PM10 and PM2.5 emitted from animal confinement buildings. Atmos. Environ. 45(39): 7096–104. doi:10.1016/j.atmosenv.2011.09.037