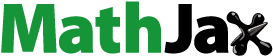
ABSTRACT
Investigation of suspect surface contamination in a building may require comparative sampling across different zones to provide meaningful information with regard to contaminant sources, pathways and/or extent of dispersal. However, evaluation of the data using traditional null hypothesis significance testing (NHST) based upon the mean may result in misleading inference when encountering erratic distributions typical of environmental contaminant data. Sampling data (n = 90) for lead content in surface dust collected throughout a historic building with suspect contamination from uncontrolled disturbance to lead coatings were evaluated using traditional NHST and randomization/permutation inference; the latter metric was the maximum difference in frequency of detection (Δfd max), to directly calculate the probability of the observed differences. In the examples for lead in surface dust presented herein, areas with “lower” mean concentration and/or no significant difference via NHST actually represented “greater contamination,” as Δfd max indicated a greater probability of encountering lead at higher concentrations. Resulting conclusions with regard to sources and pathways contradicted those generated from traditional NHST, and underscore the need to recognize differences in applicability of different inference approaches, depending upon the distribution of the data and the particular problem. This is particularly relevant for forensic purposes.
Implications
The use of permutation/randomization inference to gain insight into sources and pathways of contamination may be more appropriate than the conventional Neyman/Pearson (N/P) logic in negative hypothesis significance testing (NHST). This suggests a broader understanding by environmental professionals of the assumptions and limitations of NHST and alternative inference such as through permutation/randomization is warranted.
Introduction
Environmental/public health investigators not infrequently are faced with developing strategies for assessment of suspect surface contamination in a building or commercial/industrial facility. While there are few if any fixed health-based numerical standards for surface contamination, comparative sampling across different zones in a structure may be utilized to provide meaningful information with regard to contaminant sources, pathways and/or extent of dispersal (Beauchamp, Ceballos, and King Citation2017; Dixon et al. Citation2005, Citation2008; Sanderson et al. Citation2008; Spicer and Gangloff Citation2000). Assessment of the data is almost invariably through traditional environmental/public health statistical methods, driven in varying degrees by the natural concerns for the associated potential health effects from contaminant exposure. Null hypothesis significance testing (NHST) has been the dominant quantitative inference model utilized to manage and assess data through the applied engineering and life sciences in the modern era and is almost invariably incorporated into the design and execution of sampling in these circumstances. However, what is not generally recognized is that NHST is a fluid interfusion of two very different philosophical models with regard to quantitative logic which have not been completely integrated. This has been the source of a long-standing disagreement within the statistical community that is not widely understood or appreciated in the scientific fields that inherently depend upon statistical methods (Agresti Citation2001; Biau, Jolles, and Porcher Citation2010; Chernoff Citation2003; Gliner, Leech, and Morgan Citation2002; Goodman Citation2008; Haggstrom Citation2017; Hubbard and Bayarri Citation2003; Hurlbert and Lombardi Citation2009; Kass Citation2011; Lehmann Citation1993; Sterne Citation2002). The historical derivation of the major aspects of NHST, which has been addressed extensively by others and previously referenced by this author, is relevant to these issues (Spicer and Gangloff Citation2016). A brief overview and summary of the salient points is restated here for appropriate context.
NHST as currently applied was derived through two models originally forwarded by Sir Ronald Fisher on one hand, and the collaboration of Jersey Neyman and Egon Pearson on the other (Kass Citation2011; Lehmann Citation1993; Ludbrook and Dudley Citation1998). The fact that both Fisher’s approach and the Neyman/Pearson (N/P) model can be viewed generally as a “hypothesis test” by which to make some inference regarding the relationship of variables has contributed to either the blurring or disregarding of very fundamental differences in the two approaches. The general logic of both is based on a testable assumption that there is no difference (“null hypothesis”) in variables of interest, customarily represented by the mean as the best measure of central tendency. Thus, an environmental contaminant detected across two comparative data sets is considered noteworthy or “significant” if it can be shown that observed difference occurs randomly at or less than a benchmark frequency (interpreted as a probability). By convention, probability equal to or less than 0.05 has evolved into the criterion most often applied by engineering and environmental/public health practitioners. The aspects and differences between the two models applicable to the assessment of environmental surface contaminants, using lead as an example, is discussed herein.
Fisher forwarded “significance testing” to derive a probability by which to evaluate the results of an experiment. That is, by deriving a value for the random occurrence (denoted p), of an observed result, a researcher would be able to judge the strength of the evidence in the context of background “noise” and as qualified (or limited) by one’s training and experience. Fisher’s inferential logic is the generalized model from the famous experiment to test the claim that the order in which tea and milk were added together influenced the taste of the beverage. By segregating eight (8) test beverages into two (2) equal groups by their treatment and presenting them randomly, Fisher recognized that the task of correctly differentiating the two (2) test groups could be modeled as unordered permutations. Thus, all possible outcomes are determined using the binomial coefficient and the associated probability can be assigned. By representing possible outcomes as a proportion based on the total number of possibilities, Fisher demonstrated that, for example, one could successfully choose at least three (3) out of four (4) beverages correctly, approximately one-fourth of the time (17/70). That is, what might appear to be a notable “success” rate actually could occur through random guessing without even tasting the tea/milk infusion. This underscores one of Fisher’s fundamental emphases that quantitative logic in all circumstances should be based on demonstrable mathematics and not heuristic or “intuitive” sense of data (Fisher Citation[1971a] 2003; Citation[1971b] 2003). Fisher’s logic has alternatively been referred to as the randomization model, in which the random assignment of the variable factor (i.e., order of tea/milk) is a key element in deriving quantitative inference (Ernst Citation2004; Ludbrook and Dudley Citation1998). Describing the approach as “permutation/randomization” (favored by this writer) incorporates Fisher’s use of unordered permutations to directly assign probability to all possible outcomes from the data actually collected.
The N/P model also tests a null of similarity between variables, but unlike the Fisher model, an alternative hypothesis is specified and accepted if the null is rejected. By virtue of the symmetrical nature of the normal distribution, the mean plays a central role as the primary N/P metric for comparison. Similarly, random sampling is a foundational requirement to generate a normal sampling distribution. Otherwise, the validity of the test for the null hypothesis cannot be validated. In contrast, Fisher’s core logic is not dependent upon the mean or random sampling (Fisher Citation[1971c] 2003; Ludbrook Citation1994; Ludbrook and Dudley Citation1998; DiNocera and Ferlazzo Citation2000; Ernst Citation2004; David Citation2008). Additionally, the probability of providing the foundation for inference under N/P denoted α, is derived within a different context than the probability (p) as viewed by Fisher. The conventional 0.05 α “significance level” under N/P is a predetermined probability which implies that repeated random sampling from the population to which the sample is assumed to belong, would produce a difference in means equal to or greater than that exhibited in the particular test at least 0.95 (95 percent) of the time. The N/P model was derived principally for applications in which the costs or consequences of misidentifying a “significant difference” (thus committing an “error”) were the driving factor, as in manufacturing and laboratory quality control (Hubbard and Bayarri Citation2003; Yates Citation1990). Thus N/P establishes probability within the context of an error in the rejection of a null hypothesis, not necessarily whether the hypothesis is actually false (Ernst Citation2004; Hubbard and Bayarri Citation2003; Szucs and Ioannidis Citation2017). The fact that both Fisher and N/P approaches loosely referenced the tentative usefulness of a frequency/probability of 0.05 (p and α, respectively) has resulted in much of the confusion in distinguishing the fundamental differences in logic, and hence the applicability of the two approaches to a particular problem (Biau, Jolles, and Porcher Citation2010; Chernoff Citation2003; David Citation2008; Ernst Citation2004; Hubbard and Bayarri Citation2003; Lehmann Citation1993; Moran and Soloman Citation2002). While a great many problems can be successfully assessed using either N/P or the permutation/randomization approach, there are also instances in which the model chosen can lead to conflicting inference, particularly with small sample sets as frequently occur in environmental investigations (Ludbrook Citation1994; Ludbrook and Dudley Citation1998). The differences in the underlying logic, derivation of the probability of interest, and inference derived under each model are fundamentals which continue to be widely unrecognized and unreconciled in much of the engineering and environmental/public health sciences. The foundational axiom of environmental/public health practice as the “dose makes the poison” inherently incorporates N/P logic. In this instance, standardized sampling and analytical protocols for monitoring exposure can be compared to similar data from a large population by which average exposure to a contaminant is associated with biological markers or health effects. In the case of lead, for example, current EPA and Housing and Urban Development (HUD) risk assessment is derived from the correlation of average surface lead loading to elevated blood lead in children (United States Department of Housing and Urban Development Citation2012; United States Environmental Protection Agency Citation1995). Thus, by extension under this model, one would conclude that an environment with a higher average surface lead loading presents a greater lead exposure risk to the target child population. However as demonstrated herein, determining cause and origin for a ubiquitous contaminant such as lead requires evaluation of comparative environmental data from reference zone(s), rather than a large health-based database. Practically limited in sample sizes and potentially confounded with residual background contamination, the inference model employed to assess differences across characteristically sparse and/or highly erratic distributions becomes paramount (Ludbrook Citation1994; Stewart-Oaten Citation1995; United States Environmental Protection Agency Citation2002).
Methods
Lead in settled dust
A three (3) story (with basement) former courthouse building in a major southeastern metropolitan area provided the opportunity to collect surface dust samples to characterize suspect lead contamination in surface dust. Original construction in the first half of the twentieth century established the structure as historically significant, while major interior renovations in various parts of the building were being undertaken to convert the building to educational use. Miscellaneous and sporadically conducted historic environmental surveys for the site indicated lead in surface paint on some plaster ceilings and/or walls interspersed throughout the building, typical for the era of original construction. Miscommunication of the environmental surveys with regard to lead content in surface coatings resulted in the general contractor mobilizing under the assumption that interior demolition would not impact lead-containing surfaces. As a result, demolition without appropriate environmental controls was conducted for several weeks in the basement. While there was likely airborne exposure to the workers, an additional concern was possible contribution to surface lead loading over background in other areas of the building. To gain insight into the degree of potential dispersal of lead, a building wide surface dust sampling program was undertaken.
The general sampling scheme for lead dust was driven by the building architecture. Within the approximate 55,000 square foot square site footprint, a central two-level courtroom and surrounding courtyard was enclosed by a three-level general office structure. Third floor offices in the east and west wings (approximately 7500 square feet each) had not undergone any recent renovation. Accordingly, the third floor was designated as the Background zone as it was considered to represent historic building conditions prior to the general contractor’s activities. An office area of approximately 1500 square feet along the west side of the second floor and which had not been demolished was designated as zone A. The basement, approximately half of which was unfinished crawlspace, had functional spaces arranged in a “U” along the north, west, and south quadrants. The uncontrolled demolition by the general contractor occurred in an approximate 10,000 square foot area in the basement was a suspect source for lead dispersal into other areas of the building, as well as generating accumulation of contaminated construction debris that remained after demolition was completed in the space. For sampling purposes, the basement was denoted as zone B. An “L” shaped zone of approximately 8500 square feet on the second floor (adjacent to zone A) was designated as zone C. The area had undergone partial demolition of the ceiling with a lead containing paint prior to the general contractor’s mobilization (and prior to the demolition in the basement). On the first floor, an area of approximately 2500 square feet in the southwest corner was converted from original office space into the general contractor’s administrative field office. While historic demolition debris from previous interior renovation prior to the general contractor’s mobilization was present in nearby areas, the administrative space was subjected to light surface cleaning before occupancy by the general contractor’s field staff. The area was designated zone D and was anticipated to be a useful comparative zone with lower lead loading.
Sampling on horizontal surfaces within each test zone was conducted as per ASTM E 1728 Standard Practice for Collection of Settled Dust Samples Using Wipe Sampling Methods for Subsequent Lead Determination, by an EPA certified lead inspector. In this method, a measured area of 0.9688 square feet was demarcated using a 900 square centimeter template, and laboratory supplied, prepackaged sampling media was used to wipe the surface within the defined area in a prescribed pattern. The sampling media was then transmitted to an (accredited) environmental laboratory for analysis via flame atomic absorption spectrometry (EPA Method SW 846 3050B/700B). Lead was reported (as discussed throughout) in micrograms per square foot (ug/ft2) as per standard practice and convention in the lead regulatory and public health communities. summarizes the sampling in each zone.
Table 1. Sample zones.
Data analysis – permutation/randomization-based inference on detection frequency
It can be stated axiomatically that if two comparative sample sets representing different locations in the building are from the same population (assumed to represent the same source), there should not be a significant difference in the number of data points greater than some reference value that is representative of the combined data. This is defined as difference in detection frequency or Δfd and applies regardless of the distribution of the data. Since environmental data are often characterized by very erratic distributions, Δfd becomes a very useful metric due to the limitation of the mean when data deviates substantially from normal. The data point around which the greatest Δfd is calculated is denoted as the “critical reference value” (CRV), which will often, but not in all instances, be the median of the combined data being compared. Thus, the metric is actually the “maximum” Δfd or “Δfdmax,” but is expressed here and historically by this author (and coauthors) as “Δfd” for simplicity. Under permutation/randomization, the mechanics of the direct probability calculation on Δfd have previously been described and are summarized here (Spicer and Gangloff Citation2003, Citation2008, Citation2010, Citation2015, Citation2016). Using the binomial random function (BRF), the probability for each possible frequency of detection (fd) relative to the CRV can be directly calculated. Consider seven (7) surface dust samples collected from a zone which for the sake of example will be designated the control, to be compared quantitatively to a suspect building zone also represented by seven (7) samples. Data for this example are shown in and is a random selection from actual surface data from the third-floor Background zone and second-floor historic demolition zone C further discussed herein.
Table 2. Example data randomly selected from Building zone C (serving as the control) and the Background zone (suspect).
A matrix type calculation (i.e., Excel TM) to determine the probability of Δfd of interest can be conveniently applied. In this case, the value at which the Δfd occurs is the median of the combined data from the two comparative zones or 1185 ug/ft2; this value is therefore the CRV. There are two (2) results in zone C greater than 1185 ug/ft2 whereas five (5) results in the Background zone data are greater than this reference value (1185 ug/ft2). All possible frequencies of detection (fd) for the seven (7) samples in zone C are shown in the second column of , with all possible fd’s for the seven (7) samples in the Background zone indicated in the second row. By convention, the reference or control zone (generically denoted as CZ) is displayed along the vertical axis, while the test zone (TZ) which in this case is the third-floor Background zone is displayed along the horizontal. Thus the calculation is configured to determine whether lead loading in the third floor Background zone is greater than in zone C. By the results of the particular sample set, two (2) detections in zone C equates to an fd of 0.2857 (2/7); the Background zone fd is 0.71434 (5/7). The fd for the combined data is 0.5 (7/14). The probability for each possible fd across the two comparative zones is calculated with the appropriate BRF substitutions, using the combined fd of 0.5 as P. (As this is empirically derived from the experimental data, it is conventionally denoted “P hat” or P̂; P will be used here for convenience of representation.) Thus
Table 3. Randomization/permutation calculation of significance based upon example data; probability values for Δfd equal to or greater than exhibited in the data are bolded/italicized.
where p is the probability of the occurrence
P is the proportion of samples > CRV
Q is the proportion of samples ≤ CRV; (1)
n is the number of samples in the respective zone
x is the number of samples > CRV
nCx is the number of unordered permutations > the CRV;
As an example, the probability for the occurrence of five (5) out of seven (7) detections in the Background zone (fd = 0.7143) is defined by the BRF as (7!/(5!*2!))* (0.500)5 * (0.500)2 = 0.16406 as shown in the bordered/shaded cells of the third row in . The probability values in the body of are derived from the product of the underlying calculated probabilities along the two axes. As can be seen, the matrix representation comports with Fisher’s permutation/randomization concept of identifying and assigning a probability to all possible outcomes in a particular experiment, based on the data actually collected (rather than an assumption of a normal distribution). The probability for all possible Δfd’s greater than or equal to that exhibited (5/7-2/7 = 0.42857) in the data are the bolded-italicized values in the body of , the sum of which produces the probability (p) value for obtaining the observed (or greater) Δfd. (Cells in which no value is indicated correspond to the probability of fd’s that are less than exhibited in the data and are therefore not of interest.) Thus, in the example, the resulting p value under permutation/randomization-based inference is (rounded to) .09
Results and discussion
Surface lead loading data from the different zones in the building are shown in .
Table 4. Lead surface data from five (5) zones within the building.
Six (6) different zone comparisons were generated to gain insight into (a) the general background dust loading in the building (b) dispersion of lead from uncontrolled demolition on the second floor prior to the general contractor’s mobilization, and (c) the impact on building lead burden as a result of uncontrolled demolition conducted in the basement by the general contractor. displays comparison across zones of interest from the data displayed in . The directly calculated probability on Δfd under permutation/randomization inference is identified in the column identified “pTZ>CZ”. Student’s t, the routinely applied statistical test for comparison of means in small samples within traditional NHST, is shown in the last column. Mann–Whitney U is a non parametric NHST equivalent of Student’s t. The statistic tests the hypothesis that two sample sets are from the same population based upon the comparison of the means of the data rankings. Statistical inference using Mann–Whitney is also shown in the last column of .
Table 5. Comparison of lead surface loading across various zones using N/P vs. permutation/randomization inference.
The critical value for t based upon the number of samples for the various comparisons must reach at least 1.699 to represent p = .05 (or less). As can be seen, none of the zone comparisons reached t = 1.699, and thus did not demonstrate a statistical difference via N/P inference. This implies lead surface loading based upon the mean (as applied per the EPA health-based standards) is essentially equal throughout the building. The Mann Whitney, for which the Z statistic of at least 1.645 represents α (probability) of 0.05 (or less), only demonstrated a difference in three (3) of the six (6) comparisons. Thus, only three (3) of the twelve (12) total comparisons done under traditional approaches based on N/P logic (bolded values in the last column of showing probability associated with test zone and control zone comparison) identified a significant difference. Conversely, directly calculated probability for difference in detection frequency (probability the lead loading in the test zone is greater than in the control zone; bolded values under pTZ>CZ), clearly demonstrated a variation in lead surface conditions across the building. Specifically, the lead loading in the third-floor Background zone (most distant from zone B basement demolition) is greater than each of the other zones in the building. Thus, background/historical conditions contributed much more to overall surface lead loading in the building and predated the general contractor’s mobilization to the site. An example of the potential for misleading inference using comparison of mean surface loading data is best shown in the comparison of zones C and A, where mean lead level in zone A is numerically “greater” than the second floor zone C. Axiomatic probability calculated for Δfd indicates lead loading is greater in zone C, which is consistent with the space history of uncontrolled demolition (and suspect lead disturbance) prior to the general contractor’s mobilization.
In a broad view, the Δfd metric under permutation/randomization inference clearly demonstrated significant differences in lead loading throughout the building and was greatest in the third-floor Background zone. In most instances, this was not apparent by inspection of the data and/or by classical mean-based N/P inference. Rather, the data demonstrated a notable background level of surface contamination at locations in the building remote from the basement zone which strongly supports the conclusion that demolition in the basement had little to no impact over background surface contamination throughout the building.
The salient point in this discussion is that the permutation/randomization inference approach is not within the same model by which actual occupational/environmental exposure data are assessed. In the latter, data are appropriately “averaged” over the time and/or body surface area, such as full shift integrated air sampling or dermal patch testing. Deviations from normality in the underlying data are essentially irrelevant (California Department of Environmental Protection Agency Citation2003; Dixon et al. Citation2005, Citation2008; Lange Citation2001; United States Environmental Protection Agency Citation1992). In contrast, the analysis of several discrete environmental samples of limited volume or surface area across comparative zones for the purpose of determining relative differences and inferring likely pathways is inherently dependent upon the distribution of the data. The two potentially different mathematical/statistical problems posed are not often recognized or even acknowledged by many in the technical communities due to historical deference to the “one size fits all” of N/P logic and dependence upon the mean (Gliner, Leech, and Morgan Citation2002; Hubbard and Bayarri Citation2003; Ludbrook Citation1994; Szucs and Ioannidis Citation2017; Yates Citation1990). The data reported herein are consistent with previous published studies that have shown misleading inference based upon the comparison of mean contaminant levels for asbestos and metals in settled dust as well as airborne mold (Johnson et al. Citation2008; Modarres, Gastwirth, and Ewens Citation2005; Spicer and Gangloff Citation2008, Citation2010, Citation2011, Citation2015, Citation2016; United States Environmental Protection Agency Citation1992).
Conclusion
Any environmental data will be defined and limited by the particular parameters and circumstances when collected, and the investigator will be faced with making inference with the available data. In situations where there may not be a specific chemical or environmental “marker” to track contaminant pathways and sources, the statistical/probabilistic aspects dictated by the distribution of the data are paramount in making inferences on the relative prevalence of a particular contaminant across locations. Peremptorily applying traditional N/P logic, irrespective of the underlying sampling and distributional assumptions or applicability to a particular problem, is therefore neither a trivial nor merely an academic argument. The appropriate inference from data to characterize conditions and make conclusions on sources and pathways may pose significant economic as well as occupational/environmental health implications (Beauchamp, Ceballos, and King Citation2017). Challenges to what has been referred to as the “orthodoxy” of N/P inference have emerged in the biomedical and social sciences over the last three decades (Agresti Citation2001; Chernoff Citation2003; Hurlbert and Lombardi Citation2009; Szucs and Ioannidis Citation2017; Yates Citation1990). As shown in the examples herein, a similar need exists in environmental/public health investigations, with a broader recognition that NHST is not the single objective approach to statistical inference as is widely accepted and assumed. At the very least, permutation/randomization on Δfd can serve as verification against traditional N/P inference when the data deviate from normality. This may be particularly relevant in forensic situations where not only should the representativeness of sampling and validity of laboratory analysis be scrutinized but also the inference model utilized to assess the data.
Disclosure statement
No potential conflict of interest was reported by the author.
Additional information
Notes on contributors
R. Christopher Spicer
R. Christopher Spicer is Director of Industrial Hygiene for Gallagher Bassett Technical Services, and is a Certified Industrial Hygienist (CIH) and a Certified Hazardous Materials Manager (CHMM). He has more than thirty five years of experience in environmental consulting involving a variety of general and indoor environmental issues facing the construction, real estate and insurance communities. He currently is a member of ASTM committees on mold and asbestos and has previously published several peer reviewed technical articles on environmental data assessment.
References
- Agresti, A. 2001. Exact inference for categorical data: Recent advances and continuing controversies. Stat. Med. 20:2709–22. doi:10.1002/sim.738.
- Beauchamp, C., D. Ceballos, and B. King. 2017. Lessons learned from surface wipe sampling for lead in three workplaces. J. Occup. Environ. Hyg. 14 (8):609–17. doi:10.1080/15459624.2017.1309047.
- Biau, D. J., B. M. Jolles, and R. Porcher. 2010. P value and the theory of hypothesis testing: An explanation for new researchers. Clin. Orthop. Relat. Res. 468:885–92. doi:10.1007/s11999-0091164-4.
- California Environmental Protection Agency. 2003. Memorandum from S. Powell to J. P. Frank, Worker Health and Safety Branch. Why Worker Health and Safety Branch uses arithmetic means in exposure assessments, September 22.
- Chernoff, H. 2003. A view of past present and future. Indian J. Stat. San Antonio Conf. Sel. Articles 64 (A2):183–94.
- David, H. A. 2008. The beginnings of randomization tests. Am. Stat. 62:70–72. doi:10.1198/000313008X269576.
- DiNocera, F., and F. Ferlazzo. 2000. Resampling approach to statistical inference: Bootstrapping from event-related potentials data. Behav. Res. Methods Instrum. Comput. 32 (1):111–19. doi:10.3758/BF03200793.
- Dixon, S., J. Wilson, C. Kawecki, R. Green, J. Phoenix, W. Galke, S. Clark, and J. Breysse. 2008. Selecting a lead hazard control strategy on dust lead loading and housing condition: Methods and results. J. Occup. Environ. Hyg. 8:530–39. doi:10.1080/15459620802219799.
- Dixon, S. L., J. W. Wilson, C. S. Clark, W. A. Galke, P. A. Succop, and M. Chen. 2005. The influence of common area lead hazards and lead hazard control on dust lead loadings in multiunit buildings. J. Occup. Environ. Hyg. 2:659–66. doi:10.1080/15459620500403737.
- Ernst, M. D. 2004. Permutation methods: A basis for exact inference. Stat. Sci. 19:676–85. doi:10.1214/088342304000000396.
- Fisher, R. A. [1971a] 2003. The principles of experimentation, illustrated by a psycho-social experiment. Chap. II in The design of experiments. 8th ed.,11–25. New York: Haffner Publishing. Reprinted in Statistical methods experimental design and scientific inference. New York: Oxford University Press.
- Fisher, R. A. [1971b] 2003. Introduction. Chap.I in The design of experiments, 8th ed., 1–10. New York: Haffner Publishing. Reprinted in Statistical methods experimental design and scientific inference. New York: Oxford University Press.
- Fisher, R. A. [1971c] 2003. The generalisation of null hypotheses. Fiducial probability. Chap. X in The design of experiments, 8th ed., 184–213. New York: Haffner Publishing. Reprinted in Statistical methods experimental design and scientific inference. New York: Oxford University Press.
- Gliner, J. A., N. L. Leech, and G. A. Morgan. 2002. Problems with null hypothesis significance testing (NHST): What do the textbooks say? J. Exp. Educ. 71:83–92. doi:10.1080/00220970209602058.
- Goodman, S. 2008. A dirty dozen: Twelve p-value misconceptions. Semin. Hematol. 45:135–40. doi:10.1053/j.seminhematol.2008.04.003.
- Haggstrom, O. 2017. The need for nuance in the null hypothesis testing debate. Educ. Psychol. Meas. 77:616–30. doi:10.1177/0013164416668233.
- Hubbard, R., and M. J. Bayarri. 2003. Confusion over measures of evidence (p’s) versus errors (α’s) in classical statistical testing. Am. Stat. 57:171–82. doi:10.1198/0003130031856.
- Hurlbert, S. H., and C. M. Lombardi. 2009. Final collapse of the Neyman-Pearson decision theoretic framework and rise of the neoFisherian. Ann. Zool. Fenici. 46:311–49. doi:10.5737/086.048.0501.
- Johnson, D., D. Thompson, R. Clinkenbeard, and J. Redus. 2008. Professional judgment and interpretation of viable mold air sampling data. J. Occup. Environ. Hyg. 5:656–63. doi:10.1080/15459620802310796.
- Kass, R. E. 2011. Statistical inference: The big picture. Stat. Sci. 26:1–9. doi:10.1214/10-STS337.
- Lange, J. H. 2001. A suggested lead surface concentration standard for final clearance of floors in Commercial and industrial buildings. Indoor Built Environ. 10:48–51. doi:10.1177/1420326X010100010.
- Lehmann, E. L. 1993. The Fisher, Neyman-Pearson theories of testing hypotheses: One theory or two? J. Am. Stat. Assoc. 88:1242–49. doi:10.1080/01621459.1993.10476404.
- Ludbrook, J. 1994. Advantages of permutation (randomization) tests in clinical and experimental pharmacology and physiology. Clin. Exp. Pharmacol. Physiol. 21:673–86. doi:10.1111/j.1440-1681.1994.tb02570.x.
- Ludbrook, J., and H. Dudley. 1998. Why permutation tests are superior to t and F tests in biomedical Research. Am. Stat. 52:127–32.
- Modarres, R., J. L. Gastwirth, and W. Ewens. 2005. A cautionary note on the use of non-parametric tests in the analysis of environmental data. Environmetrics 16:319–26. doi:10.1002/env.695.
- Moran, J. L., and P. Solomon. 2002. Worrying about normality. Crit. Care Resuscitation 4:316–19.
- Sanderson, W. T., S. Leonard, D. Ott, L. Fuortes, and W. Field. 2008. Beryllium surface levels in a Military Ammunition Plant. J. Occup. Environ. Hyg. 5:475–81. doi:10.1080/15459620802131408.
- Spicer, R. C., and H. J. Gangloff. 2000. A probability model for evaluating contaminant data from an environmental event. J. Air Waste Manage. Assoc. 50:1637–46. doi:10.1080/10473289.2000.10464198.
- Spicer, R. C., and H. J. Gangloff. 2003. Bioaerosol data distribution: Probability and implications for sampling in evaluating problematic buildings. Appl. Ind. Hyg. 18:584–90. doi:10.1080/10473220301411.
- Spicer, R. C., and H. J. Gangloff. 2008. Verifying interpretive criteria for bioaerosol data using (bootstrap) Monte Carlo techniques. J. Occup. Environ. Hyg. 5:85–92. doi:10.1080/15459620701804717.
- Spicer, R. C., and H. J. Gangloff. 2010. Differences in detection frequency as a bioaerosol data criterion for evaluating suspect fungal contamination. Build. Environ. 45:1304–11. doi:10.1016/j.buildenv.2009.11.012.
- Spicer, R. C., and H. J. Gangloff. 2011. Implications of error rates associated with numerical criteria for airborne fungal data. Proceedings of Indoor Air 2011, the 12th International Conference on Indoor Air Quality and Climate, Austin, TX 1:398–404.
- Spicer, R. C., and H. J. Gangloff. 2015. The building performance model for evaluating bioaerosol data from suspect indoor environments. Indoor Built Environ. 24:640–49. doi:10.1177/1420326X14535790.
- Spicer, R. C., and H. J. Gangloff. 2016. Permutation/randomization-based inference for environmental data. Environ. Monit. Assess. 188:147. doi:10.1007/s10661-016-5090-0.
- Sterne, J. A. C. 2002. Teaching hypothesis tests-time for a significant change? Stat. Med. 21:985–94. doi:10.1002/sim.1129.
- Stewart-Oaten, A. 1995. Rules and judgments in statistics: Three examples. Ecology 76 (6):2001–09. doi:10.2307/1940736.
- Szucs, D., and J. P. A. Ioannidis. 2017. When null hypothesis significance testing is unsuitable for research: A reassessment. Front. Hum. Neurosci. 11:390. doi:10.3389/inhum.2017.00390.
- United States Department of Housing and Urban Development. 2012. Chap. 5 Risk Assessment in Guidelines for the evaluation and control of lead based paint hazards in housing. 2nd ed. Washington, DC: United States Department of Housing and Urban Development.
- United States Environmental Protection Agency. 1992. Statistical methods for evaluating the attainment of cleanup standards. Volume 3: reference-based standards for soils and solid media. EPA 230-R-94-004. Washington, DC: United States Environmental Protection Agency.
- United States Environmental Protection Agency. 1995. Final report sampling house dust for lead basic concepts and literature review. EPA 747-R-95-007. Washington, DC: United States Environmental Protection Agency.
- United States Environmental Protection Agency. 2002. Guidance for comparing background and chemical concentrations in soil for CERCLA sites. EPA 540-R-01-003 OSWER 9285, 7–4. Washington, DC: United States Environmental Protection Agency.
- Yates, F. 1990. Forward to statistical methods experimental design and scientific inference, vii– xxxii. New York: Oxford University Press.