ABSTRACT
Today’s heavy-duty natural gas–fueled fleet is estimated to represent less than 2% of the total fleet. However, over the next couple of decades, predictions are that the percentage could grow to represent as much as 50%. Although fueling switching to natural gas could provide a climate benefit relative to diesel fuel, the potential for emissions of methane (a potent greenhouse gas) from natural gas–fueled vehicles has been identified as a concern. Since today’s heavy-duty natural gas–fueled fleet penetration is low, today’s total fleet-wide emissions will be also be low regardless of per vehicle emissions. However, predicted growth could result in a significant quantity of methane emissions. To evaluate this potential and identify effective options for minimizing emissions, future growth scenarios of heavy-duty natural gas–fueled vehicles, and compressed natural gas and liquefied natural gas fueling stations that serve them, have been developed for 2035, when the populations could be significant. The scenarios rely on the most recent measurement campaign of the latest manufactured technology, equipment, and vehicles reported in a companion paper as well as projections of technology and practice advances. These “pump-to-wheels”(PTW) projections do not include methane emissions outside of the bounds of the vehicles and fuel stations themselves and should not be confused with a complete wells-to-wheels analysis. Stasis, high, medium, and low scenario PTW emissions projections for 2035 were 1.32%, 0.67%, 0.33%, and 0.15% of the fuel used. The scenarios highlight that a large emissions reductions could be realized with closed crankcase operation, improved best practices, and implementation of vent mitigation technologies. Recognition of the potential pathways for emissions reductions could further enhance the heavy-duty transportation sectors ability to reduce carbon emissions.
Implications: Newly collected pump-to-wheels methane emissions data for current natural gas technologies were combined with future market growth scenarios, estimated technology advancements, and best practices to examine the climate benefit of future fuel switching. The analysis indicates the necessary targets of efficiency, methane emissions, market penetration, and best practices necessary to enable a pathway for natural gas to reduce the carbon intensity of the heavy-duty transportation sector.
Introduction
Domestic production of natural gas (NG) has surpassed 24 trillion cubic feet per year (Energy Information Administration [EIA], Citation2014). Due to the increased availability and low cost (relative to diesel fuel) of NG from traditional, tight shale and renewable sources, it is now being offered as an alternative fuel for heavy-duty (HD) transportation (Bennick, Citation2013). With a lower carbon content per unit of energy than diesel fuel, switching from diesel to NG could provide a climate benefit in reduced carbon dioxide (CO2) emissions. However, NG vehicles and their associated fueling infrastructure release unburned methane into the atmosphere. Methane is a substantially more potent greenhouse gas (GHG) than CO2, and hence leakage of only a small percentage of methane from the supply chain could alter the net climate benefits of NG as a HD vehicle fuel (Alvarez et al., Citation2012; Brandt et al., Citation2014). For reference, the Intergovernmental Panel on Climate Change (IPCC) proposed a methane global warming potential (GWP) of 34 compared with CO2 over a 100-yr period and 86 over a 20-yr period (U.S. Environmental Protection Agency [EPA], 2016; IPCC, Citation2013). If overall methane emissions were sufficiently low or if the gas were certified as an ultra-low-carbon fuel (sometimes referred to as renewable NG, such as biomethane), then potential GHG advantages (from methane’s lower carbon content) exist from increased adoption of NG as a HD transportation fuel (California Energy Commissions [CEC], Citation2007; Cucchiella et al., Citation2015).
Studies supported by the Environmental Defense Fund (EDF) and industry have addressed methane emissions from the production, transmission, distribution, storage, and processing of NG (Allen et al., Citation2013; EDF, Citation2014; Harrison et al., Citation2011). Significant work continues to expand on these studies to understand more clearly methane emissions of the oil and gas sector (Kleinman et al., Citation2016; Thompson et al., 2016; Kuo et al., Citation2015), as well as air pollutants associated with gas production (Roy et al., 2013). It is also important to note that methane emissions are caused by other activities such as operation of landfills and through mining of coal (Figueroa et al., Citation2009; Kirchgessner et al., Citation2000).
Our program goal was targeted solely at methane emissions from the HD transportation sector. The scenarios that we report here address methane emissions from the fueling infrastructure for HD vehicles and from the vehicles themselves, without consideration of sources upstream of the fueling station that were the focus of other studies (Brandt et al., Citation2014; EDF, Citation2014). Methane has often been excluded from emissions analysis, as the earliest of regulations focused on higher hydrocarbons due to their contribution to smog (Kamens and Stern, Citation1973). Only recent studies have focused on unregulated GHG emissions from current-technology vehicles, and those focused specifically on tailpipe-only emissions (Thiruvengadam et al., Citation2016). In fact, Argonne National Laboratory reported, “Limited publicly available data on total vehicle (or crankcase) methane emissions are available” (Cai et al., 2015). Given that the current percentage of the NG HD fleet and infrastructure is small, but growth predictions are high, this study focused on methane emissions from recent technologies and current practices, which could provide insight into future methane emissions predictions.
Future scenarios were developed with a target date two decades ahead (2035), when HD fleet growth projections suggest that NG vehicles may represent half of the fleet (American Clean Skies Foundation [ACSF], Citation2013; EIA, Citation2013; National Petroleum Council [NPC], Citation2012; Americas Commercial Transportation Research Company, LLC [ACTRC], 2012). Given the emphasis on future fleet projections, this study focused on technology currently being manufactured, as these technologies are the best surrogates for future NG HD fleet makeup. Hence, no attempt was made to produce a current inventory of NG HD vehicle and fueling station emissions, where both old and recent technologies are in use. The insights provided by the measurements made in this study and the projections provided by the scenarios are timely because they provide information to industry and other interested parties as to what technology and other improvements could be important to reduce methane emissions and improve the climate benefits of a future NG HD fleet.
We provide additional details in the Supplemental Information Document (SID) to augment material in this paper, including model overviews, data processing, demonstrations, and case studies. Details on the methods and results of the measurement campaign are reported in a previous paper (Clark et al., Citation2016).
Methodology
New methane emissions data
GHG emissions, including CO2 and methane, can be estimated for compressed natural gas (CNG) and liquefied (LNG) vehicles using models such as Greenhouse Gases, Regulated Emissions, and Energy Use in Transportation (GREET; Cai et al., Citation2015). Prior to GREET 2016, methane emissions data were not updated to reflect most recent vehicle technologies (Wang and Burnham, Citation2016). In GREET 2015, upstream components from the wells-to-pump portion had been updated based on recent EDF studies (Burnham, Citation2016). In GREET 2016, the vehicle emissions factors were updated based on recent testing and certification data for 2010 and newer vehicles that included both crankcase and exhaust components. However, these data were still limited and did not include emissions sources beyond the crankcase and tailpipe, which were included in the pump-to-wheels (PTW) study (Clark et al., Citation2016). These new PTW measurements and modeled data included in-use and chassis testing of 22 vehicles, audits of eight CNG stations and six LNG stations, and long-term pressure rise data from two LNG station tanks and two LNG vehicle tanks. A summary of these average data is presented in . We note that the standard deviations are large compared with the average values. Future work should be completed to increase sample sizes to better understand the distribution of the data and identify any possible outliers or “fat tails.” Since these new data are the best to date, we used the arithmetic means within the scenario models. Note that these PTW studies focus on the fuel-specific methane emissions (FSME; mass of methane emitted per mass of methane consumed as fuel) once the fuel arrives at the stations by LNG tanker or by natural gas pipeline for use as a transportation fuel. A fuel wells-to-wheels (WTW) analysis would require information regarding the methane losses from the well to the “fenceline” of the stations. Upstream data and analyses are available in the literature and can be combined with PTW data to yield an overall methane loss.
Table 1. Methane emissions rates for the PTW portion of the HD NG transportation sector (Clark et al., Citation2016).
Emissions estimation model
Similar to the approach taken by the IPCC, we developed scenarios in this study as a means to evaluate future methane emissions in the NG HD fleet, which are the product of complex dynamic systems (IPCC, Citation2000). The scenarios should be considered as alternative images of how the future might unfold and provide a tool to evaluate how plausible and practicable technology and other improvements could affect future methane emissions profiles in the NG HD fleet and associated infrastructure. As the IPCC notes, any scenario necessarily includes subjective elements and is open to various interpretations. We developed the scenarios presented herein through collaboration with a technical advisory panel (TAP) consisting of industry experts, see SID S8. Industry insight and published literature were combined with the measurements made in our study (Clark et al., Citation2016) () to develop a PTW methane emissions estimation model. represents a schematic overview of numerous submodels, which were developed as part of this study. A more detailed discussion of the model components is presented in the SID S1, S3, and S5.
Figure 1. PTW methane emissions estimation model. See the SID for more details (Mongold, Citation2015).
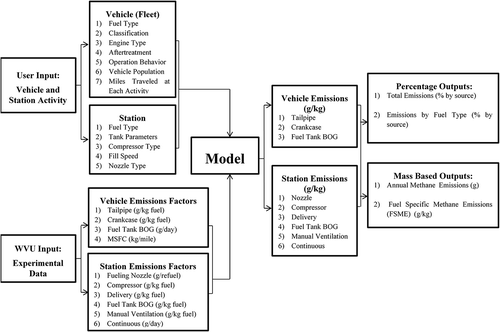
The methane emissions estimation model consists of a spreadsheet and database that includes predefined emissions data collected from the PTW measurement campaigns. Users are able to make selections and input data regarding vehicle type, population, and activity behavior. Note that emissions data presented for vehicles in represent the equally weighted arithmetic means of each vehicle activity type. However, the scenarios modeled in this paper used more granular vehicle activity data that were binned into idle, city, arterial, and highway modes of operation instead of single averages presented in . See Clark et al. (Citation2016) for information regarding the variation in vehicle, crankcase, and vent emissions as a function of activity type.
The model calculates and reports the vehicle and station emissions separately, although they are directly a function of each other, and the model employs the basis of vehicle population demographics and activity. Our model outputs include the percentages of emissions by component source or by fuel type (LNG or CNG). The model also reports the total annual methane emissions (g) for the given input scenario, along with the FSME in g/kg. The model can calculate the methane emissions for any scenario input. Of particular interest, the model can be used to examine relative contributions based on different market penetrations of the various vehicle types. We used the model as a tool to estimate the methane emissions using the scenarios developed in collaboration with the TAP. Model validation was completed and reviewed by the authors and advisory panels. Details of the development and validation have been presented in an academic thesis (Mongold, Citation2015).
Scenarios
Stasis, high, medium, and low
Any of our future scenarios will include uncertainties due to measurement uncertainties of the PTW data and statistical uncertainty of future projections—which include uncertainties in fleet and infrastructure growth, technology advancement, and fleet demographics. To address uncertainty indirectly, we developed four different scenarios to represent the scenario without future technology or practice advances (stasis), the expected average (medium), and the range of possibilities (low and high). To estimate the fraction of methane emitted, each scenario required assumptions about the relative size and makeup of future fleets, the energy efficiency of vehicles, and future emissions rates. shows the four scenarios that we examined. The medium populations assume medium forecasted growth, the high-pressure direct injection (HPDI) market penetration assumes the medium penetration of HPDI vehicles into over-the-road (OTR) trucks, and fuel consumption values range from stasis to low, where high represents a slight improvement in fuel economy whereas low represents the best predicted fuel economy or the lowest specific fuel consumption. The future emissions rates are relative to the stasis rates so that the case of low emissions rates would represent the largest specific reductions in methane emissions. Given the variables of fleet population and mix, fuel economy, and future emissions rates, 144 scenario combinations could be developed. Some additional sensitivity analyses are presented in the SID and are briefly discussed below, but we focused on future emissions rates and fuel economy, as these are important in guiding future technology development and best practices necessary to ensure methane mitigation.
Table 2. Variable selections for our stasis, high, medium, and low scenarios.
These methane emissions scenarios assumed that the hardware used in 2035 will be associated with a range of standards, technologies, and practices, in part due to the useful life of the hardware. The methane estimation model results are presented for four specific scenarios, but the SID S4 and S6 contain information on additional case studies that examined the effects of vehicle population, fleet makeup, and fuel economy. Note that our vehicle population growth scenarios represent three predicted values, but truck count could vary greatly based on trends of traditional fuel prices, availability of NG, industry initiatives, and regulations. No judgment is offered as to probabilities of occurrence of any of the scenarios that are presented, but the authors believe that the scenarios are plausible and practicable expressions of potential future outcomes.
Future estimates of methane emissions by component
A basic premise of the scenarios is that methane emissions will be reduced through technology advances and practice changes, so that emissions will be below the stasis scenario by 2035. These changes may be driven by regulation or by establishing industry best practice programs (Jaffee et al., Citation2003) and are represented in the high, medium, and low methane emissions scenarios described below as percentages of the stasis value, which is set equal to emissions rates with recent technology and practices at the time of the study. Rapid technology turnover, rapid growth of the NG transportation sector, technology breakthroughs, and adoption or enforcement of best practices would all favor the low scenario, whereas a moribund market would favor the high scenario. We worked with the TAP to review the literature and develop our best estimates on potential reductions in methane emissions compared with those reported in (Clark et al., Citation2016). Details of the assumed future emissions rates follow, and summarizes these future emissions estimates. The scenario assumptions were formulated based on clear future technology pathways, effects of adopting best practices, rate of fleet turnover, and effects of increased infrastructure utilization. The assumptions were developed in collaboration with the program’s Technical Advisory Panel with support of the Steering Committee and final review by the independent Scientific Advisory Panel, see SID S8.
LNG delivery losses
The stasis scenario was set at the average measured LNG fuel loss, 0.13% of delivered fuel. There were few study observations, but eliminating the highest emissions event from offloads suggested that the percentage emitted could drop readily from 0.13% to 0.08%. The high value was set to reflect consistent good practice, at 60% of stasis based on the exclusion of the high emitter, which reduced emissions by 40%. Assuming that connector volume emissions could be reduced or captured, medium and low values were 40% and 20% of stasis.
LNG station boil-off
Modeling demonstrated that severe underutilization led to station boil-off, but we did not quantify this effect nationally because utilization rates are not known. Growth of NG transportation will serve to increase station utilization. LNG station modeling shows that a tipping point exists for each station where increases in station use will reduce vent emissions of a station to zero; therefore, 20% of the stasis scenario was used as the high scenario value due to an expected reduction in the number of stations venting. Currently, there are methods to prevent boil-off, use boil-off gas for other purposes, redirect gas to a supply line, or oxidize (burn, catalyze) boil-off gas. Methane emissions from an oxidizer are probably 1% of methane burned. If half of the 2035 stations have boil-off reduction technology, a representative medium value is 10% of today’s value. Assuming full adoption of boil-off abatement, with 95% technology availability, and 1% in combustion exhaust, the low is 2%.
CNG and LNG fuel station continuous emissions
In the scenarios, LNG station continuous emissions were set to zero, as the estimated stasis value was below the third decimal place (0.00007%). For CNG stations of the stasis scenario, all continuous emissions were set at the average estimate of 0.009%. The low scenario assumes that continuous emissions would be reduced through improved practice in eliminating plumbing leaks, through improved component technology, employment of compressed air (versus NG) for pneumatic controls, and by routing vent flows to an oxidizer. However, methane emissions from station leaks are generally low, and inspections are already frequent. The three scenario values were 80%, 50%, and 20% of stasis for CNG stations and were irrelevant for LNG losses.
CNG station compressor losses
CNG stations in this study represented two decades of technologies, and the range of compressor emissions measured suggested that substantial reductions from the average are possible. For the stasis scenario, average fuel-specific compressor emissions of 0.075% were used. Emissions can be captured and recycled or oxidized. However, there may still be old technology compressors in service in 2035. The scenario values chosen were 50%, 20%, and 5% of stasis.
CNG and LNG fueling nozzle losses
Methane emissions from CNG nozzles consist of vent emissions, which could be routed to an oxidizer, and disconnection emissions, which are smaller than vent emissions. For the stasis scenario, CNG nozzle losses were 0.003% and LNG nozzles losses were 0.011% for an estimated 185-kg-tank fill based on measured data. For CNG, scenario values chosen were 50%, 20%, and 10% of stasis. Only new technology LNG nozzles were measured and where again training will be critical to future reductions demonstrated high event variability in methane emissions. The ability to prevent high emissions events through training and technology improvement is critical to future reductions. The scenario values were set at 50%, 20%, and 10% of stasis.
LNG vehicle tank manual vent
Manual venting of vehicle tanks to atmosphere will be reduced by the ability of most LNG stations to accept all return product from a vehicle tank, and through adoption of better practices for fueling where again training will be critical to future reductions. For the stasis scenario, manual venting was estimated to be 0.250% of fuel mass, assuming that 1 in every 20 truck tanks was manually vented, based on observations of venting and measurements of HPDI vehicle tank pressure data prior to fueling. The high scenario value was set at 50% of the stasis scenario, based on better practices and colder stations due to higher utilization. The medium value, 25%, and the low value, 3%, were based on the fraction of stations having boil-off reduction technology, which would aid in accepting returned warm product from trucks.
Crankcase losses
For current engine emissions certification, crankcase methane and tailpipe emissions are measured and regulated together. Future joint regulation could be met with varied combinations of crankcase and exhaust reductions. The stasis scenario values were based on average measured values and all vehicles tested under the PTW campaign that used open crankcases. The technology for closing the crankcase is already available, providing a clear pathway for methane reductions from spark-ignition (SI) engines, and these methods are already being employed on new HD vehicles to meet emissions standards. For the high scenario, it is assumed that the oldest 25% of the fleet would still have open crankcase operation because the timing of a mandated or voluntary closure is unknown. This leads to a high scenario of 25% of the stasis scenario. The medium value was taken as 10%, and the low value was taken as 2% of stasis.
LNG vehicle tank automatic vent
This is a corollary to station boil-off, but some possible station technologies (cooling; running power generators) are not available on-board trucks. For the stasis scenario, it was assumed that 1% of trucks might lose 10% of their fuel from extended periods out of service, so that a fuel fraction loss of 0.1% was adopted in absence of empirical data. Some stationary boil-off reduction technology might be transferrable to mobile sources. Design improvements for LNG tanks and fuel systems, such as improved insulation and reduction in heat loads, will lengthen hold times. The high, medium, and low scenario values selected were 75%, 50%, and 25% of stasis.
HPDI dynamic venting
Stasis scenario values were based on average measured values of the PTW campaign. HPDI engines require venting of the high-pressure fuel rail to balance NG and diesel fueling pressures. This action occurs during transient operation of the engine where the required fuel flow rates of each fuel change suddenly and some NG pressure must be reduced. The HDPI dynamic venting reduction would be dependent on future fuel-system design or capture and use of the vent gas. Scenario values of 50%, 20%, and 10% of stasis were used.
Tailpipe emissions
Historical trends in both diesel and gasoline engine emissions reduction suggest that both in-cylinder and catalyst strategies will be successful for SI and HPDI engines. The 2035 fleet will include older technology that does not achieve emissions reduction levels of the newest vehicles. In addition, at cold temperatures, benefits of advanced catalyst systems may be reduced. Stasis scenario values were based on average measured values of the PTW campaign. The high, medium, and low values were taken as 75%, 35%, and 15%.
Fuel efficiency
It was assumed that less fuel would be used per mile, both through legislation-driven engine efficiency improvements (highly dependent on political climate) and through vehicle efficiency improvements (including drive-train loss, aerodynamic drag, and tire rolling resistance reductions). However, heating catalysts during low power operation will increase fuel use. Reduction of NG fuel use must be compounded with the fuel-specific crankcase, dynamic vent, and tailpipe methane reductions discussed above. For the stasis scenario, an overall efficiency for both methane and diesel was used for HPDI vehicles. Pilot diesel fuel use estimates for HPDI vehicles, provided by the manufacturer, were 10% by energy fraction while under power and 42.5% at idle. For the scenarios, high fuel use (low fuel economy improvement) was combined only with high emissions, medium with medium, and low with low, shown previously in . Penetration of idle reduction and stop-start technology is anticipated and is more easily included as a reduction in fuel use than by adjusting vehicle activity: it is included in the low value for idle. For power operation, the reductions for 2035 were 90%, 75%, and 50% of stasis fuel use. For idle, the values were 100%, 90%, and 60%. Therefore, the stasis scenario assumes no change in fuel economy. The high scenario assumes a slight improvement in fuel economy—yielding relatively high specific fuel consumption compared with the stasis. The low scenario uses the largest fuel economy improvement, thereby yielding a relatively low fuel consumption rate compared with the stasis value.
Vehicle population growth
A literature review of HD NG vehicle population projections was performed to develop population estimates for 2035 scenarios. The U.S. Energy Information Administration (EIA) and National Petroleum Council (NPC) provided NG consumption by HD vehicles and vehicle market shares through 2040, respectively (EIA, Citation2013; NPC, Citation2012). The Americas Commercial Transportation Research Company (ACTRC) and the American Clean Skies Foundation (ACSF) provided HD NG vehicle sales through 2030 and market share of HD vehicles through 2025, respectively (ACTRC, Citation2012; ACSF, Citation2013). In the latter cases, we extrapolated the presented trends to 2035. We used these data to develop a low, a medium, and a high penetration scenario. We determined the low penetration scenario by averaging data reported by EIA and NPC, medium penetration scenario by averaging data reported by all four sources, and the high penetration scenario by averaging data reported by ACTRC and ACFS. shows the 2035 vehicle population assumptions and includes the breakdown between short-haul and long-haul over-the-road (OTR) tractors as well as secondary breakdowns of each type by engine and fuel system. Retrofit vehicles and lean-burn technologies were not included in future populations.
Table 3. Expected future emissions rates.
Table 4. HD NG OTR trucks total market share and distribution scenarios for 2035.
Station populations
includes estimated populations by vehicle type and their relative percentages of market penetration by vehicle type. The percentage values represent the total expected market penetration for each respective vehicle type. For example, the low refuse-truck scenario assumes that NG engines will power 50% of the total refuse-truck population (80,000 out of 160,000 total refuse trucks). The medium and high refuse scenarios assume that the percentage of this vehicle type will increase for the fixed total medium population. It should be noted that all transit buses and refuse trucks were assumed to be powered by SI NG engines with a power less than 320 hp. Background for development of vehicle activity and population scenarios is included in SID S2. The projected population of NG fuel stations for the HD vehicle sector is also included in . Station populations were developed assuming that a station will refuel 50 refuse trucks, 80 transit buses, or 80 OTR trucks per day. The America’s Natural Gas Alliance (ANGA) estimated station growth in 2035 to include 12,100 CNG and 700 LNG stations, respectively (ANGA, Citation2016). However, based on our forecasted population growth and station design requirements, we predicted lower populations of CNG stations and higher populations of LNG stations, due to the high percentage of LNG technology long-haul trucks.
Table 5. Projected HD NG vehicle populations for 2035.
Vehicle miles traveled—microtrips and activity
As presented in the PTW study (Clark et al., Citation2016), we classified vehicle activity as idle, city, arterial, or highway. Annual vehicle miles traveled (AVMT) for individual refuse trucks, transit buses, short-haul OTR trucks, and long-haul OTR trucks were 25,000, 40,000, 50,000, and 100,000 miles, respectively. These values were selected based on those reported in the literature and through discussions with the TAP and fleet operators (APTA, Citation2013; Federal Highway Administration [FHA], 2014). Once these AVMT were selected, a review of the literature was completed to determine the distribution of activity for each vehicle type.
Refuse trucks
To determine the distribution of refuse-truck activity, in-use data collected under the PTW campaign of 26 refuse-truck driving schedules were combined with three chassis dynamometer-driving schedules, three in-use measurements by Texas A&M, and five in-use measurements by North Carolina State University (Sandhu et al., Citation2014; Gokhale, Citation2007). Data segments of in-use data or dynamometer drive cycles were divided based on microtrips for distribution among bin types. See SID S2.1.4 for more details.
Transit buses
We used a similar approach to determine activity distribution for transit buses by examining 12 driving schedules, which included Orange County Transit Agency (OCTA), Washington Metropolitan Area Transit Agency (WMATA), and others described in the SID. All cycles were used as prescribed except for the Knoxville Area Transit (KAT) driving schedule, where we removed 3000 sec of idle that occurred at the end of the cycle (Gao et al., Citation2014). The average velocities without idle in city, arterial, and highway activities of the 12 driving schedules were 6.6, 18.9, and 49.8 miles per hour (mph), respectively. Average velocity of all driving schedules without idle was 17.8 mph. Average velocity of all driving schedules with idle was 12.7 mph, which was consistent with average velocity of U.S. transit bus operation, as reported in the literature (American Public Transportation Association [APTA], Citation2013; Clark et al., Citation2007). See SID S2.1.5 for more details.
OTR trucks
To obtain the average distribution of data for OTR trucks, we reviewed data from five additional studies. One study included the distribution of 15 million miles of urban and rural vehicle activity as collected from engine electronic control modules (ECMs) (Boriboonsomsin et al., Citation2011). Data were processed with MOVES (Motor Vehicle Emissions Simulator) into 16 speed bins, which were further processed to reflect our defined activity types. Oak Ridge National Laboratory completed a similar study collecting real-word highway activity for Class 8 HD OTR trucks—also processed into 16 speed bins (Capps et al., Citation2008). We subsequently reduced these data statistics for our activity types. Huai et al., examined the operation activity characteristics of HD OTR trucks through the analysis of 270 ECM data sets obtained from the California Air Resources Board (Huai et al., 2005). These long-haul and medium-haul activities were dominated by highway operation. Another study presented data on average idle time for long-haul HD OTR trucks (Wagner, Citation2006). The final study examined intercity activity for HD OTR trucks—which included significant idle time (36%) and was applicable to short-haul trucks (Ostria, Citation1996). See SID S2.1.6 for more details.
provides the summary breakdown of AVMT by category and the average idle time in hours per day of operation. Note: To determine idle time, it was assumed that each vehicle type operated for an 8-hr shift. Refuse trucks were assumed to operate 5 days per week and 52 weeks a year, whereas the other vehicle types were assumed to operate 6 days per week and 52 weeks per year.
Table 6. Projected AVMT and idle time by vehicle type.
A note on the stasis scenario
The stasis scenario acknowledges retirement of today’s older technology, such as older nozzle technologies, lean-burn NG engines, and retrofit dual-fuel engines, and uses the medium population and medium HPDI market penetration projections; therefore, it does not represent a present-day methane emissions inventory. Three dual-fuel retrofit vehicles were tested as part of the campaign; however, these vehicles were excluded from future scenarios, as their FSME ranged from 30 to 470 g/kg. In the future, such kits will likely have difficulty meeting new GHG standards. The stasis scenario uses present-day methane emissions factors that were derived from measurements shown in and current fuel economy. Some data sets are small and may not have captured the full range of in-use values. However, there are few data available from current technologies beyond the new data from our recent study.
Results and discussion
The scenario contributions were combined to yield estimates of methane emissions associated with HD NG vehicles, and an estimate of the overall quantity of methane used by vehicles as fuel. Combinations of mixed scenarios (e.g., high, medium, and low) were not considered and our four overall scenarios are presented below.
The 2035 scenario methane emissions estimates are shown in by fuel type and a combination of both CNG and LNG. Stasis, high, medium, and low scenario FSME projections for 2035 were 1.32%, 0.67%, 0.33%, and 0.15% of the natural gas used. It has been estimated that the WTW methane emissions for fuel switching of HD vehicles to offer immediate climate benefit must be less than 1% of the gas consumed (Alvarez et al., Citation2012). With expected technology improvements and best practices, the PTW portion has the potential to be well below this threshold but is dependent on the future WTP emissions rates; see SID S2–S5 for additional information.
Table 7. Methane estimations for CNG, LNG, and their combination for scenarios presented in .
shows the emissions by fuel type, whereas presents the fleet average emissions for each scenario. Emissions associated with vehicles and their operation were significantly higher contributors than station-related emissions for the stasis scenario, representing over 79% of the total emissions. Multiple case studies are presented in SID S5, which includes details on how FSME rates of were developed. In the stasis scenario, the largest contributor to methane emissions was engine crankcases (39% of total emissions), with a rate of 5.16 g/kg or 0.516%. Given that technology has been demonstrated (Cummins-Westport, Citation2015) and with forthcoming regulations, it is likely that crankcase closure will represent the largest reduction potential. For example, the high scenario expects that only 25% of the stasis vehicles will continue to use open crankcases in 2035, and as such, this single action represents nearly 60% of the reduction potential between the stasis and the high scenario—an annual reduction in methane emissions of 0.167 million metric tons (MMT). The low scenario crankcase emissions show that their relative contribution could be reduced by a factor of over 6, even with improved fuel economy.
Figure 2. Future methane emissions for stasis, high, medium, and low scenarios. Each source label is followed by a number representing FSME, in g/kg, and finally by a relative percentage for each component contribution for a given scenario. Note the pie charts show relative contribution—total methane emissions and fuel consumptions are provided in .
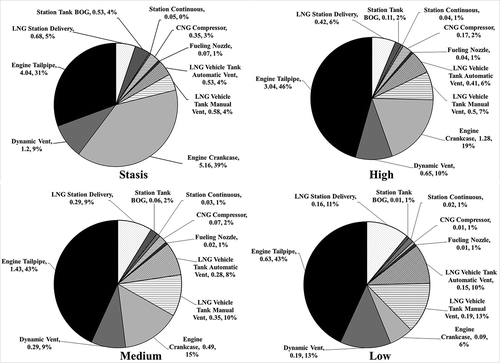
Although total emissions decreased from the stasis to low scenarios (), shows that vehicle tank emissions and manual venting emissions nearly triple in their combined relative contribution (8% for stasis to 23% for low). Best practices regarding vehicle tank venting could be employed currently for immediate benefit, and implementing technologies that eliminate manual venting capabilities or that reuse vented gas should be examined to further reduce their total and relative emissions contributions. The relative contribution of LNG station delivery emissions and HPDI dynamic vent emissions all increase, with a reduction in total emissions. LNG delivery losses were affected by both practice and technology, whereas HPDI dynamic vent emissions reductions will rely solely on technology developments.
In the case of both LNG and CNG stations, increased throughput reduced the relative contribution of methane emissions. Most importantly, for any growth scenario, LNG stations and the fleets they serve should be designed to maximize throughput and any station serving SI vehicles should deliver at least 2000 gallons of fuel per day, whereas stations serving HPDI vehicles, which may return warm vapor, should deliver at least 3000 gallons per day to prevent boil-off. These values are based on a station modeled with a 25,000-gallon storage tank. Station continuous leaks, station tank boil-off gases, CNG compressor losses, and nozzle emissions are all at or below 4% of relative emissions for any scenario. and show examples of sensitivity analyses conducted on various vehicle activity options utilizing the LNG Station model (see SID Section S1.1). For CNG stations, the gross methane emissions from the CNG compressors increases with increased fuel events due to activity and their vent emissions. However, the example shows that for transit buses and OTR tractors, FSME for CNG stations can be reduced by factors of nearly 4 and 3, respectively, by increasing refueling events from 20 to 180 per day. shows a decreasing trend in FSME for LNG vehicles using both HPDI and SI technologies. As expected, HPDI FSME are higher because of the possibility of returning “warm” LNG vapors. Note, however, that increased utilization had only a 4% impact on FSME. So long as the LNG stations meet the daily utilization requirements presented above, additional refueling activity has little beneficial effects.
Figure 3. Reduction in fuel-specific methane emissions from CNG stations with increased vehicle activity/fuel throughput (Mongold, Citation2015).
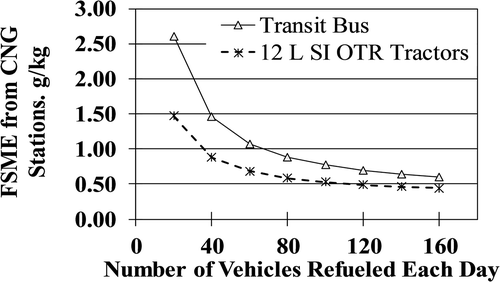
Figure 4. Reduction in fuel-specific methane emissions from LNG stations with increased vehicle activity/fuel throughput (Mongold, Citation2015).
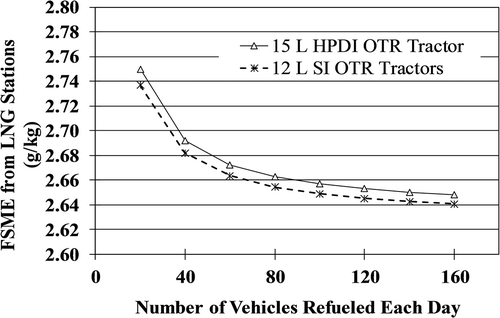
Note on fuel efficiency improvements
Note that reductions in fuel consumption through efficiency improvements can be both beneficial and detrimental from a FSME analysis. For example, the reduction in fuel consumption may reduce the amount of fuel distributed by a CNG station and therefore decrease associated compressor-on emissions, a prominent source of CNG station emissions. However, a reduction in fuel consumption does not affect fuel nozzle emissions per event but does affect the number of refueling events each year. See the SID S5 for additional details on efficiency impacts (National Highway Traffic Safety Administration [NHTSA], Citation2014; Transportation Research Board [TRB], Citation2010) and effects of station utilization on FSME.
Note on flexibility of the model
The methane emissions estimation model can also be used to examine more granular data such as FSME of the different technologies that are currently available under different rates of market penetrations as compared with those data presented for our four scenarios. is an example of a similar analysis for different market penetrations of HPDI vehicles (Mongold, Citation2015). In this case, all variables were selected as medium except for the level of HPDI market penetration. Note that changes in HPDI market penetration only affects long-haul OTR tractors, as short-haul, refuse, and transit buses were all assumed to use SI engines. The high, medium, and low levels of HPDI market penetration were 80%, 50%, and 20%, respectively. The total medium population for OTR tractors was fixed, but the balance between 12-L and 15-L CNG and LNG vehicles was varied based on the HPDI market penetrations to determine annual methane emissions of each engine and fuel type. Such analyses do not change the FSME emissions but could be used for fleets to estimate their annual methane footprint and allow fleet managers to compare technology options that are available to meet their activity demands. Note is presented to show the functionality of the model, but our stasis, high, medium, and low scenarios all assumed a medium HPDI market penetration.
Figure 5. Effects on methane emissions for different levels of HPDI market penetration (Mongold, Citation2015).
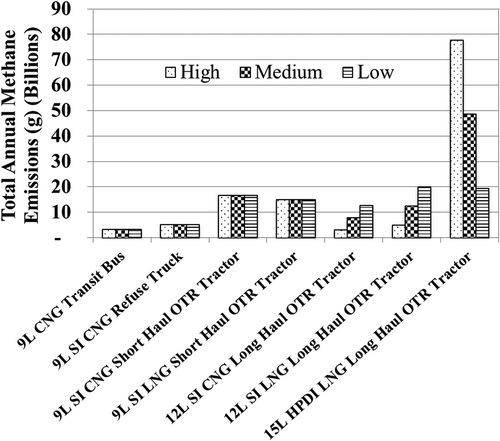
Summary
Natural gas reserves continue to grow, and the literature suggests that the HD NG transportation sector could grow through 2035. However, emissions of methane—the primary component of NG and a potent GHG, must be reduced to ensure climate benefits from increased market penetration of HD NG vehicles. Data on the current inventories, practices, and emissions rates of components within the pump-to-wheels portion of the sector is lacking. We previously collected new emissions measurements for the main components of this sector (Clark et al., Citation2016). These new data were combined with future vehicle population and vehicle distribution projections along with four potential future estimates of methane emissions rates and fuel efficiencies to examine future fleet methane emissions in 2035—when HD NG vehicles could represent a significant portion of the fleet. Our analysis shows that the lowest hanging fruit for future methane emissions reductions is through implementing closed crankcase ventilation systems on HD NG SI engines. Other technologies such as LNG vent oxidation or capture systems are also currently available and should be implemented as the fleet grows. Beyond technological advances, best practices can also reduce methane emissions through reduction in manual LNG tank venting and proper design of station and fleet combinations. In addition to current and new technologies, regulation and policy may lead to further developments that could reduce methane emissions and, when combined with high utilization, could reduce relative methane emissions by an order of magnitude. Our model uses the best data available to develop emissions factors for the various components; however, additional research and measurements should be undertaken in future work.
Key conclusions
Stasis, high, medium, and low scenario total FSME for 2035 were 1.32%, 0.67%, 0.33%, and 0.15%.
Closing the SI engine crankcases offers significant benefit in reducing future methane emissions.
Manual venting of LNG vehicle and station tanks should be avoided regardless of market penetration.
Vehicle-related emissions continue to dominate over station-related emissions so long as stations are properly utilized.
Station utilization is paramount for reducing methane emissions.
FSME can be reduced from CNG stations by factors of 3–4 with increased throughput.
LNG stations must meet minimum throughput requirements to ensure station boil-off is prevented—2000 gallons per day for SI vehicles and 3000 gallons per day for HPDI vehicles for stations with tanks of 25,000 gallons.
Supplemental Information
Download MS Word (7.5 MB)Acknowledgment
The authors acknowledge the support provided by the West Virginia University Transportable Chassis Testing Laboratory personnel, including Drs. Arvind Thiruvengadam and Marc Besch. The authors also thank members of the Technical Advisory Panel, Science Advisory Panel, and Steering Committee for their commitment, expertise, and feedback during this program and the development of our estimation model, see the SID S8 for committee and panel members and associations.
Funding
Support was provided by the Environmental Defense Fund, Cummins, Cummins Westport, Royal Dutch Shell, the American Gas Association, Chart Industries, Clean Energy, the International Council on Clean Transportation, PepsiCo, Volvo Group, Waste Management, and Westport Innovations. Funding for EDF’s methane research series, including the West Virginia University study, is provided for by Fiona and Stan Druckenmiller, Heising-Simons Foundation, Bill and Susan Oberndorf, Betsy and Sam Reeves, Robertson Foundation, Alfred P. Sloan Foundation, TomKat Charitable Trust, and the Walton Family Foundation. Support was also provided by West Virginia University’s George Berry Chair endowment.
Supplemental data
Supplemental data for this article can be accessed on the publisher’s website.
Additional information
Funding
Notes on contributors
Nigel N. Clark
Dr. Nigel Clark is a professor of mechanical engineering at West Virginia University and the provost of West Virginia University’s Institute of Technology.
Derek R. Johnson
Drs. Scott Wayne and Hailin Li are associate professors of mechanical engineering at West Virginia University.
David L. McKain
Dr. Derek Johnson is a research assistant professor of mechanical engineering at West Virginia University.
W. Scott Wayne
Mr. David McKain is a supervisory engineer at West Virginia University’s Center for Alternative Fuels, Engines, and Emissions.
Joseph Rudek
Cesar Sandoval, April Covington, Ronald Mongold, and John Hailer are graduate research assistants at West Virginia University.
Cesar Sandoval
Dr. Joseph Rudek is lead senior scientist at the Environmental Defense Fund.
References
- Allen, D.T., V.M. Torres, J. Thomas, D.W. Sullivan, M. Harrison, A. Hendler, S.C. Herndon, C.E. Kolb, M.P. Fraser, A.D. Hill, B.K. Lamb, J. Miskimisns, R.F. Sawyer, and J. Seinfeld. 2013. Measurements of methane emissions at natural gas production sites in the United States. Proc. Natl. Acad. Sci. U. S. A. 110:17768–73. doi: 10.1073/pnas.1304880110.
- Alvarez, R. A., S. W. Pacala, J. J. Winebrake, W. L. Chameides, and S. P. Hamburg. 2012. Greater focus needed on methane leakage from natural gas infrastructure. Proc. Natl. Acad. Sci. U. S. A. 109:6435–40. doi: 10.1073/pnas.1202407109.
- America’s Natural Gas Alliance (ANGA). 2016. US and Canadian natural gas vehicle market analysis: Natural gas vehicle industry overview. http://www.cngnow.com/Tagged%20PDFs/TIAX_NGVIndustryOverview.pdf (accessed December 27, 2016).
- American Clean Skies Foundation (ACSF). 2013. Driving on natural gas: Fuel price and demand scenarios for natural gas vehicles to 2025. http://www.cleanskies.org/wp-content/uploads/2013/04/driving-natural-gas-report.pdf (accessed December 27, 2016).
- American Public Transportation Association (APTA). 2013. 2013 Public Transportation Fact Book, 64th ed. Washington, DC: American Public Transportation Association.
- Americas Commercial Transportation Research Company, LLC (ACTRC). 2012. The future of natural gas engines in heavy-duty trucks: The diesel of tomorrow? http://www.actresearch.net/wp-content/uploads/2013/04/ACT_NGP.pdf (accessed December 27, 2016).
- Bennick, C. 2013. Natural gas charges into the future: OEM off-highway. http://www.oemoffhighway.com/article/10855398/natural-gas-charges-into-the-future (accessed December 27, 2016).
- Boriboonsomsin, K., G. Scora, G. Wu, and M. Barth. 2011. Improving Vehicle Fleet, Activity, and Emissions Data for On-Road Mobile Sources Emissions Inventories. Riverdale, CA: Center for Environmental Research and Technology, University of California at Riverside. https://www.fhwa.dot.gov/environment/air_quality/conformity/research/improving_data/ (accessed December 27, 2016).
- Brandt, A. R., G. A. Heath, E. A. Kort, F. O’Sullivan, G. Pétron, S. M. Jordaan, P. Tans, J. Wilcox, A. M. Gopstein, D. Arent, S. Wofsy, N. J. Brown, R. Bradley, G. D. Stucky, D. Eardley, and R. Harris. 2014. Methane leaks from North American natural gas systems. Science 343:733–5. doi: 10.1126/science.1247045.
- Burnham, A. 2016. Updated Fugitive Greenhouse Gas Emissions for Natural Gas Pathways in GREET1_2016 Model. Lemont, IL: Argonne National Laboratory. https://greet.es.anl.gov/publication-updated-ghg-2016 (accessed December 27, 2016).
- Cai, H., A. Burnham, W. Hang, and H. Vyas. 2015. The GREET Model Expansion for Well To Wheels Analysis of Heavy-Duty Vehicles. Lemont, IL: Argonne National Laboratories. https://greet.es.anl.gov/publication-heavy-duty (accessed December 27, 2016).
- California Energy Commissions (CEC). 2007. Full fuel cycle assessment: Well-to-wheels energy inputs, emissions, and water impact—State plan to increase the use of nonpetroleum transportation fuel. http://www.energy.ca.gov/2007publications/CEC-600-2007-004/CEC-600-2007-004-F.PDF (accessed December 27, 2016).
- Capps, G., O. Franzese, B. Knee, M. B. Lascurain, and P. Otaduy. 2008. Class-8 Heavy Truck Duty Cycle Project Final Report. Oak Ridge, TN: Oak Ridge National Laboratory. http://cta.ornl.gov/cta/Publications/Reports/ORNL_TM_2008-122.pdf (accessed December 27, 2016).
- Clark, N., D. McKain, D. Johnson, W. S. Wayne, H. Li, V. Akkerman, C. Sandoval, A. Covington, R. Mongold, T. Hailer, and O. Ugarte. 2016. Pump-to-wheels methane emissions from the heavy-duty transportation sector. Environ. Sci. Technol. 51(2):968–76. doi: 10.1021/acs.est.5b06059.
- Clark, N., F. Zhen, S. Wayne, and D. W. Lyons. 2007. Transit Bus Life Cycle Cost and Year 2007 Emissions Estimation Final Report. Transportation Research Board. https://trid.trb.org/view.aspx?id=814667 (accessed December 27, 2016).
- Cucchiella, F., I. D’Adano, and M. Gastaldi. 2015. Profitability analysis for biomethane: A strategic role in the Italian transport sector. Int. J. Energy Econ. Policy 5:440–9.
- Cummins-Westport. 2015. ISL G near zero natural gas engine certified to near zero—First midrange engine in North America to reduce NOx emissions by 90% from EPA 2010. http://www.cumminswestport.com/press-releases/2015/isl-g-near-zero-natural-gas-engine-certified-to-near-zero (accessed December 27, 2016).
- Energy Information Administration (EIA). 2013. Annual energy outlook 2013 with projections to 2040. http://www.eia.gov/forecasts/aeo/pdf/0383(2013).pdf (accessed December 27, 2016).
- Energy Information Administration (EIA). 2014. Natural gas—Data. http://www.eia.gov/naturalgas/ (accessed January 1, 2015).
- Environmental Defense Fund (EDF). 2014. Gathering facts to find climate solutions: An unprecedented look at methane from the natural gas system. http://www.edf.org/sites/default/files/methane_studies_fact_sheet.pdf (accessed December 27, 2016).
- Federal Highway Administration (FHA). 2014. Highway statistics 2013. https://www.fhwa.dot.gov/policyinformation/statistics/2013/vm1.cfm (accessed December 27, 2016).
- Figueroa, V., K. Mackie, N. Guarriello, and C. Cooper. 2009. A robust method for estimating landfill methane emissions. J. Air Waste Manage. Assoc. 59:925–35. doi: 10.3155/1047-3289.59.8.925.
- Gao, Z., T. LaClair, C. Daw, D. Smith, and O. Franzese. 2014. Simulations of the fuel economy and emissions of hybrid transit buses over planned local routes. SAE Int. J. Commer. Veh. 7:216–37. doi: 10.4271/2014-01-1562.
- Gokhale, B. 2007. Application of landfill gas as a liquefied natural gas fuel for refuse trucks in Texas. Master’s dissertation, Texas A&M University, College Station, Texas.
- Harrison, M. R., K. E. Galloway, A. Hendler, T. M. Shires, D. Allen, M. Foss, J. Thomas, and J. Spinhirne. 2011. Natural Gas Industry Methane Emission Factor Improvement Study—Final Report. U.S. Environmental Protection Agency Cooperative Agreement No. XA-83376101. https://dept.ceer.utexas.edu/ceer/GHG/files/FReports/XA_83376101_Final_Report.pdf (accessed December 27, 2016).
- Huai, T., S. D. Shah, J. W. Miller, T. Younglove, D. J. Chernich, and A. Ayala. 2005. Analysis of heavy-duty diesel truck activity and emissions data. Atmos. Environ. 40:2333–4. doi: 10.1016/j.atmosenv.2005.12.006.
- Intergovernmental Panel on Climate Change (IPCC). 2000. IPCC Special Report: Emissions Scenarios—Summary for Policymakers. https://www.ipcc.ch/pdf/special-reports/spm/sres-en.pdf (accessed December 27, 2016).
- Intergovernmental Panel on Climate Change (IPCC). 2013. Working Group I Contribution to the IPCC Fifth Assessment Report Climate Change 2013: The Physical Science Basis. http://www.ipcc.ch/pdf/assessment-report/ar5/wg1/WG1AR5_Chapter08_FINAL.pdf (accessed December 27, 2016).
- Jaffee, A. B., R. G. Newell, and R. N. Stavins. 2003. Technological change and the environment. In Handbook of Environmental Economics, ed. K. Mäler and V. J. Elsevier, Vol. 1, 461–516. Boston: Elsevier Science B.V. doi: 10.1016/S1574-0099(03)01016-7.
- Kamens, R., and A. Stern. 1973. Methane in air quality and automobile exhaust emission standards. J. Air Pollut. Control Assoc. 23:592–6. doi: 10.1080/00022470.1973.10469812.
- Kirchgessner, D., S. Piccot, and S. Masemore. 2000. An improved inventory of methane emissions from coal mining in the United States. J. Air Waste Manage. Assoc. 50:1904–19. doi: 10.1080/10473289.2000.10464227.
- Kleinman, M., G. Mueller, E. Stevenson, R. Alvarez, A. Marchese, and D. Allen. 2016. Emissions from oil and gas operations in the united states and their air quality implications. J. Air Waste Manage. Assoc. 66:1165–70. doi: 10.1080/10962247.2016.1238201.
- Kuo, J., T. Hicks, B. Drake, and T. Chan. 2015. Estimation of methane emissions from california natural gas industry. J. Air Waste Manage. Assoc. 65:844–55. doi: 10.108/10962247.2015.1025924.
- Mongold, R. 2015. Scenario-based estimation model for methane emissions in the heavy-duty transportation sector. Master’s thesis, West Virginia University, Morgantown, West Virginia.
- National Highway Traffic Safety Administration (NHTSA). 2014. Medium- and Heavy-Duty Vehicle Fuel Efficiency Program. http://www.nhtsa.gov/staticfiles/rulemaking/pdf/cafe/Phase-2-HD-Fuel-Efficiency-GHG-Final-Rule.pdf (accessed December 27, 2016).
- National Petroleum Council (NPC). 2012. Advancing Technology for America’s Transportation 2012. http://www.npc.org/reports/trans.html (accessed December 27, 2016).
- Ostria, S. J. 1996. Assessing Emissions Contribution of Intercity Trucking. Transport. Res. Rec. 1520:35–43. doi: 10.3141/1520-05.
- Roy, A., P. Adams, and A. Robinson. 2013. Air pollutant emissions from the development, production, and processing of Marcellus shale natural gas. J. Air Waste Manage. Assoc. 64:19–37. doi: 10.1080/10962247.2013.826151.
- Sandhu, G. S., H. C. Frey, S. Bartelt-Hunt, and E. Jones. 2014. In-use measurement of the activity, fuel use, and emissions of frontloader refuse trucks. Atmos. Environ. 92:557–65. doi: 10.1016/j.atmosenv.2014.04.036.
- Thiruvengadam, A., M. Besch, D. Carder, A. Oshinuga, R. Pasek, H. Hogo, and M. Gautum. 2016. Unregulated greenhouse gas and ammonia emissions from current technology heavy-duty vehicles. J. Air Waste Manage. Assoc. 66:1045–60. doi: 10.1080/10962247.2016.1158751.
- Thompson, T., D. Shepherd, A. Stacy, M. Barna, and B. Schichtel. 2016. Modeling to evaluation contribution of oil and gas emissions to air pollution. J. Air Waste Manage. Assoc. 67:445–61. doi: 10.1080/10962247.2016.1251508.
- Transportation Research Board (TRB). 2010. Technologies and Approaches to Reducing the Fuel Consumption of Medium- And Heavy-Duty Vehicles. Washington, DC: National Research Council, National Academies Press. https://www.nap.edu/read/12845/chapter/1 (accessed December 27, 2016).
- U.S. Environmental Protection Agency (EPA). 2016. Climate change—Overview of greenhouse gases: Methane emissions. https://www.epa.gov/ghgemissions/overview-greenhouse-gases (accessed December 27, 2016).
- Wagner, F. 2006. Heavy-Duty Truck Idle Reduction Technology Demonstrations. Washington, DC: U.S. Department of Energy. https://www1.eere.energy.gov/vehiclesandfuels/pdfs/program/2005_idle_reduction_status_rpt.pdf (accessed December 27, 2016).
- Wang, M., and A. Burnham. 2016. Personal communication. E-mail correspondence, Argonne National Laboratory.