ABSTRACT
Exposure to ambient particulate matter (PM) is known as a significant risk factor for mortality and morbidity due to cardiorespiratory causes. Owing to increased interest in assessing personal and community exposures to PM, we evaluated the feasibility of employing a low-cost portable direct-reading instrument for measurement of ambient air PM exposure. A Dylos DC 1700 PM sensor was collocated with a Grimm 11-R in an urban residential area of Houston Texas. The 1-min averages of particle number concentrations for sizes between 0.5 and 2.5 µm (small size) and sizes larger than 2.5 µm (large size) from a DC 1700 were compared with the 1-min averages of PM2.5 (aerodynamic size less than 2.5 µm) and coarse PM (aerodynamic size between 2.5 and 10 µm) concentrations from a Grimm 11-R. We used a linear regression equation to convert DC 1700 number concentrations to mass concentrations, utilizing measurements from the Grimm 11-R. The estimated average DC 1700 PM2.5 concentration (13.2 ± 13.7 µg/m3) was similar to the average measured Grimm 11-R PM2.5 concentration (11.3 ± 15.1 µg/m3). The overall correlation (r2) for PM2.5 between the DC 1700 and Grimm 11-R was 0.778. The estimated average coarse PM concentration from the DC 1700 (5.6 ± 12.1 µg/m3) was also similar to that measured with the Grimm 11-R (4.8 ± 16.5 µg/m3) with an r2 of 0.481. The effects of relative humidity and particle size on the association between the DC 1700 and the Grimm 11-R results were also examined. The calculated PM mass concentrations from the DC 1700 were close to those measured with the Grimm 11-R when relative humidity was less than 60% for both PM2.5 and coarse PM. Particle size distribution was more important for the association of coarse PM between the DC 1700 and Grimm 11-R than it was for PM2.5.
Implications: The performance of a low-cost particulate matter (PM) sensor was evaluated in an urban residential area. Both PM2.5 and coarse PM (PM10-2.5) mass concentrations were estimated using a DC1700 PM sensor. The calculated PM mass concentrations from the number concentrations of DC 1700 were close to those measured with the Grimm 11-R when relative humidity was less than 60% for both PM2.5 and coarse PM. Particle size distribution was more important for the association of coarse PM between the DC 1700 and Grimm 11-R than it was for PM2.5.
Introduction
Inhalable particulate matter (PM10), with aerodynamic diameter equal to or less than 10 µm, can be classified into two categories: PM2.5 (particle size, i.e., aerodynamic diameter, equal to or less than 2.5 µm) and coarse PM or PM10-2.5 (particle size between 2.5 and 10 µm). It is known that exposure to ambient air PM2.5 and coarse PM is a risk factor for increased mortality and morbidity due to cardiovascular and respiratory disease (Adar et al., Citation2014; Peng et al., Citation2008; Pope and Dockery, Citation2006; Zanobetti et al., Citation2008). Because both PM2.5 and coarse PM can be deposited in the lower respiratory tract and lung cells, exposure to PM2.5 and coarse PM may play a role in adverse health effects (Adar et al., Citation2014; Krall et al., Citation2013; Thurston et al., Citation2016). Further, the International Agency for Research on Cancer (IARC) concluded that ambient PM2.5 and coarse PM are associated with an increase in cancer of the lung and urinary bladder (IARC, Citation2013).
Estimation of population exposures to air pollution is critical to examine the contribution of air pollution to disease burden. For exposure assessment, environmental health studies often evaluate community ambient air PM exposures using land-use regression models, dispersion models, or a hybrid approach of both, utilizing measured PM concentrations from the nearest air monitoring stations (Delfino et al., Citation2014; Eeftens et al., Citation2012; Jerrett et al., Citation2014; Wu et al., Citation2009; Wu et al., Citation2011). These methods generally assume that levels measured at the air monitoring stations reasonably reflect ambient air PM concentrations nearby. The spatial variability of airborne PM, however, can be quite large if one air monitoring station is located close to emission sources at a downwind location as compared to an air monitoring station that is farther away from emission sources or located in an upwind location (Wang et al., Citation2008). Thus, it is challenging to accurately assess community exposures to ambient air PM for health studies because of the resources required to build and maintain an adequately dense air-monitoring network.
Across the United States and throughout the world, community members are using low-cost portable direct-reading instruments for better understanding of air quality in their environments (Kumar et al., Citation2015; Williams et al., Citation2015). These instruments are easily deployed at multiple locations, providing the potential to improve exposure estimates by accounting for sources of spatial variability. Another advantage of a low-cost portable direct-reading instrument is that it requires fewer resources for air monitoring. However, questions arise regarding the quality of data collected by low-cost portable direct-reading instruments compared to Federal Reference Method instruments used to measure ambient air quality for regulatory compliance. Several studies have begun to evaluate their utility in characterizing outdoor and indoor air pollutant levels (Klepeis et al., Citation2013; Snyder et al., Citation2013; Williams et al., Citation2015).
Because many low-cost PM sensors (< $500) typically do not quantify particle mass concentrations but measure number of particles in the air, low-cost PM sensors are generally deployed side-by-side with an instrument directly measuring PM mass concentrations. Then the recorded particle number concentrations from the low-cost sensor are converted to PM mass concentrations using regression equations. Among various low-cost portable particle monitors, Dylos PM sensors (Dylos Corporation, Riverside, CA) have been evaluated with regard to whether the measured particle number concentrations can be used as a surrogate for estimating particle mass concentrations in general environments (Brown et al., Citation2014; Dacunto et al., Citation2015; Semple et al., Citation2015). According to the manufacturer, the Dylos DC 1100 and DC 1700 monitors can measure particle number concentrations for two particle size ranges: small, that is, particles larger than 0.5 µm, and large, that is, particles larger than 2.5 µm. One difference between the monitors is that the DC 1700 is equipped with a rechargeable battery and serial port for data download. Although previous studies reported that particle number concentrations for sizes greater than 0.5 µm but smaller than 2.5 µm, that is, [(small) – (large)], measured with the DC 1100 (or DC 1700) were highly correlated with PM2.5 mass concentrations measured with TSI Sidepak or DustTrak instruments (TSI, Incorporated, Shoreview, MN) in community environmental settings (Dacunto et al., Citation2015; Rosen et al., Citation2015; Semple et al., Citation2013), no studies have focused on airborne coarse PM (PM10-2.5). The application of the DC 1700 for the measurement of coarse PM has not been fully explored.
Another knowledge gap for the application of the DC 1700 is the impact of other factors (e.g., relative humidity or particle size distribution) on the measurement of particle number concentrations. The relationship between measured particle number concentrations from direct-reading instruments and particle mass concentrations can be significantly affected by relative humidity and particle size distribution. Under atmospheric conditions with high relative humidity, for instance, particle sizes in the air are increased due to the condensational growth of hygroscopic particles that may result in overestimation of PM2.5 mass concentrations (Chakrabarti et al., Citation2004; Lee et al., Citation2008; Ramachandran et al., Citation2003). Typically, daily relative humidity in the United States ranges from 40% to over 90%. Thus, it is likely that low-cost PM sensors can be substantially affected by relative humidity if the devices do not have correction mechanisms for water vapor in the air. While some studies have suggested the importance of relative humidity on the measurement of airborne particles using low-cost portable PM sensors in controlled environments or laboratory settings (Brown et al., Citation2014; Dacunto et al., Citation2015), none have been conducted in real-world community settings.
The overall goal of this study was to examine the usefulness of a low-cost portable direct-reading instrument to assess outdoor air levels of both PM2.5 and coarse PM (PM10-2.5). The first objective of our study was to compare particle number concentrations for PM2.5 and coarse PM from the DC 1700 with corresponding particle mass concentrations from the Grimm 11-R. The second objective of this study was to examine the effects of relative humidity and particle size distribution on the association between the calculated mass concentrations from the DC 1700 and the measured particle mass concentrations from the Grimm 11-R for both PM2.5 and coarse PM.
Experimental methods
Equipment
Dylos DC 1700 PM sensor
The DC 1700 (~$400) Air Quality Monitor (Dylos Corporation, Riverside, CA) is a relatively low-cost device that counts particle numbers per volume of air but does not measure particle mass concentrations. The unit can record particle number concentrations for two particle size ranges: (1) small size: particle size larger than 0.5 μm and (2) large size: particle size larger than 2.5 μm. To obtain particle number concentrations between 0.5 µm and 2.5 µm, we computed the difference in the particle number concentrations between the large (>2.5 µm) and small (>0.5 µm) particles (Dacunto et al., Citation2015; Klepeis et al., Citation2013; Northcross et al., Citation2013; Semple et al., Citation2013; Semple et al., Citation2015). The DC 1700 with a small fan cannot maintain constant airflow at the sampling inlet but sends an airstream to a laser detector located at approximately 90° to the airstream. The laser detector measures the scattered light intensity by the number of particles in the airstream and the changes of light intensity reported as particle number counts. The DC 1700 shows the particle numbers per 0.01 ft3 for both small (>0.5 μm) and large (>2.5 μm) particles. The measured data are automatically stored in internal memory with the logging interval at 1 min for 30 days. The DC 1700 is equipped with a serial port for data download to a computer using a serial/universal serial bus (USB) cable.
Grimm 11-R Spectrometer
The Grimm 11-R Mini Laser Aerosol Spectrometer (~$20,000) (Grimm Aerosol Technik GmbH & Co. KG, Ainring, Germany) can count particle number concentrations for particle size ranges from 0.25 µm to 32 µm with 31 size bins. The device uses laser optics (wavelength = 660 nm) to measure particle number concentrations for each size bin. Particle number concentrations can be counted from 1 to 3,000,000 particles/L or particle mass concentrations can be reported between 0.1 µg/m3 and 100 mg/m3. In addition, the Grimm 11-R shows particle mass concentrations for each size bin as well as for PM2.5 and PM10. The inlet flow rate is maintained at 1.2 ± 0.06 L/min with a constant-flow control device. The logging intervals can be from 6 sec to 1 hr. (We set the logging interval of the Grimm 11-R as 1 min to directly compare with the reading from the DC 1700 with the same 1-min interval.) Real-time data can be stored to a memory card or can be transmitted wirelessly to a laptop computer or a tablet. We used a 32-GB secure digital (SD) memory card to record the logged data. The calibration of mass concentrations for the Grimm 11-R was performed by the manufacturer with a filter-based sampling method (Peters et al., Citation2006).
Experimental design
To examine the usefulness of the DC 1700 for the estimation of ambient air PM2.5 and PM10-2.5 mass concentrations, we deployed one DC 1700 air quality monitor and one Grimm 11-R spectrometer side-by-side in the backyard of a residential home for 12 days between December 2015 and January 2016. The sampling location is located on the west side of Houston, TX, and is a suburban area, about 35 km from downtown Houston. The sampling location is in a residential community where there are not any industrial sources within a 10-km radius. A 12-lane freeway is located approximately 3 km north of the sampling location; however, the sampling site was upwind of this freeway during the experimental periods. A couple of residential houses near the sampling location used fireplaces in the evenings during the sampling periods. At the sampling site, we placed a Grimm 11-R and DC 1700 on a portable folding table to ensure that the heights of both inlets were about 1.2 m from the ground. Both direct-reading instruments continuously logged the data at a 1-min log interval. The sampling duration for each experiment ranged from 30 min to 24 hr. We collected particle samples under various weather conditions, including rainy days. We used a HOBO data logger U12-012 (Onset Computer Cooperation, Bourne, MA) to measure ambient air temperature and relative humidity (RH) during the same sampling periods. We programmed the HOBO data logger with a 1-min sampling interval corresponding to the DC 1700 and Grimm 11-R intervals. Before we deployed the samplers and data logger, we synchronized the date and time of all instruments using software provided by the manufacturers installed on a laptop computer.
Data analysis
We downloaded the recorded data on the DC 1700 with Dylos Logger v1.6 software (Dylos Corporation, Riverside, CA). The downloaded text file contains three variables: date/time, and particle number counts per 0.01 ft3 for small (particle size >0.5 µm) and for large (particle size >2.5 µm) particles. To determine particle number concentrations for PM2.5, we subtracted the particle number concentrations of the large-size bin from the particle number concentrations of the small-size bin. For particle number concentrations of coarse PM (PM10-2.5), we used the measured particle number concentrations of the large-size bin.
We also downloaded the recorded data on the Grimm 11-R with LabView 1.178 software (Grimm Aerosol Technik GmbH & Co, KG, Ainring, Germany). The software reports date/time and mass concentrations for PM10, PM2.5, and PM1 at each 1-min log interval during the sampling periods. We computed coarse PM (PM10-2.5) mass concentrations from the Grimm 11-R as the PM10 mass concentrations minus the PM2.5 mass concentrations. Additionally, the software shows particle number and mass concentrations for 31 particle size bins from 0.25 µm to 32 µm with 1-min log interval. We used the information from the size bins to calculate the mass median diameter (MMD) for the data at each 1-min interval. The MMD is the particle size for which half the particles are smaller and half are larger based on the mass in each size distribution. We calculated the MMD as described in Kulkarni et al. (Citation2011).
We used HOBOWare version 3.7.5 (Onset Computer Corporation, Bourne, MA) to download the recorded date/time, ambient temperature, and RH from the HOBO loggers. Prior to statistical analysis, we reviewed database files by checking the measured values, missing observations, and potential outliers, and validated date/time for all measurements such as particle number concentrations for PM2.5 and PM10-2.5 from the DC 1700, particle mass concentrations for PM2.5 and PM10-2.5 from the Grimm 11-R, and ambient temperature and RH from the HOBO data logger.
We first performed descriptive statistical analyses to examine the mean number concentrations with standard deviation (SD) for the DC 1700 data, mean particle mass concentrations with SD for the Grimm 11-R data, and means with SD for ambient temperature and RH. In addition to the calculation of mean with SD for each measured and calculated parameter, we examined the number of observations, and minimum, maximum, and median values. Second, we used a simple linear regression model to evaluate the relationship of particle values between the DC 1700 and the Grimm 11-R. Although it is desirable to use the data from the Grimm 11-R as the independent variable (X-axis) and that from the DC 1700 as dependent variable (Y-axis), we actually used the measured PM2.5 or coarse PM (PM10-2.5) mass concentrations from the Grimm 11-R as the dependent variable (Y-axis), and particle number concentrations of PM2.5 or coarse PM from the DC 1700 as the independent variable (X-axis) to ease comparisons of our results with the results from previous studies (Dacunto et al., Citation2015; Klepeis et al., Citation2013; Semple et al., Citation2013; Semple et al., Citation2015). Based on the linear regression analyses with all data, we used regression equations to convert particle number concentrations of PM2.5 or coarse PM from the DC 1700 to mass concentrations of PM2.5 or coarse PM, respectively. We compared the ratios of DC 1700 calculated PM mass concentrations to Grimm 11-R PM mass concentrations to examine the effects of RH and MMD. All statistical analyses were performed in SAS (Version 9.4, Cary, NC).
Results
Statistical summary
We collected a total of 8,330 measurements for each parameter from December 10, 2015, to January 10, 2016. The DC 1700 mean concentrations, with ranges, of PM2.5 (particle size between 0.5 and 2.5 µm) and coarse PM (particle size >2.5 µm) were 1,896/0.01 ft3 (30–36,400 per 0.01 ft3), and 103/0.01 ft3 (1–5,435 per 0.01 ft3), respectively. The average mass concentrations of PM2.5 and PM10-2.5 from the Grimm 11-R were 11.3 µg/m3 (0.2–318.7 µg/m3) and 4.8 µg/m3 (0–378.7 µg/m3), respectively. During the monitoring periods, average ambient temperature was 14.0 ºC (6.3–29.3 ºC) and the average RH was 63.2% (26.5–91.4%). The descriptive statistics are summarized in .
Table 1. Summary of statistics for measured ambient temperature, relative humidity, and particle sampling results for the DC 1700 and the Grimm11-R.
Association between particle number concentrations and mass concentrations
shows the overall association between particle number concentrations and mass concentrations for both PM2.5 and coarse PM. The regression coefficient (slope) and the coefficient of determination (r2) for these associations from simple linear regression are shown in . The slope for PM2.5 was 0.0071 µg/m3 per particle/0.01 ft3 and r2 was 0.778 (). We found that the linearity was somewhat decreased as particle number concentrations exceeded 10,000 per 0.01 ft3. For the association between DC 1700 coarse PM number concentrations and Grimm 11-R PM10-2.5 mass concentrations, we observed that the slope was 0.054 µg/m3 per particle/0.01 ft3 and r2 was 0.481 based on simple linear regression analysis (). Similar to the association between PM2.5 number concentrations and PM2.5 mass concentrations, the linear association between DC 1700 coarse PM number concentrations and PM10-2.5 mass concentrations from the Grimm 11-R was decreased as particle number counts exceeded 1,000 per 0.01 ft3. It is noteworthy that there seemed to be two different relationships between coarse PM particle number concentrations from the DC 1700 and PM10-2.5 mass concentrations from the Grimm 11-R.
Figure 1. Scatter plots of particle number count concentrations from the DC 1700 and PM mass concentrations from the Grimm 11-R. (a) Paired scatter plot (open circles) between the DC 1700 PM2.5 particle number count concentrations (PN2.5-0.5) and PM2.5 mass concentrations from the Grimm 11-R. The linear regression (dashed line) equation is summarized at the top. (b) Paired scatter plot (open squares) between the DC 1700 coarse PM particle count concentrations (PN>2.5) and the PM10-2.5 mass concentrations from the Grimm 11-R. The regression equation is summarized at the top.
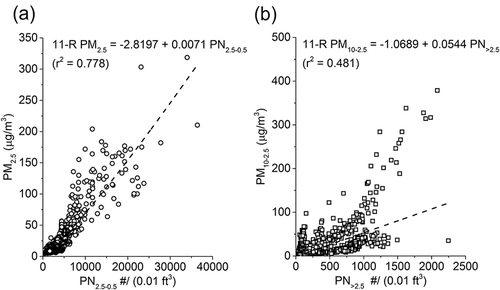
Comparison of calculated DC 1700 PM mass concentrations with measured Grimm 11-R mass concentrations
Using regression equations obtained from , we converted measured particle number concentrations from the DC 1700 to mass concentrations and compared them to the concurrently measured mass concentrations of PM2.5 and PM10-2.5 from the Grimm 11-R. Over the entire sampling periods, the average of the measured PM2.5 mass concentrations from the Grimm 11-R was 11.3 ± 15.1 µg/m3. The calculated DC 1700 PM2.5 mean mass concentration was 13.2 ± 13.7 µg/m3 using linear regression analysis from . Similar to the results for PM2.5, we used a linear regression equation from to compare the calculated DC 1700 coarse PM (larger than 2.5 µm) mass with the measured PM10-2.5 mass concentration from the Grimm 11-R. The average of the Grimm 11-R PM10-2.5 mass concentrations was 4.8 ± 16.5 µg/m3. The mean of the calculated DC 1700 coarse PM mass concentrations was 5.6 ± 12.1 µg/m3 using linear regression analysis for all data.
The calculated DC 1700 PM2.5 mass concentrations showed a trend similar to that of the measured PM2.5 mass concentrations from the Grimm 11-R over the entire 800-min sampling period shown (). In , the PM2.5 mass ranged from 0 to 200 µg/m3. Over the wide range of concentrations, the calculated DC 1700 PM2.5 mass concentrations were similar to the Grimm 11-R PM2.5 mass concentrations. also compares the calculated DC 1700 coarse PM concentrations with the mass concentrations of PM10-2.5 from the Grimm 11-R during the same sampling period. The calculated DC 1700 coarse PM mass concentrations were comparable to the Grimm 11-R PM10-2.5 mass concentrations from 0 min to 400 min, whereas the calculated DC 1700 coarse PM mass concentrations were 4-6 times higher than the Grimm 11-R PM10-2.5 mass concentrations from 400 min to 800 min. During the sampling periods, the mass concentrations of coarse PM from the DC 1700 ranged from 0.1 to 200 µg/m3 but the particle mass concentrations of PM10-2.5 from the Grimm 11-R varied between 0.1 and 50 µg/m3 ().
Effects of RH on the mass concentration ratios of DC 1700 to Grimm 11-R
Using the regression equations from , we examined the effects of ambient RH on the ratios of the DC 1700 mass concentrations to the Grimm 11-R PM mass concentrations. We stratified our data by hour of day. The diurnal changes of the hourly mean ratios of the DC 1700 to the Grimm 11-R for both PM2.5 and coarse PM are presented in . The overall mean ratio of paired DC 1700 PM2.5 mass concentration to Grimm 11-R PM2.5 mass concentration was 1.02 ± 0.48 and the overall mean ratio of paired DC 1700 coarse PM mass concentration to Grimm 11-R PM10-2.5 mass concentration was 2.00 ± 2.59. The ratios of DC 1700 to Grimm 11-R widely vagried within a day. In general, the ratios of both PM2.5 (above 1.3) and coarse PM (above 2.0) were the highest at night and early morning (10 p.m.– 6 a.m.). The ratios for both PM2.5 (0.8 to 1.1) and coarse PM (0.5 to 1.6) were closer to 1 between 10 a.m. and 4 p.m. We also observed that RH was the highest at night and early in the morning (10 p.m.–6 a.m.) and the RH (<60%) was the lowest during the daytime (between 10 a.m. and 4 p.m.).
Effects of particle size on the association between DC 1700 and Grimm 11-R mass concentrations
We examined the effects of particle size on the association between DC 1700 and Grimm 11-R data by mass median diameter (MMD). shows the associations between the calculated DC 1700 mass concentration and the measured 11-R mass concentration. We excluded 57 observations larger than 100 µg/m3 (0.7% of total measured observations) for both PM2.5 and coarse PM. The calculated DC 1700 PM2.5 mass concentrations showed good agreement with the measurements from the Grimm 11-R although the DC 1700 PM2.5 mass concentrations underestimated PM2.5 mass concentrations from the Grimm 11-R (). We observed that the ratios of calculated DC 1700 PM2.5 mass concentrations to measured Grimm 11-R PM2.5 mass concentrations were not significantly different when stratified by the three MMD groups, ranging from 1.9 to 4.4 µm. But we found that the association between DC 1700 coarse PM mass concentrations and the Grimm 11-R PM10-2.5 mass concentrations varied to a great degree. shows that the DC 1700 coarse PM concentration overestimated by a factor of 4 compared with the PM10-2.5 mass concentration from the Grimm 11-R when the MMD values were smaller than 2 µm. When the MMD of the measured aerosol was 2.9 µm, the calculated DC 1700 coarse PM mass concentrations were 30% higher than those measured by the Grimm 11-R. As the particle size increased (MMD = 4.4 µm), the DC 1700 underestimated coarse PM mass concentrations in the air relative to the Grimm 11-R measurements.
Figure 4. Scatter plots of calculated PM mass concentrations from the DC 1700 vs. Grimm 11-R PM mass concentrations. (a) Paired scatter plots by three different mass median diameters (MMD, µm) between the DC 1700 PM2.5 mass concentrations and PM2.5 mass concentrations from the Grimm 11-R. (b) Paired scatter plots by three different mass median diameters (MMD, µm) between the DC 1700 coarse PM mass concentrations and the PM10-2.5 mass concentrations from the Grimm 11-R. Each graph also shows the 1:1 (dashed) line.
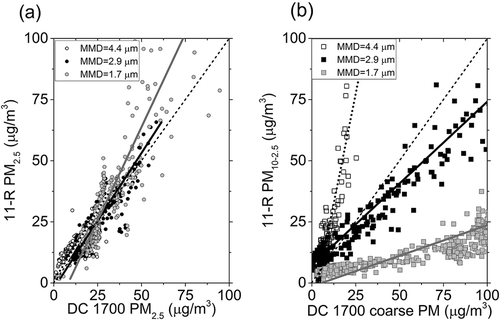
Discussion
We evaluated the feasibility of utilizing a low-cost PM sensor (Dylos DC 1700, approximately $400) to estimate ambient air PM2.5 and coarse PM mass concentrations by comparing its output with measurements taken with a widely used direct-reading instrument (Grimm 11-R, approximately $20,000). Overall, the trends, peak values, and magnitudes of both PM2.5 and coarse PM concentrations from the DC 1700 were similar to those from the Grimm 11-R over the sampling periods used in this study. Our results showed that the DC 1700 can be used to estimate both PM2.5 and coarse PM up to at least 300 µg/m3. The comparative results from this study are consistent with previous findings. Steinle et al. (Citation2015) used the DC 1700 to characterize personal exposure to PM2.5 in urban and rural environments in Scotland. Prior to conducting personal exposure assessment, the authors validated hourly-averaged PM2.5 data from the DC 1700 by collocating the DC 1700 with tapered element oscillating microbalances (TEOMs) and Filter Dynamics Measurement Systems (FDMS) over the range of 0–30 µg/m3 at national air monitoring sites. Another study, performed in Serbia, determined that the temporal trends, peak values, and magnitudes of particle number concentrations from the DC 1700 were consistent with both ambient air PM2.5 and PM10 mass concentrations from a Grimm 1108 spectrometer (precursor model of the Grimm 11-R), with PM2.5 and PM10 ranging from 0 to approximately 70 µg/m3 and from 0 to approximately 250 µg/m3, respectively (Jovasevic-Stojanovic et al., Citation2015). This suggests that the DC 1700 can be used to track temporal changes of both outdoor air PM2.5 and PM10-2.5 (or PM10) over a wide range of concentrations (0–319 µg/m3 for PM2.5 and 0-379 µg/m3 for PM10-2.5, respectively).
The results from this study showed that particle number concentrations of PM2.5 from the DC 1700 were well correlated with mass concentrations of PM2.5 measured using the Grimm 11-R. We found that the overall coefficient of determination (r2= 0.778) for PM2.5 in this study was similar to the results from previous studies. Other studies reported that the r2 values determined for the associations between particle number concentrations from the Dylos monitors and PM2.5 mass concentrations from reference instruments ranged between 0.70 and 0.99 in outdoor air environments. For example, Steinle et al. (Citation2015) reported correlation coefficients (r) ranging from 0.7 to 0.9 using 1-hr averaged PM2.5, and Northcross et al. (Citation2013) reported slightly higher coefficients of determination (r2), between 0.81 and 0.99, with 24-hr averaged PM2.5 concentrations. This suggests that the Dylos DC monitors can be potentially used to estimate acute (~1 min), short-term (~1 hr), or daily community exposures to PM2.5 in various outdoor air environments.
With regard to the particle mass concentrations determined from the DC 1700 particle number count concentrations, computed PM2.5 mass concentrations using all measured data are similar to those determined in other studies. For instance, the calculated PM2.5 mass concentration was 67.8 µg/m3 with linear regression () for a DC 1700 PM2.5 particle number concentration of 10,000/0.01 ft3. With the same particle number concentration (10,000/0.01 ft3), the calculated PM2.5 mass concentration was 63.2 µg/m3 from the regression equation of Klepeis and coauthors (Klepeis et al., Citation2013), and 80.7 µg/m3 from the work of Semple and coauthors (Semple et al., Citation2013). The findings suggest that the DC 1700 can be practically used to assess approximate PM2.5 mass concentrations in community environments if the sampled environments in our study were similar to previous ones. However, we cannot exclude the possibility that the comparable results from this study with others were obtained by chance. The physical and chemical characteristics of airborne PM would be different for each study. The airborne PM2.5 in this study had been collected in ambient air with a wide range of emission sources, as well as relative humidities, whereas the airborne PM2.5 in other studies (Klepeis et al., Citation2013; Semple et al., Citation2013) was measured mostly in indoor environments with relatively constant relative humidity.
The relationships of coarse PM between the DC 1700 and the Grimm 11-R were not as strong as those for PM2.5. The r2 value (coefficient of determination) from linear regression analyses between particle number concentrations and mass concentrations of coarse PM was moderate (0.481). Because there are as yet no other studies addressing the application of Dylos DC monitors for assessing community exposure to coarse PM, we could not compare our results with other studies. Although we do not know the reason why the coefficient of determination for coarse PM between the Dylos DC 1700 and the Grimm 11-R was significantly lower than for PM2.5, the moderate association may be partially related to the widely variable concentrations of coarse PM influenced by RH, particle size distribution, and chemical composition throughout a day.
As demonstrated in and , the reported widely variable coarse PM concentrations may be dependent on diurnal patterns of RH and particle size distributions. The increase in error of estimates for the DC 1700 coarse PM mass concentrations in outdoor air may be related to RH affecting the growth of hygroscopic particles (Lee et al., Citation2008). The density of a particle is dependent on RH—that is, as RH increases, the particle condenses more water vapor from the air. It is likely that the DC 1700 may imprecisely measure particle number concentrations in outdoor air with varying RH. Chakrabarti and co-investigators (Citation2004) reported that laser particle sensors overestimated PM mass concentrations by more than 70% when RH in the air was above 60%. Other studies also showed that laser particle measurement instruments demonstrated increased error at high RH in ambient air (Sioutas et al., Citation2000; Soneja et al., Citation2014).
In our study, we observed that airborne particle size is an important factor in assessing PM mass concentrations, especially coarse PM, using the DC 1700. As the particle size, defined by MMD, decreased to less than 2 µm, the concentration ratios of DC 1700 to Grimm 11-R for coarse PM increased to a great extent in this study. We observed that the ratios of DC 1700 coarse PM to Grimm 11-R PM10-2.5 were the largest (ratios >3) during the night and early morning when the particle size was typically less than 1 µm. In contrast, the ratios of DC 1700 coarse PM to Grimm 11-R PM10-2.5 were close to 1 during the daytime when the particle size was larger than 3 µm. Overall, the averages of calculated DC 1700 coarse PM were two times higher than those from the Grimm 11-R. An understanding of changes in particle size distributions is critical to assess the relationship of coarse PM between the DC 1700 and the Grimm 11-R.
The complexity of chemical composition and particle size in the measured aerosols can strongly contribute to the low association of coarse PM between the DC 1700 and the Grimm 11-R. In urban residential areas, there are multiple sources of particle emissions such as vehicular emissions, residential heating, biomass burning, and natural sources. Another source of airborne particles is secondary organic aerosols that are formed in the air following gas to particle conversion (Moise et al., Citation2015). It would have been ideal to analyze chemical composition and particle size distribution in the air as we were measuring airborne PM2.5 and coarse PM using the DC 1700 and the Grimm 11-R. However, such evaluation using the DC 1700 and the Grimm 11-R with 1-min logging intervals was not feasible in this study.
A significant and novel strength of our study is the evaluation of the relationship between the DC 1700 particle number concentrations and the coarse PM (PM10-2.5) concentrations from the Grimm 11-R. To date, no studies have systemically examined the association between DC 1700 coarse PM number concentrations and coarse PM (PM10-2.5) mass concentrations. Although Jovasevic-Stojanovic et al. (Citation2015) examined the association between the DC 1700 and the Grimm 1108 for PM10 and reported a correlation coefficient (r) of 0.858 (or coefficient of determination r2 = 0.736), the association between the DC 1700 and the Grimm 1108 for PM10-2.5 was not directly reported. The true association for coarse PM (PM10-2.5) would be lower in their study because the association of PM10 between the two instruments was heavily dependent on the association of PM2.5 between them.
Another strength of our study was an examination of the effects of particle size and RH on the association between the DC 1700 particle number concentrations and the Grimm 11-R mass concentrations. Numerous studies have characterized the conversion of DC 1700 particle number concentrations to PM2.5 mass concentrations for indoor and outdoor air quality measurements (Brown et al., Citation2014; Rosen et al., Citation2015; Semple et al., Citation2013; Semple et al., Citation2015). However, no studies have yet evaluated the effects of particle size distribution and RH on the relationship between the DC 1700 (or DC 1100) particle count concentrations and PM mass concentrations in the air.
We observed that deviations from the regression line were larger when the DC 1700 particle number concentrations exceeded 10,000/0.01 ft3 for PM2.5 or 1,000/0.01 ft3 for PM10-2.5 (see ). A few previous studies used polynomial regression equations because these studies observed that polynomial regression equations were better able to estimate PM2.5 mass concentrations than linear regression equations (Dacunto et al., Citation2015; Semple et al., Citation2013; Semple et al., Citation2015). The deviation between the DC 1700 and 11-R readings may be related to light sensor saturation when counting the number of particles as the airstream enters into the chamber where the laser sensor is located. If a large number of particles (i.e., a high challenge concentration) are introduced into the laser source, the response of the saturated laser is not linear and continues to read at a constant level (Perkins, Citation2008). Other potential factors may result from different emission sources in different environments, the presence of fresh or aged particles, and meteorological factors, such as relative humidity, which was examined in this study.
Limitations of this study include a relatively short sampling campaign (about a month), the absence of any chemical composition data, and no seasonal variation. Unlike the Grimm 11-R, information about the upper ends of the particle size ranges for the Dylos 1700 is not available. This limitation, in part, may result in a lower correlation between the Dylos 1700 and the Grimm 11-R for coarse PM. Furthermore, we compared the DC 1700 with the Grimm 11-R for both PM2.5 and coarse PM rather than the Federal Reference Method or other gravimetric PM mass measurements. Both instruments in this study measure light scattering intensity rather than mass concentrations directly. We also acknowledge that we did not examine instrument intervariability of the DC 1700 since we had tested only one DC 1700 in this study. Although we did not know the intervariability of the DC 1700, the results from Klepeis et al. (Citation2013) showed that the variability of the Dylos instruments was less than 10% in general environments. To improve the understanding of the performance of low-cost PM sensor such as the DC 1700, the listed limitations should be addressed in future studies.
Conclusion
We demonstrated the relationship between number concentrations from a low-cost PM sensor such as the Dylos DC 1700 and mass concentrations from a mainstream more expensive instrument such as the Grimm 11-R to estimate both PM2.5 and coarse PM (PM10-2.5) mass concentrations in urban ambient air. The range of calculated mass concentrations for both PM2.5 and coarse PM was from 0 to more than 300 µg/m3. The recorded particle number concentration from the DC 1700 needed to be converted to mass concentrations in order to be useful in determining relevant community or personal exposures to PM. The association between measured particle number concentrations from the DC 1700 and PM mass concentrations from the Grimm 11-R was satisfactorily explained using a linear regression fit, especially at concentrations lower than 100 µg/m3. The 1-min average PM2.5 and coarse PM mass concentration levels estimated by the DC 1700 overestimated the Grimm 11-R measured concentrations by approximately 1.02 and 2.00 times, respectively. We found that relative humidity significantly changes the association between the DC 1700 particle number concentrations and the measured PM mass concentrations from the Grimm 11-R. In general, relative humidity less than 60% showed that the mass concentration ratios of the DC 1700 to the Grimm 11-R were close to 1, but relative humidity greater than 60% resulted in ratios larger than 1 for both PM2.5 and PM10-2.5. Aerosol particle size was an important factor influencing the relationship between the two instruments for coarse PM, but was less important to the relationship between the instruments for PM2.5. Given these results, a low-cost PM sensor such as the DC 1700, albeit not specifically designed for evaluation of ambient air quality, may be useful to monitor for PM2.5 and coarse PM in community settings in future environmental health studies. The benefits of such a PM sensor include low cost, light weight, ease of use, ability to obtain real-time data, and the potential for large-scale simultaneous deployment of multiple units.
Funding
This study was supported in part by the CDC/NIOSH, 2T42OH008421.
Additional information
Funding
Notes on contributors
Inkyu Han
Inkyu Han is an Industrial Hygiene Faculty member of the Southwest Center for Occupational and Environmental Health (SWCOEH) and an assistant professor at the University of Texas Health Science Center (UTHealth) at Houston School of Public Health.
Elaine Symanski
Elaine Symanski is the director of the SWCOEH and an associate professor at the UTHealth at Houston School of Public Health.
Thomas H. Stock
Thomas H. Stock is an Industrial Hygiene Faculty member of the SWCOEH and an associate professor at the UTHealth at Houston School of Public Health.
References
- Adar, S.D., P.A. Filigrana, N. Clements, and J.L. Peel. 2014. Ambient coarse particulate matter and human health: A systematic review and meta-analysis. Curr. Environ. Health Rep. 1:258–74. doi:10.1007/s40572-014-0022-z
- Brown, D.R., N. Alderman, B. Weinberger, C. Lewis, J. Bradley, and L. Curtis. 2014. Outdoor wood furnaces create significant indoor particulate pollution in neighboring homes. Inhal. Toxicol. 26(10):628–35. doi:10.3109/08958378.2014.946633
- Chakrabarti, B., P.M. Fine, R. Delfino, and C. Sioutas. 2004. Performance evaluation of the active-flow personal DataRAM PM2.5 mass monitor (Thermo Anderson pDR-1200) designed for continuous personal exposure measurements. Atmos. Environ. 38:3329–3340. doi:10.1016/j.atmosenv.2004.03.007
- Dacunto, P.J., N.E. Klepeis, K.C. Cheng, V. Acevedo-Bolton, R.T. Jiang, J.L. Repace, W.R. Ott, and L.M. Hildemann. 2015. Determining PM2.5 calibration curves for a low-cost particle monitor: common indoor residential aerosols. Environ. Sci. Process Impacts 17(11):1959–66. doi:10.1039/c5em00365b
- Delfino, R.J., J. Wu, T. Tjoa, S. K. Gullesserian, B. Nickerson, and D.L. Gillen. 2014. Asthma morbidity and ambient air pollution: effect modification by residential traffic-related air pollution. Epidemiology 25(1):48–57. doi:10.1097/EDE.0000000000000016
- Eeftens, M., R. Beelen, K. de Hoogh, T. Bellander, G. Cesaroni, M. Cirach, C. Declercq, A. Dedele, E. Dons, A. de Nazelle, K. Dimakopoulou, K. Eriksen, G. Falq, P. Fischer, C. Galassi, R. Grazuleviciene, J. Heinrich, B. Hoffmann, M. Jerrett, D. Keidel, M. Korek, T. Lanki, S. Lindley, C. Madsen, A. Molter, G. Nador, M. Nieuwenhuijsen, M. Nonnemacher, X. Pedeli, O. Raaschou-Nielsen, E. Patelarou, U. Quass, A. Ranzi, C. Schindler, M. Stempfelet, E. Stephanou, D. Sugiri, M.Y. Tsai, T. Yli-Tuomi, M.J. Varro, D. Vienneau, S. Klot, K. Wolf, B. Brunekreef, and G. Hoek. 2012. Development of land use regression models for PM2.5, PM2.5 absorbance, PM10 and PMcoarse in 20 European study areas; Results of the ESCAPE project. Environ. Sci. Technol. 46(20):11195–205. doi:10.1021/es301948k
- International Agency for Research on Cancer. 2013. Air Pollution and Cancer, eds. K. Straif, A. Cohen and J.M. Samet. Lyon, France: IARC.
- Jerrett, M., R. McConnell, J. Wolch, R. Chang, C. Lam, G. Dunton, F. Gilliland, F. Lurmann, T. Islam, and K. Berhane. 2014. Traffic-related air pollution and obesity formation in children: A longitudinal, multilevel analysis. Environ. Health 13:49. doi:10.1186/1476-069X-13-49
- Jovasevic-Stojanovic, M., A. Bartonova, D. Topalovic, I. Lazovic, B. Pokric, and Z. Ristovski. 2015. On the use of small and cheaper sensors and devices for indicative citizen-based monitoring of respirable particulate matter. Environ. Pollut. 206:696–704. doi:10.1016/j.envpol.2015.08.035
- Klepeis, N.E., S.C. Hughes, R.D. Edwards, T. Allen, M. Johnson, Z. Chowdhury, K.R. Smith, M. Boman-Davis, J. Bellettiere, and M.F. Hovell. 2013. Promoting smoke-free homes: A novel behavioral intervention using real-time audio-visual feedback on airborne particle levels. PLoS ONE 8(8):e73251. doi:10.1371/journal.pone.0073251
- Krall, J.R., G.B. Anderson, F. Dominici, M.L. Bell, and R.D. Peng. 2013. Short-term exposure to particulate matter constituents and mortality in a national study of U.S. urban communities. Environ. Health Perspect. 121(10):1148–53. doi:10.1289/ehp.1206185.
- Kulkarni, P., P.A. Baron, and K. Willeke. 2011. Aerosol Measurement: Principles, Techniques, and Applications. New York, NY: John Wiley & Sons.
- Kumar, P., L. Morawska, C. Martani, G. Biskos, M. Neophytou, S. Di Sabatino, M. Bell, L. Norford, and R. Britter. 2015. The rise of low-cost sensing for managing air pollution in cities. Environ. Int. 75:199–205. doi:10.1016/j.envint.2014.11.019
- Lee, J. Y., H.J. Shin, S.Y. Bae, Y.P. Kim, and C.H. Kang. 2008. Seasonal variation of particle size distributions. Air Qual. Atmos. Health 1:57–68.
- Moise, T., J.M. Flores, and Y. Rudich. 2015. Optical properties of secondary organic aerosols and their changes by chemical processes. Chem. Rev. 115(10):4400–39. doi:10.1021/cr5005259
- Northcross, A.L., R.J. Edwards, M.A. Johnson, Z.M. Wang, K. Zhu, T. Allen, and K.R. Smith. 2013. A low-cost particle counter as a realtime fine-particle mass monitor. Environ. Sci. Process Impacts 15(2):433–39. doi:10.1039/c2em30568b
- Peng, R.D., H.H. Chang, M.L. Bell, A. McDermott, S.L. Zeger, J.M. Samet, and F. Dominici. 2008. Coarse particulate matter air pollution and hospital admissions for cardiovascular and respiratory diseases among Medicare patients. J. Am. Med. Assoc. 299(18):2172–79. doi:10.1001/jama.299.18.2172
- Perkins, J. 2008. Modern Industrial Hygiene, Volume 1—Recognition and Evaluation of Chemical Agents. Cincinnati, OH: ACGIH.
- Peters, T.M., D. Ott, and P.T. O’Shaughnessy. 2006. Comparision of the Grimm 1.108 and 1.109 portable aerosol spectrometer to the TSI 3321 aerodynamic particle sizer for dry particles. Ann. Occup. Hyg. 50(8):843–50.
- Pope, C. A. 3rd, and D.W. Dockery. 2006. Health effects of fine particulate air pollution: lines that connect. J. Air Waste Manage. Assoc. 56(6):709–42. doi:10.1080/10473289.2006.10464485
- Ramachandran, G., J.L. Adgate, G.C. Pratt, and K. Sexton. 2003. Characterizing indoor and outdoor 15 minute average PM2.5 concentrations in urban neighborhoods. Aerosol Sci. Technol. 37:33–45.
- Rosen, L., D. Zucker, M. Hovell, N. Brown, A. Ram, and V. Myers. 2015. Feasibility of measuring tobacco smoke air pollution in homes: Report from a pilot study. Int. J. Environ. Res. Public Health 12(12):15129–42. doi:10.3390/ijerph121214970
- Semple, S., A. Apsley, and L. Maccalman. 2013. An inexpensive particle monitor for smoker behaviour modification in homes. Tobacco Control 22(5):295–98. doi:10.1136/tobaccocontrol-2011-050401
- Semple, S., A.E. Ibrahim, A. Apsley, M. Steiner, and S. Turner. 2015. Using a new, low-cost air quality sensor to quantify second-hand smoke (SHS) levels in homes. Tobacco Control 24(2):153–58. doi:10.1136/tobaccocontrol-2013-051188
- Sioutas, C., S. Kim, M. Chang, L.L. Terrell, and H. Gong. 2000. Field evaluation of a modified DataRAM MIE scattering monitor for real-time PM2.5 mass concentration measurements. Atmos. Environ. (1994) 34:4829–38. doi:10.1016/S1352-2310(00)00244-2
- Snyder, E.G., T.H. Watkins, P.A. Solomon, E.D. Thoma, R.W. Williams, G.S. Hagler, D. Shelow, D.A. Hindin, V.J. Kilaru, and P.W. Preuss. 2013. The changing paradigm of air pollution monitoring. Environ. Sci. Technol. 47(20):11369–77. doi:10.1021/es4022602
- Soneja, S., C. Chen, J.M. Tielsch, J. Katz, S.L. Zeger, W. Checkley, F.C. Curriero, and P.N. Breysse. 2014. Humidity and gravimetric equivalency adjustments for nephelometer-based particulate matter measurements of emissions from solid biomass fuel use in cookstoves. Int. J. Environ. Res. Public Health 11(6):6400–16. doi:10.3390/ijerph110606400
- Steinle, S., S. Reis, C.E. Sabel, S. Semple, M.M. Twigg, C.F. Braban, S.R. Leeson, M.R. Heal, D. Harrison, C. Lin, and H. Wu. 2015. Personal exposure monitoring of PM2.5 in indoor and outdoor microenvironments. Sci. Total Environ. 508:383–94. doi:10.1016/j.scitotenv.2014.12.003
- Thurston, G.D., J. Ahn, K.R. Cromar, Y. Shao, H.R. Reynolds, M. Jerrett, C.C. Lim, R. Shanley, Y. Park, and R.B. Hayes. 2016. Ambient particulate matter air pollution exposure and mortality in the NIH-AARP Diet and Health Cohort. Environ. Health Perspect. 124(4):484–90. doi:10.1289/ehp.1509676
- Wang, Y., Y. Zhu, R. Salinas, D. Ramirez, S. Karnae, and K. John. 2008. Roadside measurements of ultrafine particles at a busy urban intersection. J. Air Waste Manage. Assoc. 58(11):1449–57. doi:10.3155/1047-3289.58.11.1449
- Williams, R.W., A. Kaufman, and S. Garvey. 2015. Dylos DC1100 Citizen science Operating Procedure. Washington, DC: U.S. Environmental Protection Agency.
- Wu, J., C. Ren, R.J. Delfino, J. Chung, M. Wilhelm, and B. Ritz. 2009. Association between local traffic-generated air pollution and preeclampsia and preterm delivery in the south coast air basin of California. Environ. Health Perspect. 117(11):1773–79. doi:10.1289/ehp.0800334
- Wu, J., M. Wilhelm, J. Chung, and B. Ritz. 2011. Comparing exposure assessment methods for traffic-related air pollution in an adverse pregnancy outcome study. Environ. Res. 111(5):685–92. doi:10.1016/j.envres.2011.03.008
- Zanobetti, A., M.A. Bind, and J. Schwartz. 2008. Particulate air pollution and survival in a COPD cohort. Environ. Health 7:48. doi:10.1186/1476-069X-7-48