ABSTRACT
The objective of this study was to estimate the residential infiltration factor (Finf) of fine particulate matter (PM2.5) and to develop models to predict PM2.5 Finf in Beijing. Eighty-eight paired indoor–outdoor PM2.5 samples were collected by Teflon filters for seven consecutive days during both non-heating and heating seasons (from a total of 55 families between August, 2013 and February, 2014). The mass concentrations of PM2.5 were measured by gravimetric method, and elemental concentrations of sulfur in filter deposits were determined by energy-dispersive x-ray fluorescence (ED-XRF) spectrometry. PM2.5 Finf was estimated as the indoor/outdoor sulfur ratio. Multiple linear regression was used to construct Finf predicting models. The residential PM2.5 Finf in non-heating season (0.70 ± 0.21, median = 0.78, n = 43) was significantly greater than in heating season (0.54 ± 0.18, median = 0.52, n = 45, p < 0.001). Outdoor temperature, window width, frequency of window opening, and air conditioner use were the most important predictors during non-heating season, which could explain 57% variations across residences, while the outdoor temperature was the only predictor identified in heating season, which could explain 18% variations across residences. The substantial variations of PM2.5 Finf between seasons and among residences found in this study highlight the importance of incorporating Finf into exposure assessment in epidemiological studies of air pollution and human health in Beijing. The Finf predicting models developed in this study hold promise for incorporating PM2.5 Finf into large epidemiology studies, thereby reducing exposure misclassification.
Implications: Failure to consider the differences between indoor and outdoor PM2.5 may contribute to exposure misclassification in epidemiological studies estimating exposure from a central site measurement. This study was conducted in Beijing to investigate residential PM2.5 infiltration factor and to develop a localized predictive model in both nonheating and heating seasons. High variations of PM2.5 infiltration factor between the two seasons and across homes within each season were found, highlighting the importance of including infiltration factor in the assessment of exposure to PM2.5 of outdoor origin in epidemiological studies. Localized predictive models for PM2.5 infiltration factor were also developed.
Introduction
Epidemiologic studies usually use fine particulate matter (PM2.5) mass concentrations obtained from ambient fixed monitoring sites as surrogates for population exposure to PM2.5 of ambient origin (Dockery et al., Citation1993; Dominici et al., Citation2003; Pope and Dockery, Citation2006). However, most people spend 85–90% of their time indoors (Klepeis et al., Citation2001; Robinson and Nelson, Citation1995), and thus exposure to ambient PM2.5 primarily occurs indoors. The chemical properties and physical characteristics such as concentrations, chemical compositions, and size distribution of PM2.5 may change in the process of movement from outdoor to indoor environments (Landis et al., Citation2001; Sangiorgi et al., Citation2013; Sarnat et al., Citation2006; Talbot et al., Citation2016). Therefore, failure to consider the differences between indoor and outdoor PM2.5 may contribute to exposure misclassification in epidemiological studies estimating exposure from a central site measurement (Meng et al., Citation2005).
Infiltration factor (Finf) was defined as the fraction of outdoor particles that penetrate the building envelope and remain suspended (Chen and Zhao, Citation2011). It is one of the most important parameters to characterize the impacts of outdoor to indoor transit on particulate matter concentrations; hence, the Finf is useful to better characterize ambient PM2.5 exposure. Several studies conducted in the United States (Allen et al., Citation2003; Allen et al., Citation2012; Hystad et al., Citation2009; Meng et al., Citation2009; Wallace and Williams, Citation2005), Canada (Barn et al., Citation2008; Clark et al., Citation2010; Kearney et al., Citation2014; MacNeill et al., Citation2012; MacNeill et al., Citation2014), and European nations (Hänninen et al., Citation2004; Meier et al., Citation2015) investigated the levels of Finf. The reported Finf varied substantially between regions and seasons, with ranges of 0.27 ± 0.18 in Prince George, British Columbia, Canada (Barn et al., Citation2008) to 0.82 ± 0.14 in New York, NY (during the warm season) (Allen et al., Citation2012). The spatial and temporal variability of Finf was considered to be due to differences in influential factors across areas and seasons, including climate, building characteristics, human activity, and heating and ventilation systems (Chen and Zhao, Citation2011).
PM2.5 Finf values have been estimated by different methods, including indoor/outdoor ratio of sulfur (Allen et al., Citation2012; Clark et al., Citation2010; Hänninen et al., Citation2004; MacNeill et al., Citation2014; Wallace and Williams, Citation2005), censored inside/outside (I/O) ratio (Allen et al., Citation2003; Kearney et al., Citation2014; MacNeill et al., Citation2012; MacNeill et al., Citation2014), and a recursive model (Barn et al., Citation2008; Huang et al., Citation2015; Hystad et al., Citation2009). Simultaneous indoor and outdoor PM2.5 concentrations are required for these methods. The costs of collecting site-specific field data, participant burden, and building access restrictions have prevented large epidemiologic studies from incorporating Finf in their exposure assessments. To overcome this limitation, some investigators tried to develop Finf-predicting models using potential influential factors. Allen et al. (Citation2012) found central air conditioning (AC) use, frequency of AC use, and window opening frequency were the most important predictors during the warm season, while outdoor temperature and forced-air heat were the best cold-season predictors in six U.S. cities. Kearney et al. (Citation2014) reported that the building ages, air exchange rates, air cleaner use, temperature difference between indoors and outdoors, and wind speeds were the determining factors for Finf in winter for Edmonton, AB, Canada, while no predictive factor was identified in summer from the study. These results clearly indicate that localized prediction models may need to be developed (Hystad et al., Citation2009; MacNeill et al., Citation2012; Meier et al., Citation2015).
Mega cities in China, especially Beijing, have high levels of ambient air pollution. Thus, more and more epidemiological studies have been conducted to explore the adverse health risks of atmospheric air pollution recently. It is not appropriate to apply the Finf values obtained from Western countries to assess exposure to ambient PM2.5 in China due to the drastically different building characteristics, human behavior, heating and ventilation systems, and climate. To the best of our knowledge, Shi et al. (Citation2015) recently reported the Finf for urban residences in Beijing based on 24-hr real-time indoor and outdoor PM2.5 mass concentrations during the nonheating season, but there is no report on Finf during the heating season in Beijing. To address this need, we conducted a field study to estimate the residential PM2.5 Finf and to develop models to predict PM2.5 Finf in Beijing for both nonheating and heating seasons. Infiltration was estimated using the indoor/outdoor sulfur ratio, and multiple linear regression was employed to develop PM2.5 Finf predicting models.
Methods
Participant recruitment
Homes in an urban area of Beijing were selected using stratified sampling based on building type (apartment, house and quadrangle courtyard) and floors (1st–3rd, 4th–9th, 10th–). Because Finf varies by season, we collected air samples in both the nonheating season (August 2013–October 2013) and the heating season (December 2013–February 2014). In total, 71 homes were recruited for the study initially. However, seven homes were excluded from the study because the homes had smoking inhabitants or opening kitchens that were reported as indoor sulfur sources (Koutrakis et al., Citation1992; Leaderer et al., Citation1999). In total, 52 homes were eventually selected and participated in the nonheating season study, while 55 homes were selected and participated in the heating season study (43 of the 55 homes that participated in the heating season also participated in the nonheating season). In the process of data cleaning, 9 homes in the nonheating season were removed from data analysis for flow rate failure (n = 4), abnormal filter weighting results (n = 1), or Finf > 1.05 (n = 4), while 10 homes in the heating season were removed for flow rate failure (n = 7), abnormal filter weighting results (n = 1), or Finf > 1.05 (n = 2). Thus, data from 43 homes in the nonheating season and 45 homes in the heating season were included in the final data analysis, and 33 homes have valid data for both seasons. The study was approved by the Human Investigation Committee of the Chinese NIEHS, and all study participants provided written informed consent.
Investigation of housing parameters and daily activities
A standardized and structured questionnaire was used to collect information from each family on factors that were anticipated to influence PM2.5 Finf, such as home age and size, number of inhabitants, household incomes, type of ventilation, energy used for cooking or heating, presence of air cleaner and air conditioner, and heating and cooling systems used. During the sampling periods, the households were also required to record activities that occurred during sampling, such as cooking, cleaning, exterior window opening for the room in which indoor measurements were conducted, guest smoking, and use of air conditioner and air cleaner.
Air pollution measurements
Simultaneous indoor and outdoor PM2.5 levels were continuously sampled by an active-pattern sampler (PCXR8; SKC, Inc., Eighty Four, PA) with 37-mm Teflon filters (PA 15330; Pall Gelman, Ann Arbor, MI) with flow rates set at 0.5 L/min. Each home was sampled for a 7-day period in each season. Outdoor sampling devices were extended approximately 1 m out an available window, and the window was sealed with weather stripping to prevent the influence of flow of indoor air on outdoor concentrations. Indoor sampling devices were placed in the living room or bedroom where participants spent the majority of their time. The inlet of indoor samplers was 1–1.5 m above the floor with at least 1 m distance from the room wall, and away from pollution sources and ventilation systems. As quality control measures, flow rates were assessed pre- and postsampling using a calibrated electronic flowmeter (4140; TSI, Inc., Shoreview, MN). Samples with flow rates that differed by more than 20% from the target rates were considered invalid.
Indoor and outdoor PM2.5 mass concentrations were measured by a gravimetric method (XP6; Mettler-Toledo International Inc., Switzerland). All filters were preconditioned for a minimum of 24 hr before weighing at a constant air temperature of 25 ±1ºC and constant relative humidity (RH) of 50% (±5%). After PM2.5 weight analysis was completed, elemental concentrations of sulfur in filter deposits were determined by energy-dispersive x-ray fluorescence (ED-XRF) spectrometry (by Key Laboratory of Aerosol Chemistry and Physics, Institute of Earth Environment, Chinese Academy of Sciences, Xi’an, China). The typical analytical limit of sulfur detection was estimated to be 3.0 ng/cm2. As a quality control measurement, a calibration check was performed by analyzing NIST standards (SRM2783). Measurement uncertainties were determined as the relative standard deviation of several analyses of the same samples, yielding <10%.
Daily outdoor temperatures and wind speeds during sampling periods were obtained from the National Meteorological Information Center.
Statistical analysis
Finf was estimated by calculating the ratio of the indoor and outdoor sulfur concentrations. Multiple linear regression was used to examine the association between potential predictors and Finf. A two-stage approach was used for Finf model development. First, univariate regression analysis was employed to determine the relationships between candidate variables and Finf. Second, a multivariate linear regression model was developed based on preexisting knowledge and variables that were associated (p < 0.10) with Finf in the univariate analysis and that were not highly correlated (r > 0.50) with other independent variables (Hystad et al., Citation2009). The focused candidate predictors included outdoor temperature, building type, floors, air conditioner use, and window opening behavior. A stepwise variable selection procedure was conducted to choose the final model. Season-specific models were constructed under the assumption that the Finf predictors and their model coefficients would vary between seasons. SPSS 19.0 statistical software was applied for all analysis.
Results
Household characteristics
The detailed information on building characteristics and household demographics is shown in . Information from the questionnaire indicated that none of investigated homes used an air cleaner or mechanical ventilation equipment in either of the two sampling sessions. Natural ventilation was the only ventilation mode. Window opening was much more prevalent in the nonheating season than in the heating season. The recorded median time with at least one exterior window opening during 7-day sampling periods was 95 hr in the nonheating season; the response value was only 3.5 hr in the heating season. During the nonheating season, 21% (9/43) homes reported use of an air conditioner, with the mean total time for using air conditioner 35.4 ± 11.4 hr (median = 33.5 hr) in a 7-day sampling period; however, there was no use of air conditioning in the heating season. Urban central heating without fresh air delivery units was the dominant heating mode during winter (43/45), and only two homes used a household heating system using natural gas or electricity.
Table 1. The general household characteristics.
Residential indoor and outdoor levels of PM2.5 and sulfur and Finf estimates
As shown in , the residential indoor and outdoor PM2.5 concentrations were 59 ± 17 μg m−3 and 73 ± 20 μg m−3, respectively, in the nonheating season. The corresponding values for the heating season were 69 ± 39 μg m−3 and 97 ± 39 μg m−3, respectively. The indoor PM2.5 concentrations were significantly related to outdoor PM2.5 concentrations in both the nonheating season (Pearson r = 0.63) and the heating season (Pearson r = 0.37), while the indoor concentrations were lower than outdoor levels (p < 0.001). This result indicated that outdoor PM2.5 could influence indoor PM2.5 pollution, but not all outdoor PM2.5 would transfer to indoors. The indoor and outdoor concentrations of sulfur element in PM2.5 also showed similar patterns, as presented in .
Table 2. Residential indoor and outdoor levels of PM2.5 and Finf estimates by season.
The mean (±SD) PM2.5 Finf across all residences and seasons was 0.62 ± 0.21. Seasonal PM2.5 Finf was also calculated and is shown in . The mean (±SD) residential Finf in the nonheating season (0.70 ± 0.21, median = 0.78) was significantly greater than in the heating season (0.54 ± 0.18, median = 0.52, p < 0.001). The PM2.5 Finf of 33 residences monitored in both the heating and nonheating seasons is shown in . Seventy-six percent (25/33) of these residences have greater Finf in the nonheating season than in the heating season, and the difference was also significant (p < 0.001). Within each season, considerable variability of PM2.5 Finf between homes was also found. The PM2.5 Finf ranged from 0.23 to 0.98 (more than a fourfold difference) in the nonheating season and from 0.27 to 0.92 (more than a threefold difference) in the heating season.
Figure 1. Comparison of PM2.5 Finf between the nonheating season and the heating season for 33 residences monitored in both seasons.
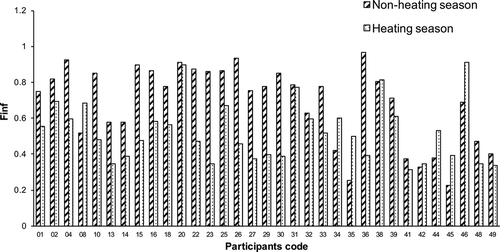
No significant difference existed between home types of apartment (0.60 ± 0.20, n = 50), house (0.59 ± 0.25, n = 10), and quadrangle courtyard (0.67 ± 0.21, n = 28, p = 0.25), and between building age of <10 yr (0.66 ± 0.20, n = 15), 10–20 yr (0.60 ± 0.20, n = 24), and >20 yr (0.62 ± 0.22, n = 49, p = 0.691). There was also no significant difference between building types and building ages within the heating or nonheating season.
Finf model
Univariate regression analysis was employed to determine the relationships between candidate variables and Finf. In general, the outdoor temperatures, average wind speeds, floors, and opening window behavior (frequency and width) were associated with Finf (p < 0.1) in univariate regression analysis. The results from univariate regression analysis also verified the assumption that Finf predictors and their correlation coefficients varied between seasons, such as for outdoor temperature.
The factors identified in the univariate regression analysis and reported in previous study, such as air conditioner use, building age, and number of cigarettes smoked in home by guests, were selected as candidate predictors to develop predicting models. The results of multiple linear regression analysis are shown in and . In the nonheating season, the final predictors included outdoor temperature, window opening behavior (opening time and width), and time of air conditioner use. All the variables could explain 57% of the Finf variance in the nonheating season. Higher outdoor temperate, more frequent and wider opening window, and less time of air conditioner use would be related to higher Finf values. However, in the heating season, only outdoor temperature was included in the final predicting model. We did not find the main factors influencing the Finf in the heating season, since only a limited portion of Finf variance (18%) could be explained.
Table 3. Prediction model for Finf in the nonheating season (R2 = 0.566).
Table 4. Prediction model for Finf in heating season (R2 = 0.175).
Discussion
The PM2.5 Finf for urban residences in Beijing was investigated in this study, and we have added onto a past study (Shi et al., Citation2015) in terms of data collection for both heating and nonheating seasons. First, a seasonal variance of PM2.5 Finf was found in this study. The lower PM2.5 Finf in the heating season was not unexpected, given that inhabitants closed windows for a much longer time in the heating season. The same seasonal Finf difference has also been reported in a number of studies (Allen et al., Citation2003; Allen et al., Citation2012; Barn et al., Citation2008; Hystad et al., Citation2009; Kearney et al., Citation2014; MacNeill et al., Citation2014). In comparison, some other studies found few seasonal differences (Clark et al., Citation2010; Kearney et al., Citation2011; MacNeill et al., Citation2012) or even higher PM2.5 Finf in the heating season (Wallace and Williams, Citation2005). A likely explanation for these inconsistent results is that the season itself was just an indirect influential factor for PM2.5 Finf, and the relationship between season and other direct influential factors, such as window opening, air conditioner use, and mechanical ventilation, varied among areas. For example, in contrast to Beijing, the inhabitants in Raleigh, North Carolina are more likely to close windows and to use air conditioning for the extremely humid and hot weather in summer, and thus the average air exchange rates in summer (0.49 ± 0.57 hr−1) were lower than in winter (1.01 ± 0.73 hr−1), and that made the PM2.5 Finf in summer lower (0.50) than for other seasons (0.63) (Hystad et al., Citation2009; Wallace and Williams, Citation2005).
There were more than four- and threefold Finf differences across homes within the nonheating and heating seasons in Beijing, respectively. According to the definition of Finf, the population in residences of Beijing would be exposed to PM2.5 of outdoor origin for more than four- to threefold difference even under the same ambient pollution condition. The considerable variation in Finf found in this study and other reports (Allen et al., Citation2012; Barn et al., Citation2008; Chen and Zhao, Citation2011; Clark et al., Citation2010; Hystad et al., Citation2009; Kearney et al., Citation2014; MacNeill et al., Citation2012; MacNeill et al., Citation2014), highlighted that variance of Finf was an important source of misclassification in exposure assessment to PM2.5 of outdoor origin in epidemiological studies estimating exposure from a central site measurement.
The PM2.5 Finf (0.70 ± 0.21, median = 0.78) in the nonheating season found in this investigation was quite similar to the result from another study conducted in Beijing by Huang using a steady-state model (0.72 ± 0.01) (Huang et al., Citation2015). We also compared the PM2.5 Finf estimate in Beijing with other regions. For the nonheating season, our PM2.5 Finf (0.70 ± 0.21, median = 0.78) was similar to Halifax (median = 0.80) (MacNeill et al., Citation2014), combined Victoria and Seattle (0.72 ± 0.19) (Hystad et al., Citation2009), Athens (0.70 ± 0.12) (Allen et al., Citation2003), and Seattle (0.79 ± 0.18) (Allen et al., Citation2003), where window opening was also very frequent (Kearney et al., Citation2014), but higher than for Toronto (0.54 ± 0.22) (Clark et al., Citation2010), Prince Raleigh (0.50 ± 0.06) (Wallace and Williams, Citation2005), and Windsor (median range = 0.24–0.29) (MacNeill et al., Citation2012). For the heating season, the PM2.5 Finf (0.54 ± 0.18, M = 0.52) obtained in this study was comparable to Seattle (0.53 ± 0.16) (Allen et al., Citation2003), Toronto (0.48 ± 0.21) (Clark et al., Citation2010), combined Victoria and Seattle (0.49 ± 0.15) (Hystad et al., Citation2009), and Seattle (0.53 ± 0.16) (Allen et al., Citation2003), but considerably higher than for Edmonton (median = 0.30) (Kearney et al., Citation2014), Windsor (median range = 0.33–0.34) (MacNeill et al., Citation2012), and Prince George (0.27 ± 0.18) (Barn et al., Citation2008), where the residences were sealed quite well due to the extremely cold winter. Generally, the PM2.5 Finf estimate in Beijing was among the highest levels for both the nonheating and heating reasons.
Season-specific models have been developed to predict PM2.5 for residences in Beijing for this study. As expected, the predicting variables and coefficients were quite different between the nonheating and heating seasons. In the nonheating season, two variables related to window opening (width of window and time spent open) were included in the predicting model, consistent with the result from Halifax (MacNeill et al., Citation2014), Windsor (MacNeill et al., Citation2012), and six cities (Baltimore, MD, Chicago, IL, Forsyth, GA, Los Angeles, CA, New York, NY, and St. Paul, MN) in the United States for the Multi-Ethnic Study of Atherosclerosis and Air Pollution (MESA Air) (Allen et al., Citation2012). This is due to larger windows and more frequent window opening being associated with greater bulk flow of air from outdoors to indoors and thus higher Finf values. However, no variable related to opening windows was identified as a predictor in the heating season. This result could be partially explained by the short window opening time and small window opening areas in the heating session, as mentioned earlier.
The use of an air conditioner was also found to be significantly related to lower PM2.5 Finf in the nonheating season. This finding was not unexpected, given that people usually close all the windows when an air conditioner is in use in order to save energy, according to the information from questionnaires. Meng et al. (Citation2009) reported the mean air exchange rate was 0.5 hr−1 for homes where a central air conditioning system was in operation, while the corresponding value was 1.3 hr−1 for homes where it was not. In addition, air conditioning was also reported to increase indoor PM2.5 deposition by filtering process (Howard-Reed et al., Citation2003). The same effect of air conditioner use on Finf was also reported in several other studies (Allen et al., Citation2012; Clark et al., Citation2010; MacNeill et al., Citation2012).
Outdoor temperature was the only variable that was significantly associated with PM2.5 Finf in both the heating and nonheating seasons. It was interesting that the directions of association were contrary in the two sampling sessions. This opposite relationship could be explained as follows. In the nonheating season, the inhabitants in Beijing tended to increase window opening for ventilation with the increase of temperature ranging from 9.4 to 27.9ºC in sampling periods, and thus increased temperatures are associated with greater PM2.5 Finf. Similarly, Allen et al. (Allen et al., Citation2012; Hystad et al., Citation2009) also concluded that outdoor temperatures provided additional information on window opening behavior beyond what was captured by the questionnaire. However, in the heating season, outdoor PM2.5 intruded indoors predominantly via infiltration through cracks and leaks in the building envelope, since the windows were almost always closed. Differences between the indoor and outdoor temperatures were one of the most important driving forces of infiltration (Breen et al., Citation2014; Linden, Citation1999; Shi et al., Citation2015). Decreases in outdoor temperature in addition to the existence of indoor central heating systems resulted in an increase in the temperature difference between indoor and outdoor environments, which could contribute to PM2.5 infiltration.
There are several limitations of this study. First, the low determination coefficient (R2) of the predictive model in the heating season suggested that more influential factors should be investigated and included into model development in future studies in Beijing. Second, the Finf models were developed from a limited number of monitoring events and need to be validated using an independent Finf sample. Third, this is a cross-sectional study where homes were monitored one or two times, and therefore it is difficult to detect the variability of PM2.5 Finf within the home between days and to explore the influence of occupants’ daily behavior. A study with longitudinal design where a smaller number of households are sampled more frequently is recommended for future studies.
Conclusion
The residential PM2.5 Finf in Beijing was investigated for both the nonheating and heating seasons in this study. In general, the average PM2.5 Finf for Beijing residences in both seasons was relatively higher compared to other regions, presumably due to frequent window opening in the nonheating season and poor residential construction in the heating season. High variations of PM2.5 Finf between the two seasons and across homes within each season were also found, highlighting the importance of including Finf in assessment of exposure to PM2.5 of outdoor origin in epidemiological studies. The localized predictive models for residential PM2.5 Finf in Beijing were also developed in this study. The variables identified in this predictive model, such as outdoor temperature, window opening behavior, and air conditioner use, could explain most of the Finf variation among residences for the nonheating season but not for the heating season. The PM2.5 Finf estimates and predictive models developed in this study hold promise for incorporating PM2.5 Finf into larger epidemiological studies, thereby reducing exposure misclassification.
Funding
This study was supported by the Gong-Yi Program of Chinese Ministry of Environmental Protection (201209008), Gong-Yi Program of Chinese Health and Family Planning Commission (201402022), and Natural Science Foundation of Beijing (L150011).
Additional information
Funding
Notes on contributors
Chunyu Xu
Chunyu Xu, Na Li, Yibing Yang, Yunpu Li, Zhe Liu, and Qin Wang are research scientists in the National Institute of Environmental Health, Chinese Center for Disease Control and Prevention, Beijing, People’s Republic of China.
Na Li
Chunyu Xu, Na Li, Yibing Yang, Yunpu Li, Zhe Liu, and Qin Wang are research scientists in the National Institute of Environmental Health, Chinese Center for Disease Control and Prevention, Beijing, People’s Republic of China.
Yibing Yang
Chunyu Xu, Na Li, Yibing Yang, Yunpu Li, Zhe Liu, and Qin Wang are research scientists in the National Institute of Environmental Health, Chinese Center for Disease Control and Prevention, Beijing, People’s Republic of China.
Yunpu Li
Chunyu Xu, Na Li, Yibing Yang, Yunpu Li, Zhe Liu, and Qin Wang are research scientists in the National Institute of Environmental Health, Chinese Center for Disease Control and Prevention, Beijing, People’s Republic of China.
Zhe Liu
Chunyu Xu, Na Li, Yibing Yang, Yunpu Li, Zhe Liu, and Qin Wang are research scientists in the National Institute of Environmental Health, Chinese Center for Disease Control and Prevention, Beijing, People’s Republic of China.
Qin Wang
Chunyu Xu, Na Li, Yibing Yang, Yunpu Li, Zhe Liu, and Qin Wang are research scientists in the National Institute of Environmental Health, Chinese Center for Disease Control and Prevention, Beijing, People’s Republic of China.
Tongzhang Zheng
Tongzhang Zheng is a professor of environmental epidemiology and Anna Civitarese is an MPH in the School of Public Health, Brown University, Providence, RI.
Anna Civitarese
Tongzhang Zheng is a professor of environmental epidemiology and Anna Civitarese is an MPH in the School of Public Health, Brown University, Providence, RI.
Dongqun Xu
Dongqun Xu is a research professor of environmental health in the National Institute of Environmental Health, Chinese Center for Disease Control and Prevention, Beijing, People’s Republic of China.
References
- Allen, R., T. Larson, L. Sheppard, L. Wallace, and L.-J.S. Liu. 2003. Use of real-time light scattering data to estimate the contribution of infiltrated and indoor-generated particles to indoor air. Environ. Sci. Technol. 37(16):3484–92.
- Allen, R.W., S.D. Adar, E. Avol, M. Cohen, C.L. Curl, T. Larson, L. Sally Liu, L. Sheppard, and J.D. Kaufman. 2012. Modeling the residential infiltration of outdoor PM2.5 in the Multi-Ethnic Study of Atherosclerosis and Air Pollution (MESA Air). Environ. Health Perspect. 120(6):824–30. doi:10.1289/ehp.1104447
- Barn, P., T. Larson, M. Noullett, S. Kennedy, R. Copes, and M. Brauer. 2008. Infiltration of forest fire and residential wood smoke: An evaluation of air cleaner effectiveness. J. Expos. Sci. Environ. Epidemiol. 18(5):503–11. doi:10.1038/sj.jes.7500640
- Breen, M.S., B.D. Schultz, M.D. Sohn, T. Long, J. Langstaff, R. Williams, K. Isaacs, Q.Y. Meng, C. Stallings, and L. Smith. 2014. A review of air exchange rate models for air pollution exposure assessments. J. Expos. Sci. Environ. Epidemiol. 24(6):555–63. doi:10.1038/jes.2013.30
- Chen, C., and B. Zhao. 2011. Review of relationship between indoor and outdoor particles: I/O ratio, infiltration factor and penetration factor. Atmos. Environ. 45(2):275–88. doi:10.1016/j.atmosenv.2010.09.048
- Clark, N.A., R.W. Allen, P. Hystad, L. Wallace, S.D. Dell, R. Foty, E. Dabek-Zlotorzynska, G. Evans, and A.J. Wheeler. 2010. Exploring variation and predictors of residential fine particulate matter infiltration. Int. J. Environ. Res. Public Health 7(8):3211–24. doi: 10.3390/ijerph7083211
- Dockery, D.W., C.A. Pope, X. Xu, J.D. Spengler, J.H. Ware, M.E. Fay, B.G. Ferris, Jr., and F.E. Speizer. 1993. An association between air pollution and mortality in six US cities. N. Engl. J. Med. 329(24):1753–9. doi:10.1056/NEJM199312093292401
- Dominici, F., A. McDermott, S.L. Zeger, and J.M. Samet. 2003. National maps of the effects of particulate matter on mortality: Exploring geographical variation. Environ. Health Perspect. 111(1):39–44.
- Hänninen, O., E. Lebret, V. Ilacqua, K. Katsouyanni, N. Künzli, R.J. Srám, and M. Jantunen. 2004. Infiltration of ambient PM 2.5 and levels of indoor generated non-ETS PM 2.5 in residences of four european cities. Atmos. Environ. 38(37):6411–23. doi:10.1016/j.atmosenv.2004.07.015
- Howard-Reed, C., L.A. Wallace, and S.J. Emmerich. 2003. Effect of ventilation systems and air filters on decay rates of particles produced by indoor sources in an occupied townhouse. Atmos. Environ. 37(38):5295–306. doi:10.1016/j.atmosenv.2003.09.012
- Huang, L., P.K. Hopke, W. Zhao, and M. Li. 2015. Determinants on ambient PM 2.5 infiltration in non-heating season for urban residences in Beijing: Building characteristics, interior surface coverings and human behavior. Atmos. Pollut. Res. 6(6):1046–54. doi:10.1016/j.apr.2015.05.009
- Hystad, P.U., E.M. Setton, R.W. Allen, P.C. Keller, and M. Brauer. 2009. Modeling residential fine particulate matter infiltration for exposure assessment. J. Expos. Sci. Environ. Epidemiol. 19(6):570–9. doi:10.1038/jes.2008.45
- Kearney, J., L. Wallace, M. MacNeill, X. Xu, K. VanRyswyk, H. You, R. Kulka, and A.J. Wheeler. 2011. Residential indoor and outdoor ultrafine particles in Windsor, Ontario. Atmos. Environ. 45(40):7583–93. doi:10.1016/j.atmosenv.2010.11.002
- Kearney, J., L. Wallace, M. MacNeill, M.-E. Héroux, W. Kindzierski, and A. Wheeler. 2014. Residential infiltration of fine and ultrafine particles in Edmonton. Atmos. Environ. 94:793–805. doi:10.1016/j.atmosenv.2014.05.020
- Klepeis, N.E., W.C. Nelson, W.R. Ott, J.P. Robinson, A.M. Tsang, P. Switzer, J.V. Behar, S.C. Hern, and W.H. Engelmann. 2001. The National Human Activity Pattern Survey (NHAPS): A resource for assessing exposure to environmental pollutants. J. Expos. Anal. Environ. Epidemiol. 11(3):231–52. doi:10.1038/sj.jea.7500165
- Koutrakis, P., S.L. Briggs, and B.P. Leaderer. 1992. Source apportionment of indoor aerosols in Suffolk and Onondaga counties, New York. Environ. Sci. Technol. 26(3):521–7. doi:10.1021/es00027a012
- Landis, M.S., G.A. Norris, R.W. Williams, and J.P. Weinstein. 2001. Personal exposures to PM 2.5 mass and trace elements in Baltimore, MD, USA. Atmos. Environ. 35(36):6511–24. doi:10.1016/S1352-2310(01)00407-1
- Leaderer, B.P., L. Naeher, T. Jankun, K. Balenger, T.R. Holford, C. Toth, J. Sullivan, J.M. Wolfson, and P. Koutrakis. 1999. Indoor, outdoor, and regional summer and winter concentrations of PM10, PM2. 5, SO4 (2)-, H+, NH4+, NO3-, NH3, and nitrous acid in homes with and without kerosene space heaters. Environ. Health Perspect. 107(3):223–31. doi:10.1289/ehp.99107223
- Linden, P.F. 1999. The fluid mechanics of natural ventilation. Annu. Rev. Fluid Mechanics 31(1):201–38. doi:10.1146/annurev.fluid.31.1.201
- MacNeill, M., L. Wallace, J. Kearney, R. Allen, K. Van Ryswyk, S. Judek, X. Xu, and A. Wheeler. 2012. Factors influencing variability in the infiltration of PM2.5 mass and its components. Atmos. Environ. 61:518–32.
- MacNeill, M., J. Kearney, L. Wallace, M. Gibson, M. Héroux, J. Kuchta, J. Guernsey, and A. Wheeler. 2014. Quantifying the contribution of ambient and indoor‐generated fine particles to indoor air in residential environments. Indoor Air 24(4):362–75. doi:10.1111/ina.12084
- Meier, R., C. Schindler, M. Eeftens, I. Aguilera, R.E. Ducret-Stich, A. Ineichen, M. Davey, H.C. Phuleria, N. Probst-Hensch, and M.-Y. Tsai. 2015. Modeling indoor air pollution of outdoor origin in homes of Sapaldia subjects in Switzerland. Environ. Int. 82:85–91. doi:10.1016/j.envint.2015.05.013
- Meng, Q.Y., B.J. Turpin, A. Polidori, J.H. Lee, C. Weisel, M. Morandi, S. Colome, T. Stock, A. Winer, and J. Zhang. 2005. PM2.5 of ambient origin: Estimates and exposure errors relevant to PM epidemiology. Environ. Sci. Technol. 39(14):5105–12. doi:10.1021/es048226f
- Meng, Q.Y., D. Spector, S. Colome, and B. Turpin. 2009. Determinants of indoor and personal exposure to PM 2.5 of indoor and outdoor origin during the Riopa study. Atmos. Environ. 43(36):5750–8.doi:10.1016/j.atmosenv.2009.07.066
- Pope, C.A. III, and D.W. Dockery. 2006. Health effects of fine particulate air pollution: Lines that connect. J. Air Waste Manage. Assoc. 56(6):709–42. doi:10.1080/10473289.2006.10464485
- Robinson, J., and W. Nelson. 1995. National human activity pattern survey data base. U.S. EPA, Research Triangle Park, NC.
- Sangiorgi, G., L. Ferrero, B. Ferrini, C. Lo Porto, M. Perrone, R. Zangrando, A. Gambaro, Z. Lazzati, and E. Bolzacchini. 2013. Indoor airborne particle sources and semi-volatile partitioning effect of outdoor fine PM in offices. Atmos. Environ. 65:205–14. doi:10.1016/j.atmosenv.2012.10.050
- Sarnat, S.E., B.A. Coull, P.A. Ruiz, P. Koutrakis, and H.H. Suh. 2006. The influences of ambient particle composition and size on particle infiltration in Los Angeles, CA, residences. J. Air Waste Manage. Assoc. 56(2):186–96. doi:10.1080/10473289.2006.10464449
- Shi, S., C. Chen, and B. Zhao. 2015. Air infiltration rate distributions of residences in Beijing. Build. Environ. 92:528–37. doi:10.1016/j.buildenv.2015.05.027
- Talbot, N., L. Kubelova, O. Makes, M. Cusack, J. Ondracek, P. Vodička, J. Schwarz, and V. Zdimal. 2016. Outdoor and indoor aerosol size, number, mass and compositional dynamics at an urban background site during warm season. Atmos. Environ. 131:171–84. doi:10.1016/j.atmosenv.2016.01.055
- Wallace, L., and R. Williams. 2005. Use of personal-indoor-outdoor sulfur concentrations to estimate the infiltration factor and outdoor exposure factor for individual homes and persons. Environ. Sci. Technol. 39(6):1707–14.doi:10.1021/es049547u