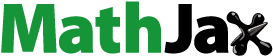
ABSTRACT
This study used fine and coarse PM concentrator technology in a Mobile Particle Concentrator Platform (MPCP) designed and built to allow the collection of large amounts of ambient PM, enabling time-resolved speciation analysis, which would not be feasible using conventional methods. One hour of sampling yielded sufficient sample loading for trace elemental analysis using X-Ray Fluorescence (XRF). In addition, we developed a novel Road Dust Aerosolizer (RDA) sampler in order to collect PM2.5 and PM10 surface road dust in situ. This sampler aerosolizes dust from the road surface, simulating ambient road dust resuspension, resulting in measured PM composition and size more appropriately (and less labor-intensive) than those obtained from studies using bulk road dust sieved and re-suspended in the laboratory. Overall, our modified fine and coarse particle concentrators yielded good reproducibility between co-located samples and sufficient loading for trace elemental analysis. For particle mass concentration, we observed a relative error of 3% and 4% among pairs of filters for fine and coarse concentrators, respectively; confirming that the mass collected on an unweighted quartz filter in parallel with a Teflon filter will have the same PM mass as the weighed Teflon filter. For samples with elements that are well above the LOD, relative uncertainty values were between 5% and 10% for the fine and 3% and 10% for the coarse. Our results show that the RDA system has an excellent precision for mass and elements as well. The relative error for mass is 7% for PM10 and 3% for PM2.5 within pairs and ranged from 2 to10% for elements. In conclusion, we developed a method for collecting PM10 and PM2.5 near-road air and surface road dust for short durations, which allows investigation of the composition of direct (airborne) and indirect (re-suspended road dust) non-tailpipe vehicular emissions.
Implications: The methods we developed in this study allow the collection of one-hour PM10-2.5 and PM2.5–0.2 samples from near-road ambient air at several distances from the same roadway in 1 day, and collection of road dust directly from the road surface, with sufficient loading for trace elemental analysis. This will allow investigation of the composition of direct (airborne) and indirect (re-suspended road dust) vehicular emissions.
Introduction
Health effects of air pollutants have been studied for a long time, and it has been shown that traffic emissions impact individuals living and/or commuting on or near busy roads (Hamra et al. Citation2015; Hankey, Lindsey, and Marshall Citation2017; Huang et al. Citation2018; Kim et al. Citation2004; Puett et al. Citation2014; Zhang and Batterman Citation2013). To better understand the relation between exposures and health effects, near-road air pollutant exposures have been measured by numerous research groups in the U.S. and abroad. Many of these studies have been presented in a review paper by Pant and Harrison (Citation2013). Several groups have used mobile platforms equipped with sophisticated real-time monitors that measure ambient particle matter (PM) mass, number, and size, as well as criteria gases (Adams and Corr Citation2019; Apte et al. Citation2017; Kozawa et al. Citation2014; Larson et al. Citation2017; Riley et al. Citation2014, Citation2016; Shairsingh et al. Citation2018; Shi, Lau, and Ng Citation2016; Tao et al. Citation2015; Tessum et al. Citation2018; Westerdahl et al. Citation2005). However, the ability of these mobile platforms to characterize the temporal and spatial patterns of PM species is limited.
In some cases, small-integrated samplers have been used, but these operate at relatively low flow rates and require many hours of sampling to collect enough material for speciation analysis (Kam et al. Citation2012a, Citation2012b). Portable time-of-flight mass spectrometers have been used onboard mobile platforms to measure some PM species; however, these instruments measure only some non-refractory elements and do not measure many elements that are tracers for vehicular and non-vehicular sources (Salcedo et al. Citation2012). Near-road samples, collected by many studies at stationary sites, have provided exhaustive characterizations of PM and gaseous pollutants. However, investigation of the spatial patterns of traffic exposures requires PM speciation measurements at many locations, which is costly and impractical using currently available methods.
To overcome these limitations, we designed and built a Mobile Particle Concentrator Platform (MPCP), which is equipped with fine and coarse ambient particle concentrators that collect PM at a very high flow rate (2,200 LPM for the fine and 5,000 LPM for the coarse). Initially, our group developed these technologies for human and animal inhalation toxicological studies, which require high concentrations of airborne PM (Sioutas et al. Citation1997; Lawrence et al. Citation2004; Demokritou et al. Citation2002a), and we modified them for use in this platform.
In addition to near-road air sampling of traffic-related air pollution, researchers have been trying to understand the non-tailpipe emissions, such as tire and brake wear that can be generated by vehicles (direct emissions) or re-suspended from the roads by traffic (indirect emission) (Harrison et al. Citation2012; Hjortenkrans, Bergbäck, and Häggerud Citation2007; Jayarathne et al. Citation2018; Kam et al. Citation2012a; Khan et al. Citation2018; Zhang et al. Citation2018). This is of particular interest because exhaust (tailpipe) emissions have been reduced over the last several years due to regulations. Consequently, non-tailpipe emissions represent an increasing fraction of traffic-related airborne PM. Despite the tailpipe reductions, there is still a strong association of traffic-related PM and health effects (Huang et al. Citation2018; Jayarathne et al. Citation2018; Khan et al. Citation2018; Khan and Strand Citation2018; Men et al. Citation2018).
There are different methods to collect Road Dust as shown in a recent review paper by Khan and Strand (Citation2018), and the primary method reported in the literature to collect road dust has been to sweep the roads with brushes (Apeagyei, Bank, and Spengler Citation2011; Cao et al. Citation2017; Chang et al. Citation2009; Khpalwak et al. Citation2019; Lee et al. Citation2012; Li et al. Citation2018; Liu et al. Citation2014; Lorenzi et al. Citation2011; Men et al. Citation2018; Pan, Lu, and Lei Citation2017; Potgieter-Vermaak et al. Citation2012; Soltani et al. Citation2015; Thorpe and Harrison Citation2008; Valotto et al. Citation2015; Xu et al. Citation2013; Yu et al. Citation2014). Only a few studies used vacuum cleaners (Alves et al. Citation2018; Amato et al. Citation2009; Campen et al. Citation2006; Jayarathne et al. Citation2017, Citation2018; Zhang et al. Citation2018). Subsequently, these samples were usually re-suspended in the laboratory where size fractionated PM was collected. For this study, we developed a sampler that aerosolizes and collects PM2.5 and PM10 from surface road dust in situ. Thus, it simulates ambient road dust resuspension, resulting in a more realistic measurement of PM composition and size. An additional feature of this method is that it is less labor-intensive than those using bulk road dust sieved and re-suspended in the laboratory. Moreover, sieving separates by physical size, which does not correspond to aerodynamic diameter, which is usually larger than physical diameter due to particle density typically greater than unity. Thus for separation by sieving, some particles that are larger than either 2.5 or 10 µm aerodynamic diameter are misclassified as being in either of the two size categories. Finally, our new Road Dust Aerosolizer (RDA) collects dust PM directly onto filters, which are then analyzed in the same way as those used for directly sampling ambient PM.
Using both the MPCP and the RDA sampler together, we were able to collect paired short-duration samples of ambient PM and road dust in two size fractions on the same day at several locations. We sampled at three different distances from a major roadway during each day of the study. Samples were collected at the roadside (0–50 m), at an intermediate location (51–200 m) and at background site (500–900 m) for several different highways and heavily traveled roads.
Before conducting the field study, we optimized our mobile platform, RDA sampler, and our sampling techniques. This paper reports on the equipment design and validation.
Methods
A 16-foot box truck was modified for this study as a Mobile Particle Concentrator Platform (MPCP). The MPCP was equipped with fine (PM0.2 to 2.5 µm) and coarse (PM2.5 to 10 µm) particle concentrators, as well as (non-concentrator) air samplers for PM0.2, PM2.5, and PM10. A Road Dust Aerosolizer sampler (RDA) was designed and constructed to simultaneously re-suspend road dust and separate it into fine and coarse fractions. The characterization tests of the MPCP and RDA were conducted on 5 days. The samples were collected during the late morning, middle of the day, and during the late afternoon.
For electric power supply, a Tier-4 generator (Power Tech – PTS30) was chosen due to its low PM emissions according to EPA standards (0.03 g/kW-hr of PM; EPA Citation2004). During sampling, we parked the Mobile platform with the concentrator inlets facing the wind. The generator exhaust was located at the opposite end of the platform from the inlet of the samplers. A flexible exhaust extension was used to direct the exhaust downwind of the MPCP. Continuous NO2 measurements during sampling were used to monitor for impact of generator exhaust.
A picture of the MPCP is presented in and b, and a schematic drawing in .
Mobile Particle Concentrator Platform (MPCP)
A modified Harvard Ambient Fine Particle Concentrator (HAFPC) system (Lawrence et al. Citation2004; Sioutas et al. Citation1997) was used to concentrate and collect ambient PM2.5. Briefly, the concentrator enriches ambient PM2.5 (0.2–2.5 µm) from ambient air, effectively removing a large fraction of the gas phase, thus increasing particle mass concentrations while keeping particles airborne, without substantially changing their physicochemical characteristics (Lawrence et al. Citation2004; Sioutas et al. Citation1997). The HAFPC uses virtual impaction (Loo and Cork Citation1988) to concentrate particle mass from ambient air, separating particles from the surrounding gases by particulate inertia. Ambient air is accelerated through a slit-shaped nozzle. A collection slit directly downstream of the acceleration nozzle collects 20% of the flow. Particles larger than the impactor cut point (0.2 µm aerodynamic diameter), have sufficient momentum to cross the streamlines created in the air flow between the acceleration and collection nozzles. These larger particles pass into the collection nozzle (minor flow), and thus are concentrated nominally 5 times higher than in the ambient air. The remaining 80% of the flow passes around the collection slit and contains only particles below the cut-point (major flow).
The original HAFPC system used three stages in series and required a very large blower to accelerate air through slits narrow enough to provide a cut-point of 0.2 µm at a flowrate of 5,500 LPM. Because the system used in a mobile platform, it was necessary to reduce the electric power requirement. Therefore, we used a modified HAFPC system that has two parallel two-stage concentrators whose outputs were combined and well mixed. Subsequently, two samples were collected simultaneously on 47 mm Teflon and/or Quartz fiber filters, at 45 LPM each. Each of the two-stage concentrators was equipped with a modified high-volume cascade impactor (HVCI) PM2.5 stage (Demokritou et al. Citation2002c) as a size selective inlet (SSI) to remove particles with an aerodynamic diameter greater than 2.5 µm. The intake flowrate for each concentrator was 1,100 LPM, for a combined intake flowrate of 2,200 LPM.
The performance of the SSI and the concentrators stages were tested separately in the laboratory using an aerosol generator system (Demokritou et al. Citation2004), aluminum ducts (6 inches diameter), isokinetic probes (before and after the tested unit), and an aerodynamic particle sizer (APS, TSI Model 3310). The laboratory test systems were configured the same way as the field systems.
A Harvard Ambient Coarse Particle Concentrator (HACPC) system was used to collect ambient coarse particles (PM2.5–10) (Demokritou et al. Citation2002a). The HACPC uses a size-selective inlet to remove particles with an aerodynamic diameter greater than 10 µm (Demokritou et al. Citation2003).
The HACPC intake flow is 5,000 LPM, and after two virtual impactor stages in series, with minor flows of 10% each, the output is approximately 50 LPM. The output flow was divided to collect two coarse particle samples simultaneously, on Teflon and/or pre-fired quartz fiber filters at 25 LPM each.
The HAFPC collects but does not concentrate PM below 0.2 µm. Because installing an ultrafine particle concentrator (Demokritou, Gupta, and Koutrakis Citation2002b) in the mobile platform was not possible due to constraints of time, space, power, and expense, the MCPC is equipped also with PM0.2 samplers (Demokritou et al. Citation2004) that are used to collect pooled samples of roadside, intermediate and background ultrafine PM during our field study. These samplers were not developed or modified as part of this project and are not discussed here.
Analytical methods
For equipment validation, only Teflon filters were used as duplicate samples to ensure the similarity of the parallel samples. The particle mass concentration was determined by gravimetric analysis and trace elemental composition by X-Ray Fluorescence (XRF) analysis. A microbalance (MT-5 Mettler-Toledo, Columbus, Ohio) in a temperature and humidity-controlled room was used for gravimetric analysis. XRF was performed using a PANalytical Epsilon 5 spectrometer (Malvern-PANalytical, Almelo, the Netherlands).
Concentrator calibration using concentration enrichment factor (CEF)
Since, as discussed above, it is not feasible to collect enough ambient particles with short-duration samples to be able to do speciation, speciation was only done for the concentrated samples. To estimate the ambient PM concentration it is necessary to determine the Concentration Enrichment Factor (CEF). Because the CEF can show temporal and geographic variability depending on the ambient particle size distribution and other parameters (Lawrence et al. Citation2004), we determined the CEF for each concentrator sampling period at each location. To do so, we simultaneously collected non-concentrated ambient PM2.5 and PM10 samples at a flow rate of 90 and 60 LPM, respectively. The mass loading of these non-concentrated ambient samples was sufficient for quantitative gravimetric analysis, but inadequate for speciation analysis, since most individual components represent a small fraction of the particle mass. The CEF for coarse and fine PM concentrators for each sampling period is determined using the ratio of the concentrated mass concentration (PM) and the ambient mass concentration (PMa).
For the HACPC, PMa is obtained by subtracting the ambient PM2.5 from the ambient PM10. The CEF for each sample is then used to determine the elemental composition of both, ambient coarse and fine PM, based on XRF analysis of the concentrated samples.
Road Dust Aerosolizer (RDA)
The RDA draws dust from the surface of each roadway vertically through an antistatic hose into a stainless-steel settling chamber at a flow rate of 100 CFM (2,830 LPM). Inside the chamber, the larger debris settles, and the remaining airborne dust passes through a stainless-steel screen (1/4” mesh) to remove lighter large debris, such as paper, plastic, or leaf bits. From there, an isokinetic probe was utilized to sample 300 LPM which flowed into a size selective inlet (SSI) that removes particles with an aerodynamic diameter larger than 10 µm. The excess flow was discarded.
After the SSI, the flow was split in half, using a custom-designed Y-divider with a 15° angle to minimize particle losses. From one channel an isokinetic probe was used to collect PM10 on a 47 mm filter at 15 LPM. Another isokinetic probe on the other channel was used with a conventional 30 LPM impactor (with an impaction stage to remove particles above 2.5 µm) to collect PM2.5 at 30 LPM. PM samples were collected on Teflon/or quartz fiber filters. Two photos and a schematic drawing of the RDA are shown in , respectively. For the validation tests, duplicate Teflon filters were used. A five-minute sampling time was sufficient to achieve quantitative filter loadings for gravimetric and trace elemental analysis, and the area vacuumed was approximately 12 m2 or 129 ft2.
The RDA flows were measured using calibrated orifices (orifice meters), which consist of a thin plate with an orifice in the center that is inserted onto the flow stream causing a pressure differential across the plate that varies consistently with flow (Hinds Citation1999). We calibrated these in situ () with a mass flow meter (TSI-4000 series). For road dust, elemental concentrations were calculated as fractions of the net mass (µg of element/mg of road dust).
Determining measurement uncertainties
We evaluated the performance of the mobile platform and the RDA using a series of duplicate samples to assess the measurement uncertainty. These uncertainties were estimated using the Root Mean Square Error (RMSE) of the duplicate samples, based on the difference between the sample measurement and the mean of each pair.
Where:
a = channel a of the pair
b = channel b of the pair
N = number of pairs
Due to the range in concentrations of our duplicate measurements (different days or location of collection), we calculated the relative uncertainty (δ), dividing the RMSE by the overall average of all measurements for that species and multiplying by 100 to get the percentage uncertainty.
Results and discussion
For method evaluation, we collected five pairs of duplicate ambient PM samples using the coarse and fine particle concentrators and eight pairs of duplicate road dust PM samples using the RDA. In this section, we include only results for pairs where both samples are above the limit of detection (LOD) for an element. The number of pairs shown differs between elements due to variation in the composition or amount of material collected between the days sampled.
Fine particle concentrator
The Concentrated Ambient Particles (CAPs) PM2.5 average concentration, standard deviation (SD), average LOD (calculated based on two times the uncertainty for each element), RMSE, and the uncertainty (δ) for mass and each element are presented in . Overall, our modified fine particle concentrator yielded good reproducibility between duplicate samples with sufficient loading for trace elemental analysis. For particle mass concentration, we observed a relative error of 3% and 4% among pairs of filters for fine and coarse concentrators, respectively. Thus, the mass collected on a quartz filter sampled in parallel with a Teflon filter will be the same as the mass collected on the Teflon filter, which is determined gravimetrically. The method in general gives excellent precision for mass and for elements that are at levels above the LOD (based on two times the XRF uncertainty for each element). For elements that are well above LOD in all five samples, relative uncertainty values were between 5% and 10%. When the elements are below LOD in one or more samples and the RMSE is based on fewer sample pairs (indicated with asterisk in ), or when the concentrations are relatively low, the relative uncertainties are generally higher.
Table 1. Harvard ambient fine particle concentrator (PM0.2–2.5)
The CEF range for the HAFPC during the days that these duplicate samples were collected were between 5.0 and 7.4 times.
Coarse particle concentrator
Concentrated ambient coarse PM duplicate filters were sampled at 25 LPM using the HACPC system. The CAPs average concentration with the SD, the concentration range, the average LOD, RMSE, and the relative uncertainty (δ) for mass and each element are presented in . Results from the HACPC were consistent with those of the HAFPC, with low overall relative error among pairs of filters (4% for PM, and 3–10% for elements), indicating that the filters collected simultaneously on the system could be compared. As for fine CAPS, when the elements were below LOD in one or two sample pairs, the RMSE was calculated using fewer samples (indicated with asterisk in ).
Table 2. Harvard ambient coarse particle concentrator (PM2.5–10)
The CEF range for the HACPC during the days that these duplicate samples were collected were between 50 and 96 times.
Road Dust Aerosolizer (RDA) sampler
Similar testing was performed on the RDA sampler where duplicate filters were collected for both PM10 and PM2.5. Due to the collection method (directly vacuuming the road surface), the loadings on the filters were higher as compared to those of the air samples collected by the concentrators, even with a very short duration of sampling (5 min).
show the average filter loading (mg/filter) and the mass fraction (µg/mg PM), SD, mass fraction range, average LOD, RMSE, and the relative uncertainty (δ) for RDA PM10 and RDA PM2.5, respectively. As with the HAFPC and HACPC filters, elements in with higher relative uncertainty are generally those that are below the LOD in two or more sample pairs, indicated by asterisk, and where the mass fraction is relatively small.
Table 3. Road dust aerosolizer – PM10.
Table 4. Road Dust Aerosolizer - PM2.5
Our results show that the RDA system also has an excellent precision for mass and elements. The relative error for mass is 7% for PM10 and 3% for PM2.5 within pairs and ranged from 1% to 13% for elements.
Conclusions
We have developed methods for collecting short-duration, near-road samples of coarse and fine ambient PM with enough sample loading for speciation analysis, including determination of trace elements by XRF and elemental and organic carbon fractions by Thermo-Optical methods. In addition, we have developed a sampler which aerosolizes and collects PM10 and PM2.5 from surface road dust in situ. Used together, these methods can enable the investigation of the impacts of direct and indirect vehicular emissions from major and minor roadways on air quality within a community. Short duration measurements of ambient fine and coarse PM and road dust at multiple distances from the major road in the same day could provide information on how different roadway types or traffic conditions influence air quality and exposure. In addition, with a sufficiently large number of measurements, these data could be used in conjunction with factor analysis or source apportionment techniques to investigate the composition of direct and indirect vehicular emissions, including tailpipe and non-tailpipe sources.
Both the Road Dust Aerosolizer (RDA) and the Coarse and Fine particle concentrators mounted in our Mobile Particle Concentrator Platform collect filter samples with excellent precision for particle mass and for elements that were present at levels above the LOD.
The mobile particle concentrator platform and RDA sampler were employed in a field study, the results of which will be reported in subsequent publications.
Acknowledgments
Research described in this article was conducted under contract to the Health Effects Institute (HEI), an organization jointly funded by the United States Environmental Protection Agency (US EPA) (Assistance Award No. CR-83467701) and certain motor vehicle and engine manufacturers. The contents of this article do not necessarily reflect the views of HEI, or its sponsors, nor do they necessarily reflect the views and policies of the EPA or motor vehicle and engine manufacturers.
This publication was made possible by US EPA grant RD-83587201. Its contents are solely the responsibility of the grantee and do not necessarily represent the official views of the US EPA. Further, US EPA does not endorse the purchase of any commercial products or services mentioned in the publication.
We thank Prof. Jack Harkema from Michigan State University for lending us the coarse concentrator used in this study.
Disclosure statement
No potential conflict of interest was reported by the authors.
Additional information
Funding
Notes on contributors
Marco Martins
Marco Martins is a Researcher Associate in the Exposure, Epidemiology, and Risk Program of the Department of Environmental Health at Harvard T.H. Chan School of Public Health.
Joy Lawrence
Joy Lawrence is a Researcher Associate in the Exposure, Epidemiology, and Risk Program of the Department of Environmental Health at Harvard T.H. Chan School of Public Health.
Stephen Ferguson
Stephen Ferguson is a Engineer Supervisor in the Exposure, Epidemiology, and Risk Program of the Department of Environmental Health at Harvard T.H. Chan School of Public Health.
Jack M. Wolfson
Jack M. Wolfson is a Researcher Associate in the Exposure, Epidemiology, and Risk Program of the Department of Environmental Health at Harvard T.H. Chan School of Public Health.
Petros Koutrakis
Petros Koutrakis is a Professor of Environmental Sciences in the Department of Environmental Health at Harvard T.H. Chan School of Public Health.
References
- Adams, M. D., and D. Corr. 2019. A mobile air pollution monitoring data set. Data 4 (2):1–8. doi:10.3390/data4010002.
- Alves, C. A., M. Evtyugina, A. M. P. Vicente, E. D. Vicente, T. V. Nunes, P. M. A. Silva, M. A. C. Duarte, C. A. Pio, F. Amato, and X. Querol. 2018. Chemical profiling of PM 10 from Urban Road Dust. Sci. Total Environ. 634:41–51. doi:10.1016/j.scitotenv.2018.03.338.
- Amato, F., M. Pandolfi, M. Viana, X. Querol, A. Alastuey, and T. Moreno. 2009. Spatial and chemical patterns of PM10 in road dust deposited in urban environment. Atmos. Environ. 43:1650–59. doi:10.1016/j.atmosenv.2008.12.009.
- Apeagyei, E., M. S. Bank, and J. D. Spengler. 2011. Distribution of heavy metals in road dust along an urban-rural gradient in Massachusetts. Atmos. Environ. 45 (13):2310–23. doi:10.1016/j.atmosenv.2010.11.015.
- Apte, J. S., K. P. Messier, S. Gani, M. Brauer, T. W. Kirchstetter, M. M. Lunden, J. D. Marshall, C. J. Portier, R. C. H. Vermeulen, and S. P. Hamburg. 2017. High-resolution air pollution mapping with Google Street view cars: Exploiting Big Data. Environ. Sci. Technol. 51:6999–7008. doi:10.1021/acs.est.7b00891.
- Campen, M. J., J. D. McDonald, M. D. Reed, and J. Seagrave. 2006. Fresh gasoline emissions, not paved road dust, alter cardiac repolarization in ApoE−/− mice. Cardiovasc. Toxicol. 6:199–209. doi:10.1385/CT:6:3:199.
- Cao, Z., L. Zhao, J. Kuang, Q. Chen, G. Zhu, K. Zhang, S. Wang, X. Zhang, X. Wang, S. Harrad, and J. Sun. 2017. Vehicles as outdoor BFR sources: Evidence from an investigation of BFR occurrence in ROAD DUST. Chemosphere 179:29–36. doi:10.1016/j.chemosphere.2017.03.095.
- Chang, S., K. Wang, H. Chang, W. Ni, B. Wu, R. Wong, and H. Lee. 2009. Comparison of source identification of metals in road-dust and soil. Soil Sediment Contam. Int. J. 18:669–83. doi:10.1080/15320380903085691.
- Demokritou, P., T. Gupta, S. Ferguson, and P. Koutrakis. 2002a. Development and laboratory characterization of a prototype coarse particle concentrator for inhalation toxicological studies. J. Aerosol Sci. 33 (8):1111–23. doi:10.1016/S0021-8502(02)00064-2.
- Demokritou, P., T. Gupta, S. Ferguson, and P. Koutrakis. 2003. Development of a high-volume Concentrated Ambient Particles System (CAPS) for human and animal inhalation toxicological studies. Inhal Toxicol 15 (2):111–29. doi:10.1080/08958370304475.
- Demokritou, P., T. Gupta, and P. Koutrakis. 2002b. A high volume apparatus for the condensational growth of ultrafine particles for inhalation toxicological studies. Aerosol Sci. Technol. 36 (11):1061–72. doi:10.1080/02786820290092230.
- Demokritou, P., I. G. Kavouras, S. T. Ferguson, and P. Koutrakis. 2002c. Development of a high volume cascade impactor for toxicological and chemical characterization studies. Aerosol Sci. Technol. 36 (9):925–33. doi:10.1080/02786820290092113.
- Demokritou, P., S. J. Lee, S. Ferguson, and P. Koutrakis. 2004. A compact multistage (Cascade) impactor for the characterization of atmospheric aerosols. J. Aerosol Sci. 35 (3):281–99. doi:10.1016/j.jaerosci.2003.09.003.
- EPA. 2004. Control of emissions of air pollution from nonroad diesel engines and fuel; Final rule. Federal Register. https://www.govinfo.gov/content/pkg/FR-2004-06-29/pdf/04-11293.pdf.
- Hamra, G. B., F. Laden, A. J. Cohen, O. Raaschou-Nielsen, M. Brauer, and D. Loomis. 2015. Lung cancer and exposure to nitrogen dioxide and traffic: A systematic review and meta-analysis. Environ. Health Perspect. 123 (11):1107–12. doi:10.1289/ehp.1408882.
- Hankey, S., G. Lindsey, and J. D. Marshall. 2017. Population-level exposure to particulate air pollution during active travel: Planning for low-exposure, health-promoting cities. Environ. Health Perspect. 125 (4):527–34. doi:10.1289/EHP442.
- Harrison, R. M., A. M. Jones, J. Gietl, J. Yin, and D. C. Green. 2012. Estimation of the contributions of brake dust, tire wear, and resuspension to nonexhaust traffic particles derived from atmospheric measurements. Environ. Sci. Technol. 46 (12):6523–29. doi:10.1021/es300894r.
- Hinds, W. C. 1999. Aerosol technology: Properties, behavior, and measurement of airborne particles. 2nd ed. New York: Wiley.
- Hjortenkrans, D., B. Bergbäck, and A. Häggerud. 2007. Metal emissions from brake linings and tires: Case studies of Stockholm, Sweden 1995/1998 and 2005. Environ. Sci. Technol. 41 (15):5224–30. doi:10.1021/es070198o.
- Huang, S., J. Lawrence, C. Kang, J. Li, M. Martins, P. Vokonas, D. R. Gold, J. Schwartz, B. A. Coull, and P. Koutrakis. 2018. Road proximity influences indoor exposures to ambient fine particle mass and components. Environ. Pollut. 243 (December):978–87. doi:10.1016/j.envpol.2018.09.046.
- Jayarathne, A., P. Egodawatta, G. A. Ayoko, and A. Goonetilleke. 2017. Geochemical phase and particle size relationships of metals in Urban Road Dust. Environ. Pollut. 230 (November):218–26. doi:10.1016/j.envpol.2017.06.059.
- Jayarathne, A., P. Egodawatta, G. A. Ayoko, and A. Goonetilleke. 2018. Assessment of ecological and human health risks of metals in Urban Road Dust based on geochemical fractionation and potential bioavailability. Sci. Total Environ. 635 (September):1609–19. doi:10.1016/j.scitotenv.2018.04.098.
- Kam, W., J. W. Liacos, J. J. Schauer, R. J. Delfino, and C. Sioutas. 2012a. Size-segregated composition of Particulate Matter (PM) in major roadways and surface streets. Atmos. Environ. 55 (August):90–97. doi:10.1016/j.atmosenv.2012.03.028.
- Kam, W., J. W. Liacos, J. J. Schauer, R. J. Delfino, and C. Sioutas. 2012b. On-road emission factors of PM pollutants for Light-Duty Vehicles (LDVs) based on urban street driving conditions. Atmos. Environ. 61 (December):378–86. doi:10.1016/j.atmosenv.2012.07.072.
- Khan, J., M. Ketzel, K. Kakosimos, M. Sørensen, and S. S. Jensen. 2018. Road traffic air and noise pollution exposure assessment – A review of tools and techniques. Sci. Total Environ. 634 (September):661–76. doi:10.1016/j.scitotenv.2018.03.374.
- Khan, R. K., and M. A. Strand. 2018. Road dust and its effect on human health: A literature review. Epidemiol. Health 40 (April):e2018013. doi:10.4178/epih.e2018013.
- Khpalwak, W., W. A. Jadoon, S. M. Abdel-dayem, and H. Sakugawa. 2019. Polycyclic aromatic hydrocarbons in urban road dust, Afghanistan: Implications for human health. Chemosphere 218 (March):517–26. doi:10.1016/j.chemosphere.2018.11.087.
- Kim, J. J., S. Smorodinsky, M. Lipsett, B. C. Singer, A. T. Hodgson, and B. Ostro. 2004. Traffic-related air pollution near busy roads: The East Bay Children’s Respiratory Health Study. Am. J. Respir. Crit. Care Med. 170 (5):520–26. doi:10.1164/rccm.200403-281OC.
- Kozawa, K. H., S. S. Park, S. L. Mara, and J. D. Herner. 2014. Verifying emission reductions from heavy-duty diesel trucks operating on Southern California freeways. Environ. Sci. Technol. 48 (3):1475–83. doi:10.1021/es4044177.
- Larson, T., T. Gould, E. A. Riley, E. Austin, J. Fintzi, L. Sheppard, M. Yost, and C. Simpson. 2017. Ambient air quality measurements from a continuously moving mobile platform: Estimation of area-wide, fuel-based, mobile source emission factors using absolute principal component scores. Atmos. Environ. 152 (March):201–11. doi:10.1016/j.atmosenv.2016.12.037.
- Lawrence, J., J. M. Wolfson, S. Ferguson, P. Koutrakis, and J. Godleski. 2004. Performance stability of the Harvard ambient particle concentrator. Aerosol Sci. Technol. 38 (3):219–27. doi:10.1080/02786820490261735.
- Lee, H., H. Chon, M. Sager, and L. Marton. 2012. Platinum pollution in road dusts, roadside soils, and tree barks in Seoul, Korea. Environ. Geochem. Health 34 (S1):5–12. doi:10.1007/s10653-011-9403-5.
- Li, W., Y. Shi, L. Gao, C. Wu, J. Liu, and Y. Cai. 2018. Occurrence, distribution and risk of organophosphate esters in Urban Road Dust in Beijing, China. Environ. Pollut. 241 (October):566–75. doi:10.1016/j.envpol.2018.05.092.
- Liu, E., T. Yan, G. Birch, and Y. Zhu. 2014. Pollution and health risk of potentially toxic metals in Urban Road Dust in Nanjing, a Mega-City of China. Sci. Total Environ. 476–477 (April):522–31. doi:10.1016/j.scitotenv.2014.01.055.
- Loo, B. W., and C. P. Cork. 1988. Development of high efficiency virtual impactors. Aerosol Sci. Technol. 9 (3):167–76. doi:10.1080/02786828808959205.
- Lorenzi, D., J. A. Entwistle, M. Cave, and J. R. Dean. 2011. Determination of polycyclic aromatic hydrocarbons in Urban Street Dust: Implications for human health. Chemosphere 83 (7):970–77. doi:10.1016/j.chemosphere.2011.02.020.
- Men, C., R. Liu, F. Xu, Q. Wang, L. Guo, and Z. Shen. 2018. Pollution characteristics, risk assessment, and source apportionment of heavy metals in road dust in Beijing, China. Sci. Total Environ. 612 (January):138–47. doi:10.1016/j.scitotenv.2017.08.123.
- Pan, H., X. Lu, and K. Lei. 2017. A comprehensive analysis of heavy metals in Urban Road Dust of Xi’an, China: Contamination, source apportionment and spatial distribution. Sci. Total Environ. 609 (December):1361–69. doi:10.1016/j.scitotenv.2017.08.004.
- Pant, P., and R. M. Harrison. 2013. Estimation of the contribution of road traffic emissions to particulate matter concentrations from field measurements: A review. Atmos. Environ. 77 (October):78–97. doi:10.1016/j.atmosenv.2013.04.028.
- Potgieter-Vermaak, S., G. Rotondo, V. Novakovic, S. Rollins, and R. Van Grieken. 2012. Component-specific toxic concerns of the inhalable fraction of Urban Road Dust. Environ. Geochem. Health 34 (6):689–96. doi:10.1007/s10653-012-9488-5.
- Puett, R. C., J. E. Hart, J. D. Yanosky, D. Spiegelman, M. Wang, J. A. Fisher, B. Hong, and F. Laden. 2014. Particulate matter air pollution exposure, distance to road, and incident lung cancer in the Nurses’ Health Study Cohort. Environ. Health Perspect. 122 (9):926–32. doi:10.1289/ehp.1307490.
- Riley, E. A., L. Banks, J. Fintzi, T. R. Gould, K. Hartin, L. Schaal, M. Davey, L. Sheppard, T. Larson, M. G. Yost, and C. D. Simpson. 2014. Multi-pollutant mobile platform measurements of air pollutants adjacent to a major roadway. Atmos. Environ. 98 (December):492–99. doi:10.1016/j.atmosenv.2014.09.018.
- Riley, E. A., L. Schaal, M. Sasakura, R. Crampton, T. R. Gould, K. Hartin, L. Sheppard, T. Larson, C. D. Simpson, and M. G. Yost. 2016. Correlations between short-term mobile monitoring and long-term passive sampler measurements of traffic-related air pollution. Atmos. Environ. 132 (May):229–39. doi:10.1016/j.atmosenv.2016.03.001.
- Salcedo, D., A. Laskin, V. Shutthanandan, and J. L. Jimenez. 2012. Feasibility of the detection of trace elements in particulate matter using online high-resolution aerosol mass spectrometry. Aerosol Sci. Technol. 46 (11):1187–200. doi:10.1080/02786826.2012.701354.
- Shairsingh, K. K., C. Jeong, J. M. Wang, and G. J. Evans. 2018. Characterizing the spatial variability of local and background concentration signals for air pollution at the neighbourhood scale. Atmos. Environ. 183 (June):57–68. doi:10.1016/j.atmosenv.2018.04.010.
- Shi, Y., K. K. Lau, and E. Ng. 2016. Developing street-level PM 2.5 and PM 10 land use regression models in high-density Hong Kong with urban morphological factors. Environ. Sci. Technol. 50 (15):8178–87. doi:10.1021/acs.est.6b01807.
- Sioutas, C., P. Koutrakis, J. J. Godleski, S. T. Ferguson, C. S. Kim, and R. M. Burton. 1997. Fine particle concentrators for inhalation exposures—Effect of particle size and composition. J. Aerosol Sci. 28 (6):1057–71. doi:10.1016/S0021-8502(96)00493-4.
- Soltani, N., B. Keshavarzi, F. Moore, T. Tavakol, A. R. Lahijanzadeh, N. Jaafarzadeh, and M. Kermani. 2015. Ecological and human health hazards of heavy metals and Polycyclic Aromatic Hydrocarbons (PAHs) in road dust of Isfahan Metropolis, Iran. Sci. Total Environ. 505 (February):712–23. doi:10.1016/j.scitotenv.2014.09.097.
- Tao, L., K. Sun, D. J. Miller, D. Pan, L. M. Golston, and M. A. Zondlo. 2015. Low-power, open-path mobile sensing platform for high-resolution measurements of greenhouse gases and air pollutants. Appl. Phys. B 119 (1):153–64. doi:10.1007/s00340-015-6069-1.
- Tessum, M. W., T. Larson, T. R. Gould, C. D. Simpson, M. G. Yost, and S. Vedal. 2018. Mobile and fixed-site measurements to identify spatial distributions of traffic-related pollution sources in Los Angeles. Environ. Sci. Technol. 52 (5):2844–53. doi:10.1021/acs.est.7b04889.
- Thorpe, A., and R. M. Harrison. 2008. Sources and properties of non-exhaust particulate matter from road traffic: A review. Sci. Total Environ. 400 (1–3):270–82. doi:10.1016/j.scitotenv.2008.06.007.
- Valotto, G., G. Rampazzo, F. Visin, F. Gonella, E. Cattaruzza, A. Glisenti, G. Formenton, and P. Tieppo. 2015. Environmental and traffic-related parameters affecting road dust composition: A multi-technique approach applied to Venice Area (Italy). Atmos. Environ. 122 (December):596–608. doi:10.1016/j.atmosenv.2015.10.006.
- Westerdahl, D., S. Fruin, T. Sax, P. M. Fine, and C. Sioutas. 2005. Mobile platform measurements of ultrafine particles and associated pollutant concentrations on freeways and residential streets in Los Angeles. Atmos. Environ. 39 (20):3597–610. doi:10.1016/j.atmosenv.2005.02.034.
- Xu, S., N. Zheng, J. Liu, Y. Wang, and S. Chang. 2013. Geochemistry and health risk assessment of arsenic exposure to street dust in the Zinc Smelting District, Northeast China. Environ. Geochem. Health 35 (1):89–99. doi:10.1007/s10653-012-9463-1.
- Yu, B., X. Xie, L. Q. Ma, H. Kan, and Q. Zhou. 2014. Source, distribution, and health risk assessment of polycyclic aromatic hydrocarbons in Urban Street Dust from Tianjin, China. Environ. Sci. Pollut. Res. 21 (4):2817–25. doi:10.1007/s11356-013-2190-z.
- Zhang, J., X. Zhang, L. Wu, T. Wang, J. Zhao, Y. Zhang, Z. Men, and H. Mao. 2018. Occurrence of benzothiazole and its derivates in tire wear, road dust, and roadside soil. Chemosphere 201:310–17. doi:10.1016/j.chemosphere.2018.03.007.
- Zhang, K., and S. Batterman. 2013. Air pollution and health risks due to vehicle traffic. Sci. Total Environ. 450–451 (April):307–16. doi:10.1016/j.scitotenv.2013.01.074.