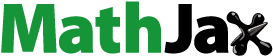
ABSTRACT
In this study, we investigated the association between short-term exposure to different sources of fine particulate matter (PM2.5) and biomarkers of coagulation and inflammation in two different panels of elderly and healthy young individuals in central Tehran. Five biomarkers, including white blood cells (WBC), high sensitive C-reactive protein (hsCRP), tumor necrosis factor-soluble receptor-II (sTNF-RII), interleukin-6 (IL-6), and von Willebrand factor (vWF) were analyzed in the blood samples drawn every 8 weeks from the subjects between May 2012 and May 2013. The studied populations consisted of 44 elderly individuals at a retirement home as well as 40 young adults residing at a school dormitory. Positive Matrix Factorization (PMF)-resolved source-specific PM2.5 mass concentrations and biomarker levels were used as the input to the linear mixed-effects regression model to evaluate the impact of exposure to previously identified PM sources at retirement home and school dormitory in two time lag configurations: lag 1–3 (1–3 days before the blood sampling), and lag 4–6 (4–6 days before the blood sampling). Our analysis of the elderly revealed positive associations of all biomarkers (except hsCRP) with particles of secondary origin in both time lags, further corroborating the toxicity of secondary aerosols formed by photochemical processing in central Tehran. Moreover, industrial emissions, and road dust particles were positively associated with WBC, sTNF-RII, and IL-6 among seniors, while vehicular emissions exhibited positive associations with all biomarkers in either first- or second-time lag. In contrast, most of the PM2.5 sources showed insignificant associations with biomarkers of inflammation in the panel of healthy young subjects. Therefore, findings from this study indicated that various PM2.5 sources increase the levels of inflammation and coagulation biomarkers, although the strength and significance of these associations vary depending on the type of PM sources, demographic characteristics, and differ across the different time lags.
Implications: Tehran, the capital of Iran with a population of more than 9 million people, has been facing serious air pollution challenges as a result of extensive vehicular, and industrial activities in the previous years. Among various air pollutants in Tehran, fine particulate matters (PM2.5, particles with aerodynamic diameters < 2.5 µm) are known as one of the most important critical pollutants, causing several adverse health impacts including lung cancer, respiratory, cardiovascular, and cardiopulmonary diseases. Therefore, a number of studies in the area have tried to investigate the adverse health impacts of exposure to PM2.5. However, no studies have ever been conducted in Tehran to examine the association between specific PM2.5 sources and biomarkers of coagulation and systemic inflammation as indicators of cardiovascular disorders. Indeed, this is the first study in the area investigating the association of source-specific PM2.5 with biomarkers of inflammation including white blood cells (WBC), high sensitive C-reactive protein (hsCRP), tumor necrosis factor-soluble receptor-II (sTNF-RII), interleukin-6 (IL-6), and von Willebrand factor (vWF). Our results have important implications for policy makers in identifying the most toxic sources of PM2.5, and in turn designing schemes for mitigating adverse health impacts of air pollution in Tehran.
Introduction
Many metropolitan areas around the world are impacted by severe air pollution due to the rapid rate of urbanization and industrialization. According to the most recent World Health Organization (WHO) report, ambient air pollution contributed to 7.6% of all deaths (approximately 4.2 million individuals) around the globe in 2016 (WHO, Citation2018; World health statistics 2018). Among various air pollutants, fine particulate matter (PM, particles with aerodynamic diameter less than 2.5 µm) are of notable importance due to their chemical composition, higher oxidative capacity, higher surface area to particle mass ratio, and their ability to reach the lung alveoli (Kim et al. Citation2017a), resulting in several adverse health consequences, including neurodegenerative disorders, respiratory, cardiovascular, and cardiopulmonary disease (Brook et al. Citation2010; Gauderman et al. Citation2015; Ng et al. Citation2017; Pope et al. Citation2015; Rich et al. Citation2013; Sun et al. Citation2016). Despite the complexity of biological pathways leading to the abovementioned detrimental health outcomes, numerous epidemiological and toxicological studies have confirmed the major role of PM2.5 in inducing oxidative stress response and inflammation within macrophages and epithelial cells (Ayres et al. Citation2008; Bates et al. Citation2019; Lionetto et al. Citation2019; Ostro et al. Citation2014; Pope et al. Citation2016). Exposure to PM2.5 has also been associated with enhanced levels of several blood circulating and inflammation biomarkers, including white blood cell (WBC), von Willebrand factor (vWF), high sensitive C-reactive protein (hsCRP), tumor necrosis factor-soluble receptor-II (sTNF-RII), and Interleukin-6 (IL-6) (Chen et al. Citation2015; Croft et al. Citation2017; Liu et al. Citation2017b).
Complementary studies have indicated the significant linkage between the above listed blood biomarkers and several adverse health endpoints (Brook et al. Citation2010; Velde, van Der, Meijers, and de Boer Citation2014). For instance, Velde, van Der, Meijers, and de Boer (Citation2014) showed an association between elevated hsCRP blood biomarker with heart disease and mortality. In addition, WBC counts were associated with the enhanced coronary heart disease occurrence as well as cardiovascular illnesses (Kim et al. Citation2017b). vWF, a blood glycoprotein involved in hemostasis and stored in endothelial cell and platelet granules (Croft et al. Citation2017) was demonstrated to be significantly associated with myocardial infarction, strokes, and cardiovascular disorders (Green et al. Citation2017). Multiple sclerosis (Ribeiro et al. Citation2019), mortality in the elderly (Bruunsgaard et al. Citation2003), diabetic kidney, and heart disease (Carlsson et al. Citation2016) were also found to be associated with increased levels of sTNF-RII. Finally, IL-6, a pleiotropic inflammatory cytokine, was shown to be associated with cardiovascular diseases and type 2 diabetes (Heikkilä, Ebrahim, and Lawlor Citation2008; Lowe et al. Citation2014) as well as mortality in seniors (Lee et al. Citation2012).
Despite the well-documented toxicological characteristics of PM2.5, some PM constituents are more toxic than others, indicating the inadequacy of implementing total PM mass concentration as the sole metric in health exposure assessments (Lippmann Citation2010). In fact, a number of studies have confirmed significant associations of some PM compounds such as redox active metals (Charrier and Anastasio Citation2012; Steenhof et al. Citation2014), elemental carbon (EC) (Delfino et al. Citation2009; Samara Citation2017; Su et al. Citation2017), organic carbon (OC) (Chirizzi et al. Citation2017), water soluble organic carbon (WSOC) (Delfino et al. Citation2010b; Verma et al. Citation2012; Vreeland et al. Citation2017), and polycyclic aromatic hydrocarbons (PAHs) (Jeng et al. Citation2011; Lundstedt et al. Citation2007) with the increased levels of inflammation biomarkers and PM-induced toxicity. Moreover, according to the literature, ambient PM2.5 can originate from multiple sources with distinct bulk chemical composition, including traffic emissions, industrial activities, soil, road dust, secondary aerosols, biomass burning (wood smoke), and coal combustion (Altuwayjiri et al Citation2020; Cohen et al. Citation2009; Crilley et al. Citation2017; Hasheminassab et al. Citation2014; Liu et al. Citation2017a). Consequently, it is vital from a regulatory perspective to investigate the association of chemical species, and in turn, PM sources with biomarkers of inflammation as indicators of various adverse health effects. Findings from such studies are very important to air quality officials in determining the most toxic sources of PM, particularly in polluted metropolitan areas, in order to adopt effective emission reduction policies for sources with alarming levels of PM toxicity.
Tehran, the most populated city of Iran with more than 9 million residents, is suffered from severe air pollution episodes, due mainly to vehicular emissions, industrial activities, frequent dust storms, and residential heating (Amini et al. Citation2014; Arhami et al. Citation2017; Hosseini and Shahbazi Citation2016; Shahbazi et al. Citation2016b; Soleimanian et al. Citation2019b; Taghvaee et al. Citation2018). In addition, the particular topography of Tehran limits the dispersion of air pollutants from the northern and eastern neighborhoods of the city, due to the presence of the Alborz Mountains with an elevation of 2,700–3,000 meters (Axen et al. Citation2001). Therefore, the transported anthropogenic emissions via the prevailing westerly and southerly winds are trapped in the central zones of the city, further deteriorating the air quality in central Tehran (Atash Citation2007), and increasing its potential to cause severe cardiopulmonary diseases (Bayat et al. Citation2019; Faridi et al. Citation2019; Hosseinpoor et al. Citation2005; Naddafi et al. Citation2012). Due to the significance of PM2.5 as a criteria pollutant in Tehran, a number of studies in the literature have reported potential associations between harmful health outcomes and exposure to PM2.5 in this mega city (Akbarzadeh et al. Citation2018; Amini et al. Citation2019; Hassanvand et al. Citation2017; MohseniBandpi et al. Citation2017). For instance, Amini et al. (Citation2019) observed significant short-term associations between total PM2.5 and non-accidental daily mortality in Tehran. Moreover, Hassanvand et al. (Citation2017) investigated the short-term impacts of exposure to different PM size ranges on inflammation biomarkers among young and elderly population in central Tehran. However, to the best of our knowledge, no studies have ever been performed in the area to evaluate the association of blood biomarkers with individual sources of PM2.5.
The main aim of this study was to investigate the association between various blood biomarkers (WBC, vWF, hsCRP, IL-6, and sTNF-RII) and the source-specific PM2.5 mass concentrations in central Tehran in two panels of young and elderly subjects. The second objective was to examine the possible heterogeneity between the effects of exposure to different PM2.5 sources among the young and the elderly panel. Therefore, the abovementioned coagulation and systemic inflammation biomarkers were measured in the blood samples drawn from each participant every 8 weeks (a total of 6 blood draws for each participant) from May 2012 to May 2013. In addition, the source-specific PM2.5 levels were determined in both sampling locations by the means of the United States Environmental Protection Agency Positive Matrix Factorization (USEPA PMF version 5.0) model. These data were then used as inputs to the single-pollutant linear mixed-effects regression model in two different lag configurations; lag 1–3 (1 to 3 days prior to blood sampling), and lag 4–6 (4–6 days prior to blood sampling) to further investigate the delayed and progressive effects of exposure to PM2.5 sources among elderly and young panel.
Methodology
Study design
In this study, blood samples were drawn from elderly and young subjects in two parallel panels. Sixty elderly volunteers (>65 years old) living in a retirement home (35° 42′ 20.30′′ N, 51° 22′ 14.41′′ E) as well as forty-five young male adult volunteers (15–18 years old) living in a school dormitory (35° 42′ 40.33′′ N, 51° 22′ 49.75′′ E) were selected for blood draws. Air sampling took place at these two sites that were located at a close distance (1.1 km) relative to each other in central Tehran. Both sampling locations were heavily impacted by vehicular emissions, due to their vicinity to a major freeway (i.e., Chamran freeway) as well as congested local streets nearby (Citation2014; Hassanvand et al. Citation2015). In addition, researchers in the field have documented the presence of traffic-related, residential, and commercial activities in the central zones of Tehran (Atash Citation2007; Shahbazi et al. Citation2016a, Citation2016b), leading to high levels of air pollution in the densely populated city center (Shahbazi et al. Citation2016a), which makes it an ideal location for exposure assessment studies. Further information regarding the study sites can be found in Hassanvand et al. (Citation2015, Citation2014)
Sixteen out of the 60 elderly volunteers and 5 out of the 45 students were excluded from our analyses due to the lack of sufficient biomarker data, caused by frequent infections and death. From May 2012 to May 2013, six blood draws, 8 weeks apart, were scheduled for each participant on a fixed time of the week (i.e., Wednesday afternoon from 1p.m. to 3 p.m.), resulting in a total of 240 and 264 samples at the school dormitory and the retirement home, respectively. PM2.5 concentrations showed distinct seasonal patterns (Hassanvand et al. Citation2015, Citation2014); thus longer time periods between the measurements were chosen to increase the variability in the fine PM concentrations. Prior to blood sampling, a physician also visited participants and collected data on their health status, medication use, and disease to control the confounding factors at each step of blood sampling. Moreover, no blood sampling was conducted if acute infectious illnesses were observed in a particular volunteer and only participants with full six blood samples were included in the analyses. All participants signed a written informed consent to attend in this study which was approved by the Research Ethics Boards of Tehran University of Medical Sciences (Research Number 90–03-46-15705). More details about the demographic and clinical characteristics of the participants can be found in Hassanvand et al. (Citation2017), and in Table S1.
Blood biomarker measurements
Utilizing enzyme- linked immunosorbent assay (Quantikine, R&D Systems), we analyzed three biomarkers including vWF, sTNF-RII, and IL-6 at the Immunology, Asthma, and Allergy Research Institute, Tehran University of Medical Sciences. Moreover, an immunoturbidimetric method (Sentinel CRP Vario List No. 6K26-02) was used for hsCRP analysis, whereas an automatic hematological analyzer (CellDyn 4000, Abbott) was employed for counting the WBC in blood samples. All specimens were analytically replicated to ensure the biomarker data reproducibility.
PM2.5 measurement and source apportionment
Detailed description of the PM2.5 measurement and chemical analyses can be found elsewhere (Citation2014; Hassanvand et al. Citation2015). In summary, a low-volume air sampler (FRM OMNU™ air Sampler, multi-cut inlet; BGI, USA) was used to collect 24-hour time integrated ambient PM2.5 samples on quartz (47 μm diameter, Whatman Inc.) and Polytetrafluoroethylene (PTFE): (47 μm diameter, SKC Inc.) filters in both locations from May 2012 to June 2013. The average PM2.5 mass concentrations were 30.9 and 33.2 μg/m3 in the retirement home and school dormitory, respectively. To achieve the required mass loading for further chemical analyses, the daily collected samples were composited every three (or four) days. The composited PTFE samples were chemically analyzed for their trace elements and metallic contents (i.e., Al, Ti, Ba, Fe, Ni, Cd, Se, Li, Sr, Zn, Sn, Cu, Mn, Cr, and Si), using an Inductively Coupled Plasma Optical Emission Spectrometer (ICP-OES) instrument. Concentrations of water-soluble ions (i.e., K+, NH4+, Mg+2, Ca+2, NO3−, and SO4−2) in the samples were also determined by the means of an ion chromatography (IC) instrument. Moreover, following the National Institute forOccupational Safety and Health 5040 protocol, elemental carbon (EC) and organic carbon (OC) were quantified for limited number (i.e., 12) of quartz filters by thermo-optical transmittance (TOT) analysis.
Overall, 39 ambient PM2.5 samples were chemically analyzed at each sampling site and were used as the input concentration matrix to the USEPA PMF version 5.0 for identifying sources and quantifying their relative contribution to the ambient PM2.5 at the school dormitory and the retirement home (Norris et al. Citation2014; Paatero Citation1997; Paatero and Tapper Citation1994). Furthermore, the PMF model was run in the robust mode and the uncertainties associated with the PMF-resolved source specific mass concentrations were investigated at both sampling sites by the means of various PMF error estimation tools including displacement (DISP) method, bootstrap (BS) analyses, and a combination of DISP and BS (BS-DISP). Figures S1 and S2 indicate the uncertainties associated with the PMF solution at the school dormitory and the retirement home, respectively. According to these figures and the output of PMF built-in error estimation tools, our PMF solution in both sampling location was valid in terms of BS analyses, since > 80% of resolved factors were mapped. In school the dormitory, the percentage of mapping was within the range of 88% (for biomass burning) to 96% (for the vehicular emissions). Similarly, in the retirement home, the percentage of mapping for resolved BS factors to each base factor varied from 83% (for soil factor) to 90% (for vehicular emissions). Moreover, quite small BS errors were associated with the chemical markers of PMF-resolved sources, indicating negligible impact of random errors and (to some extent) rotational ambiguity on the final PMF outputs. Moreover, results of the PMF runs were acceptable in terms of DISP analyses due to negligible decrease (i.e., dQ = 0.001% in the school dormitory, and dQ = 0.01% in the Tohid retirement home) in PMF objective function (Q) as well as absence of any factor swaps for dQmax = 4. In addition, as shown in Figures S1 and S2, there were significantly negligible DISP errors associated with the tracers of PM2.5 sources, further underscoring the insignificancy of rotational ambiguity in PMF-resolved source specific mass concentration. Finally, BS-DISP (frequently used as the hybrid uncertainty estimation approach) corroborated the robustness of our PMF analysis, judging by the less than 0.5% decrement of Q, and negligible uncertainties associated with the source-specific tracers of PMF solution (Norris et al. Citation2014; Paatero et al. Citation2014; Reff, Eberly, and Bhave Citation2007). Comprehensive explanations regarding the PM2.5 source apportionment via the PMF model can be found in Taghvaee et al. (Citation2018).
Statistical analysis
The association between the blood biomarkers (i.e., WBC, vWF, hsCRP, IL-6, and sTNF-RII) and the PM2.5 source contributions was evaluated by single-pollutant linear mixed-effects regression model using Stata software 2013 (Stata Statistical Software: Release 13. College Station, TX: StataCorp LP) in the healthy young adults and elderly subjects, separately. As mentioned earlier, repeated blood sampling was scheduled for each individual every 8 weeks to ensure independent examination of each subject over time. We included the random intercept term in our statistical model. Since there was no concern for controlling the effect of time-invariant subject characteristics by the study design, adjustment was done for the effect of meteorological variables (i.e., temperature and relative humidity) by setting these parameters as fixed in the model. The delayed and progressive impacts of exposure to PM sources were investigated by considering the concentration of source-specific particulate matter and meteorological parameters in two-time lags: lag 1–3 (1 to 3 days prior to blood sampling) and lag 4–6 (4–6 days prior to blood sampling). In addition, the logarithms of IL-6 and sTNF-RII were used in the analysis due to the skewness observed in their residuals.
The percent change in blood biomarker levels per an interquartile range (IQR) increase in exposure to PM sources (with 95% confidence interval (CI)) was calculated using equation (Equation1(1)
(1) ) for log10-transformed biomarkers and equation (Equation2
(2)
(2) ) for other biomarkers:
Results
Characteristics of PMF-resolved PM2.5 sources in central Tehran
Table S2 indicates the relative contributions of the PMF-resolved sources to ambient PM2.5 concentrations as well as source-specific PM2.5 mass concentration at our sampling sites. According to the table, the PMF model identified vehicular emissions, secondary aerosols, industrial emissions, biomass burning, soil, and road dust as PM2.5 sources at both the retirement home and the school dormitory. Vehicular emissions and secondary aerosols were the two major sources, contributing to approximately 50% and 25% of PM2.5 mass concentrations in central Tehran, respectively. Specifically, vehicular emissions contributed to 15.1 μg/m3 and 16.4 μg/m3 of PM2.5 mass concentrations in the retirement home and school dormitory, respectively. Moreover, secondary aerosol particles contributed to nearly 8 μg/m3 of the total PM2.5 mass concentration at both sites. While the industrial activities exhibited minimal contribution to PM2.5 at the school dormitory, their corresponding contribution was around 18% at the retirement home, probably because of the closer proximity of the retirement home to the industrial activities located in the western zones of the city. Detailed description of the resolved PM2.5 source contributions in the selected sampling sites can be found in Taghvaee et al. (Citation2018).
Moreover, (a-b) shows the results of Spearman rank order correlation analyses between the PMF-resolved PM2.5 sources in both the school dormitory and the retirement home. According to the table, no meaningful associations were observed between the source-specific PM2.5 mass concentrations in both the school dormitory and the retirement home, indicating that the reported associations of blood biomarkers with a particular PM2.5 source are independent of other PM2.5 sources. Also, vehicular emission was the only PM2.5 source that exhibited strong correlations (R2 = 0.7 in the retirement home and R2 = 0.9 in the dormitory) with the total ambient PM2.5. This observation is in agreement with the findings of Taghvaee et al. (Citation2018), in which vehicular activities contributed to approximately half of the total PM2.5 mass concentrations at both selected sampling sites. Nonetheless, there is less confidence in the reported associationsof blood biomarkers with vehicular emissions independent of theirassociations with total PM2.5, which is supported by the observedstrong correlation of vehicular PM2.5 with total ambient PM2.5.
Table 1. Spearman rank order correlation analyses between PM2.5 sources in (a) the Tohid retirement home and (b) the school dormitory
Association of blood biomarkers with PM2.5 sources
White blood cells (WBC)
The associations of WBC versus various PM2.5 sources are illustrated in for both elderly and young populations. In the elderly panel ()), the WBC levels were positively associated with all PM2.5 sources in both time lags, except for biomass burning emissions. In detail, secondary aerosol, industrial emissions, road dust, and total PM2.5 showed statistically significant associations (Pvalue < .05) with WBC in the lag 1–3 and lag 4–6. The percentage changes in the WBC were 24% (95% CI: 18%, 32%) per an IQR increase in the secondary originated PM2.5 mass concentrations in the first lag. It is also worth mentioning that traffic-related PM2.5 were associated with the increase of WBC in the elderly for both time lags, although the associations were not statistically significant (Pvalue = .230 and .167, for lag 1–3 and lag 4–6, respectively). In contrast, as shown in ), no positive and meaningful associations were observed between this health biomarker and most of the PM2.5 sources in young people, with the exception of vehicular PM2.5, which exhibited significantly positive associations (Pvalue < .05) with WBC in the first-time lag for young people. The maximum increase in the percentage of WBC (6.6%, 95% Cl: 2.7%, 10.6) among the young panel was related to vehicular PM2.5 in the first lag. Our findings revealed negative associations of biomass burning with leukocyte counts in both subjects for all time lags.
Figure 1. Associations between white blood cells (WBC) and source-specific PM2.5 concentrations in the panel of (a) the elderly (n = 44) and (b) healthy young adults (n = 40). Estimated percent changes in the blood marker (adjusted coefficient and 95% CI) corresponds to an IQR increase in total PM2.5 and source-specific PM2.5 concentrations (see IQR in Table S2 in the Supplementary material), adjusted for temperature and relative humidity
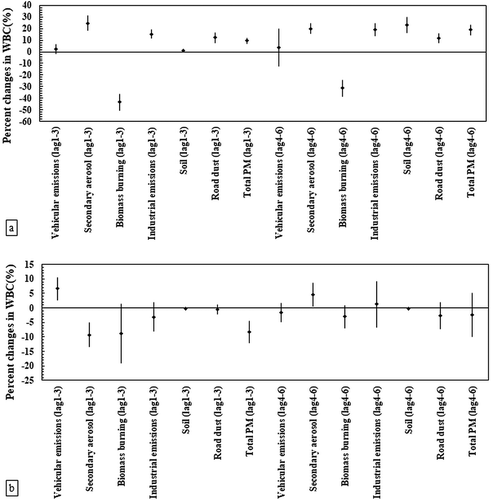
Von Willebrand factor (vWF)
The associations between vWF and PM sources are shown for elderly and young subjects in . In the elderly panel (), secondary source and road dust were positively associated (Pvalue < .05) with vWF (i.e., a marker of endothelial dysfunction) in both time lags. In contrast, no meaningful associations were observed between this blood biomarker and soil as well as industrial emissions in either of time lags. Vehicle-derived PM2.5 had significantly positive association (Pvalue < .05) with this blood biomarker in the second time lag in the elderly panel. The highest increase in the vWF levels (28.9%, 95% CI: 2.5%, 55.3%) were related to an IQR increase of the traffic emissions in time lag 4–6. Furthermore, biomass burning activity was negatively associated with vWF in both time lags among the elderly ()). Similar to the results presented for WBC, we did not find any significant associations between PM sources and vWF in young subjects ()).
Figure 2. Associations between von Willebrand factor (vWF) and source-specific PM2.5 concentrations in the panel of (a) the elderly (n = 44) and (b) healthy young adults (n = 40). Estimated percent changes in the blood marker (adjusted coefficient and 95% CI) corresponds to an IQR increase in total PM2.5 and source-specific PM2.5 concentrations (see IQR in Table S2 in Supplementary material), adjusted for temperature and relative humidity
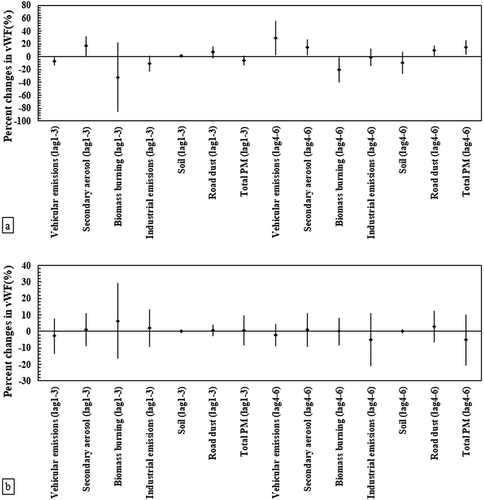
High sensitive C-reactive protein (hsCRP)
illustrates the relationship between hsCRP and PM sources for the senior populations during our sampling campaign. Based on , industry-originated and total PM2.5 were positively and significantly associated with hsCRP in lag 1–3 among elderly (Pvalue < .05). Indeed, industrial activities were the major contributing source to the elevated hsCRP levels (15.8%, 95% CI: 3.2%, 23.3%) per an IQR increase in their corresponding emissions in the first-time lag. Moreover, vehicular emissions and secondary aerosols demonstrated positive association with this biomarker among seniors in time lag 1–3, although their associations were not significant (Pvalue = .253 and .127, for vehicular emissions and secondary aerosols, respectively). On the other hand, no significant associations were observed between source-specific PM concentrations and hsCRP in the second time lag. However, secondary aerosols, industrial emissions, soil, and total PM2.5 were positively associated with this indicator of cardiovascular diseases for the elderly in time lag 4–6. In addition, similar to the case of other biomarkers, we found unexpected and significant (Pvalue < .05) inverse associations of biomass burning with hsCRP in retirement home during the entire sampling campaign. Moreover, our statistical analysis revealed insignificant association of hsCRP with all PM2.5 sources in the school dormitory within all-time lags.
Figure 3. Associations between high sensitive C-reactive protein (hsCRP) and source-specific PM2.5 concentrations in the panel of the elderly (n = 44). Estimated percent changes in the blood marker (adjusted coefficient and 95% CI) corresponds to an IQR increase in total PM2.5 and source-specific PM2.5 concentrations (see IQR in Table S2 in Supplementary material), adjusted for temperature and relative humidity
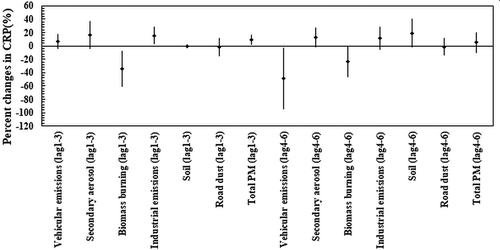
Tumor necrosis factor-soluble receptor-II (sTNF-RII)
The association between sTNF-RII versus PM2.5 sources are presented for both elderly and young subjects in . As shown in ), sTNF-RII was positively associated with all PMF-resolved sources among seniors (Pvalue < .05), except for biomass burning (in all time lags) and vehicular emissions (in lag 4–6). In addition, generally stronger associations were observed between this biomarker and PM sources in the second time lag. For instance, the industry-related increase in the sTNF-RII levels was higher in the second lag (24.3%, 95% CI: 13.5%, 36.1%) compared to that of the first lag (20.3%, 95% CI: 12.4%, 28.7%) in the elderly panel. Similarly to other biomarkers, we found negative associations between biomass burning and sTNF-RII for all-time lags. Conversely, we observed no meaningful associations between sTNF-RII and most of the identified PM sources in the blood samples of the young panel during the sampling time lags ()). However, industrial emissions and biomass burning exhibited positive associations with this blood biomarker in the first-time lag, although their associations were marginally significant (Pvalue = .121 and .130, for industrial emissions and biomass burning, respectively).
Figure 4. Associations between tumor necrosis factor-soluble receptor-II (sTNF-RII) and source-specific PM2.5 concentrations in the panel of (a) the elderly (n = 44) and (b) healthy young adults (n = 40). Estimated percent changes in the blood marker (adjusted coefficient and 95% CI) corresponds to an IQR increase in total PM2.5 and source-specific PM2.5concentrations (see IQR in Table S2 in Supplementary material), adjusted for temperature and relative humidity
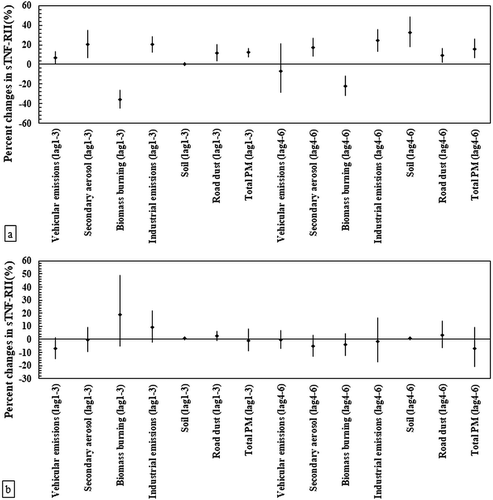
Interleukin-6 (IL-6)
presents the associations between IL-6 and source-specific PM2.5 levels in the elderly and the young panels. Our statistical analysis of elderly subjects revealed positive and significant associations of IL-6 with total ambient PM2.5 levels as well as various PM sources including secondary aerosol, industry, soil, and road dust in both time lags. In addition, exposure of the elderly population to vehicular PM2.5 caused significant (Pvalue < .05) increases in IL-6 levels in the second time lag ()). For both studied time lags, the maximum percentage increase in IL-6 was due to secondary aerosols, indicating 402.9% (95% CI: 251.7%, 619.2%) and 239.1% (95% CI: 166.4%, 331.8%) elevations in this pro-inflammatory cytokine per an IQR increase of secondary PM concentrations. IL-6 was inversely associated (Pvalue < .05) with biomass burning particles in the elderly group for both time lags. Finally, no significant associations were observed between IL-6 and most of the source-specific PM concentrations in the healthy young adults. Among all identified PM sources, IL-6 had significantly negative associations (Pvalue < .05) with only one PM source (i.e., particles formed by secondary photochemical processes) in the first-time lag, while vehicular emissions and secondary particles were the only sources indicating significant positive associations (Pvalue < .05) with IL-6 in the lag 1–3 and lag 4–6, respectively ()).
Figure 5. Associations between interleukin-6 (IL-6) and source-specific PM2.5 concentrations in the panel of (a) the elderly (n = 44) and (b) healthy young adults (n = 40). Estimated percent changes in the blood marker (adjusted coefficient and 95% CI) corresponds to an IQR increase in total PM2.5 and source-specific PM2.5 concentrations (see IQR in Table S2 in Supplementary material), adjusted for temperature and relative humidity
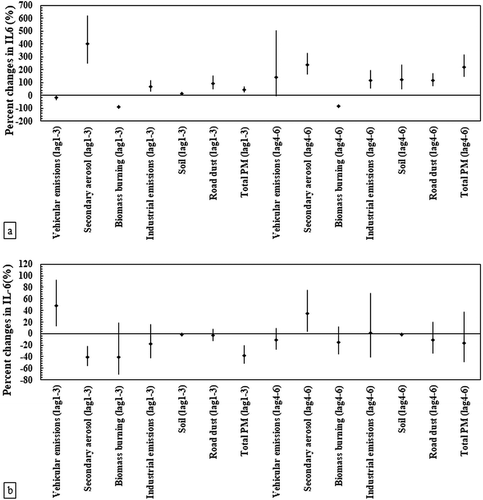
Discussion
WBC count is an inflammatory biomarker which is used to detect vascular inflammation and cardiovascular illnesses (Chen and Schwartz Citation2008). Previous studies have indicated significantly positive associations of WBC counts with transition metals (i.e., Cu, Ni, and V) (Steenhof et al. Citation2014). According to the literature, Cu is a chemical tracer of road dust (Karanasiou, Siskos, and Eleftheriadis Citation2009; Lim et al. Citation2010), while Ni and V are chemical markers of various industrial emissions including cement, stainless steel, and brick production (Banerjee et al. Citation2015; Song et al. Citation2006), heavy oil combustion (Cesari et al. Citation2014), and non-tail pipe emissions (Duong and Lee Citation2011; Wang et al. Citation2005). Therefore, significant and positive associations were observed between WBC and industry-related PM2.5 as well as road dust in the elderly subjects. Among the seniors, the observed 12% road dust-related increases in the WBC levels were also comparable with the findings of Steenhof et al. (Citation2014), in which the authors reported around 10% increase in the average WBC counts as a result of exposure to Cu and Ni as tracers of road dust PM. Furthermore, the presented positive association of traffic-originated PM and leucocyte counts among the elderly (in both time lags) and the young panels (in the first time lag) can also be attributed to the previously documented association of EC (i.e., a well-known tracer of vehicular emissions (Zhang et al. Citation2013b; Zong et al. Citation2016)) and NOx with this blood biomarker (Steenhof et al. Citation2014; Su et al. Citation2017). Zhang et al. (Citation2018) reported lower WBC counts with higher physical activities, further corroborating the observed insignificant associations of most source-specific PM concentrations with leukocyte counts in the more active young populations. Lastly, the unexpected negative associations of biomass burning emissions with WBC might be due to the inverse association of this PM source with secondary aerosols. Specifically, while higher biomass burning contributions were observed during the colder period of the year in our sampling locations, particles originating from secondary formation peak at summer time due to the higher photochemical oxidation during that period (Taghvaee et al. Citation2018). Since our statistical analyses showed strong and positive associations of various blood biomarkers with secondary particles in different time lags, the abovementioned hypothesis probably explains the inverse association of WBC (as well as other blood biomarkers such as vWF, hsCRP, with sTNF-RII, and IL-6) with PM originating from biomass combustion. Similarly to our findings, Ng et al. (Citation2017) attributed the negative association of biomass burning PM2.5 with term low birth weight (TLBW) to the anti-association of this PM source with the secondary ammonium sulfate as the major contributing source to the adverse birth outcomes in California. In another study, Al Hanai et al. (Citation2019) also observed the negative association of levoglucosan (i.e., a well-known tracer of biomass burning (Simoneit et al. Citation1999) with TNF-α as a biomarker of coagulation in Tehran, Iran. In addition, while biomass burning emissions tend to peak at colder seasons, individuals spend less time outdoors during that period (which decreases their overall exposure to ambient PM2.5), probably justifying the negative association between particles originating from biomass burning with various blood biomarkers among both the young and the elderly.
vWF is a prognostic marker that can be utilized to predict systemic inflammation and cardiovascular illnesses (Gragnano et al. Citation2017). Several studies have indicated positive associations of aluminum (i.e., tracer of road dust (Keuken et al. Citation2013)), and precursors of secondary PM (e.g., SOx, and NOx) with vWF, a biomarker of coagulation (Wu et al. Citation2012; Zhang et al. Citation2013a); in concert with our observations in terms of positive associations between abovementioned sources and vWF in the retirement home. Among the elderly group, our study revealed a secondary PM-related increase of 15%--16% in the vWF levels, which is comparable with the results of Zhang et al. (Citation2013a), reporting an 8% increase in vWF associated with IQR increase in NO2 and SO2 concentrations at various time lags. In addition, our statistical findings were in line with the results of Bell et al. (Citation2014), in which the authors reported significantly positive association of PM2.5 road dust with the cardiovascular hospital admissions. Furthermore, results from chamber and atmospheric measurement studies have indicated that the toxicity of primary combustion products increases as they undergo photo-chemical processing due to the increased oxidative potential of the reaction products, i.e., Secondary Organic Aerosol (Jiang et al. Citation2016; Tuet et al. Citation2017) compared to the precursors. Additionally, the positive association (Pvalue = .032) of vWF with vehicular emissions among seniors (in the second lag) is consistent with the results of Yuan et al. (Citation2013) and Yue et al. (Citation2007), exhibiting the impact of traffic emissions on increased vWF plasma levels. However, our reported traffic-related increase (around 20%) in the vWF levels was higher than the results of Yuan et al. (Citation2013), in which vehicular emissions contributed to 11.3% (95% CI: 5,17.6%) increase in this biomarker level during the 0–4 days of exposure. Nevertheless, other studies have observed insignificant association of vWF with vehicular sources (Jacobs et al. Citation2011; Wu et al. Citation2014). This inconsistency regarding the association of vWF with vehicular emissions was also reflected in ), in which insignificant associations were observed for vehicular sources versus vWF in the first-time lag, despite the significantly positive associations observed in the second lag.
hsCRP is a biomarker widely used for predicting the risk of cardiovascular diseases (Kamath et al. Citation2015). In our study, hsCRP had positive associations (Pvalue < .05) with industry-related (12%--15% increase in hsCRP levels) and total PM2.5 (5%--9% increase in hsCRP levels) in the elderly group, which is consistent with the results of Hennig et al. (Citation2014), in which the authors observed 7.96% (95% CI: 3.45, 12.67%) and 4.53% (95% CI: 2.76, 6.33%) changes in hsCRP levels per 1 µg/m3 increase in ambient PM2.5 and industry-related PM2.5 concentrations, respectively. In addition, the reported positive association (in the first time lag) of this biomarker with traffic-related emissions among elderly was in agreement with a number of earlier studies in literature (Delfino et al. Citation2009; Jacobs et al. Citation2010; Pilz et al. Citation2018; Rückerl et al. Citation2014; Siponen et al. Citation2015). For instance, Delfino et al. (Citation2009) observed positive associations of vehicular tracers (i.e., EC, primary organic carbon [POC], NOx, and CO) with this biomarker of systematic inflammation. Previous studies have also reported positive association of hsCRP with sulfate (Li et al. Citation2017) and ozone (Arjomandi et al. Citation2015), corroborating the observed association of secondary sources with this biomarker in the elderly panel. Nonetheless, the observed secondary-related increase (i.e., 12%--16%) in hsCRP among the elderly was higher than that of Li et al. (Citation2017), reporting 4%--5% increase in hsCRP levels per 2 µg/m3 increase in sulfate concentrations. The fast response nature of hsCRP (Siponen et al. Citation2015) probably explains the slightly higher associations of this biomarker and various PM sources in the first lag compared to the second lag in the retirement home. Additionally, the observed insignificant associations of PM sources with hsCRP (as well as other biomarkers including sTNF-RII and IL-6) in young people may be due to the demographic characteristics (e.g., age and stress factors) that influence the susceptibility of subjects during exposure campaigns (Clougherty Citation2010; Laskin et al. Citation2010).
sTNF-RII, which is a soluble form of the TNF receptor, is an indicator of coronary heart failure (Carlsson et al. Citation2016; Hassanvand et al. Citation2017; Heaney and Golde Citation1998). In the elderly panel, the increased levels of this blood biomarker as a result of exposure to secondary, industry, and road dust PM are in agreement with previous studies in the literature, indicating positive associations of sTNF-RII with O3 (tracer of secondary organic aerosol; Soleimanian et al. Citation2019a; Li et al. Citation2017), WSOC (chemical marker of secondary aerosol; Snyder et al. Citation2009; Delfino et al. Citation2010a), secondary organic carbon (i.e., tracer of secondary PM2.5; Wu et al. Citation2014), and zinc (tracer of both industrial and road dust emissions; Keuken et al. Citation2013; Wu et al. Citation2012). For instance, Wu et al. (Citation2012) reported a 12.50% (95% CI: 5.88, 19.54) increase in this blood biomarker with an IQR increase in Zn concentrations (as a tracer of road dust), further corroborating our findings showing that road dust PM caused an approximate 10% increase in sTNF-RII level in both time lags among the elderly population. In addition, vehicular emissions were positively associated with sTNF-RII (Delfino et al. Citation2010a, Citation2009, Citation2008; Jiang et al. Citation2019), further supporting our observations among the senior group. In contrast, Bräuner et al. (Citation2008) concluded that healthy young responses to the changes in tumor necrosis factor α levels is rarely influenced by ambient air pollution, which supports our own results showing insignificant associations of this blood biomarker with different PM2.5 sources in young subjects.
IL-6 is an important indicator of coronary diseases by exciting immune cells within human body (Hassanvand et al. Citation2017; Kritchevsky, Cesari, and Pahor Citation2005). According to our statistical model, we observed positive associations (Pvalue < .05) of IL-6 with secondary, industrial, soil, and road dust PM in the elderly group. This observation is in line with previously documented positive associations of secondary organic aerosol (Jiang et al. Citation2019; Ma et al. Citation2019; Niu et al. Citation2017; Sheng and Lu Citation2017), industry-related (Eze et al. Citation2016; Quay et al. Citation1998), and soil/road dust derived PM (Veranth et al. Citation2006) with pro-inflammatory cytokines IL-6. In addition, our statistical analyses in the elderly panel revealed significant elevations of IL-6 (Pvalue < .05) per an IQR increase in traffic-related PM2.5 in the second time lag, which is in agreement with results of previous biomarker studies (Delfino et al. Citation2009, Citation2008, Citation2010b; Jiang et al. Citation2019), attributing the IL-6 secretion to the traffic-related PM2.5. Moreover, the strengthening in the associations of most PM sources with IL-6 from lag 1–3 to lag 4–6 in the retirement home can probably be attributed to the relatively slower response rate of this biomarker (Hassanvand et al. Citation2017).
Limitations
Although the results of this study provide insights into the association of various inflammation and coagulation blood biomarkers with source-specific PM2.5 mass concentration among young and elderly groups in Tehran, we acknowledge that some limitations are associated with the implemented methodology and analyses. First, we acknowledge that seasonal variations in the source specific PM2.5 mass concentrations might create seasonal trends in the PM2.5 exposure among both the young and the elderly. However, the limited number of PM2.5 samples prevented us from conducting seasonal correlation analyses between various biomarkers and PM2.5 sources in the multilinear regression model. Secondly, seasonal differences in PM2.5 exposure might vary across the young and the elderly due to the different patterns of physical activity and residential penetration of PM indoors. However, as mentioned in Table S2 and according to Taghvaee et al. (Citation2018), the ambient source-specific PM2.5 mass concentration levels were comparable in both dormitory and retirement homes during the cold and warm seasons. In addition, Hassanvand et al. (Citation2015) and Hassanvand et al. (Citation2014) reported similar ratios of PMindoor/PMoutdoor (i.e., around 0.56) in both locations. Consequently, both young and senior populations are being exposed to comparable levels of source-specific PM mass concentrations during cold and warm seasons. We also acknowledge that young people (in comparison to the elderly) spend more time outside of their residential building; thus, they are likely to be exposed to higher levels of PM mass concentrations. Particularly during the cold season, young people are more exposed to the outdoor PM, since the senior people tend to spend more time indoors. However, despite the presumed higher exposure of young people to PM throughout the year, our results revealed that young people were less vulnerable to source-specific PM2.5 emissions.
As another limitation of this study, no gaseous air pollutants (e.g., CO, NO2, O3, and SO2) mass concentrations were monitored during our sampling campaign. Thus, we could not examine the correlation between PM2.5 and criteria gaseous air pollutants to ensure that our reported associations of blood biomarkers with PM2.5 were independent of those gaseous pollutants. These caveats notwithstanding, no meaningful associations were observed between the fine and coarse fractions of particulate matter in the school dormitory (R2 = 0.01) and the Tohid retirement home (R2 = 0.01), further corroborating that the reported associations between blood biomarkers and the total PM2.5 are independent of the larger size fraction of PM. Another possible explanation for the observed different health impacts of various PM2.5 sources is the variation in the degree to which emissions from particular sources contribute to the population exposures in our selected sampling sites. While the complexity of physical and chemical pollutant transport processes make it difficult to quantify the exact amount of individual exposure to emissions from various sources, PM2.5 mass concentrations in both dormitories and retirement homes were dominated by particles emitted by vehicular sources and secondary reactions, contributing to nearly 50% and 25% of total PM2.5 mass concentration, respectively. Therefore, it is a reasonable assumption that both elderly and young groups are exposed to higher degree of PM2.5 emissions from vehicular activities and secondary aerosols in comparison to other sources of PM2.5. The higher rate of population exposure to vehicular and secondary PM2.5 is in line with the results of our single pollutant multilinear regression model among seniors, indicating positive associations of most biomarkers with particles of secondary origin and vehicular emissions. Lastly, although we implemented some of the methods presented in Mostofsky et al. (Citation2012) for the highly correlated PM2.5 sources (e.g., vehicular emissions) with biomarkers among the young and elderly group, no meaningful changes were observed in the association results. Thus, considering that the interpretation and comparability of the abovementioned models were not straightforward, we did not use such models in our analysis.
Summary and conclusion
The current study examined the association of inflammation blood biomarkers (i.e., hsCRP, WBC, vWF, sTNF-RII, and IL-6) with various PM2.5 sources among young and elderly populations in central Tehran. In the elderly panel, secondary aerosol was positively associated with all blood biomarkers (except hsCRP) within all-time lags, whereas vehicular emissions exhibited the same trend in either first- or second-time lag. Furthermore, we observed positive associations of industry-generated and road dust PM2.5 with WBC, sTNF-RII, and IL-6 among the senior group. In contrast, the majority of PM2.5 sources were not meaningfully associated with increased levels of biomarkers in the school dormitory, probably because young healthy individuals are less vulnerable to pollution resulting from PM2.5 exposure. Nonetheless, traffic-emitted particles had significant associations with the WBC and IL-6 levels that are indicators of cardiovascular disorders in the young panel. Our results indicate that elderly individuals seem to be more susceptible by exposure to source-specific PM2.5 in comparison to young subjects. Further research is required to elucidate the impact of other environmental factors on the increased levels of health biomarkers in highly polluted urban environments.
Supplemental Material
Download MS Word (493.7 KB)Acknowledgment
The authors would like to acknowledge the experimental samplings were conducted under the Health Effects of Air Pollution Panel Study (HEAPPS).
Supplemental Material
Supplemental data for this article can be accessed on the publisher’s website.
Additional information
Funding
Notes on contributors
Abdulmalik Altuwayjiri
Abdulmalik Altuwayjiri is a Ph.D. student and research assistant majoring in air pollution at the University of Southern California (USC). His main research focus is on particulate matter (PM), its gaseous precursors in the atmosphere, sources, and physio-chemical characteristics.
Sina Taghvaee
Sina Taghvaee is a recently graduated Ph.D. student currently working as a postdoc at the University of California Los Angeles (UCLA). His research focuses on the physio-chemical characteristics and oxidative potential of particulate matter (PM).
Amirhosein Mousavi
Amirhosein Mousavi is a recently graduated Ph.D. student currently working as a postdoc at the University of California Irvin (UCI). His research focuses on particulate matter (PM) and its spatial and temporal variations.
Mohammad H. Sowlat
Mohammad H. Sowlat is an air quality specialist currently working at South Coast Air Quality Management District. His research focuses on particulate matter (PM) and its sources in urban environments.
Mohammad Sadegh Hassanvand
Mohammad Sadegh Hassanvand is currently an assistant professor at Tehran University of Medical Sciences, Institute for Environmental Research. His focus of research is on air pollution and its health effects.
Homa Kashani
Homa Kashani is currently an assistant professor working at Tehran University of Medical Sciences, Institute for Environmental Research (IER). Her research focus is on environmental statistics and air pollution.
Sasan Faridi
Sasan Faridi is currently a professor working at Tehran University of Medical Sciences, School of Public Health. His research focus is on the aerosol science and environmental epidemiology.
Masud Yunesian
Masud Yunesian is currently a professor working at Tehran University of Medical Sciences, Institute for Environmental Research (IER). His research focus is on air pollution, epidemiology, and environmental health.
Kazem Naddafi
Kazem Naddafi is currently a professor working at Tehran University of Medical Sciences, Institute for Environmental Research (IER). His research focus is on environmental pollution control and environmental health engineering.
Constantinos Sioutas
Constantinos Sioutas is a Fred Champion Professor in the Civil and Environmental Engineering department at the University of Southern California, investigating the underlying mechanisms that produce the health effects associated with exposure to air pollutants generated by various sources. His primary focus is on particulate matter (PM) and its gaseous precursors in the atmosphere.
References
- Akbarzadeh, M. A., I. Khaheshi, A. Sharifi, N. Yousefi, M. Naderian, M. H. Namazi, M. Safi, H. Vakili, H. Saadat, S. Alipour Parsa, et al. 2018. The association between exposure to air pollutants including PM10, PM2.5, ozone, carbon monoxide, sulfur dioxide, and nitrogen dioxide concentration and the relative risk of developing STEMI: A case-crossover design. Environ. Res. 161:299–303. doi:10.1016/j.envres.2017.11.020.
- Al Hanai, A. H., D. S. Antkiewicz, J. D. C. Hemming, M. M. Shafer, A. M. Lai, M. Arhami, V. Hosseini, and J. J. Schauer. 2019. Seasonal variations in the oxidative stress and inflammatory potential of PM 2. 5 in Tehran using an alveolar macrophage model; The role of chemical composition and sources. Environ. Int. 123:417–27. doi:10.1016/j.envint.2018.12.023.
- Altuwayjiri, A., Pirhadi,M., Taghvaee, S., & Sioutas, C. (2020). Long-term trends in thecontribution of PM2. 5 sources to organic carbon (OC) in the LosAngeles basin and the effect of PM emission regulations. FaradayDiscussions. https://doi.org/10.1039/D0FD00074D
- Amini, H., N. T. Trang Nhung, C. Schindler, M. Yunesian, V. Hosseini, M. Shamsipour, M. S. Hassanvand, Y. Mohammadi, F. Farzadfar, A. M. Vicedo-Cabrera, et al. 2019. Short-term associations between daily mortality and ambient particulate matter, nitrogen dioxide, and the air quality index in a Middle Eastern megacity. Environ. Pollut. 254:113121. doi:10.1016/j.envpol.2019.113121.
- Amini, H., S. M. Taghavi-Shahri, S. B. Henderson, K. Naddafi, R. Nabizadeh, and M. Yunesian. 2014. Land use regression models to estimate the annual and seasonal spatial variability of sulfur dioxide and particulate matter in Tehran, Iran. Sci. Total Environ. 488–489:343–53. doi:10.1016/j.scitotenv.2014.04.106.
- Arhami, M., V. Hosseini, M. Zare Shahne, M. Bigdeli, A. Lai, and J. J. Schauer. 2017. Seasonal trends, chemical speciation and source apportionment of fine PM in Tehran. Atmos. Environ. 153:70–82. doi:10.1016/j.atmosenv.2016.12.046.
- Arjomandi, M., H. Wong, A. Donde, J. Frelinger, S. Dalton, W. Ching, K. Power, and J. R. Balmes. 2015. Exposure to medium and high ambient levels of ozone causes adverse systemic inflammatory and cardiac autonomic effects. Am. J. Physiol. - Hear. Circ. Physiol. 308:H1499–H1509. doi:10.1152/ajpheart.00849.2014.
- Atash, F. 2007. The deterioration of urban environments in developing countries: Mitigating the air pollution crisis in Tehran, Iran. Cities 24:399–409. doi:10.1016/j.cities.2007.04.001.
- Axen, G. J., P. S. Lam, M. Grove, D. F. Stockli, and J. Hassa-nzadeh. 2001. Exhumation of the west-central Alborz Mountains, Iran, Caspian subsidence, and collision-related tectonics. Geology 29:559–62. doi:10.1130/0091-7613(2001)029<0559:EOTWCA>2.0.CO;2.
- Ayres, J. G., P. Borm, F. R. Cassee, V. Castranova, K. Donaldson, A. Ghio, R. M. Harrison, R. Hider, F. Kelly, I. M. Kooter, et al., 2008. Evaluating the toxicity of airborne particulate matter and nanoparticles by measuring oxidative stress potential - A workshop report and consensus statement. Inhal. Toxicol. 20, 75–99. doi: 10.1080/08958370701665517
- Banerjee, T., V. Murari, M. Kumar, and M. P. Raju. 2015. Source apportionment of airborne particulates through receptor modeling: Indian scenario. Atmos. Res. 164–165:167–87. doi:10.1016/j.atmosres.2015.04.017.
- Bates, J. T., T. Fang, V. Verma, L. Zeng, R. J. Weber, P. E. Tolbert, J. Y. Abrams, S. E. Sarnat, M. Klein, J. A. Mulholland, et al. 2019. Review of acellular assays of ambient particulate matter oxidative potential: Methods and relationships with composition, sources, and health effects. Environ. Sci. Technol. 53:4003–19. doi:10.1021/acs.est.8b03430.
- Bayat, R., K. Ashrafi, M. Shafiepour Motlagh, M. S. Hassanvand, R. Daroudi, G. Fink, and N. Künzli. 2019. Health impact and related cost of ambient air pollution in Tehran. Environ. Res 176. doi:10.1016/j.envres.2019.108547.
- Bell, M. L., K. Ebisu, B. P. Leaderer, J. F. Gent, H. J. Lee, P. Koutrakis, Y. Wang, F. Dominici, and R. D. Peng. 2014. Associations of PM2.5 constituents and sources with hospital admissions: Analysis of four counties in connecticut and Massachusetts (USA) for persons ≥ 65 years of age. Environ. Health Perspect 122:138–44. doi:10.1289/ehp.1306656.
- Bräuner, E. V., P. Møller, L. Barregard, L. O. Dragsted, M. Glasius, P. Wåhlin, P. Vinzents, O. Raaschou-Nielsen, and S. Loft. 2008. Exposure to ambient concentrations of particulate air pollution does not influence vascular function or inflammatory pathways in young healthy individuals. Part. Fibre Toxicol. 5:1–9. doi:10.1186/1743-8977-5-13.
- Brook, R. D., S. Rajagopalan, C. A. Pope, J. R. Brook, A. Bhatnagar, A. V. Diez-Roux, F. Holguin, Y. Hong, R. V. Luepker, M. A. Mittleman, et al. 2010. Particulate matter air pollution and cardiovascular disease: An update to the scientific statement from the american heart association. Circulation 121:2331–78. doi:10.1161/CIR.0b013e3181dbece1.
- Bruunsgaard, H., K. Andersen-Ranberg, J. V. B. Hjelmborg, B. K. Pedersen, and B. Jeune. 2003. Elevated levels of tumor necrosis factor alpha and mortality in centenarians. Am. J. Med. 115:278–83. doi:10.1016/S0002-9343(03)00329-2.
- Carlsson, A. C., C. J. Östgren, F. H. Nystrom, T. Länne, P. Jennersjö, A. Larsson, and J. Arnlöv. 2016. Association of soluble tumor necrosis factor receptors 1 and 2 with nephropathy, cardiovascular events, and total mortality in type 2 diabetes. Cardiovasc. Diabetol. 15:1–8. doi:10.1186/s12933-016-0359-8.
- Cesari, D., A. Genga, P. Ielpo, M. Siciliano, G. Mascolo, F. M. Grasso, and D. Contini. 2014. Source apportionment of PM2.5 in the harbour-industrial area of Brindisi (Italy): Identification and estimation of the contribution of in-port ship emissions. Sci. Total Environ 497–498:392–400. doi:10.1016/j.scitotenv.2014.08.007.
- Charrier, J. G., and C. Anastasio. 2012. On dithiothreitol (DTT) as a measure of oxidative potential for ambient particles: Evidence for the importance of soluble\newline transition metals. Atmos. Chem. Phys. 12:9321–33. doi:10.5194/acp-12-9321-2012.
- Chen, J. C., and J. Schwartz. 2008. Metabolic syndrome and inflammatory responses to long-term particulate air pollutants. Environ. Health Perspect. 116:612–17. doi:10.1289/ehp.10565.
- Chen, R., Z. Zhao, Q. Sun, Z. Lin, A. Zhao, C. Wang, Y. Xi, X. Xu, and H. Kan. 2015. Size-fractionated particulate air pollution and circulating biomarkers of inflammation, coagulation, and vasoconstriction in a panel of young adults. Epidemiology 26:328–36. doi:10.1097/eDe.0000000000000273.
- Chirizzi, D., D. Cesari, M. R. Guascito, A. Dinoi, L. Giotta, A. Donateo, and D. Contini. 2017. Influence of Saharan dust outbreaks and carbon content on oxidative potential of water-soluble fractions of PM2.5 and PM10. Atmos. Environ. 163:1–8. doi:10.1016/j.atmosenv.2017.05.021.
- Clougherty, J. E. 2010. A growing role for gender analysis in air pollution epidemiology. Environ. Health Perspect. 118:167–76. doi:10.1289/ehp.0900994.
- Cohen, D. D., J. Crawford, E. Stelcer, and V. T. Bac. 2009. Characterisation and source apportionment of fine particulate sources at Hanoi from 2001 to 2008. Atmos. Environ. 44:320–28. doi:10.1016/j.atmosenv.2009.10.037.
- Crilley, L. R., F. Lucarelli, W. J. Bloss, R. M. Harrison, D. C. Beddows, G. Calzolai, S. Nava, G. Valli, V. Bernardoni, and R. Vecchi. 2017. Source apportionment of fine and coarse particles at a roadside and urban background site in London during the 2012 summer ClearfLo campaign. Environ. Pollut. 220:766–78. doi:10.1016/j.envpol.2016.06.002.
- Croft, D. P., S. J. Cameron, C. N. Morrell, C. J. Lowenstein, F. Ling, W. Zareba, P. K. Hopke, M. J. Utell, S. W. Thurston, K. Thevenet-Morrison, et al. 2017. Associations between ambient wood smoke and other particulate pollutants and biomarkers of systemic inflammation, coagulation and thrombosis in cardiac patients. Environ. Res. 154:352–61. doi:10.1016/j.envres.2017.01.027.
- Delfino, R. J., N. Staimer, T. Tjoa, A. Polidori, M. Arhami, D. L. Gillen, M. T. Kleinman, N. D. Vaziri, J. Longhurst, F. Zaldivar, et al. 2008. Circulating biomarkers of inflammation, antioxidant activity, and platelet activation are associated with primary combustion aerosols in subjects with coronary artery disease. Environ. Health Perspect. 116:898–906. doi:10.1289/ehp.11189.
- Delfino, R. J., N. Staimer, T. Tjoa, D. L. Gillen, A. Polidori, M. Arhami, M. T. Kleinman, N. D. Vaziri, J. Longhurst, and C. Sioutas. 2009. Air pollution exposures and circulating biomarkers of effect in a susceptible population: Clues to potential causal component mixtures and mechanisms. Environ. Health Perspect. 117:1232–38. doi:10.1289/ehp.0800194.
- Delfino, R. J., N. Staimer, T. Tjoa, M. Arhami, A. Polidori, D. L. Gillen, M. T. Kleinman, J. J. Schauer, and C. Sioutas. 2010a. Association of biomarkers of systemic inflammation with organic components and source tracers in quasi-ultrafine particles. Environ. Health Perspect. 118:756–62. doi:10.1289/ehp.0901407.
- Delfino, R. J., N. Staimera, T. Tjoaa, M. Arhami, A. Polidori, D. L. Gillend, S. C. George, M. M. Shafer, J. J. Schauer, and C. Sioutas. 2010b. Associations of Primary and secondary organic aerosols with airway and systemic inflammation in an elderly panel cohort. Epidemiology 21. doi:10.1097/EDE.0b013e3181f20e6c..
- Duong, T. T. T., and B. K. Lee. 2011. Determining contamination level of heavy metals in road dust from busy traffic areas with different characteristics. J. Environ. Manage. 92:554–62. doi:10.1016/j.jenvman.2010.09.010.
- Eze, I. C., M. Imboden, A. Kumar, M. Adam, A. Von Eckardstein, D. Stolz, M. W. Gerbase, N. Künzli, A. Turk, C. Schindler, et al. 2016. A common functional variant on the pro-inflammatory Interleukin-6 gene may modify the association between long-term PM10 exposure and diabetes. Environ. Heal. A Glob. Access Sci. Source 15:1–11. doi:10.1186/s12940-016-0120-5.
- Faridi, S., S. Niazi, M. Shamsipour, and M. S. Hassanvand. 2019. Comments on: “Meteorological correlates and AirQ+ health risk assessment of ambient fine particulate matter in Tehran, Iran.”. Environ. Res. 174:122–24. doi:10.1016/j.envres.2019.04.026.
- Gauderman, W. J., R. Urman, E. Avol, K. Berhane, R. McConnell, E. Rappaport, R. Chang, F. Lurmann, and F. Gilliland. 2015. Association of improved air quality with lung development in children. N. Engl. J. Med. 372:905–13. doi:10.1056/NEJMoa1414123.
- Gragnano, F., S. Sperlongano, E. Golia, F. Natale, R. Bianchi, M. Crisci, F. Fimiani, I. Pariggiano, V. Diana, A. Carbone, et al. 2017. The role of von willebrand factor in vascular inflammation: From pathogenesis to targeted therapy. Mediators Inflamm. 2017. doi:10.1155/2017/5620314.
- Green, D., L. Tian, P. Greenland, K. Liu, M. Kibbe, R. Tracy, S. Shah, J. T. Wilkins, M. D. Huffman, Y. Liao, et al. 2017. Association of the von willebrand factor-ADAMTS13 ratio with incident cardiovascular events in patients with peripheral arterial disease. Clin. Appl. Thromb. 23:807–13. doi:10.1177/1076029616655615.
- Hasheminassab, S., N. Daher, B. D. Ostro, and C. Sioutas. 2014. Long-term source apportionment of ambient fine particulate matter (PM 2.5) in the Los Angeles Basin: A focus on emissions reduction from vehicular sources. Environ. Pollut 193:54–64. doi:10.1016/j.envpol.2014.06.012.
- Hassanvand, M. S., K. Naddafi, H. Kashani, S. Faridi, N. Kunzli, R. Nabizadeh, F. Momeniha, A. Gholampour, M. Arhami, A. Zare, et al. 2017. Short-term effects of particle size fractions on circulating biomarkers of inflammation in a panel of elderly subjects and healthy young adults. Environ. Pollut. 223:695–704. doi:10.1016/j.envpol.2017.02.005.
- Hassanvand, M. S., K. Naddafi, S. Faridi, M. Arhami, R. Nabizadeh, M. H. Sowlat, Z. Pourpak, N. Rastkari, F. Momeniha, H. Kashani, et al. 2014. Indoor/outdoor relationships of PM10, PM2.5, and PM1 mass concentrations and their water-soluble ions in a retirement home and a school dormitory. Atmos. Environ. 82:375–82. doi:10.1016/j.atmosenv.2013.10.048.
- Hassanvand, M. S., K. Naddafi, S. Faridi, R. Nabizadeh, M. H. Sowlat, F. Momeniha, A. Gholampour, M. Arhami, H. Kashani, A. Zare, et al. 2015. Characterization of PAHs and metals in indoor/outdoor PM10/PM2.5/PM1 in a retirement home and a school dormitory. Sci. Total Environ. 527–528:100–10. doi:10.1016/j.scitotenv.2015.05.001.
- Heaney, M. L., and D. W. Golde. 1998. Soluble receptors in human disease. J. Leukoc. Biol. 64:135–46. doi:10.1002/jlb.64.2.135.
- Heikkilä, K., S. Ebrahim, and D. A. Lawlor. 2008. Systematic review of the association between circulating interleukin-6 (IL-6) and cancer. Eur. J. Cancer 44:937–45. doi:10.1016/j.ejca.2008.02.047.
- Hennig, F., K. Fuks, S. Moebus, G. Weinmayr, M. Memmesheimer, H. Jakobs, M. Bröcker-Preuss, D. Führer-Sakel, S. Möhlenkamp, R. Erbel, et al. 2014. Association between source-specific particulate matter air pollution and hs-CRP: Local traffic and industrial emissions. Environ. Health Perspect. 122:703–10. doi:10.1289/ehp.1307081.
- Hosseini, V., and H. Shahbazi. 2016. Urban Air Pollution in Iran. Iran. Stud. 49:1029–46. doi:10.1080/00210862.2016.1241587.
- Hosseinpoor, A. R., M. H. Forouzanfar, M. Yunesian, F. Asghari, K. H. Naieni, and D. Farhood. 2005. Air pollution and hospitalization due to angina pectoris in Tehran, Iran: A time-series study. Environ. Res. 99:126–31. doi:10.1016/j.envres.2004.12.004.
- Jacobs, L., J. Emmerechts, C. Mathieu, M. F. Hoylaerts, F. Fierens, P. H. Hoet, B. Nemery, and T. S. Nawrot. 2010. Air pollution-related prothrombotic changes in persons with diabetes. Environ. Health Perspect. 118:191–96. doi:10.1289/ehp.0900942.
- Jacobs, L., J. Emmerechts, M. F. Hoylaerts, C. Mathieu, P. H. Hoet, B. Nemery, and T. S. Nawrot. 2011. Traffic air pollution and oxidized LDL. PLoS One 6. doi:10.1371/journal.pone.0016200.
- Jeng, H. A., C. H. Pan, N. Diawara, G. P. Chang-Chien, W. Y. Lin, C. T. Huang, C. K. Ho, and M. T. Wu. 2011. Polycyclic aromatic hydrocarbon-induced oxidative stress and lipid peroxidation in relation to immunological alteration. Occup. Environ. Med. 68:653–58. doi:10.1136/oem.2010.055020.
- Jiang, H., M. Jang, T. Sabo-Attwood, and S. E. Robinson. 2016. Oxidative potential of secondary organic aerosols produced from photooxidation of different hydrocarbons using outdoor chamber under ambient sunlight. Atmos. Environ. 131:382–89. doi:10.1016/j.atmosenv.2016.02.016.
- Jiang, X., F. Xu, X. Qiu, X. Shi, M. Pardo, Y. Shang, J. Wang, Y. Rudich, and T. Zhu. 2019. Hydrophobic organic components of ambient fine particulate matter (PM 2.5) associated with inflammatory cellular response. Environ. Sci. Technol 53:10479–86. doi:10.1021/acs.est.9b02902.
- Kamath, D. Y., D. Xavier, A. Sigamani, and P. Pais. 2015. High sensitivity C-reactive protein (hsCRP) & cardiovascular disease: An Indian perspective. Indian J. Med. Res. 142:261–68. doi:10.4103/0971-5916.166582.
- Karanasiou, A. A., P. A. Siskos, and K. Eleftheriadis. 2009. Assessment of source apportionment by positive matrix factorization analysis on fine and coarse urban aerosol size fractions. Atmos. Environ. 43:3385–95. doi:10.1016/j.atmosenv.2009.03.051.
- Keuken, M. P., M. Moerman, M. Voogt, M. Blom, E. P. Weijers, T. Röckmann, and U. Dusek. 2013. Source contributions to PM2.5 and PM10 at an urban background and a street location. Atmos. Environ. 71:26–35. doi:10.1016/j.atmosenv.2013.01.032.
- Kim, H. J., M. G. Choi, M. K. Park, and Y. R. Seo. 2017a. Predictive and prognostic biomarkers of respiratory diseases due to particulate matter exposure. J. Cancer Prev. 22:6–15. doi:10.15430/jcp.2017.22.1.6.
- Kim, J. H., S. Lim, K. S. Park, H. C. Jang, and S. H. Choi. 2017b. Total and differential WBC counts are related with coronary artery atherosclerosis and increase the risk for cardiovascular disease in Koreans. PLoS One 12:1–12. doi:10.1371/journal.pone.0180332.
- Kritchevsky, S. B., M. Cesari, and M. Pahor. 2005. Inflammatory markers and cardiovascular health in older adults. Cardiovasc. Res. 66:265–75. doi:10.1016/j.cardiores.2004.12.026.
- Laskin, D. L., G. Mainelis, B. Turpin, K. J. Patel, and V. R. Sunil. 2010. Pulmonary effects of inhaled diesel exhaust in young and old mice. A Pilot Project 18:1199–216. doi:10.1016/j.micinf.2011.07.011.Innate.
- Lee, J. K., R. Bettencourt, D. Brenner, T. A. Le, E. Barrett-Connor, and R. Loomba. 2012. Association between serum interleukin-6 concentrations and mortality in older adults: The rancho bernardo study. PLoS One 7. doi:10.1371/journal.pone.0034218.
- Li, W., K. S. Dorans, E. H. Wilker, M. B. Rice, P. L. Ljungman, J. D. Schwartz, B. A. Coull, P. Koutrakis, D. R. Gold, J. F. Keaney, et al. 2017. Short-term exposure to ambient air pollution and biomarkers of systemic inflammation: The framingham heart study. Arterioscler. Thromb. Vasc. Biol. 37:1793–800. doi:10.1161/ATVBAHA.117.309799.
- Lim, J. M., J. H. Lee, J. H. Moon, Y. S. Chung, and K. H. Kim. 2010. Source apportionment of PM10 at a small industrial area using Positive Matrix Factorization. Atmos. Res. 95:88–100. doi:10.1016/j.atmosres.2009.08.009.
- Lionetto, M. G., M. R. Guascito, R. Caricato, M. E. Giordano, A. R. Bartolomeo, M. P. De, Romano, M. Conte, A. Dinoi, and D. Contini. 2019. Correlation of oxidative potential with ecotoxicological and cytotoxicological potential of PM 10 at an urban background site in Italy. Atmosphere (Basel) 10:733. doi:10.3390/atmos10120733.
- Lippmann, M. 2010. Targeting the components most responsible for airborne particulate matter health risks. J. Expo. Sci. Environ. Epidemiol. 20:117–18. doi:10.1038/jes.2010.1.
- Liu, B., J. Wu, J. Zhang, L. Wang, J. Yang, D. Liang, Q. Dai, X. Bi, Y. Feng, Y. Zhang, et al. 2017a. Characterization and source apportionment of PM 2.5 based on error estimation from EPA PMF 5.0 model at a medium city in China. Environ. Pollut. 222:10–22. doi:10.1016/j.envpol.2017.01.005.
- Liu, C., J. Cai, L. Qiao, H. Wang, W. Xu, H. Li, Z. Zhao, R. Chen, and H. Kan. 2017b. The acute effects of fine particulate matter constituents on blood inflammation and coagulation. Environ. Sci. Technol. 51:8128–37. doi:10.1021/acs.est.7b00312.
- Lowe, G., M. Woodward, G. Hillis, A. Rumley, Q. Li, S. Harrap, M. Marre, P. Hamet, A. Patel, N. Poulter, et al. 2014. Circulating inflammatory markers and the risk of vascular complications and mortality in people with type 2 diabetes and cardiovascular disease or risk factors: The advance study. Diabetes 63:1115–23. doi:10.2337/db12-1625.
- Lundstedt, S., P. A. White, C. L. Lemieux, K. D. Lynes, I. B. Lambert, L. Öberg, P. Haglund, and M. Tysklind. 2007. Sources, fate, and toxic hazards of oxygenated polycyclic aromatic hydrocarbons (PAHs) at PAH-contaminated sites. R. Swedish Acad. Sci. 36:475–85. doi:10.1579/0044-7447(2007)36[475:SFATHO]2.0.CO;2.
- Ma, H., J. Li, C. Wan, Y. Liang, X. Zhang, G. Dong, L. Hu, B. Yang, X. Zeng, T. Su, et al. 2019. Inflammation response of water-soluble fractions in atmospheric fine particulates: A seasonal observation in 10 large chinese cities. Environ. Sci. Technol. 53:3782–90. doi:10.1021/acs.est.8b05814.
- MohseniBandpi, A., A. Eslami, A. Shahsavani, F. Khodagholi, and A. Alinejad. 2017. Physicochemical characterization of ambient PM2.5in Tehran air and its potential cytotoxicity in human lung epithelial cells (A549). Sci. Total Environ 593–594:182–90. doi:10.1016/j.scitotenv.2017.03.150.
- Mostofsky, E., J. Schwartz, B. A. Coull, P. Koutrakis, G. A. Wellenius, H. H. Suh, D. R. Gold, and M. A. Mittleman. 2012. Modeling the association between particle constituents of air pollution and health outcomes several studies have shown that short-term increases in levels of fi ne ambient particulate matter (particles with Studies in which the asso. Am. J. Epidemiol. 176:317–26. doi:10.1093/aje/kws018.
- Naddafi, K., M. S. Hassanvand, M. Yunesian, F. Momeniha, R. Nabizadeh, S. Faridi, and A. Gholampour. 2012. Health impact assessment of air pollution in megacity of Tehran, Iran. J. Environ. Heal. Sci. Eng. 9:1–7. doi:10.1186/1735-2746-9-28.
- Ng, C., B. Malig, S. Hasheminassab, C. Sioutas, R. Basu, and K. Ebisu. 2017. Source apportionment of fine particulate matter and risk of term low birth weight in California: Exploring modification by region and maternal characteristics. Sci. Total Environ. 605–606:647–54. doi:10.1016/j.scitotenv.2017.06.053.
- Niu, X., S. S. H. Ho, K. F. Ho, Y. Huang, J. Sun, Q. Wang, Y. Zhou, Z. Zhao, and J. Cao. 2017. Atmospheric levels and cytotoxicity of polycyclic aromatic hydrocarbons and oxygenated-PAHs in PM2.5 in the Beijing-Tianjin-Hebei region. Environ. Pollut. 231:1075–84. doi:10.1016/j.envpol.2017.08.099.
- Norris, G., R. Duvall, S. Brown, and S. Ba, 2014. EPA Positive Matrix Factorization (PMF) 5.0 Fundamentals and User Guide 136.
- Ostro, B., B. Malig, R. Broadwin, R. Basu, E. B. Gold, J. T. Bromberger, C. Derby, S. Feinstein, G. A. Greendale, E. A. Jackson, et al. 2014. Chronic PM2.5 exposure and inflammation: Determining sensitive subgroups in mid-life women. Environ. Res. 132:168–75. doi:10.1016/j.envres.2014.03.042.
- Paatero, P. 1997. Least squares formulation of robust non-negative factor analysis. Chemom. Intell. Lab. Syst. 37:23–35. doi:10.1016/S0169-7439(96)00044-5.
- Paatero, P., S. Eberly, S. G. Brown, and G. A. Norris. 2014. Methods for estimating uncertainty in factor analytic solutions. Atmos. Meas. Tech. 7:781–97. doi:10.5194/amt-7-781-2014.
- Paatero, P., and U. Tapper. 1994. Positive matrix factorization: A non-negative factor model with optimal utilization of error estimates of data values. Environmetrics 5:111–26. doi:10.1002/env.3170050203.
- Pilz, V., K. Wolf, S. Breitner, R. Rückerl, W. Koenig, W. Rathmann, J. Cyrys, A. Peters, and A. Schneider. 2018. C-reactive protein (CRP) and long-term air pollution with a focus on ultrafine particles. Int. J. Hyg. Environ. Health 221:510–18. doi:10.1016/j.ijheh.2018.01.016.
- Pope, C. A., A. Bhatnagar, J. P. McCracken, W. Abplanalp, D. J. Conklin, and T. O’Toole. 2016. Exposure to fine particulate air pollution is associated with endothelial injury and systemic inflammation. Circ. Res. 119:1204–14. doi:10.1161/CIRCRESAHA.116.309279.
- Pope, C. A., J. B. Muhlestein, J. L. Anderson, J. B. Cannon, N. M. Hales, K. G. Meredith, V. Le, and B. D. Horne. 2015. Short-term exposure to fine particulate matter air pollution is preferentially associated with the risk of ST-segment elevation acute coronary events. J. Am. Heart Assoc. 4:1–10. doi:10.1161/JAHA.115.002506.
- Quay, J. L., W. Reed, J. Samet, and R. B. Devlin. 1998. Air pollution particles induce IL-6 gene expression in human airway epithelial cells via NF-κB activation. Am. J. Respir. Cell Mol. Biol. 19:98–106. doi:10.1165/ajrcmb.19.1.3132.
- Reff, A., S. I. Eberly, and P. V. Bhave. 2007. Receptor modeling of ambient particulate matter data using positive matrix factorization: Review of existing methods. J. Air Waste Manag. Assoc. 57:146–54. doi:10.1080/10473289.2007.10465319.
- Ribeiro, C. M., S. R. Oliveira, D. F. Alfieri, T. Flauzino, D. R. Kaimen-Maciel, A. N. C. Simão, M. Maes, and E. M. V. Reiche. 2019. Tumor necrosis factor alpha (TNF-α) and its soluble receptors are associated with disability, disability progression and clinical forms of multiple sclerosis. Inflamm. Res 1–11. doi:10.1007/s00011-019-01286-0.
- Rich, D. Q., H. Özkaynak, J. Crooks, L. Baxter, J. Burke, P. Ohman-Strickland, K. Thevenet-Morrison, H. M. Kipen, J. Zhang, J. B. Kostis, et al. 2013. The triggering of myocardial infarction by fine particles is enhanced when particles are enriched in secondary species. Environ. Sci. Technol. 47:9414–23. doi:10.1021/es4027248.
- Rückerl, R., R. Hampel, S. Breitner, J. Cyrys, U. Kraus, J. Carter, L. Dailey, R. B. Devlin, D. Diaz-Sanchez, W. Koenig, et al. 2014. Associations between ambient air pollution and blood markers of inflammation and coagulation/fibrinolysis in susceptible populations. Environ. Int. 70:32–49. doi:10.1016/j.envint.2014.05.013.
- Samara, C. 2017. On the redox activity of urban aerosol particles: Implications for size distribution and relationships with organic aerosol components. Atmosphere (Basel) 8. doi:10.3390/atmos8100205.
- Shahbazi, H., M. Reyhanian, V. Hosseini, and H. Afshin. 2016a. The relative contributions of mobile sources to air pollutant emissions in Tehran, Iran: An emission inventory approach. Emiss. Control Sci. Technol. 2:44–56. doi:10.1007/s40825-015-0031-x.
- Shahbazi, H., S. Taghvaee, V. Hosseini, and H. Afshin. 2016b. A GIS based emission inventory development for Tehran. Urban Clim 17:216–29. doi:10.1016/j.uclim.2016.08.005.
- Sheng, K., and J. Lu. 2017. Typical airborne quinones modulate oxidative stress and cytokine expression in lung epithelial A549 cells. J. Environ. Sci. Heal. - Part A Toxic/Hazardous Subst. Environ. Eng. 52:127–34. doi:10.1080/10934529.2016.1237127.
- Simoneit, B. R., Schauer, J. J., Nolte, C. G., Oros, D. R., Elias, V. O., Fraser, M. P., ... & Cass, G. R. (1999). Levoglucosan, a tracer for cellulose in biomass burning and atmospheric particles. Atmospheric Environment, 33(2), 173–182
- Siponen, T., T. Yli-Tuomi, M. Aurela, H. Dufva, R. Hillamo, M. R. Hirvonen, K. Huttunen, J. Pekkanen, A. Pennanen, I. Salonen, et al. 2015. Source-specific fine particulate air pollution and systemic inflammation in ischaemic heart disease patients. Occup. Environ. Med. 72:277–83. doi:10.1136/oemed-2014-102240.
- Snyder, D. C., A. P. Rutter, R. Collins, C. Worley, and J. J. Schauer. 2009. Insights into the origin of water soluble organic carbon in atmospheric fine particulate matter. Aerosol Sci. Technol. 43:1099–107. doi:10.1080/02786820903188701.
- Soleimanian, E., A. Mousavi, S. Taghvaee, M. H. Sowlat, S. Hasheminassab, A. Polidori, and C. Sioutas. 2019a. Spatial trends and sources of PM2.5 organic carbon volatility fractions (OCx) across the Los Angeles Basin. Atmos. Environ 209:201–11. doi:10.1016/j.atmosenv.2019.04.027.
- Soleimanian, E., S. Taghvaee, A. Mousavi, M. Sowlat, M. Hassanvand, M. Yunesian, K. Naddafi, and C. Sioutas. 2019b. Sources and temporal variations of coarse particulate matter (PM) in Central Tehran, Iran. Atmosphere (Basel) 10:291. doi:10.3390/atmos10050291.
- Song, Y., Y. Zhang, S. Xie, L. Zeng, M. Zheng, L. G. Salmon, M. Shao, and S. Slanina. 2006. Source apportionment of PM2.5 in Beijing by positive matrix factorization. Atmos. Environ. 40:1526–37. doi:10.1016/j.atmosenv.2005.10.039.
- Steenhof, M., N. A. H. Janssen, M. Strak, G. Hoek, I. Gosens, I. S. Mudway, F. J. Kelly, R. M. Harrison, R. H. H. Pieters, F. R. Cassee, et al. 2014. Air pollution exposure affects circulating white blood cell counts in healthy subjects: The role of particle composition, oxidative potential and gaseous pollutants-the RAPTES project. Inhal. Toxicol. 26:141–65. doi:10.3109/08958378.2013.861884.
- Su, T. C., J. J. Hwang, Y. R. Yang, and C. C. Chan. 2017. Association between long-term exposure to traffic-related air pollution and inflammatory and thrombotic markers in middle-aged adults. Epidemiology 28:S74–S81. doi:10.1097/EDE.0000000000000715.
- Sun, X., X. Luo, C. Zhao, B. Zhang, J. Tao, Z. Yang, W. Ma, and T. Liu. 2016. The associations between birth weight and exposure to fine particulate matter (PM2.5) and its chemical constituents during pregnancy: A meta-analysis. Environ. Pollut 211:38–47. doi:10.1016/j.envpol.2015.12.022.
- Taghvaee, S., M. H. Sowlat, A. Mousavi, M. S. Hassanvand, M. Yunesian, K. Naddafi, and C. Sioutas. 2018. Source apportionment of ambient PM2.5 in two locations in central Tehran using the Positive Matrix Factorization (PMF) model. Sci. Total Environ 628–629:672–86. doi:10.1016/j.scitotenv.2018.02.096.
- Tuet, W. Y., Y. Chen, L. Xu, S. Fok, D. Gao, R. J. Weber, and N. L. Ng. 2017. Chemical oxidative potential of secondary organic aerosol (SOA) generated from the photooxidation of biogenic and anthropogenic volatile organic compounds. Atmos. Chem. Phys. 17:839–53. doi:10.5194/acp-17-839-2017.
- Velde, A. R., W. C. van Der, Meijers, and R. A. de Boer. 2014. Biomarkers for risk prediction in acute decompensated heart failure. Curr. Heart Fail. Rep. 11:246–59. doi:10.1007/s11897-014-0207-7.
- Veranth, J. M., T. A. Moss, J. C. Chow, R. Labban, W. K. Nichols, J. C. Walton, J. G. Watson, and G. S. Yost. 2006. Correlation of in vitro cytokine responses with the chemical composition of soil-derived particulate matter. Environ. Health Perspect. 114:341–49. doi:10.1289/ehp.8360.
- Verma, V., R. Rico-Martinez, N. Kotra, L. King, J. Liu, T. W. Snell, and R. J. Weber. 2012. Contribution of water-soluble and insoluble components and their hydrophobic/hydrophilic subfractions to the reactive oxygen species-generating potential of fine ambient aerosols. Environ. Sci. Technol. 46:11384–92. doi:10.1021/es302484r.
- Vreeland, H., R. Weber, M. Bergin, R. Greenwald, R. Golan, A. G. Russell, V. Verma, and J. A. Sarnat. 2017. Oxidative potential of PM 2.5 during Atlanta rush hour: Measurements of in-vehicle dithiothreitol (DTT) activity. Atmos. Environ. 165:169–78. doi:10.1016/j.atmosenv.2017.06.044.
- Wang, C. F., C. Y. Chang, S. F. Tsai, and H. L. Chiang. 2005. Characteristics of road dust from different sampling sites in northern taiwan. J. Air Waste Manag. Assoc. 55:1236–44. doi:10.1080/10473289.2005.10464717.
- WHO. 2018. World health statistics, 2018: monitoring health for the SDGs, sustainable development goals. Geneva.
- Wu, S., F. Deng, H. Wei, J. Huang, H. Wang, M. Shima, X. Wang, Y. Qin, C. Zheng, Y. Hao, et al. 2012. Chemical constituents of ambient particulate air pollution and biomarkers of inflammation, coagulation and homocysteine in healthy adults: A prospective panel study. Part. Fibre Toxicol. 9:1–13. doi:10.1186/1743-8977-9-49.
- Wu, S., F. Deng, H. Wei, J. Huang, X. Wang, Y. Hao, C. Zheng, Y. Qin, H. Lv, M. Shima, et al. 2014. Association of cardiopulmonary health effects with source-appointed ambient fine particulate in Beijing, China: A combined analysis from the healthy volunteer natural relocation (HVNR) study. Environ. Sci. Technol. 48:3438–48. doi:10.1021/es404778w.
- Yuan, Z., Y. Chen, Y. Zhang, H. Liu, Q. Liu, J. Zhao, M. Hu, W. Huang, G. Wang, T. Zhu, et al. 2013. Changes of plasma vWF level in response to the improvement of air quality: An observation of 114 healthy young adults. Ann. Hematol. 92:543–48. doi:10.1007/s00277-013-1679-3.
- Yue, W., A. Schneider, M. Stölzel, R. Rückerl, J. Cyrys, X. Pan, W. Zareba, W. Koenig, H. E. Wichmann, and A. Peters. 2007. Ambient source-specific particles are associated with prolonged repolarization and increased levels of inflammation in male coronary artery disease patients. Mutat. Res. - Fundam. Mol. Mech. Mutagen. 621:50–60. doi:10.1016/j.mrfmmm.2007.02.009.
- Zhang, J., J. Gong, T. Zhu, H. Kipen, G. Wang, M. Hu, P. Ohman-Strickland, S. E. Lu, L. Zhang, Y. Wang, et al. 2013a. Cardiorespiratory biomarker responses in healthy young adults to drastic air quality changes surrounding the 2008 beijing olympics. J. Expo. Sci. Environ. Epidemiol. 23:322–27. doi:10.1038/jes.2012.127.
- Zhang, R., J. Jing, J. Tao, S. C. Hsu, G. Wang, J. Cao, C. S. L. Lee, L. Zhu, Z. Chen, Y. Zhao, et al. 2013b. Chemical characterization and source apportionment of PM2.5 in Beijing: Seasonal perspective. Atmos. Chem. Phys. 13:7053–74. doi:10.5194/acp-13-7053-2013.
- Zhang, Z., G. Hoek, L. Y. Chang, T. C. Chan, C. Guo, Y. C. Chuang, J. Chan, C. Lin, W. K. Jiang, Y. Guo, et al. 2018. Particulate matter air pollution, physical activity and systemic inflammation in Taiwanese adults. Int. J. Hyg. Environ. Health 221:41–47. doi:10.1016/j.ijheh.2017.10.001.
- Zong, Z., X. Wang, C. Tian, Y. Chen, L. Qu, L. Ji, G. Zhi, J. Li, and G. Zhang. 2016. Source apportionment of PM2.5 at a regional background site in North China using PMF linked with radiocarbon analysis: Insight into the contribution of biomass burning. Atmos. Chem. Phys. 16:11249–65. doi:10.5194/acp-16-11249-2016.