ABSTRACT
Sustainable management of hospital waste requires an active involvement of all key players. This study aims to test the hypothesis that three motivating factors, namely, Reputation, Liability, and Expense, influence hospital waste management. The survey for this study was conducted in two phases, with the pilot study used for exploratory factor analysis and the subsequent main survey used for cross-validation using confirmatory factor analysis. The hypotheses were validated through one-sample t tests. Correlations were established between the three motivating factors and organizational characteristics of hospital type, location, category, and size. The hypotheses were validated, and it was found that the factors of Liability and Expense varied considerably with respect to location and size of a hospital. The factor of Reputation, however, did not exhibit significant variation. In conclusion, concerns about the reputation of a facility and an apprehension of liability act as incentives for sound hospital waste management, whereas concerns about financial costs and perceived overburden on staff act as disincentives.
Implications: This paper identifies the non economic motivating factors that can be used to encourage behavioral changes regarding waste management at hospitals in resource constrained environments. This study discovered that organizational characteristics such as hospital size and location cause the responses to vary among the subjects. Hence a policy maker must take into account the institutional setting before introducing a change geared towards better waste management outcomes across hospitals. This study covers a topic that has hitherto been neglected in resource constrained countries. Thus it can be used as one of the first steps to highlight and tackle the issue.
Introduction
Management of healthcare waste is a critical concern for healthcare facilities. According to World Health Organization (WHO), around 75–90% of the waste generated across healthcare facilities can be considered as nonhazardous; it’s the remaining 10–25% that cannot be ignored (Yves Chartier et al., Citation2013). Many developed countries enforce strict guidelines regarding healthcare waste segregation, storage, and transportation (Marinkovic et al., Citation2008). Developing countries, on the other hand, are found to be resource constrained when it comes to effective healthcare waste management (HWM) (Caniato et al., Citation2015). Here, poor sanitation practices might result in the mixing of hazardous waste with general waste, which may exacerbate the problem of waste management. It is important to identify and evaluate the key factors that can motivate and encourage sound HWM. It is especially important to conduct a behavioral study to establish significant influencers of HWM in a resource-constrained environment where the usual economic incentives are difficult to realize. Existing literature on the topic of HWM mainly consists of estimations of waste compositions (Komilis et al., Citation2012; Tesfahun et al., Citation2014) or studies regarding different waste management systems and technologies (Askarian et al., Citation2010; Salkin, Citation2003). Studies on the operational and managerial aspects of the HWM focus on knowledge and awareness of the hospital staff regarding rules and standard operating procedures (Jabbari et al., Citation2012; Lakbala and Lakbala, Citation2013). Studies identifying the noneconomic motivators of HWM are rare in the scientific literature. It is important to identify them because these can be used by managers and policy makers for a behavioral change when monetary incentives are unavailable. Although the exact policy to bring about this change may vary from one case to another, it is important to outline the significant factors that may instigate such a change. This paper attempts to fill the gap in the existing literature by determining the factors that can motivate healthcare staff to adopt sound HWM practices. For the present paper, we have presumed that certain social factors may lead healthcare facilities to adopt sound waste management controls. These factors can be punitive, such as legal and financial penalties, or they can be rewarding, such as improved public perception and reputation. We also want to evaluate the differences in relative importance of such factors across healthcare facilities. Hence, we will assess the impact of the organizational characteristics of a healthcare facility on the adoption of such controls. Since our focus is on external social processes, we will avoid investigating motivating factors such as disease prevention and occupational safety, as these features act as core values of a healthcare facility and hence are intrinsic to the organization’s philosophy.
Materials and methods
There exist numerous studies on social reasons and motivators behind stakeholders’ participation in municipal solid waste management (SWM). Studies on personnel in specialized institutions and organizations, however, are relatively scarce. Hence, the indicators to measure positive and negative incentives for sound HWM were developed using existing literature on SWM. Necessary modifications were made to reflect the specialized nature of the study. Existing studies on SWM usually focus on economic incentive as the main motivating factor (Cossu and Masi, Citation2013; Krystofik et al., Citation2015). However, it is difficult to employ economic incentives here because our focus is on resource-constrained environments. Most of the other studies usually focus on reputation (Hoejmose et al., Citation2014; Nguyen and Shi, Citation2012), liability concerns (Ogishi et al., Citation2003; Wilson et al., Citation2009), and the costs (Martinez-Sanchez et al., Citation2015; Parthan et al., Citation2012; Soares et al., Citation2013) associated with effective waste management. We will also focus on these three aspects in this paper. Thus, for the survey, we hypothesize that a facility’s decision to adopt HWM practices is positively associated with the perception that
Adoption of sound HWM will result in improved reputation of the facility.
The facility would not want to have any liability accruing from a lag in HWM.
and negatively associated with the perception that
Adoption of sound HWM will result in increased burden on the facility.
For the case study, we surveyed healthcare facilities in Gujranwala, a major city of Pakistan. Pakistan is a resource-constrained, middle-income country in South Asia, and Gujranwala is one of its major cities. Gujranwala has a population of 4.7 million, and currently it is ranked as one of the fastest growing cities in South Asia (Mayors, Citation2011). The collection and analysis of the data was done in two phases: (i) the pilot study (Keikelame et al., Citation2012) and (ii) the main survey. The pilot study was meant to check the construct validity and reliability (Chen et al., Citation2012a; Johnson et al., Citation2015; Mehta et al., Citation2015). Furthermore, it was used for rigorous analysis of the theoretical and dimensional nature of the construct (Vilca and Vallejos, Citation2015) . It was also meant to remove the redundant items from the questionnaire (Kakurina et al., Citation2015; Williams et al., Citation2013) and to alleviate concerns regarding common method bias (CMB). The pilot study was conducted through a series of in-depth, face-to-face interviews with the nursing staff at the District Head Quarter hospital (DHQ) through the month of November 2014. DHQ was chosen because it is the biggest and the only teaching hospital in the city. We chose nursing staff as the subjects because they serve as intermediaries between the patients (waste generators) and the sanitary staff (waste handlers) in a healthcare facility. Hence, they can significantly influence behavior regarding HWM. The questionnaire was delivered to a sample of trainee and regular nurses at DHQ, and the results were used for dimension reduction. The main survey was used to test the model based on responses from a wide array of subjects. It was carried out across 11 hospitals in the city. The hospitals were chosen based on stratified random sampling, viz., bed size (large: >100, medium: 50–100, and small: <50) and location in the four urban townships in the city. summarizes the scope of the study. It is pertinent to mention that at DHQ, only those nurses who had not participated in the pilot study were considered for the main survey.
Table 1. Hospitals covered for the main survey.
An introductory letter from the university was provided in each case, and informed consent was taken from the hospital manager as well as the person filling the questionnaire in a verbal format. The survey was only conducted after relevant permissions were granted from the concerned board of directors/owners/managers at each hospital. No health-related or personal questions were asked from the participants. Presently, an institutional review board (IRB) exists for only clinical research at the authors’ institutions and no IRB exists for studies related to the present subject. Hence, in the absence of an IRB, relevant ethical approvals were taken from university professors supervising the study. The names of the hospitals or their staff members have not been mentioned in this paper to protect their identities.
A total of 101 filled questionnaires were returned from the pilot study. Of these, 95 were retained after rejecting incomplete responses. The data were subjected to testing by exploratory factor analysis (EFA) using SPSS 21 (IBM, Armonk, NY, USA). The instrument to measure the responses consisted of 20 indicators measured on a 10-point Likert scale. Thus, an observation-to-variable ratio of almost 5:1 was achieved. The scale was developed in light of Hinkin’s review of scale development practices (Hinkin, Citation1995). Moreover, a larger scale was chosen because it offers more variance than a 5-point or 7-point scale, offers a high degree of measurement precision, provides more opportunity to detect changes, and provides more power to explain a point of view (Gunderman and Chan, Citation2013; Bayer, 2003). Since all the respondents belonged to a teaching hospital, the limitations of respondents’ lack of knowledge and awareness did not hold true. In order to check for CMB, both the Harman’s single factor (HSF) and common latent factor (CLF) procedures (MacKenzie and Podsakoff, Citation2012; Viswanathan and Kayande, Citation2012) were used in SPSS 21 and AMOS 17 (SPSS, Chicago, IL, USA), respectively. For the main survey, a sample size of 244 was obtained out of a total population of 382 nurses in the subject hospitals. This is greater than a minimum required sample size at 99% confidence level and 5% confidence interval. The responses were divided equally, with half of the observations tested under EFA using SPSS 21 and the remaining half tested under confirmatory factor analysis (CFA) using AMOS 17 (Chen et al., Citation2012b, 2015; Schaub and Stieglitz, Citation2000). Hence, in both cases, an observation-to-variable ratio of 12:1 was achieved. The instrument to measure the responses for the main survey was simplified to cater to the needs of a much broader spectrum of nurses across different hospitals. It consisted of a 5-point Likert scale varying from “strongly disagree” to “strongly agree.” For the main survey, CMB was again tested by using the CLF procedure.
Results and discussion
The face validity of the construct for the pilot study was determined through consultation with university academics who are experts in this field (Bright et al., Citation2012; Hardesty and Bearden, Citation2004; Jalink et al., Citation2015). Since the construct was supposed to have at least three dimensions, a reliability test based on Cronbach’s alpha was not employed in this case (Fisher and King, Citation2010). Rather, observations from the pilot study were analyzed using EFA to explore if the presumed three-dimensional model was tangible. Multicollinearity for the data was checked using variable correlation matrix. Since determinant of the matrix was 0.01 (>0.00001) and most of the correlations were greater than 0.05, multicollinearity was not a problem. Sample adequacy was checked using Kaiser-Meyer-Olkin (KMO) test. The KMO value came out to be 0.677, which is greater than the minimum requirement of 0.5. The P value for the Bartlett’s test was less than 0.001; hence, the null hypothesis regarding the correlation matrix being an identity matrix was rejected. A total of six factors, with eigenvalues >1, were extracted based on principal component analysis (PCA). The extracted factors represented 63% of the variance in the model. The factors were rotated using varimax to have a simple structure (Pritchard, Citation1984) with minimum cross-loadings. Since the sample size was small, we retained only those variables that had factor loadings above 0.5 (Manhenke et al., Citation2013). For cross-loading items, a cutoff value of around 0.2 (Anderson et al., Citation2015) was used. Only those factors that met those conditions and made sense together were retained. This left us with three factors having nine variables. The results are given in , with loadings of the selected variables highlighted in bold text. Note that here indicators 1, 2, 3, 11, 15, 16, and 17 were used to measure the dimension of Reputation. Similarly, indicators 4, 5, 6, 13, and 14 were used to measure the dimension of Liability. Indicators 7, 8, 9, 10, 12, 18, 19, and 20 were used to measure the dimension of Expense. A greater number of indicators were used for Expense because in this case we used measures related to financial costs as well as work burden on the staff.
Table 2. Pilot study: Exploratory factor analysis—Rotated component matrix.
For the CMB, the variances calculated by HSF and CLF procedures came out to be 22.496% and 24.01%, respectively. This is less than a cutoff value of 50% required for CMB to exist. Hence, variance caused by CMB did not have any significant effect here.
The questionnaire for the main survey consisted of five options on a Likert-type scale against each statement. The questionnaire was offered to the nursing staff at 11 different hospitals including DHQ, and eventually 244 complete responses were obtained. The results were split equally, and half of the observations were tested under EFA using SPSS 21. The results are given in . Note that the indicators 2, 3, and 8 given in above are grouped under one factor, indicators 5 and 6 are represented under the second factor, and finally, indicators 7, 10, and 18 are represented under the third factor. Hence, the results of EFA in the main survey are almost the same as that obtained from the pilot study. The only exception is that indicator 11 given in above now cross-loads on two factors. In the pilot study, it loaded on only one factor.
Table 3. Main study: Exploratory factor analysis—Rotated component matrix.
For the remaining observations, CFA was employed to identify the hypothesized underlying factor structure. Although EFA only assigns variables/indicators to different factors, CFA tests the assumption that the relationship within and among the factors and the variables can be expressed in the form of an equation. Hence, each variable is accompanied by an error term to express the variance unaccounted for by the factor. The final structure, given in ,shows that the minimum conditions were achieved (χ2 = 19.28, df = 20, P = 0.313 [>0.05]). Note that the indicators given in above have been abbreviated (see ), so that indicator 2 is represented as Patients_Perception, indicator 3 is represented as Govt_Regulation, and indicator 8 is represented as Staff_Morale. They are represented under the factor of Reputation. Similarly, indicators 5 is represented as Facility_Can_Be_Closed and indicator 6 is represented as Facility_Can_Be_Sued. Both of these indicators are represented under the factor of Liability. Finally, indicator 7 is represented as Burden_On_Staff, indicator 10 is represented as Financial_Burden, and indicator 18 is represented as Lack_Of_Funds. These three are represented under the factor of Expense. The value of adjusted minimum discrepancy, i.e., minimum value of discrepancy/degrees of freedom (CMIN/DF) should be between 1 and 2. For our model, the value was found to be 1.314. Moreover, the indicators used to measure goodness of fit, i.e., goodness-of-fit index (GFI) and adjusted GFI (AGFI), were 0.963 and 0.921, respectively. Ideally, these values should be greater than 0.95. Similarly, the values of comparative fit index (CFI) and Tucker-Lewis index (TLI) were found to be 0.989 and 0.982, respectively, which are also greater than the acceptable value of 0.95. Finally, the root mean square error of estimation (RMSEA) was found to be 0.033, which is significant (<0.05).
In order to check for CMB, once again CLF procedure was used for the results of the main survey. The resulting variance came out as 17.64%. Hence, CMB did not pose a threat to the model.
In the main survey the results were obtained on a Likert scale varying from 1 (strongly disagree) to 5 (strongly agree), with 3 being neutral. Hence, we can restate our hypotheses as follows:
Hypothesis 1:
H0 = Mean value of all variables in Reputation is ≤3.
H1 = Mean value of all variables in Reputation is >3.
Hypothesis 2:
H0 = Mean value of all variables in Liability is ≤3.
H1 = Mean value of all variables in Liability is >3.
Hypothesis 3:
H0 = Mean value of all variables in Expense is <3.
H1 = Mean value of all variables in Expense is ≥3.
According to the central limit theorem, for large samples, t distributions assume the shape of z-distributions. Hence, we use one-sample t tests in SPSS for testing our hypotheses. summarizes the results. The results show that all values are significant (P < 0.001) at the 99% confidence level. Thus, we reject the null hypotheses for Reputation, Liability, and Expense. Thus, we can say that reputation and liability concerns act as strong motivators for the implementation of sound HWM. Yet financial concerns and perceived overburden act as disincentives for the same. The results are shown in . Abbreviations have been used for the indicators, whereas their order of appearance is the same as given in .
Table 4. One-sample t test
Two-way chi-square tests of independence were performed to determine the influence of organizational characteristics of healthcare facility size, type, category, and location on the indicators. It was observed that all correlations were significant (P < 0.05) except for those between facility type and Patients_Perception and between facility location and Burden_On_Staff. shows the variation in responses for each hospital. It shows that Reputation and Liability are accorded the most importance in trust hospital T1 and the least importance in trust hospital T2. Liability receives the highest score in private hospital P5 and the least score in private hospital P6.
Figure 2. Subject response variation across the surveyed hospitals. Gov = government hospital; Pvt = private hospital; Med = medium-sized hospitals.
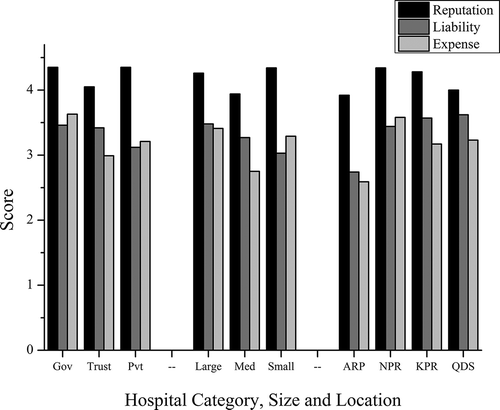
displays the variation in responses with the category, size, and location of the hospitals, respectively. It shows that the factors of Reputation and Expense received relatively lower scores in trust hospitals, whereas all the highest scores for all the three factors were recorded in the government hospital. Reputation and Expense were also relatively more important in private hospitals than in trust hospitals. Similarly, Reputation received greatest score in small hospitals, whereas Liability and Expense were accorded the highest scores in large hospitals. Reputation and Expense received lowest scores in medium hospitals, whereas Liability received the lowest score in small hospitals. Finally, Reputation and Expense received the highest scores across hospitals in the town of Nandipur. Liability received highest scores across hospitals in Qila Dedar Singh. All the three factors scored the lowest in Aroop Town.
Overall, opinions regarding Liability varied prominently within the dimensions of hospital location, whereas those regarding Expense varied prominently with hospital size and location. Reputation had relatively consistent responses.
Conclusion
In this paper, we set out to identify the factors that may influence behavior regarding sound HWM. We found that the incentives to adopt sound HWM practices include concerns about the reputation of a facility and an apprehension of liability accruing from poor HWM practices. On the other hand, concerns about financial costs and perceived overburden on staff act as disincentives for the implementation of sound HWM. We also wanted to analyze variation in the perceived importance of the identified factors with organizational characteristics such as hospital size and location. We found that whereas concerns about liability and financial burden vary considerably with respect to the location and size of a hospital, those regarding the reputation of a hospital are influenced to a relatively lesser extent.
Studies on motivation are used to understand the cognitive rationale behind a certain kind of behavior. For the present research, our purpose was to understand the noneconomic social influencers that may motivate better HWM practices among healthcare staff. This paper highlighted a hitherto neglected subject, and its findings can be used by managers and policy makers for a better implementation of HWM practices. This study can also be considered as one of the first steps in identifying and highlighting such factors. Many other studies across different time frames and different sets of respondents are required before a complete theory on this subject can be formed. Nevertheless, the methodology and results of this study can be used as a starting reference point for such future studies.
A limitation of this study is that the effect of demographic and social background of the respondents on the choice of responses has not been accounted for. Moreover, this study focused on hospitals only, and other facilities such as clinics and rural health centers were not included in the survey. In the future, a follow-up empirical study can be conducted to include such institutions. It is also important to determine practical measures required to influence a positive change in the current HWM practices. Multicriterion decision-making methods can be used to rank order such measures based on the factors identified in this study.
Acknowledgment
The authors would like to thank the respective managements at all participating hospitals for their kind support and cooperation.
Funding
The authors would like to acknowledge the support extended by China Specialized Research Fund for Doctoral Program of Higher Education (20120092110039), National Natural Science Foundation of China (71172044 and 71273047), and Major Program of National Social Science Foundation of China (12 and ZD207).
Additional information
Funding
Notes on contributors
Mustafa Ali
Mustafa Ali is a final year PhD student at the Southeast University, Nanjing, China.
Wenping Wang
Wenping Wang is a Professor of Management Science & Engineering at Southeast University, Nanjing, China.
Nawaz Chaudhry
Nawaz Chaudhry is Professor Emeritus at College of Earth and Environmental Sciences, University of the Punjab, Lahore, Pakistan.
References
- Anderson, A., M. Wilcox, A. Savitz, H. Chung, Q. Li, G. Salvadore, D. Wang, I. Nuamah, S.P. Riese, and R.M. Bilder. 2015. Sparse factors for the positive and negative syndrome scale: Which symptoms and stage of illness? Psychiatry Res. 225:283–290. doi:10.1016/j.psychres.2014.12.025
- Askarian, M., Heidarpoor, P., and Assadian, O. 2010. A total quality management approach to healthcare waste management in Namazi Hospital, Iran. Waste Manage. 30:2321–2326. doi:10.1016/j.wasman.2010.06.020
- Bright, E., Vine, S., Wilson, M.R., Masters, R.S.W., and McGrath, J.S. 2012. Face validity, construct validity and training benefits of a virtual reality turp simulator. Int. J. Surg. 10:163–166. doi:10.1016/j.ijsu.2012.02.012
- Caniato, M., Tudor, T., and Vaccari, M. 2015. International governance structures for health-care waste management: A systematic review of scientific literature. J Environ. Manage. 153:93–107. doi:10.1016/j.jenvman.2015.01.039
- Chen, D.-W., Chen, J.-W., Xu, W., Liu, W., Du, W.-J., and Li, H.-J. 2012a. A pilot study for reliability and validity of mini-physical performance test for Chinese male elders. Int. J. Gerontol. 6:16–19. doi:10.1016/j.ijge.2011.09.022
- Chen, P.-Y., Yang, C.-M., and Morin, C.M. 2015. Validating the cross-cultural factor structure and invariance property of the Insomnia Severity Index: Evidence based on ordinal EFA and CFA. Sleep Med. 16:598–603. doi:10.1016/j.sleep.2014.11.016
- Chen, S.-F., Wang, S., and Chen, C.-Y. 2012b. A simulation study using EFA and CFA programs based the impact of missing data on test dimensionality. Expert Syst. Appl. 39:4026–4031. doi:10.1016/j.eswa.2011.09.085
- Cossu, R., and Masi, S. 2013. Re-thinking incentives and penalties: Economic aspects of waste management in Italy. Waste Manage. 33:2541–2547. doi:10.1016/j.wasman.2013.04.011
- Fisher, M.J., and King, J. 2010. The Self-Directed Learning Readiness Scale for nursing education revisited: A confirmatory factor analysis. Nurse Educ. Today 30:44–48. doi:10.1016/j.nedt.2009.05.020
- Gunderman, R.B., and Chan, S. 2013. The 13-point Likert scale: A breakthrough in educational assessment. Acad. Radiol. 20:1466–1467. doi:10.1016/j.acra.2013.04.010
- Hardesty, D.M., and Bearden, W.O. 2004. The use of expert judges in scale development: Implications for improving face validity of measures of unobservable constructs. J. Bus. Res. 57:98–107. doi:10.1016/S0148-2963(01)00295-8
- Hinkin, T.R. 1995. A review of scale development practices in the study of organizations. J. Manage. 21:967–988. doi:10.1016/0149-2063(95)90050-0
- Hoejmose, S.U., Roehrich, J.K., and Grosvold, J. 2014. Is doing more doing better? The relationship between responsible supply chain management and corporate reputation. Ind. Market. Manage. 43:77–90. doi:10.1016/j.indmarman.2013.10.002
- Jabbari, H., Farokhfar, M., Jafarabadi, M.A., Taghipour, H., Bakhshian, F., and Rohane, S. 2012. Personal and organizational predictors of physicians’ knowledge, attitudes and practices related to medical waste management in Mazandaran province (northern Iran). Waste Manage. Res. 30:738–744. doi:10.1177/0734242X12440478
- Jalink, M.B., Goris, M.S.J., Heineman, E., Pierie, J.-P.E.N., and ten Cate Hoedemaker, H.O. 2015. Face validity of a Wii U video game for training basic laparoscopic skills. Am. J. Surg. 209(6):1102–1106. doi:10.1016/j.amjsurg.2014.09.034
- Johnson, L.B., Sumner, S., Duong, T., Yan, P., Bajcsy, R., Abresch, R.T., de Bie, E., and Han, J.J. 2015. Validity and reliability of smartphone magnetometer-based goniometer evaluation of shoulder abduction—A pilot study. Manual Ther. 20(6):777–782.
- Kakurina, N., Kadisa, A., Lejnieks, A., Mikazane, H., Kozireva, S., and Murovska, M. 2015. Use of exploratory factor analysis to ascertain the correlation between the activities of rheumatoid arthritis and infection by human parvovirus B19. Medicina 51:18–24. doi:10.1016/j.medici.2015.01.004
- Keikelame, M.J., Hills, R.M., Naidu, C., de Sá, A., and Zweigenthal, V. 2012. General practitioners’ perceptions on management of epilepsy in primary care settings in Cape Town, South Africa: An exploratory pilot study. Epilepsy Behav. 25:105–109. doi:10.1016/j.yebeh.2012.06.003
- Komilis, D., Fouki, A., and Papadopoulos, D. 2012. Hazardous medical waste generation rates of different categories of health-care facilities. Waste Manage. 32:1434–1441. doi:10.1016/j.wasman.2012.02.015
- Krystofik, M., Wagner, J., and Gaustad, G. 2015. Leveraging intellectual property rights to encourage green product design and remanufacturing for sustainable waste management. Resources, Conserv. Recycl. 97:44–54. doi:10.1016/j.resconrec.2015.02.005
- Lakbala, P., and Lakbala, M. 2013. Knowledge, attitude and practice of hospital staff management. Waste Manage. Res. 31:729–732. doi:10.1177/0734242X13484190
- MacKenzie, S.B., and Podsakoff, P.M. 2012. Common method bias in marketing: Causes, mechanisms, and procedural remedies. J. Retail. 88:542–555. doi:10.1016/j.jretai.2012.08.001
- Manhenke, C., Orn, S., von Haehling, S., Wollert, K.C., Ueland, T., Aukrust, P., Voors, A.A., Squire, I., Zannad, F., Anker, S.D., and Dickstein, K. 2013. Clustering of 37 circulating biomarkers by exploratory factor analysis in patients following complicated acute myocardial infarction. Int. J. Cardiol. 166:729–735. doi:10.1016/j.ijcard.2011.11.089
- Marinkovic, N., Vitale, K., Janev Holcer, N., Dzakula, A., and Pavic, T. 2008. Management of hazardous medical waste in Croatia. Waste Manage. 28:1049–1056. doi:10.1016/j.wasman.2007.01.021
- Martinez-Sanchez, V., Kromann, M.A., and Astrup, T.F. 2015. Life cycle costing of waste management systems: Overview, calculation principles and case studies. Waste Manage. 36:343–355. doi:10.1016/j.wasman.2014.10.033
- Mayors, C. 2011. The world’s fastest growing cities and urban areas from 2006 to 2020. http://www.citymayors.com/statistics/urban_growth1.html (accessed November 22, 2015).
- Mehta, S.P., MacDermid, J.C., Richardson, J., MacIntyre, N.J., and Grewal, R. 2015. Reliability and validity of selected measures associated with increased fall risk in females over the age of 45 years with distal radius fracture—A pilot study. J. Hand Ther. 28:2–10. doi:10.1016/j.jht.2014.09.009
- Nguyen, T., and Shi, W. 2012. Improving resource efficiency in data centers using reputation-based resource selection. Sustain. Comput. Informatics Syst. 2:138–150. doi:10.1016/j.suscom.2012.03.001
- Ogishi, A., Zilberman, D., and Metcalfe, M. 2003. Integrated agribusinesses and liability for animal waste. Environ. Sci. Policy 6:181–188. doi:10.1016/S1462-9011(03)00007-8
- Parthan, S.R., Milke, M.W., Wilson, D.C., and Cocks, J.H. 2012. Cost estimation for solid waste management in industrialising regions—Precedents, problems and prospects. Waste Manage. 32:584–594. doi:10.1016/j.wasman.2011.11.004
- Pritchard, W.S. 1984. PCAVR: A portable laboratory program for performing varimax-rotated principal components analysis of event-related potentials. Brain Res. Bull. 13:465–473. doi:10.1016/0361-9230(84)90099-6
- Salkin, I.F. 2003. Conventional and alternative technologies for the treatment of infectious waste. J. Mater. Cycles Waste Manage. 5(1):9–12. doi:10.1007/s101630300002
- Schaub, R.T., and Stieglitz, R.D. 2000. P01.129. Three syndromes of schizophrenia—Results of an EFA-CFA-crossvalidation approach. Eur. Psychiatry 15(Supp 2):s354.
- Soares, S.R., Finotti, A.R., Prudêncio da Silva, V., and Alvarenga, R.A.F. 2013. Applications of life cycle assessment and cost analysis in health care waste management. Waste Manage. 33: 175–183. doi:10.1016/j.wasman.2012.09.021
- Tesfahun, E., Kumie, A., Legesse, W., Kloos, H., and Beyene, A. 2014. Assessment of composition and generation rate of healthcare wastes in selected public and private hospitals of Ethiopia. Waste Manage. Res. 32:215–220. doi:10.1177/0734242X14521683
- Vilca, L.W., and Vallejos, M. 2015. Construction of the Risk of Addiction to Social Networks Scale (Cr.A.R.S.). Comput. Hum. Behav. 48:190–198. doi:10.1016/j.chb.2015.01.049
- Viswanathan, M., and Kayande, U. 2012. Commentary on “Common Method Bias in Marketing: Causes, Mechanisms, and Procedural Remedies”. J. Retail. 88:556–562. doi:10.1016/j.jretai.2012.10.002
- Williams, B., McKenna, L., French, J., and Dousek, S. 2013. The clinical teaching preference questionnaire (CTPQ): An exploratory factor analysis. Nurse Educ. Today 33:814–817. doi:10.1016/j.nedt.2012.02.017
- Wilson, E.J., Klass, A.B., and Bergan, S. 2009. Assessing a liability regime for carbon capture and storage. Energy Procedia 1:4575–4582. doi:10.1016/j.egypro.2009.02.277
- Wittink, D.R., L.R. Bayer. 1994. The measurement imperative. Marketing Research. 6(4):14.
- Yves Chartier, J.E., U. Pieper, A. Prüss, P. Rushbrook, R. Stringer, W. Townend, S. Wilburn, and R. Zghondi. 2013. Safe Management of Wastes from Health Care Activities, 2nd ed., Geneva, Switzerland: World Health Organization.