ABSTRACT
Many studies have demonstrated that cold and hot temperatures are associated with increased deaths and hospitalization rates; new findings indicate also an association with more specific cardiac risk factors. Most of these existing studies have relied on few weather stations to characterize exposures; few have used residence-specific estimates of temperature, or examined the exposure-response function. We investigated the association of arrhythmia episodes with spatial and temporal variation in temperature. We also evaluated the association btween monitored ambient temperature (central) and the same outcome. This longitudinal analysis included 701 older men participating in the VA Normative Aging Study. Arrhythmia episodes were measured as ventricular ectopy (VE) (bigeminy, trigeminy, or couplets episodes) by 4-min electrocardiogram (ECG) monitoring in repeated visits during 2000–2010. The outcome was defined as having or not VE episodes during a study visit. We applied a mixed-effect logistic regression model with a random intercept for subject, controlling for seasonality, weekday, medication use, smoking, diabetes status, body mass index, and age. We also examined effect modification by personal characteristics, confounding by air pollution, and the exposure-response function. For 1°C increase in the same day residence-specific temperature, the odds of having VE episodes was 1.10 (95% confidence interval [CI]: 1.04–1.17). The odds associated with 1°C increase in central temperature was 1.05 (95% CI: 1.02–1.09). The exposure-response function was nonlinear for averages of temperature, presenting a J-shaped pattern, suggesting greater risk at lower and higher temperatures. Increased warm temperature and decreased cold temperature may increase the risk of ventricular arrhythmias.
Implications: This is the first study to provide evidence that residence-specific temperature exposure is associated with increased risk of ventricular arrhythmias in cohort of elderly subjects without known chronic medical conditions; that the delayed effect of temperature has a nonlinear relationship; and therefore that both warm and cold temperature increase the risk of having ventricular arrhythmias. Moreover, we show that the use of residence-specific temperature data reduces downward bias due to exposure error, by comparing the estimated health effect based on our spatiotemporal exposure prediction model to those based on a single local weather monitor.
Introduction
Cardiovascular and cerebrovascular diseases are among the greatest causes of morbidity and mortality in adults in the United States and worldwide (Lloyd-Jones et al., Citation2009). Ventricular tachyarrhythmia, primarily ventricular tachycardia (VT) and ventricular fibrillation (VF), are common mechanisms of sudden cardiac death (Myerburg et al., Citation1992).
The recently released Intergovernmental Panel on Climate Change (IPCC) report (IPCC, Citation2014) provided strong evidence that CO2 emissions will lead to warmer temperatures, and to more extreme heat and cold events (Meehl and Tebaldi, Citation2004). Numerous multicity time-series analyses have demonstrated that cold and hot temperatures, as well as temperature extremes, are associated with increased death and hospitalization rates in the days following these weather conditions (Basu, Citation2009; Analitis et al., Citation2008; Ye et al., Citation2012; Breitner et al., Citation2014). Increased mortality has been linked not only to heat-wave episodes (Kaiser et al., Citation2007; Le Tertre et al., Citation2006; Ostro et al., Citation2009) but also to exposure to colder weather conditions (Analitis et al., Citation2008; Lee et al., Citation2014). These studies focused on hospital admissions or mortality, while fewer studies have investigated patient’s specific onset of arrhythmia (Nguyen et al., Citation2013; Nguyen et al., Citation2015; McGuinn et al., Citation2013). Some studies showed that chronic disease including cardiovascular disease, diabetes mellitus, and mental illness, but also sociodemographic characteristics and social isolation may heighten susceptibility to temperature-related mortality (Basu, Citation2009; O’Neill et al., Citation2003; Zanobetti et al., Citation2013), and that even without known chronic medical conditions, aging may increase susceptibility to the cardiovascular effects of temperature extremes and changes in temperature.
The relationship between temperature and mortality and hospitalization has been shown to be nonlinear (Nordio et al., Citation2015), and spline methods as well as artificial neural networks (Kassomenos et al., Citation2011) methods to study the effect of nonlinear parameters such as the temperature on cardiovascular admissions have been used.
Most epidemiologic studies have relied on weather data from the major city airport to characterize exposure, ignoring important factors that may influence temperature at individual residences, including urban heat islands, distance from water, and amount of impermeable surface (Kloog, Chudnovsky, et al., Citation2012; Kloog et al., Citation2014). This introduces substantial exposure error into all of those studies.
We have recently presented and validated a temporally and spatially resolved prediction model utilizing satellite surface temperature data (Kloog et al., Citation2014) to estimate daily ambient temperature at the participant’s home address. With this method, we estimated purely spatial variation based on the differences between predicted temperatures on a given day at each participant address relative to the central site monitor. Using resident specific exposure is particularly important when the population under study is not a working population but, as in our study, is an elderly population whose members rarely leave home.
In a previous study (Zanobetti et al., Citation2014), we investigated the association between temporally and spatially resolved black carbon (BC) and PM2.5 (particulate air matter with aerodynamic diameter less than 2.5 micrometers) and ventricular ectopy (VE) in the Normative Aging Study, and we found that increases in levels of short-term traffic-related pollutants (both black carbon and PM2.5) were associated with increased risk of such episodes in this cohort of elderly subjects. In this follow-up study, we investigated the association between fine-scale spatial and temporal variation in temperature and VE episodes among participants of the Veterans Affairs (VA) Normative Aging Study (NAS) residing in the greater Boston area. We compared the estimated health effect based on our spatiotemporal exposure prediction model to those based on a single local weather monitor located at Boston Logan Airport. We also examined the form of the exposure–response relationship.
Methods
As in our previous study, the participants included in this analysis were enrolled in the Normative Aging Study (NAS), an ongoing longitudinal study of aging established by the Veterans Administration in 1963, details of which have been published previously (Zanobetti et al., Citation2014). Briefly, the NAS is a closed cohort of 2,280 community-dwelling men from the Greater Boston area aged 21 to 80 years at the time of entry, who were enrolled after an initial health screening determined that they were free of known chronic medical conditions. Subjects were asked to return for onsite physical examinations and questionnaires every 3–5 years. Dropout has been less than 1% per year in this cohort and predominantly occurs when participants move out of the study area. The other major reason for loss to follow-up has been mortality. Eligibility for this study required continued participation as of the time when heart-rate variability measurements began in November 2000. Since 1963, every 3 to 5 years, participants have undergone routine physical examinations, laboratory tests, collection of medical history information, and completion of questionnaires on smoking history, educational level, food intake, and other factors that may influence health.
Diabetes was defined as a physician diagnosis of diabetes, use of any diabetes medication, or fasting glucose >126 mg/dL. Body mass index (BMI) was computed as the weight in kilograms divided by the squared of the height in meters. Obesity was defined as a body mass index greater than or equal to 30. In addition, BMI was recalculated based on height and weight measurements at each visit. Self-reported data on diabetes status and statin use were updated at each study visit and confirmed by a physician interview. Thus, these data reflect changes in disease status and medication use over time.
The study was approved by the Human Research Committees of the Harvard School of Public Health and the Department of Veterans Affairs Boston Healthcare System, and written informed consent was obtained from participants prior to participation (Bell et al., Citation1972).
Electrocardiogram (ECG) measurement and analysis
Examinations were done in the morning, after an overnight fast. After a 5-minute rest, with the subject seated, a 5- to 10-min electrocardiogram (ECG) was taken with a two-channel (five-lead) ECG monitor (Trillium 3000; Forest Medical, Inc., East Syracuse, NY) using a sampling rate of 256 Hz per channel. A detailed description of the protocol has been previously published (Park et al., Citation2005). Briefly, with personal computer (PC)-based software, beats were automatically labeled and assigned tentative annotations, and then an experienced scanner reviewed the results to correct for any mislabeled beats or artifacts, and the best 4-consecutive-minute intervals were used for the analysis. One hundred fifty-three measurements were excluded because of ECG recordings that had recording time <3.5 min, or insufficient T-wave amplitude.
We examined arrhythmia episodes measured as ventricular ectopy (VE) episodes. We defined VE as having bigeminy, trigeminy, or couplets episodes. We then created an indicator variable for having (= 1) or not having (= 0) VE episodes during a study visit.
We examined 1448 observations of subjects who had either one (n = 701), two (n = 451), three (n = 217), or four (n = 79) ECG measurements over time. Diabetes, obesity, and statin use were treated as time-varying covariates, where the status was updated at each visit.
Air pollution and meteorology
Hourly mean temperature (°F) measurements across the U.S. Northeast for 2000–2011 were obtained from two sources: the National Climatic Data Center (NCDC, http://www.ncdc.noaa.gov) and Weather Underground, Inc. (WU, https://www.wunderground.com).
PM2.5 and black carbon (BC) concentrations were continuously measured at the stationary ambient monitoring site at the Harvard Supersite in Boston, MA. We also estimated (1) BC exposures using a validated spatiotemporal land-use regression model that provides daily estimates of BC concentrations throughout the greater Boston, MA, area; and (2) daily predictions of PM2.5 concentration levels across New England for 2000–2008 at a 10 × 10 km spatial resolution, using satellite-based physical aerosol optical depth (AOD) measurements (Gryparis et al., Citation2007; Paciorek et al., Citation2009). Full details of the modeling framework have been previously published (Kloog et al., Citation2011; Kloog, Coull, et al., Citation2012). Estimated spatially and temporally resolved estimates of PM2.5 and BC concentrations were considered in sensitivity analyses to examine potential confounding by this coexposure.
Temperature exposure prediction
The present study uses 1 × 1 km air temperature (Ta) data estimated from surface temperature (Ts) measured by satellites. Specifically, meteorological data were obtained from the National Climatic Data Center for all weather stations in New England. Data from Weather Underground stations in New England were also obtained, and these ground-level monitors were used to calibrate satellite measurements. We started by calibrating the Ts – Ta relationship for each day using grid cells with both Ta and Ts measurements. This daily calibration was then used to predict Ta in grid cells in New England without Ta measurements but with available Ts measurements. To fill in cells or days when no Ts measurements were available, we fitted a second model; specifically, we applied a generalized additive mixed model regressing predicted temperature in each grid cell on available days against the nearest monitor, land use terms, and a smooth function of latitude and longitude of the grid cell centroid. The performance of the estimated Ta was validated by 10-fold cross-validation. Out-of-sample R2 was found to be very high (R2 = 0.947, yearly variation 0.933–0.958 for the years 2000–2011) for days with available satellite Ts measurements. Excellent performance was also observed even for days with no available Ts data (R2 = 0.940, yearly variation 0.902–0.962 for the years 2000–2011). More details are provided in Kloog et al. (Citation2014).
For both the temperature predictions based on this spatiotemporal prediction model and daily monitored temperature from Logan Airport, we constructed the same-day temperature and the mean of temperatures of lags 1–3 before a given subject visit as exposure variables. Prior studies have indicated that the former better captures the effects of hot days and the latter better captures the effect of cold days (Braga et al., Citation2001). We also created the 2- and 3-days averages of temperature before a given subject visit as exposure variables.
Statistical analyses
In this study our exposure variables were temperature measured at Logan Airport (hereafter referred to as central temperature) and spatially and temporally resolved temperature from our model (referred to as resident-specific temperature). Our outcome was VE episodes, which were dichotomized as present or absent at each study visit.
We applied mixed logistic regression models in order to account for correlation among measurements on the same subject across different medical visits. This is a standard approach for analyzing longitudinal data with repeated measures on the same subject (Fitzmaurice et al., Citation2004). We adjusted for confounders defined a priori based on our previous study. We included in all models random subject-specific intercepts and fixed effects for BMI, age, cumulative cigarette smoking in pack-years, use of medication such as angiotensin-converting-enzyme inhibitor (ACE inhibitors), β-blockers, calcium blockers, statins, having diabetes (yes/no), and alcohol consumption characterized by an indicator of whether a subject consumed two or more drinks per day. We adjusted for seasonality using sine and cosine terms, and a linear variable for year to capture long-term changes in both outcome and exposure.
We also adjusted for socioeconomic status (SES) at the individual level with average income and years of education (defined as less than 12 years, 12–16 years, over 16 years). At the neighborhood level for each subject’s residence we used the 1999 Census data, available at the Census block level, with (1) percent below poverty level and (2) percent of population over 25 years of age without a high school diploma, both for the Census block group.
As has been done in previous studies in the same NAS cohort, we applied inverse probability weighting (IPW) to correct for a potential survival bias in the cohort (Hernán et al., Citation2006). We assumed that the probability at the first visit was 1; we then estimated the probability of having a second, third, and subsequent visit using logistic regressions, given relevant factors at the previous visit: age, BMI, smoking status, pack-years, hypertension, cholesterol, and diabetes. We used the inverse of the predicted probabilities of appearing in the sample as the weights in the mixed effects logistic models for the arrhythmia outcome.
We studied the exposure-response relationship between the outcome and exposure by including a penalized spline (Ruppert et al., Citation2003) for the exposure variables in the model for the same day temperature, and up to the 3-days average of temperature. The spline for the same-day temperature was estimated with 1 degree of freedom and was therefore linear, while the relationship was nonlinear for the average of temperature.
Given that the model presented a linear relationship between VE and the same-day temperature but nonlinear with averaging times of temperature, our final model included the same day temperature as a linear variable plus the spline of the average of the previous 1 to 3 days temperature, as this longer average has been shown to better capture effects of cold temperature.
To examine effect modification we included an interaction term between the exposure and a given effect modifier that partitioned the sample into subjects. When estimating the joint effects of same-day exposure and the previous 1- to 3-day average exposure in the same model, we included an interaction term for a given modifier for the same-day exposure and an interaction in the smoothing function for the previous 1- to 3-day average exposure. The effect modifiers examined were diabetes, obesity, and statin use.
The results are reported as odds ratios (OR) per 1°C increase in the same day linear temperature. When including the penalized smoothing function for the effect of the mean of lags 1–3, we report the plots and the odds ratio at 0°C (for the cold effect) and at 22°C (for the hot effect) compared to the average temperature (11°C). The analyses were performed with statistical software R 2.15.1.
Results
In we present characteristics of the patients, together with the VE and the exposure variables, among the NAS population during the years 2000–2010. The age range of the study subjects was 57–100 years, and BMI varied between 17 and 46 kg/m2, with 27% of participants being obese.
Table 1. Characteristics of the VA Normative Aging Study subjects at baseline and across all visits and distributions of the weather and outcome variables.
shows the results of the association between VE episodes and the two different metrics of temperature, for several models. For 1°C increase in the same day residence-specific temperature exposure (, model 1), we found an increase in the odds of having ventricular ectopic beats of 1.10 (95% confidence interval [CI]: 1.04–1.17). The odds ratio associated with 1° C increase in central temperature (, model 5) was 1.05 (95% CI: 1.02–1.09). The OR estimate for the resident-specific temperature is outside the confidence interval of the association between outcome and central temperature, suggesting that the resident-specific temperature estimates (OR = 1.10) are higher compared to the estimate of the central site temperature (OR = 1.05). On the other hand, the OR for central temperature is not significantly lower compared to the OR for resident-specific temperature.
Table 2. Odds ratios (OR) and 95% confidence intervals (CI) of the association between ventricular ectopy episodes and temperauture for different models.
In sensitivity analysis, we included residence-specific black carbon (BC) and PM2.5, and we found that these pollutants did not confound the association between both temperature metrics and VE (, models 2, 3, 6, and 7).
We then examined the form of the exposure-response relationship () by including penalized smoothing functions of the exposure in the models. We found that the relationship was linear for the same day exposure (not shown), but it was nonlinear, with a U- or J-shaped pattern for the lagged moving averages as shown in for both temperature metrics. We found a different shape for the two curves. While the central temperature has an inverse J-shaped pattern, the resident-specific temperature response curve is more U-shaped, reaching higher risks with higher temperature compared to the central temperature curve. In , for each model, we report the results at 0°C and at 22°C compared to the average temperature (11°C), which correspond to a difference of 11 (22 – 11 = 11) degrees, respectively.
Figure 1. Exposure–response relationship between VE and the lagged moving averages for residence-specific temperature (top row) and for central temperature (bottom row). The solid line represents the penalized spline curve and the dotted line the 95% confidence intervals around the curve.
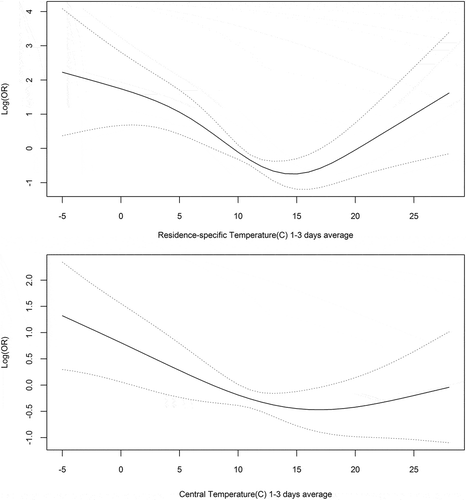
In the model (, model 4) where the exposure was fitted using both linear and nonlinear residence-specific temperature, we found increases in the odds of having ventricular ectopic beats with an OR of 1.15 (95% CI: 1.1–1.2) for the linear term for same-day temperature, while for the penalized spline for the lagged (1–3 days) average of temperature we found an OR of 5.7 (95% CI: 16.6–1.96) at 0°C and an OR of 1.4 (95% CI: 3.9–0.5) at 22°C compared to average temperature. The effects were similar but with smaller effect sizes when we used central temperature as our exposure (, model 8). In this model, we found an OR of 1.08 (95% CI: 1.03–1.12) for the linear term for the same day central temperature; an OR of 2.2 (95% CI: 4.7–1.1) at 0°C; and an OR of 0.7 (95% CI: 1.4–0.4) at 22°C compared to average temperature for the spline of 1–3 days average temperature. To compare these results with the same-day estimate, the odds ratio for an increase of 11°C in temperature (instead of 1°C increase) would be an OR of 2.99 (95% CI: 1.5–5.8) for residence-specific temperature and an OR of 1.8 (95% CI: 1.2–2.7) for central temperature.
For both temperature metrics, these results show a significant immediate effect of warm temperature as expressed by the same-day temperature. Warm temperatures in the previous days also affect VE with a similar effect estimate to the same day temperature. The OR at 0°C is much larger than the corresponding OR at 22°C, indicating a larger effect on VE at colder temperatures. Moreover, the effect estimates using the resident-specific temperature are larger compared to the estimates using the central site temperature.
We then examined effect modification by obesity, statin use, and having diabetes, but we didn’t find any evidence of modification by personal characteristics with both same-day temperature and the 1–3 days average of temperature. The interactions between each modifier and the exposure linear term were not significant, while the plots of the smoothing functions of the average exposures by each of the modifiers didn’t show a clear different pattern.
Conclusions
This study provides evidence that temperature is associated with increased risk of ventricular arrhythmias. We estimated that the immediate effect of temperature is linear, but that the delayed effect over the previous 3 days is nonlinear with a U- or J-shaped pattern, depending on the model. While the 3-days cumulative exposure presents stronger effects at 22 °C compared to the average temperature, when including both a linear term for same-day temperature and a spline for the average of 1–3 days before the ECG measurement, we found a steeper slope at colder temperatures. Therefore, we found that an increase in warm and a decrease in cold temperature increase the risk of having ventricular arrhythmias.
When comparing the results using central temperature and the resident-specific temperature we found that the associations for the resident-specific temperature were larger compared to the estimates using the central temperature as exposure, suggesting that the use of spatially resolved temperature data might reduce downward bias due to exposure error.
We also found a different shape for the two exposure-response curves, with the resident-specific temperature curve presenting higher risks for higher temperature, compared to the central temperature curve, which was more flat at higher temperature. This has potential implication for climate change, as it would suggest that localized temperatures are more important than the regional temperature.
In Boston, patients with implantable cardioverter-defibrillators exposed to drier air and lower temperatures were associated with the onset of atrial fibrillation (Nguyen et al., Citation2015). In the same cohort the authors found a nonlinear association between outdoor temperature and ventricular arrhythmias, with lower temperature and drier air associated with increased risk of ventricular arrhythmia onset (Nguyen et al., Citation2013). Similarly, in another study of implanted cardiac defibrillators and daily outdoor temperatures McGuinn et al. found a negative association between outdoor temperature and ventricular arrhythmias (McGuinn et al., Citation2013). These cohorts examined potentially life-threatening outcomes, and the patients were at higher risk (implanted defibrillators) compared to the present study, where subjects were without known chronic medical conditions.
Mechanisms for effects of temperature on the risk of cardiovascular morbidity and mortality are imperfectly understood. Studies investigating the associations between temperature and cardiac risk factors, such as blood pressure (Halonen et al., Citation2011a; Alpérovitch et al., Citation2009; Lanzinger et al., Citation2014), markers of inflammation (Halonen et al., Citation2010; Schäuble et al., Citation2012), changes in heart rate and repolarization parameters (Hampel et al., Citation2010), and cholesterol (Yamamoto et al., Citation2003; Halonen et al., Citation2011b), have shown divergent results.
The elderly and those with several chronic health conditions have difficulty thermoregulating and acclimatizing to heat and cold (Kenny et al., Citation2010). They have lower metabolic heat production and are more prone to develop disorders of thermoregulatory function (Collins, Citation1986). Extreme heat or cold conditions may be associated with an increased frequency of ventricular tachyarrhythmias and may trigger cardiac arrhythmias in vulnerable populations (Fries et al., Citation1997). Decreases in air temperature (cold) can cause a sympathetic reflex that reduce heat loss by redistributing blood to the core and can produce an increase in cardiac output (Cagle and Hubbard, Citation2005). Temperature extremes (hot or cold) may influence sympathetic drive, peripheral arterial resistance, blood pressure, central blood volume, and ventricular filling pressure, particularly in people with diabetes or with other conditions resulting in compromised regulatory responses. The physiologic adjustments made by the human body to preserve body temperature could increase cardiac work and sympathoadrenergic activation (Fries et al., Citation1997). High heat exposures, dehydration, renal compromise, and electrolyte imbalance may also worsen arrhythmia risk. Cold weather conditions could contribute to the occurrence of arrhythmias through activation of both the sympathetic nervous system and the coagulation system. Vasoconstriction induced by cold weather increases blood pressure, heart rate, and left ventricular end-diastolic pressure and volume. These changes increase heart workload and may reduce the ischemic threshold, especially among individuals with compromised coronary circulation (Abrignani et al., Citation2009; Meyer et al., Citation2010), and in turn trigger arrhythmia onset.
One limitation of this study is that these results cannot be generalized to other populations without further research, as our study population consisted entirely of elderly men, 97% of whom were white. Moreover, people spend most of their time indoors; therefore, these results might not be representative of the wider populations, even though the correlation between indoor and outdoor temperature is high. Additionally, there may be survivor bias if the subjects who continue to participate are healthier than other older people, but that we accounted for by using IPW.
In conclusion, these results add support for the hypothesis that both lower and higher temperature may be associated with increases in ventricular arrhythmias. Moreover, the associations using the resident-specific temperature are larger than the associations using central site temperature.
Funding
This work was supported by the National Institute of Aging, R21 AG040027-01, the National Institute of Environmental Health Sciences, P01 ES009825, P30 ES000002, R21 ES020194-01, and R21ES024012, and by the U.S. Environmental Protection Agency, RD-83241601 and RD-83479801. Its contents are solely the responsibility of the grantee and do not necessarily represent the official views of the EPA. Further, the EPA does not endorse the purchase of any commercial products or services mentioned in the publication. This study was also supported by a VA Research Career Scientist award to David Sparrow. The Veterans Administration’s Normative Aging Study is supported by the Cooperative Studies Program/Epidemiology Research and Information Centers of the U.S. Department of Veterans Affairs and is a component of the Massachusetts Veterans Epidemiology Research and Information Center, Boston, MA.
Additional information
Funding
Notes on contributors
Antonella Zanobetti
Antonella Zanobetti is a principal research scientist in the Exposure, Epidemiology and Risk program, in the Department of Environmental Health, Harvard T.H. Chan School of Public Health, Boston, MA.
Brent A. Coull
Brent A. Coull is a professor of biostatistics and Associate Chair of the Department of Biostatistics, Harvard T.H. Chan School of Public Health, Boston, MA.
Itai Kloog
Itai Kloog is a senior lecturer in the Department of Geography and Environmental Development, Ben-Gurion University of the Negev, Beer Sheva, Israel. He is also a visiting scientist in the Department of Environmental Health, Harvard T.H. Chan School of Public Health, Boston, MA.
David Sparrow
David Sparrow is a professor in the Department of Medicine, Boston University School of Medicine, and in the Department of Epidemiology at Boston University School of Public Health, and at VA Boston Healthcare System, Boston, MA.
Pantel S. Vokonas
Pantel S. Vokonas is a professor in the Department of Medicine, Boston University School of Medicine, and in the Department of Epidemiology at Boston University School of Public Health, and at VA Boston Healthcare System, Boston, MA.
Diane R. Gold
Diane R. Gold is a professor in the Department of Environmental Health, Harvard T.H. Chan School of Public Health, Boston, MA. She is a professor of medicine in the Channing Laboratory, Department of Medicine, Harvard Medical School, Boston, and she is an associate physician at Brigham and Women’s Hospital, Boston, MA.
Joel Schwartz
Joel Schwartz is a professor in the Department of Environmental Health, Harvard T.H. Chan School of Public Health, Boston, MA.
References
- Abrignani, M.G., S. Corrao, G.B. Biondo, N. Renda, A. Braschi, G. Novo, A. Di Girolamo, G.B. Braschi, and S. Novo. 2009. Influence of climatic variables on acute myocardial infarction hospital admissions. Int. J. Cardiol. 137(2):123–29. doi:10.1016/j.ijcard.2008.06.036.
- Alpérovitch, A., J.-M. Lacombe, O. Hanon, J.-F. Dartigues, K. Ritchie, P. Ducimetière, and C. Tzourio. 2009. Relationship between blood pressure and outdoor temperature in a large sample of elderly individuals: The Three-City Study. Arch. Intern. Med. 169(1):75–80. doi:10.1001/archinternmed.2008.512.
- Analitis, A., K. Katsouyanni, A. Biggeri, M. Baccini, B. Forsberg, L. Bisanti, U. Kirchmayer, et al. 2008. Effects of cold weather on mortality: Results from 15 European cities within the PHEWE Project. Am. J. Epidemiol. 168(12):1397–408. doi:10.1093/aje/kwn266
- Basu, R. 2009. High ambient temperature and mortality: A review of epidemiologic studies from 2001 to 2008. Environ. Health 8(1):40. doi:10.1186/1476-069X-8-40
- Bell, B., C. Rose, and A. Damon. 1972. The Normative Aging Study: An interdisciplinary and longitudinal study of health and aging. Aging Hum. Dev. 3(1):5–17. doi:10.2190/GGVP-XLB5-PC3N-EF0G
- Braga, A. L., A. Zanobetti, and J. Schwartz. 2001. The time course of weather-related deaths. Epidemiology 12(6):662–67.
- Breitner, S., K. Wolf, A. Peters, and A. Schneider. 2014. Short-term effects of air temperature on cause-specific cardiovascular mortality in Bavaria, Germany. Heart. 100(16):1272–1280. doi:10.1136/heartjnl-2014-305578.
- Cagle, A., and R. Hubbard. 2005. Cold-related cardiac mortality in King County, Washington, USA 1980–2001. Ann. Hum. Biol. 32(4):525–37. doi:10.1080/03014460500153758.
- Collins, K. J. 1986. Low indoor temperatures and morbidity in the elderly. Age Ageing 15(4):212–20. doi: 0.1093/ageing/15.4.212
- Fitzmaurice, G.M., N.M. Laird, and J.H. Ware. 2004. Applied Longitudinal Analysis. Hoboken, NJ: Wiley. doi:10.1016/S0002-9149(97)00081-7
- Fries, R.P., A.G. Heisel, J.K. Jung, and H.J. Schieffer. 1997. Circannual variation of malignant ventricular tachyarrhythmias in patients with implantable cardioverter-defibrillators and either coronary artery disease or idiopathic dilated cardiomyopathy. Am. J. Cardiol. 79(9):1194–97. doi:10.1016/S0002-9149(97)00081-7
- Gryparis, A., B.A. Coull, J. Schwartz, and H.H. Suh. 2007. Semiparametric latent variable regression models for spatiotemporal modelling of mobile source particles in the Greater Boston Area. J. Roy. Stat. Soc. Ser. C Appl. Stat. 56(2):183–209. doi:10.1111/j.1467-9876.2007.00573.x.
- Halonen, J.I., A. Zanobetti, D. Sparrow, P.S. Vokonas, and J. Schwartz. 2010. Associations between outdoor temperature and markers of inflammation: A cohort study. Environ. Health 9:42. doi:10.1186/1476-069X-9-42.
- Halonen, J.I., A. Zanobetti, D. Sparrow, P.S. Vokonas, and J. Schwartz. 2011a. Relationship between outdoor temperature and blood pressure. Occup Environ. Med 68(4):296–301. doi:10.1136/oem.2010.056507
- Halonen, J.I., A. Zanobetti, D. Sparrow, P.S. Vokonas, and J. Schwartz. 2011b. Outdoor temperature is associated with serum HDL and LDL. Environ. Res. 111(2):281–87. doi:10.1016/j.envres.2010.12.001
- Hampel, R., A. Schneider, I. Brüske, W. Zareba, J. Cyrys, R. Rückerl, S. Breitner, et al. 2010. Altered cardiac repolarization in association with air pollution and air temperature among myocardial infarction survivors. Environ. Health Perspect. 118(12):1755–61. doi:10.1289/ehp.1001995.
- Hernán, M.A., E. Lanoy, D. Costagliola, and J.M. Robins. 2006. Comparison of dynamic treatment regimes via inverse probability weighting. Basic Clin. Pharmacol. Toxicol. 98(3):237–42. doi:10.1111/j.1742-7843.2006.pto_329.x.
- Intergovernmental Panel on Climate Change. 2014. Climate Change 2014: Impacts, Adaptation, and Vulnerability. IPCC Working Group II Contribution to AR5. http://www.ipcc.ch/report/ar5/wg2/ (accessed November 16, 2016).
- Kaiser, R., A. Le Tertre, J. Schwartz, C.A. Gotway, W.R. Daley, and C.H. Rubin. 2007. The effect of the 1995 heat wave in Chicago on All-cause and cause-specific mortality. Am. J. Public Health 97( Suppl. 1):S158–62. doi:10.2105/AJPH.2006.100081
- Kassomenos, P., M. Petrakis, D. Sarigiannis, A. Gotti, and S. Karakitsios. 2011. Identifying the contribution of physical and chemical stressors to the daily number of hospital admissions implementing an artificial neural network model. Air Qual. Atmos. Health 4(3–4):263–72. doi:10.1007/s11869-011-0139-2.
- Kenny, G.P., J. Yardley, C. Brown, R.J. Sigal, and O. Jay. 2010. Heat stress in older individuals and patients with common chronic diseases. Can. Med. Assoc. J. 182(10):1053–60. doi:10.1503/cmaj.081050
- Kloog, I., A. Chudnovsky, P. Koutrakis, and J. Schwartz. 2012. Temporal and spatial assessments of minimum air temperature using satellite surface temperature measurements in Massachusetts, USA. Sci. Total Environ. 432(August):85–92. doi:10.1016/j.scitotenv.2012.05.095.
- Kloog, I., B.A. Coull, A. Zanobetti, P. Koutrakis, and J.D. Schwartz. 2012. Acute and chronic effects of particles on hospital admissions in New England. PLoS One 7(4):e34664. doi:10.1371/journal.pone.0034664.
- Kloog, I., P. Koutrakis, B.A. Coull, H.J. Lee, and J. Schwartz. 2011. Assessing temporally and spatially resolved PM2.5 exposures for epidemiological studies using satellite aerosol optical depth measurements. Atmos. Environ. 45(35):6267–75. doi:10.1016/j.atmosenv.2011.08.066.
- Kloog, I., F. Nordio, B.A. Coull, and J. Schwartz. 2014. Predicting spatiotemporal mean air temperature using MODIS satellite surface temperature measurements across the Northeastern USA. Remote Sens. Environ. 150:132–39. doi:10.1016/j.rse.2014.04.024
- Lanzinger, S., R. Hampel, S. Breitner, R. Rückerl, U. Kraus, J. Cyrys, U. Geruschkat, A. Peters, and A. Schneider. 2014. Short-term effects of air temperature on blood pressure and pulse pressure in potentially susceptible individuals. Int. J. Hyg. Environ. Health. 217(7):775–784. doi:10.1016/j.ijheh.2014.04.002.
- Lee, M., F. Nordio, A. Zanobetti, P. Kinney, R. Vautard, and J. Schwartz. 2014. Acclimatization across space and time in the effects of temperature on mortality: A time-series analysis. Environ. Health 13(1):89. doi:10.1186/1476-069X-13-89.
- Lloyd-Jones, D., R. Adams, M. Carnethon, G. De Simone, T.B. Ferguson, K. Flegal, E. Ford, et al. 2009. Heart Disease and stroke statistics—2009 Update: A report from the American Heart Association Statistics Committee and Stroke Statistics Subcommittee. Circulation 119(3):480–86.
- McGuinn, L., S. Hajat, P. Wilkinson, B. Armstrong, H.R. Anderson, V. Monk, and R. Harrison. 2013. Ambient temperature and activation of implantable cardioverter defibrillators. Int. J. Biometeorol. 57(5):655–62. doi:10.1007/s00484-012-0591-1.
- Meehl, G.A., and C. Tebaldi. 2004. More intense, more frequent, and longer lasting heat waves in the 21st century. Science 305(5686):994–97. doi:10.1126/science.1098704
- Meyer, P., T. Guiraud, D. Curnier, M. Juneau, M. Gayda, A. Nozza, and A. Nigam. 2010. Exposure to Extreme cold lowers the ischemic threshold in coronary artery disease patients. Can. J. Cardiol. 26(2):e50–53. doi:10.1016/S0828-282X(10)70007-6
- Myerburg, R.J., K.M. Kessler, and A. Castellanos. 1992. Sudden cardiac death. Structure, function, and time-dependence of risk. Circulation 85(1 Suppl.): I2–10. doi:10.1111/jce.1992.3.issue-6
- Nguyen, J.L., F. Laden, M.S. Link, J. Schwartz, H. Luttmann-Gibson, and D.W. Dockery. 2013. Weather and triggering of ventricular arrhythmias in patients with implantable cardioverter-defibrillators. J. Expos. Sci. Environ. Epidemiol. 25(2):175–181. doi:10.1038/jes.2013.72.
- Nguyen, J. L., M. S. Link, H. Luttmann-Gibson, F. Laden, J. Schwartz, B. S. Wessler, M. A. Mittleman, D. R. Gold, and D. W. Dockery. 2015. Drier air, lower temperatures, and triggering of paroxysmal atrial fibrillation. Epidemiology 26(3):374–80. doi:10.1097/EDE.0000000000000284.
- Nordio, F., A. Zanobetti, E. Colicino, I. Kloog, and J. Schwartz. 2015. Changing patterns of the temperature-mortality association by time and location in the US, and implications for climate change. Environ. Int. 81(August):80–86. doi:10.1016/j.envint.2015.04.009.
- O’Neill, M.S., A. Zanobetti, and J. Schwartz. 2003. Modifiers of the temperature and mortality association in seven US cities. Am. J. Epidemiol. 157(12):1074–82. doi:10.1093/aje/kwg096
- Ostro, B.D., L.A. Roth, R.S. Green, and R. Basu. 2009. Estimating the mortality effect of the July 2006 California heat wave. Environ. Res. 109(5):614–19. doi:10.1016/j.envres.2009.03.010
- Paciorek, C.J., J.D. Yanosky, R.C. Puett, F. Laden, and H.H. Suh. 2009. Practical large-scale spatio-temporal modeling of particulate matter concentrations. Ann. Appl. Stat. 3(1):370–97. doi:10.1214/08-AOAS204
- Park, S.K., M.S. O’Neill, P.S. Vokonas, D. Sparrow, and J. Schwartz. 2005. Effects of air pollution on heart rate variability: The VA Normative Aging Study. Environ. Health Perspect. 113(3):304–9. doi:10.1289/ehp.7447
- Ruppert, D., M.P. Wand, and R.J. Carroll. 2003. Semiparametric Regression. New York, NY: Cambridge University Press.
- Schäuble, C.L., R. Hampel, S. Breitner, R. Rückerl, R. Phipps, D. Diaz-Sanchez, R.B. Devlin, et al. 2012. Short-term effects of air temperature on blood markers of coagulation and inflammation in potentially susceptible individuals. Occup. Environ. Med. 69(9):670–78. doi:10.1136/oemed-2011-100469.
- Le Tertre, A., A. Lefranc, D. Eilstein, C. Declercq, S. Medina, M. Blanchard, B. Chardon, et al. 2006. Impact of the 2003 heatwave on all-cause mortality in 9 French cities. Epidemiology 17(1):75–79. doi:10.1097/01.ede.0000187650.36636.1f
- Yamamoto, H., K.C. Zheng, and M. Ariizumi. 2003. Influence of Heat exposure on serum lipid and lipoprotein cholesterol in young male subjects. Ind Health 41(1):1–7. doi:10.2486/indhealth.41.1
- Ye, X., R. Wolff, W. Yu, P. Vaneckova, X. Pan, and S. Tong. 2012. Ambient temperature and morbidity: A review of epidemiological evidence. Environ. Health Perspect. 120(1):19–28. doi:10.1289/ehp.1003198.
- Zanobetti, A., B.A. Coull, A. Gryparis, I. Kloog, D. Sparrow, P.S. Vokonas, R.O. Wright, D.R. Gold, and J. Schwartz. 2014. Associations between arrhythmia episodes and temporally and spatially resolved black carbon and particulate matter in elderly patients. Occup. Environ. Med. 71(3):201–7. doi:10.1136/oemed-2013-101526.
- Zanobetti, A., M.S. O’Neill, C.J. Gronlund, and J.D. Schwartz. 2013. Susceptibility to mortality in weather extremes: Effect modification by personal and small area characteristics in a multi-city case-only analysis. Epidemiology 24(6):809–19. doi:10.1097/01.ede.0000434432.06765.91.